Data enrichment is a critical process in the realm of data management and analytics, aimed at enhancing the quality and value of raw data by supplementing it with additional information. As organizations increasingly rely on data-driven insights to inform strategic decisions, the need for comprehensive and accurate datasets has never been more paramount. Effective data enrichment strategies can transform incomplete or outdated data into a robust asset, enabling businesses to gain deeper insights, improve customer experiences, and drive operational efficiencies. Key strategies for successful data enrichment include leveraging third-party data sources, employing machine learning algorithms for predictive enrichment, integrating real-time data updates, and ensuring data quality through rigorous validation processes. By adopting these strategies, organizations can unlock the full potential of their data, leading to more informed decision-making and a competitive edge in the marketplace.
Understanding Data Enrichment: Key Concepts and Benefits
Data enrichment is a crucial process in the realm of data management, aimed at enhancing the quality and utility of raw data by supplementing it with additional information. This process transforms basic data into a more valuable asset, enabling organizations to gain deeper insights and make more informed decisions. Understanding the key concepts and benefits of data enrichment is essential for businesses seeking to leverage their data effectively.
At its core, data enrichment involves integrating external data sources with internal datasets to fill in gaps, correct inaccuracies, and provide a more comprehensive view of the information at hand. This can include appending demographic details, geographic information, or behavioral data to existing records. By doing so, organizations can create a more complete and accurate picture of their customers, products, or operations. Consequently, this enriched data becomes a powerful tool for driving strategic initiatives and achieving business objectives.
One of the primary benefits of data enrichment is the enhancement of customer profiles. By enriching customer data, businesses can gain a deeper understanding of their target audience, allowing for more personalized marketing efforts and improved customer experiences. For instance, by appending demographic information such as age, income, or location, companies can tailor their marketing campaigns to better resonate with specific segments of their audience. This targeted approach not only increases the effectiveness of marketing efforts but also fosters stronger customer relationships.
Moreover, data enrichment plays a pivotal role in improving data accuracy and reliability. Inaccurate or incomplete data can lead to misguided decisions and missed opportunities. By incorporating additional data points, organizations can identify and rectify errors, ensuring that their data is both accurate and up-to-date. This, in turn, enhances the credibility of the data and the decisions based on it. Furthermore, enriched data can help in identifying patterns and trends that may not be apparent in raw data, providing valuable insights that can inform strategic planning and operational improvements.
In addition to enhancing customer profiles and improving data accuracy, data enrichment also facilitates better segmentation and targeting. By leveraging enriched data, businesses can segment their audience more effectively, identifying distinct groups with shared characteristics or behaviors. This enables more precise targeting, ensuring that marketing messages reach the right audience at the right time. As a result, organizations can optimize their marketing spend and achieve higher conversion rates.
Transitioning from understanding the benefits to implementing data enrichment strategies, it is important to consider the various methods available. One common approach is to partner with third-party data providers who specialize in supplying high-quality external data. These providers can offer a wealth of information that can be seamlessly integrated with existing datasets. Additionally, organizations can utilize machine learning algorithms to automate the enrichment process, identifying relevant data points and appending them to internal records.
In conclusion, data enrichment is a vital process that enhances the value of raw data by supplementing it with additional information. By understanding the key concepts and benefits of data enrichment, organizations can unlock the full potential of their data, leading to more informed decision-making and improved business outcomes. Whether through enhancing customer profiles, improving data accuracy, or facilitating better segmentation and targeting, data enrichment offers a multitude of advantages that can drive success in today’s data-driven world.
Leveraging Machine Learning for Enhanced Data Enrichment
In the rapidly evolving landscape of data management, leveraging machine learning for enhanced data enrichment has emerged as a pivotal strategy for organizations seeking to maximize the value of their data assets. As businesses increasingly rely on data-driven insights to inform decision-making, the need for comprehensive and accurate data becomes paramount. Machine learning, with its ability to process vast amounts of information and identify patterns, offers a powerful tool for enriching data in ways that were previously unimaginable.
