Deprecated: Implicit conversion from float 19.6 to int loses precision in /home/hottech/public_html/wp-content/plugins/internal-links/core/links/text-to-link-converter-factory.php on line 35
Deprecated: Implicit conversion from float 19.6 to int loses precision in /home/hottech/public_html/wp-content/plugins/internal-links/core/links/text-to-link-converter-factory.php on line 35
Massachusetts Institute of Technology (MIT) researchers have made significant strides in enhancing the transparency of artificial intelligence models by developing an innovative approach to automated interpretability. This advancement addresses one of the most pressing challenges in AI: understanding and explaining the decision-making processes of complex models. By automating the interpretability of AI systems, the MIT team aims to bridge the gap between model performance and human comprehension, ensuring that AI technologies can be more reliably and ethically integrated into critical applications. This breakthrough not only promises to improve trust and accountability in AI systems but also paves the way for more informed and responsible deployment of AI across various sectors.
Understanding Automated Interpretability in AI Models
In recent years, the rapid advancement of artificial intelligence (AI) has led to its integration into various sectors, ranging from healthcare to finance. However, as AI models become increasingly complex, understanding their decision-making processes has become a significant challenge. This opacity, often referred to as the “black box” problem, raises concerns about trust, accountability, and ethical implications. To address these issues, researchers at the Massachusetts Institute of Technology (MIT) have been pioneering efforts to enhance AI model transparency through automated interpretability.
Automated interpretability refers to the use of algorithms and computational techniques to elucidate the inner workings of AI models. By providing insights into how these models arrive at specific decisions, automated interpretability aims to bridge the gap between complex AI systems and human understanding. This is particularly crucial in high-stakes applications, such as medical diagnostics or autonomous driving, where the consequences of AI decisions can be profound.
The MIT researchers have developed innovative methods to automate the interpretability of AI models, focusing on creating tools that can dissect and explain the decision-making processes of these systems. One of the key approaches involves the use of interpretable machine learning models that are inherently more transparent. These models are designed to be more understandable by humans, often by simplifying the decision-making process or by using visualizations that make the model’s logic more accessible.
Moreover, the researchers have been exploring the integration of explainable AI (XAI) techniques, which aim to make AI systems more transparent by providing explanations for their outputs. These techniques include feature attribution methods, which identify the most influential factors in a model’s decision, and surrogate models, which approximate the behavior of complex models with simpler, more interpretable ones. By employing these techniques, the MIT team seeks to demystify AI models, making them more comprehensible to users and stakeholders.
In addition to enhancing transparency, automated interpretability also plays a crucial role in improving the robustness and reliability of AI models. By understanding how models make decisions, researchers can identify potential biases or errors in the data or algorithms, leading to more accurate and fair outcomes. This is particularly important in applications where biased decisions can perpetuate existing inequalities or result in unfair treatment of certain groups.
Furthermore, the work of MIT researchers in automated interpretability has significant implications for regulatory compliance and ethical AI development. As governments and organizations worldwide grapple with the ethical and legal challenges posed by AI, transparent models are becoming increasingly important. By providing clear explanations for AI decisions, automated interpretability can help ensure that AI systems adhere to ethical guidelines and legal standards, fostering greater trust and acceptance among users.
In conclusion, the efforts of MIT researchers to enhance AI model transparency through automated interpretability represent a significant step forward in addressing the challenges posed by complex AI systems. By making AI models more understandable and accountable, these advancements not only improve the reliability and fairness of AI applications but also contribute to the broader goal of developing ethical and trustworthy AI. As AI continues to evolve and permeate various aspects of society, the importance of transparency and interpretability will only grow, underscoring the need for continued research and innovation in this critical area.
The Role of MIT Researchers in Advancing AI Transparency
In recent years, the rapid advancement of artificial intelligence has brought about significant transformations across various sectors, from healthcare to finance. However, as AI systems become increasingly complex, the opacity of their decision-making processes has raised concerns among experts and the public alike. Addressing this challenge, researchers at the Massachusetts Institute of Technology (MIT) have made significant strides in enhancing AI model transparency through the development of automated interpretability techniques. This breakthrough not only promises to demystify AI systems but also aims to foster trust and accountability in their deployment.
