Enhancing drug discovery efficiency is a critical objective in the pharmaceutical industry, driven by the need to accelerate the development of new therapies while reducing costs and improving success rates. Traditional drug discovery methods, often characterized by lengthy timelines and high attrition rates, are being revolutionized by innovative approaches that leverage cutting-edge technologies and interdisciplinary collaboration. Advances in computational modeling, artificial intelligence, and machine learning are enabling researchers to predict drug-target interactions with unprecedented accuracy, streamlining the identification of promising compounds. Additionally, high-throughput screening and automated laboratory systems are expediting the testing process, allowing for the rapid evaluation of vast chemical libraries. The integration of big data analytics and systems biology is further enhancing our understanding of complex biological systems, facilitating the identification of novel therapeutic targets. By embracing these innovative strategies, the pharmaceutical industry is poised to transform drug discovery, ultimately leading to more effective treatments reaching patients faster and more efficiently.
Leveraging Artificial Intelligence in Drug Discovery
The integration of artificial intelligence (AI) into drug discovery processes marks a transformative era in pharmaceutical research, offering unprecedented opportunities to enhance efficiency and precision. Traditionally, drug discovery has been a time-consuming and costly endeavor, often taking over a decade and billions of dollars to bring a new drug to market. However, the advent of AI technologies is poised to revolutionize this landscape by streamlining various stages of drug development, from initial target identification to clinical trials.
One of the primary ways AI is enhancing drug discovery is through its ability to analyze vast datasets with remarkable speed and accuracy. In the early stages of drug development, identifying potential drug targets is crucial. AI algorithms can sift through genomic, proteomic, and metabolomic data to identify novel targets that may not be apparent through conventional methods. By employing machine learning techniques, researchers can predict the interactions between drugs and their targets, thereby reducing the likelihood of failure in later stages of development.
Moreover, AI is instrumental in the design and optimization of drug candidates. Through deep learning models, AI can predict the pharmacokinetic and pharmacodynamic properties of compounds, allowing researchers to optimize their chemical structures for better efficacy and safety profiles. This capability significantly reduces the need for extensive trial-and-error experimentation, thus accelerating the lead optimization process. Additionally, AI-driven platforms can simulate complex biological systems, providing insights into the potential effects of drug candidates on human physiology, which is invaluable for assessing their therapeutic potential.
Transitioning from preclinical to clinical phases, AI continues to play a pivotal role. In clinical trial design, AI can analyze patient data to identify suitable candidates for trials, ensuring a more targeted and efficient recruitment process. By leveraging AI, researchers can also monitor patient responses in real-time, enabling adaptive trial designs that can be modified based on interim results. This adaptability not only enhances the likelihood of trial success but also reduces costs and timeframes associated with traditional trial methodologies.
Furthermore, AI’s predictive analytics capabilities are invaluable in assessing drug safety and efficacy. By analyzing historical data from previous trials and real-world evidence, AI can identify potential adverse effects and efficacy signals earlier in the development process. This proactive approach allows for better risk management and decision-making, ultimately leading to safer and more effective therapeutic options for patients.
Despite these advancements, the integration of AI in drug discovery is not without challenges. Ensuring the quality and integrity of data used in AI models is paramount, as biased or incomplete data can lead to erroneous predictions. Additionally, the interpretability of AI models remains a concern, as the “black box” nature of some algorithms can obscure the rationale behind their predictions. Addressing these challenges requires ongoing collaboration between AI experts, biologists, and regulatory bodies to establish standards and best practices.
In conclusion, the application of artificial intelligence in drug discovery holds immense promise for enhancing the efficiency and effectiveness of developing new therapeutics. By leveraging AI’s capabilities in data analysis, drug design, clinical trial optimization, and safety assessment, the pharmaceutical industry can overcome many of the traditional barriers to drug development. As AI technologies continue to evolve, their integration into drug discovery processes will undoubtedly lead to more rapid and cost-effective solutions, ultimately benefiting patients worldwide.
Accelerating Drug Development with High-Throughput Screening
In the rapidly evolving field of pharmaceuticals, the demand for more efficient drug discovery processes has never been greater. High-throughput screening (HTS) has emerged as a pivotal technology in accelerating drug development, offering a transformative approach to identifying potential therapeutic candidates. By enabling the simultaneous testing of thousands to millions of compounds, HTS significantly reduces the time and resources traditionally required in the early stages of drug discovery. This innovative approach not only enhances the efficiency of identifying promising drug candidates but also increases the likelihood of discovering novel compounds with therapeutic potential.