To begin with, machine learning algorithms can significantly enhance data enrichment by automating the process of data cleansing. Data cleansing is a critical step in ensuring that datasets are free from errors, inconsistencies, and redundancies. Traditional methods of data cleansing often involve manual intervention, which can be time-consuming and prone to human error. However, machine learning models can be trained to identify and correct anomalies in data with remarkable precision, thereby improving the overall quality of the dataset. This automated approach not only saves time but also ensures a higher degree of accuracy, which is essential for reliable data analysis.
Furthermore, machine learning facilitates the integration of disparate data sources, a process that is crucial for comprehensive data enrichment. Organizations often collect data from various channels, including customer interactions, social media, and transactional records. Integrating these diverse datasets can be challenging due to differences in format, structure, and semantics. Machine learning algorithms can be employed to harmonize these datasets by mapping and aligning them into a unified format. This integration enables organizations to gain a holistic view of their data, uncovering insights that would otherwise remain hidden in isolated data silos.
In addition to data cleansing and integration, machine learning plays a vital role in enhancing data enrichment through predictive analytics. By analyzing historical data, machine learning models can identify trends and patterns that inform future predictions. This capability is particularly valuable for businesses looking to anticipate customer behavior, market trends, or operational challenges. Predictive analytics not only enriches the data by adding a forward-looking dimension but also empowers organizations to make proactive decisions based on data-driven forecasts.
Moreover, machine learning can enhance data enrichment by enabling real-time data processing. In today’s fast-paced business environment, the ability to process and analyze data in real-time is a competitive advantage. Machine learning algorithms can be deployed to continuously monitor data streams, providing instant insights and enabling organizations to respond swiftly to emerging opportunities or threats. This real-time enrichment ensures that decision-makers have access to the most current and relevant information, thereby enhancing the agility and responsiveness of the organization.
Finally, it is important to acknowledge the role of machine learning in personalizing data enrichment. As organizations strive to deliver personalized experiences to their customers, machine learning can be used to tailor data enrichment processes to individual preferences and behaviors. By analyzing customer data, machine learning models can identify unique patterns and preferences, allowing organizations to customize their offerings and interactions. This personalized approach not only enriches the data but also enhances customer satisfaction and loyalty.
In conclusion, leveraging machine learning for enhanced data enrichment offers a multitude of benefits, from improving data quality and integration to enabling predictive analytics and real-time processing. As organizations continue to navigate the complexities of the digital age, adopting machine learning strategies for data enrichment will be essential for unlocking the full potential of their data assets and gaining a competitive edge in the marketplace.
Best Practices for Integrating Third-Party Data Sources
In the realm of data-driven decision-making, the integration of third-party data sources has become a pivotal strategy for organizations seeking to enhance their data enrichment processes. As businesses strive to gain a competitive edge, the ability to effectively incorporate external data into existing datasets can provide valuable insights and drive more informed decisions. To achieve this, it is essential to adopt best practices that ensure seamless integration and maximize the potential of third-party data.
One of the foremost strategies for effective data enrichment is to clearly define the objectives and goals of integrating third-party data. By establishing a clear understanding of what the organization aims to achieve, whether it be improving customer segmentation, enhancing predictive analytics, or refining marketing strategies, businesses can better identify the types of external data that will be most beneficial. This clarity of purpose not only streamlines the selection process but also ensures that the data acquired aligns with the organization’s overarching objectives.
Once the objectives are set, the next step involves the careful selection of third-party data sources. It is crucial to evaluate potential data providers based on the quality, relevance, and reliability of their data. Organizations should conduct thorough due diligence, assessing the provider’s reputation, data collection methodologies, and compliance with data privacy regulations. By choosing reputable sources, businesses can mitigate the risks associated with inaccurate or outdated data, thereby enhancing the overall quality of their enriched datasets.
In addition to selecting high-quality data sources, organizations must also focus on data integration techniques that facilitate seamless merging of external data with internal datasets. Employing advanced data integration tools and technologies can significantly streamline this process. These tools often offer features such as data mapping, transformation, and cleansing, which are essential for ensuring that the integrated data is consistent, accurate, and ready for analysis. Moreover, leveraging automation in data integration can reduce manual errors and increase efficiency, allowing organizations to focus on deriving insights rather than managing data.