The core of the issue lies in the “black box” nature of many AI models, particularly deep learning systems, which are often criticized for their lack of transparency. These models, while highly effective, operate in ways that are not easily understood by humans, making it difficult to ascertain how specific decisions are made. This opacity can lead to skepticism and resistance, especially in critical applications where understanding the rationale behind AI decisions is paramount. Recognizing this, MIT researchers have focused on creating methods that can automatically interpret and explain the workings of these complex models.
One of the key innovations introduced by the MIT team is the development of algorithms that can provide insights into the decision-making processes of AI systems. By employing techniques such as feature attribution and visualization, these algorithms can highlight which inputs are most influential in a model’s predictions. This approach not only aids in understanding how models arrive at specific outcomes but also helps in identifying potential biases or errors in the data or model itself. Consequently, stakeholders can make more informed decisions about the deployment and refinement of AI systems.
Moreover, the MIT researchers have emphasized the importance of user-friendly tools that facilitate interaction with AI models. By designing interfaces that allow users to query and explore model behavior, they aim to bridge the gap between complex AI systems and end-users. This democratization of AI interpretability ensures that a broader audience, including those without technical expertise, can engage with and understand AI-driven insights. As a result, organizations can harness the full potential of AI while maintaining transparency and accountability.
In addition to technical advancements, the MIT team has also been proactive in fostering interdisciplinary collaboration to address the multifaceted challenges of AI transparency. By engaging with ethicists, legal experts, and industry leaders, they are working to establish guidelines and best practices that ensure AI systems are not only effective but also ethical and fair. This holistic approach underscores the importance of considering societal implications alongside technical innovations.
Furthermore, the efforts of MIT researchers in enhancing AI transparency align with broader initiatives aimed at promoting responsible AI development. As governments and regulatory bodies worldwide grapple with the implications of AI, the work being done at MIT provides valuable insights and tools that can inform policy-making and regulatory frameworks. By setting a precedent for transparency and interpretability, MIT’s contributions are paving the way for more accountable and trustworthy AI systems.
In conclusion, the advancements made by MIT researchers in automated interpretability represent a significant step forward in addressing the transparency challenges posed by complex AI models. Through innovative algorithms, user-friendly tools, and interdisciplinary collaboration, they are not only enhancing our understanding of AI systems but also fostering a culture of trust and accountability. As AI continues to permeate various aspects of society, these efforts will be crucial in ensuring that its benefits are realized in a transparent and ethical manner.
Benefits of Enhanced AI Model Transparency for Developers
In recent years, the rapid advancement of artificial intelligence (AI) has brought about significant transformations across various industries. However, as AI models become increasingly complex, understanding their decision-making processes has become a formidable challenge. This opacity often leads to a lack of trust among developers and end-users, who are left questioning the reliability and fairness of AI-driven outcomes. In response to this growing concern, researchers at the Massachusetts Institute of Technology (MIT) have made significant strides in enhancing AI model transparency through the development of automated interpretability tools. These innovations hold the potential to offer substantial benefits for developers, fostering a more trustworthy and efficient AI ecosystem.
To begin with, enhanced AI model transparency allows developers to gain deeper insights into the inner workings of AI systems. By employing automated interpretability tools, developers can dissect complex models and understand the rationale behind specific predictions or decisions. This newfound clarity enables developers to identify potential biases or errors within the model, facilitating the refinement and improvement of AI systems. Consequently, developers can create more robust and reliable models that are better aligned with ethical standards and user expectations.
Moreover, the ability to interpret AI models effectively empowers developers to communicate their findings to non-technical stakeholders. In many cases, AI systems are deployed in environments where decision-makers may not possess a deep understanding of the underlying technology. By providing clear and comprehensible explanations of how AI models arrive at their conclusions, developers can bridge the gap between technical and non-technical audiences. This transparency fosters greater trust and confidence in AI systems, as stakeholders can make informed decisions based on a clear understanding of the model’s behavior.
In addition to improving communication, enhanced transparency also facilitates collaboration among developers. As AI models become more interpretable, developers can more easily share insights and methodologies with their peers. This collaborative environment encourages the exchange of ideas and best practices, accelerating the pace of innovation in AI development. Furthermore, by making AI models more accessible and understandable, developers can contribute to a more inclusive AI community, where diverse perspectives and expertise are valued and integrated into the development process.