The integration of HTS into drug discovery pipelines marks a significant departure from conventional methods, which often relied on labor-intensive and time-consuming processes. Traditional drug discovery typically involved the sequential testing of compounds, a method that was not only slow but also limited in scope. In contrast, HTS leverages automation and miniaturization technologies to conduct large-scale screenings rapidly. This shift allows researchers to explore vast chemical libraries, thereby broadening the scope of potential drug candidates and increasing the chances of finding effective treatments for complex diseases.
Moreover, the application of HTS is not limited to the initial stages of drug discovery. It plays a crucial role in various phases, including target identification, lead optimization, and toxicity assessment. By providing a comprehensive analysis of compound interactions with biological targets, HTS facilitates the identification of lead compounds with desirable pharmacological profiles. This capability is particularly valuable in the context of precision medicine, where the goal is to develop targeted therapies that address specific molecular pathways involved in disease progression.
In addition to its role in identifying lead compounds, HTS contributes to the optimization of drug candidates by enabling the rapid assessment of structure-activity relationships. This process involves evaluating how modifications to a compound’s chemical structure affect its biological activity, thereby guiding the refinement of drug candidates to enhance efficacy and reduce potential side effects. The ability to quickly iterate and optimize compounds is a critical advantage in the competitive landscape of drug development, where time-to-market is a key determinant of success.
Furthermore, the integration of advanced data analytics and machine learning algorithms with HTS has further enhanced its capabilities. These technologies enable the analysis of complex datasets generated by HTS, uncovering patterns and insights that may not be immediately apparent through traditional analysis methods. By leveraging these insights, researchers can make more informed decisions about which compounds to prioritize for further development, ultimately streamlining the drug discovery process.
Despite its numerous advantages, the implementation of HTS is not without challenges. The high cost of equipment and the need for specialized expertise can be barriers to entry for some research institutions. However, ongoing advancements in technology and the increasing availability of shared resources are helping to mitigate these challenges, making HTS more accessible to a broader range of researchers.
In conclusion, high-throughput screening represents a paradigm shift in drug discovery, offering a powerful tool for accelerating the development of new therapeutics. By enabling the rapid and efficient screening of vast chemical libraries, HTS enhances the ability of researchers to identify and optimize promising drug candidates. As technology continues to advance, the integration of HTS with other innovative approaches will undoubtedly play a crucial role in shaping the future of drug development, ultimately leading to more effective treatments for patients worldwide.
Utilizing Big Data Analytics for Predictive Modeling in Drug Discovery
In the rapidly evolving field of drug discovery, the integration of big data analytics has emerged as a transformative approach, offering unprecedented opportunities to enhance efficiency and accuracy. As the pharmaceutical industry grapples with the challenges of high costs and lengthy timelines associated with traditional drug development processes, leveraging big data for predictive modeling presents a promising solution. This innovative approach not only accelerates the identification of potential drug candidates but also improves the precision of predicting their efficacy and safety profiles.
To begin with, the vast amounts of data generated from various sources, such as genomic studies, clinical trials, and electronic health records, provide a rich repository of information that can be harnessed for drug discovery. By employing advanced data analytics techniques, researchers can sift through these extensive datasets to identify patterns and correlations that may not be immediately apparent through conventional methods. This capability is particularly valuable in the early stages of drug discovery, where understanding the complex interactions between biological targets and potential drug compounds is crucial.
Moreover, predictive modeling, powered by big data analytics, enables researchers to simulate and predict the behavior of drug candidates in biological systems. This is achieved through sophisticated algorithms and machine learning techniques that analyze historical data to forecast outcomes. For instance, predictive models can assess the likelihood of a compound’s success in clinical trials by evaluating its pharmacokinetics and pharmacodynamics properties. Consequently, this reduces the reliance on costly and time-consuming experimental procedures, allowing for a more streamlined and cost-effective drug development process.
In addition to enhancing the efficiency of drug discovery, big data analytics also plays a pivotal role in improving the safety and efficacy of new drugs. By integrating data from diverse sources, such as adverse event reports and patient demographics, predictive models can identify potential safety concerns and adverse reactions early in the development process. This proactive approach not only mitigates risks but also informs the design of clinical trials, ensuring that they are more targeted and effective.