Furthermore, maintaining data privacy and security is paramount when integrating third-party data. Organizations must ensure that they comply with relevant data protection regulations, such as the General Data Protection Regulation (GDPR) or the California Consumer Privacy Act (CCPA), to safeguard sensitive information. Implementing robust data governance frameworks and encryption protocols can help protect data integrity and prevent unauthorized access. By prioritizing data security, businesses not only protect themselves from potential legal repercussions but also build trust with their customers and stakeholders.
Another critical aspect of effective data enrichment is continuous monitoring and evaluation of the integrated data. Organizations should establish metrics and key performance indicators (KPIs) to assess the impact of third-party data on their business outcomes. Regularly reviewing these metrics allows businesses to identify any discrepancies or areas for improvement, ensuring that the data remains relevant and valuable over time. Additionally, feedback loops can be established to refine data integration processes, enabling organizations to adapt to changing business needs and emerging data sources.
In conclusion, integrating third-party data sources into existing datasets is a powerful strategy for data enrichment, offering organizations the opportunity to gain deeper insights and make more informed decisions. By clearly defining objectives, selecting reputable data providers, employing advanced integration techniques, ensuring data privacy, and continuously monitoring outcomes, businesses can effectively harness the potential of external data. As the landscape of data continues to evolve, adopting these best practices will be essential for organizations seeking to maintain a competitive advantage in an increasingly data-driven world.
Ensuring Data Quality and Accuracy in Enrichment Processes
In the realm of data management, ensuring data quality and accuracy during enrichment processes is paramount. Data enrichment, the process of enhancing existing data by adding missing information or correcting inaccuracies, plays a crucial role in transforming raw data into valuable insights. However, the effectiveness of data enrichment hinges on the quality and accuracy of the data being processed. To achieve this, organizations must adopt top strategies that not only enhance data but also maintain its integrity.
To begin with, a foundational strategy is to establish a robust data governance framework. This framework serves as the backbone for all data-related activities, ensuring that data is managed consistently and responsibly across the organization. By defining clear policies and procedures for data enrichment, organizations can maintain high standards of data quality. Moreover, a well-structured governance framework facilitates accountability, as it delineates roles and responsibilities, ensuring that all stakeholders are aligned in their efforts to uphold data accuracy.
In addition to governance, leveraging advanced data validation techniques is essential. These techniques involve the use of algorithms and automated tools to verify the accuracy and consistency of data before and after enrichment. By implementing real-time validation processes, organizations can promptly identify and rectify discrepancies, thereby preventing the propagation of errors throughout the dataset. Furthermore, automated validation reduces the reliance on manual checks, which are often prone to human error, thus enhancing the overall reliability of the data.
Another critical strategy is the integration of high-quality external data sources. Enrichment processes often require supplementary data to fill gaps or provide additional context. However, the quality of these external sources directly impacts the accuracy of the enriched data. Therefore, organizations must meticulously evaluate potential data providers, considering factors such as data accuracy, timeliness, and relevance. By selecting reputable sources, organizations can ensure that the additional data enhances rather than compromises the quality of their datasets.
Moreover, continuous monitoring and auditing of data enrichment processes are vital to maintaining data quality. Regular audits help identify patterns of inaccuracies or inconsistencies, enabling organizations to address underlying issues proactively. Additionally, continuous monitoring allows for the timely detection of anomalies, ensuring that any deviations from expected data quality standards are swiftly corrected. This ongoing vigilance not only safeguards data accuracy but also fosters a culture of continuous improvement within the organization.
Furthermore, fostering collaboration between data scientists, analysts, and domain experts can significantly enhance the quality of data enrichment. Domain experts bring invaluable insights into the nuances of the data, helping to identify potential inaccuracies that may not be apparent to data scientists. By facilitating cross-functional collaboration, organizations can leverage diverse expertise to refine enrichment processes, ultimately leading to more accurate and meaningful data outcomes.
Lastly, investing in employee training and development is crucial for ensuring data quality and accuracy. As data enrichment processes evolve with technological advancements, it is imperative that employees remain adept at utilizing new tools and methodologies. By providing ongoing training opportunities, organizations can equip their workforce with the skills necessary to execute enrichment processes effectively, thereby maintaining high standards of data quality.
In conclusion, ensuring data quality and accuracy in enrichment processes requires a multifaceted approach. By establishing a robust governance framework, leveraging advanced validation techniques, integrating high-quality external sources, and fostering collaboration and continuous improvement, organizations can enhance the effectiveness of their data enrichment efforts. Through these strategies, they can transform raw data into reliable insights, driving informed decision-making and achieving a competitive edge in the data-driven landscape.
Personalization Through Data Enrichment: Strategies for Success
In the contemporary digital landscape, businesses are increasingly recognizing the value of personalization as a means to enhance customer engagement and drive growth. Central to this endeavor is the concept of data enrichment, a process that involves enhancing raw data with additional information to create a more comprehensive customer profile. By leveraging enriched data, companies can tailor their offerings to meet the specific needs and preferences of their customers, thereby fostering a more personalized experience. To achieve this, several strategies can be employed, each contributing to the overall effectiveness of data enrichment efforts.
Firstly, integrating multiple data sources is a fundamental strategy for successful data enrichment. By aggregating data from various channels such as social media, customer interactions, and third-party databases, businesses can obtain a holistic view of their customers. This comprehensive dataset allows for more accurate segmentation and targeting, enabling companies to deliver personalized content and offers that resonate with individual customers. Moreover, the integration of diverse data sources helps in identifying patterns and trends that may not be apparent when analyzing isolated datasets.
In addition to integrating data sources, employing advanced analytics and machine learning techniques is crucial for effective data enrichment. These technologies enable businesses to process large volumes of data efficiently and extract meaningful insights. Machine learning algorithms, for instance, can identify correlations and predict customer behavior, allowing companies to anticipate customer needs and preferences. Consequently, businesses can proactively tailor their marketing strategies and product offerings, thereby enhancing the overall customer experience.
Furthermore, maintaining data quality is essential for the success of data enrichment initiatives. Inaccurate or outdated data can lead to misguided personalization efforts, resulting in a negative customer experience. To mitigate this risk, businesses should implement robust data governance practices, including regular data cleansing and validation processes. By ensuring the accuracy and reliability of their data, companies can make informed decisions and deliver personalized experiences that truly reflect the needs and preferences of their customers.
Another important strategy involves leveraging customer feedback to enrich data. By actively seeking input from customers through surveys, reviews, and direct interactions, businesses can gain valuable insights into customer preferences and expectations. This feedback can be used to refine customer profiles and enhance personalization efforts. Additionally, engaging with customers in this manner demonstrates a commitment to understanding their needs, thereby fostering trust and loyalty.
Moreover, privacy and data security considerations must be at the forefront of any data enrichment strategy. As businesses collect and process vast amounts of personal data, they must ensure compliance with relevant data protection regulations and implement robust security measures to safeguard customer information. By prioritizing privacy and security, companies can build trust with their customers, which is essential for the success of personalization initiatives.
In conclusion, effective data enrichment is a multifaceted process that requires the integration of multiple data sources, the application of advanced analytics, the maintenance of data quality, the incorporation of customer feedback, and a strong focus on privacy and security. By adopting these strategies, businesses can create enriched customer profiles that enable them to deliver personalized experiences, ultimately driving customer satisfaction and loyalty. As the digital landscape continues to evolve, the importance of data enrichment in personalization efforts will only grow, making it imperative for businesses to invest in these strategies to remain competitive.
Overcoming Common Challenges in Data Enrichment Initiatives
In the realm of data-driven decision-making, data enrichment has emerged as a pivotal process that enhances the value of raw data by supplementing it with additional information. However, organizations often encounter several challenges when embarking on data enrichment initiatives. Overcoming these challenges requires a strategic approach that not only addresses the technical aspects but also aligns with the broader business objectives. One of the primary challenges in data enrichment is ensuring data quality. Poor data quality can lead to inaccurate insights, which in turn can affect decision-making processes. To tackle this, organizations must implement robust data validation and cleansing processes. By establishing stringent data quality standards and employing automated tools for data cleansing, businesses can significantly reduce errors and inconsistencies. Moreover, regular audits and updates to the data can help maintain its accuracy over time.