Furthermore, the application of big data analytics in drug discovery is not limited to the identification and validation of drug candidates. It also extends to the optimization of drug formulations and delivery mechanisms. By analyzing data on patient responses and treatment outcomes, researchers can refine drug formulations to enhance their therapeutic effects and minimize side effects. This iterative process of data-driven optimization is instrumental in developing personalized medicine approaches, where treatments are tailored to the individual characteristics of patients.
Despite the significant advantages offered by big data analytics in drug discovery, it is important to acknowledge the challenges associated with its implementation. The integration of disparate data sources, the need for robust data governance frameworks, and the ethical considerations surrounding data privacy are critical issues that must be addressed. Nevertheless, ongoing advancements in data science and technology continue to pave the way for overcoming these obstacles, ensuring that the full potential of big data analytics can be realized in the realm of drug discovery.
In conclusion, the utilization of big data analytics for predictive modeling represents a paradigm shift in the drug discovery process. By enabling more efficient and accurate identification of drug candidates, improving safety and efficacy assessments, and facilitating personalized medicine approaches, this innovative approach holds the promise of transforming the pharmaceutical industry. As researchers and industry stakeholders continue to embrace and refine these techniques, the future of drug discovery looks increasingly promising, with the potential to deliver more effective and safer treatments to patients worldwide.
Integrating Machine Learning for Target Identification and Validation
In recent years, the integration of machine learning into the field of drug discovery has emerged as a transformative approach, particularly in the areas of target identification and validation. This innovative methodology is reshaping traditional paradigms, offering unprecedented opportunities to enhance efficiency and accuracy in the early stages of drug development. As the pharmaceutical industry grapples with the challenges of high costs and lengthy timelines associated with bringing new drugs to market, machine learning presents a promising solution to streamline these processes.
Machine learning, a subset of artificial intelligence, involves the use of algorithms and statistical models to analyze and interpret complex data sets. In the context of drug discovery, it enables researchers to sift through vast amounts of biological data to identify potential drug targets—proteins or genes that play a critical role in disease progression. By leveraging machine learning, scientists can predict which targets are most likely to respond to therapeutic intervention, thereby prioritizing them for further investigation. This predictive capability is particularly valuable given the sheer volume of potential targets and the limited resources available for experimental validation.
Moreover, machine learning facilitates the validation of identified targets by analyzing patterns and correlations within biological data that may not be immediately apparent through traditional methods. For instance, machine learning algorithms can integrate diverse data types, such as genomic, proteomic, and phenotypic information, to construct comprehensive models of disease mechanisms. These models can then be used to simulate the effects of modulating specific targets, providing insights into their potential therapeutic value. Consequently, this approach not only accelerates the validation process but also enhances the reliability of the findings, reducing the likelihood of pursuing non-viable targets.
Transitioning from traditional methods to machine learning-driven approaches requires a paradigm shift in how data is utilized in drug discovery. Historically, target identification and validation have relied heavily on experimental techniques, which, while effective, are often time-consuming and resource-intensive. Machine learning, on the other hand, offers a data-driven alternative that complements these traditional methods. By automating the analysis of large-scale data sets, machine learning reduces the need for extensive laboratory experimentation, allowing researchers to focus their efforts on the most promising targets.
Furthermore, the integration of machine learning into drug discovery is facilitated by advancements in computational power and the availability of large, high-quality data sets. The proliferation of omics technologies, such as genomics and proteomics, has generated a wealth of data that can be harnessed by machine learning algorithms to uncover novel insights into disease biology. Additionally, collaborative efforts between academia, industry, and technology companies are fostering the development of sophisticated machine learning tools tailored specifically for drug discovery applications.
Despite its potential, the application of machine learning in target identification and validation is not without challenges. Ensuring the quality and relevance of input data is critical, as inaccuracies can lead to erroneous predictions. Moreover, the interpretability of machine learning models remains a concern, as complex algorithms can sometimes produce results that are difficult to understand and validate experimentally. Addressing these challenges requires ongoing collaboration between data scientists and domain experts to refine algorithms and ensure their applicability to real-world scenarios.