Another common challenge is the integration of disparate data sources. Organizations often have data scattered across various platforms and systems, making it difficult to create a unified view. To overcome this, businesses should invest in data integration solutions that facilitate seamless data flow between different systems. Utilizing APIs and data connectors can streamline the integration process, ensuring that enriched data is easily accessible and usable across the organization. Furthermore, adopting a centralized data management platform can provide a single source of truth, thereby enhancing data consistency and reliability.
Data privacy and security concerns also pose significant challenges in data enrichment initiatives. With the increasing amount of data being collected and processed, ensuring compliance with data protection regulations is crucial. Organizations must implement robust security measures to protect sensitive information and prevent unauthorized access. This includes encrypting data both in transit and at rest, as well as employing access controls and monitoring systems to detect and respond to potential threats. Additionally, fostering a culture of data privacy within the organization through regular training and awareness programs can further mitigate risks.
Scalability is another critical factor that organizations must consider when implementing data enrichment strategies. As businesses grow, the volume of data they handle increases exponentially. Therefore, it is essential to choose scalable solutions that can accommodate this growth without compromising performance. Cloud-based data enrichment platforms offer a flexible and scalable option, allowing organizations to efficiently manage large datasets and perform complex analyses without the need for significant infrastructure investments.
Moreover, aligning data enrichment initiatives with business objectives is vital for maximizing their impact. Organizations should clearly define their goals and identify the specific data attributes that will drive value. By focusing on the most relevant data points, businesses can ensure that their enrichment efforts are targeted and effective. Additionally, involving key stakeholders from different departments in the planning and implementation phases can provide valuable insights and foster collaboration, ultimately leading to more successful outcomes.
In conclusion, while data enrichment initiatives present several challenges, adopting a strategic approach can help organizations overcome these obstacles and unlock the full potential of their data. By prioritizing data quality, ensuring seamless integration, addressing privacy concerns, and focusing on scalability, businesses can enhance their data enrichment processes and drive more informed decision-making. Furthermore, aligning these initiatives with overarching business objectives ensures that the enriched data delivers tangible value, ultimately contributing to the organization’s success in an increasingly data-driven world.
Q&A
1. **What is data enrichment?**
Data enrichment is the process of enhancing existing data by adding additional information from external sources, improving its quality, accuracy, and value.
2. **Why is data enrichment important?**
Data enrichment is crucial because it helps organizations gain deeper insights, improve decision-making, enhance customer experiences, and increase the effectiveness of marketing and sales strategies.
3. **What are some common data enrichment techniques?**
Common techniques include appending missing data, correcting inaccuracies, standardizing formats, integrating third-party data, and using machine learning to derive insights.
4. **How can businesses ensure data quality during enrichment?**
Businesses can ensure data quality by implementing data validation processes, using reliable data sources, regularly updating data, and employing data governance frameworks.
5. **What role does technology play in data enrichment?**
Technology plays a significant role by providing tools and platforms for automating data collection, integration, and analysis, as well as enabling real-time data enrichment through APIs and machine learning algorithms.
6. **What are the challenges of data enrichment?**
Challenges include data privacy concerns, integration complexities, maintaining data accuracy, managing large volumes of data, and ensuring compliance with regulations like GDPR.Effective data enrichment is crucial for enhancing the value and utility of data within an organization. The top strategies for successful data enrichment include leveraging third-party data sources to fill in gaps and provide additional context, ensuring data accuracy and consistency through regular validation and cleansing processes, and utilizing advanced technologies such as machine learning and artificial intelligence to automate and optimize enrichment tasks. Additionally, integrating data enrichment processes seamlessly into existing data workflows and maintaining a focus on data privacy and compliance are essential for maximizing the benefits of enriched data. By implementing these strategies, organizations can achieve more comprehensive insights, improve decision-making, and drive better business outcomes.
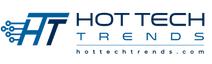