In conclusion, the integration of machine learning into drug discovery represents a significant advancement in the quest to enhance efficiency and accuracy in target identification and validation. By harnessing the power of data-driven insights, this approach holds the promise of accelerating the development of new therapeutics, ultimately benefiting patients and the healthcare system as a whole. As the field continues to evolve, ongoing innovation and collaboration will be essential to fully realize the potential of machine learning in transforming drug discovery.
Streamlining Drug Discovery with Automated Laboratory Technologies
In recent years, the field of drug discovery has witnessed a transformative shift, driven by the integration of automated laboratory technologies. These innovations are not only enhancing the efficiency of drug discovery processes but also significantly reducing the time and cost associated with bringing new therapeutics to market. As the pharmaceutical industry grapples with the challenges of developing effective drugs in a timely manner, automated technologies offer a promising solution to streamline various stages of drug discovery.
To begin with, automated laboratory technologies have revolutionized the initial phase of drug discovery, which involves high-throughput screening (HTS) of chemical compounds. Traditionally, this process required extensive manual labor and was prone to human error, leading to inefficiencies and delays. However, with the advent of robotic systems and advanced software, laboratories can now screen thousands of compounds simultaneously with remarkable precision. This not only accelerates the identification of potential drug candidates but also enhances the accuracy of the results, thereby increasing the likelihood of success in subsequent stages.
Moreover, the integration of artificial intelligence (AI) and machine learning (ML) into automated laboratory technologies has further amplified their impact. AI algorithms can analyze vast datasets generated during HTS, identifying patterns and predicting the efficacy of compounds with unprecedented speed and accuracy. This capability allows researchers to prioritize the most promising candidates for further development, optimizing resource allocation and minimizing the risk of pursuing ineffective compounds. Consequently, AI-driven automation is becoming an indispensable tool in the quest for novel therapeutics.
In addition to HTS, automated laboratory technologies are also streamlining the drug development process through enhanced data management and analysis. The sheer volume of data generated during drug discovery can be overwhelming, but automated systems equipped with sophisticated data analytics tools can efficiently organize, process, and interpret this information. By providing researchers with actionable insights, these technologies facilitate informed decision-making and enable a more targeted approach to drug development. This not only expedites the discovery process but also improves the overall quality of the resulting therapeutics.
Furthermore, automated laboratory technologies are playing a crucial role in optimizing the synthesis and testing of drug candidates. Automated synthesis platforms can rapidly produce a wide array of chemical compounds, allowing researchers to explore diverse chemical spaces and identify optimal structures for drug development. Similarly, automated testing systems can conduct a range of biological assays with high precision and reproducibility, ensuring that only the most promising candidates advance to clinical trials. By reducing the time and resources required for these critical tasks, automation is paving the way for more efficient and cost-effective drug discovery.
Despite the numerous advantages of automated laboratory technologies, it is important to acknowledge the challenges associated with their implementation. The initial investment in automation infrastructure can be substantial, and there is a learning curve associated with integrating these technologies into existing workflows. However, the long-term benefits, including increased efficiency, reduced costs, and improved success rates, make automation a worthwhile endeavor for forward-thinking pharmaceutical companies.
In conclusion, the integration of automated laboratory technologies into drug discovery processes is revolutionizing the pharmaceutical industry. By enhancing the efficiency and accuracy of high-throughput screening, data management, synthesis, and testing, these innovations are streamlining the path from discovery to market. As the industry continues to embrace automation, the potential for developing novel therapeutics more rapidly and cost-effectively becomes increasingly attainable, promising a brighter future for drug discovery and patient care.
Enhancing Drug Design with Computational Chemistry Techniques
In the rapidly evolving field of drug discovery, the integration of computational chemistry techniques has emerged as a transformative approach, significantly enhancing the efficiency and precision of drug design. As pharmaceutical companies and research institutions strive to develop new therapeutics, the traditional methods of drug discovery, which often involve labor-intensive and time-consuming processes, are increasingly being supplemented and, in some cases, replaced by computational strategies. These innovative approaches not only accelerate the discovery process but also improve the accuracy of predicting drug behavior, thereby reducing the likelihood of costly failures in later stages of development.
One of the primary advantages of computational chemistry in drug design is its ability to model and simulate molecular interactions at an atomic level. This capability allows researchers to predict how potential drug candidates will interact with their target proteins, providing valuable insights into their efficacy and safety profiles. By employing techniques such as molecular docking and molecular dynamics simulations, scientists can virtually screen vast libraries of compounds, identifying those with the highest potential for success. This virtual screening process significantly narrows down the number of compounds that need to be synthesized and tested in the laboratory, thereby saving both time and resources.
Moreover, computational chemistry techniques facilitate the optimization of lead compounds. Once a promising candidate is identified, these methods can be used to refine its structure, enhancing its binding affinity and selectivity for the target protein. Quantum mechanical calculations, for instance, provide detailed information about the electronic properties of molecules, enabling researchers to make precise modifications that improve drug performance. This iterative process of design and optimization is crucial in developing drugs that are not only effective but also have favorable pharmacokinetic and pharmacodynamic properties.
In addition to improving the design of individual drug candidates, computational chemistry also plays a pivotal role in understanding complex biological systems. Systems biology approaches, which integrate computational models with experimental data, allow researchers to explore the intricate networks of interactions within cells. By simulating these networks, scientists can identify novel drug targets and gain insights into the mechanisms of disease, paving the way for the development of more targeted and personalized therapies. This holistic understanding of biological systems is particularly important in the context of complex diseases such as cancer and neurodegenerative disorders, where multiple pathways may be involved.
Furthermore, the advent of machine learning and artificial intelligence has further enhanced the capabilities of computational chemistry in drug discovery. These technologies can analyze vast datasets, identifying patterns and correlations that may not be apparent through traditional methods. Machine learning algorithms can predict the activity of new compounds, guide the design of experiments, and even suggest novel chemical structures with desired properties. As these technologies continue to evolve, they hold the promise of revolutionizing the drug discovery process, making it faster, more efficient, and more cost-effective.
In conclusion, the integration of computational chemistry techniques into drug design represents a significant advancement in the field of drug discovery. By enabling the rapid screening and optimization of drug candidates, providing insights into complex biological systems, and leveraging the power of artificial intelligence, these innovative approaches are transforming the way new therapeutics are developed. As researchers continue to refine these techniques and explore their full potential, the future of drug discovery looks increasingly promising, with the potential to deliver safer and more effective treatments to patients worldwide.
Q&A
1. **What is the role of AI in enhancing drug discovery efficiency?**
AI accelerates drug discovery by analyzing vast datasets to identify potential drug candidates, predict their efficacy, and optimize drug design, significantly reducing time and cost.
2. **How does high-throughput screening contribute to drug discovery?**
High-throughput screening allows researchers to quickly test thousands of compounds for biological activity, identifying promising candidates for further development.
3. **What innovative approach is used to improve target identification in drug discovery?**
CRISPR-Cas9 gene editing is used to identify and validate drug targets by enabling precise manipulation of genes, helping to understand their role in disease.
4. **How do computational models aid in drug discovery?**
Computational models simulate biological processes and drug interactions, providing insights into drug behavior and helping to predict outcomes, thus guiding experimental efforts.
5. **What is the significance of personalized medicine in drug discovery?**
Personalized medicine tailors drug development to individual genetic profiles, improving efficacy and reducing adverse effects, leading to more effective treatments.
6. **How does collaboration enhance drug discovery efficiency?**
Collaboration between academia, industry, and regulatory bodies fosters knowledge sharing, resource pooling, and innovation, accelerating the drug discovery process.Enhancing drug discovery efficiency through innovative approaches is crucial for accelerating the development of new therapeutics and addressing unmet medical needs. By integrating cutting-edge technologies such as artificial intelligence, machine learning, and high-throughput screening, researchers can streamline the identification and optimization of potential drug candidates. These approaches enable the analysis of vast datasets, prediction of drug-target interactions, and simulation of biological processes, significantly reducing the time and cost associated with traditional drug discovery methods. Additionally, leveraging advancements in genomics and personalized medicine allows for the design of more targeted and effective treatments. Collaborative efforts across academia, industry, and regulatory bodies are essential to foster innovation and ensure the successful translation of these approaches into clinical practice. Ultimately, these advancements hold the promise of delivering safer, more effective drugs to patients faster, revolutionizing the pharmaceutical landscape and improving global health outcomes.
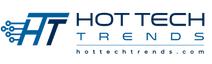