Another significant benefit of enhanced AI model transparency is the potential for improved regulatory compliance. As governments and regulatory bodies worldwide grapple with the ethical implications of AI, there is an increasing demand for transparency and accountability in AI systems. By leveraging automated interpretability tools, developers can demonstrate compliance with regulatory requirements, ensuring that their models adhere to established guidelines and standards. This proactive approach not only mitigates the risk of legal repercussions but also positions developers as responsible and ethical contributors to the AI landscape.
Finally, enhanced transparency in AI models can lead to more effective debugging and troubleshooting processes. When developers can clearly understand the decision-making pathways of AI systems, they can more efficiently identify and rectify issues that may arise during deployment. This capability reduces downtime and enhances the overall performance and reliability of AI applications, ultimately benefiting both developers and end-users.
In conclusion, the advancements made by MIT researchers in enhancing AI model transparency through automated interpretability tools offer a multitude of benefits for developers. By providing deeper insights into AI systems, facilitating communication and collaboration, ensuring regulatory compliance, and improving debugging processes, these innovations pave the way for a more trustworthy and efficient AI ecosystem. As AI continues to evolve, the importance of transparency cannot be overstated, and these developments represent a significant step forward in achieving that goal.
Challenges in Implementing Automated Interpretability in AI
The rapid advancement of artificial intelligence (AI) technologies has brought about significant transformations across various sectors, from healthcare to finance. However, as AI systems become increasingly complex, understanding their decision-making processes has become a formidable challenge. This opacity, often referred to as the “black box” problem, raises concerns about accountability, fairness, and trust. In response to these challenges, researchers at the Massachusetts Institute of Technology (MIT) have been pioneering efforts to enhance AI model transparency through automated interpretability. While this development holds promise, it also presents several challenges that need to be addressed to ensure effective implementation.
One of the primary challenges in implementing automated interpretability in AI is the inherent complexity of the models themselves. Modern AI systems, particularly those based on deep learning, consist of numerous layers and parameters that interact in intricate ways. This complexity makes it difficult to pinpoint which aspects of the model are responsible for specific decisions. Consequently, developing automated tools that can accurately interpret these models without oversimplifying their functionality is a significant hurdle. Researchers must strike a delicate balance between providing meaningful insights and maintaining the integrity of the model’s decision-making process.
Moreover, the diversity of AI applications further complicates the implementation of automated interpretability. Different domains have varying requirements for interpretability, depending on the potential impact of AI decisions. For instance, in healthcare, understanding the rationale behind a diagnosis is crucial for patient safety and treatment efficacy. In contrast, in consumer applications, such as recommendation systems, the need for interpretability may be less stringent. Therefore, creating a one-size-fits-all solution for automated interpretability is impractical. Researchers must tailor interpretability methods to suit the specific needs and constraints of each application domain, which requires a deep understanding of both the technical and contextual aspects of the problem.
Another challenge lies in the evaluation of interpretability methods. Unlike traditional performance metrics, such as accuracy or precision, interpretability lacks a standardized measure. This absence of a universal benchmark makes it difficult to assess the effectiveness of different interpretability techniques objectively. Researchers must develop new evaluation frameworks that consider various dimensions of interpretability, such as comprehensibility, fidelity, and usability. These frameworks should also account for the perspectives of different stakeholders, including developers, end-users, and regulatory bodies, to ensure that the interpretability methods meet diverse expectations and requirements.
Furthermore, the integration of automated interpretability into existing AI systems poses technical and organizational challenges. From a technical standpoint, incorporating interpretability tools into AI pipelines requires seamless integration with existing infrastructure and workflows. This integration must be efficient and scalable to handle the large volumes of data and complex models typical of modern AI applications. Organizationally, fostering a culture that values transparency and interpretability is essential. This cultural shift involves educating stakeholders about the importance of interpretability and encouraging collaboration between AI researchers, domain experts, and policymakers.
In conclusion, while the efforts by MIT researchers to enhance AI model transparency through automated interpretability are commendable, several challenges must be addressed to ensure successful implementation. These challenges include managing the complexity of AI models, tailoring interpretability methods to specific domains, developing robust evaluation frameworks, and integrating interpretability into existing systems. By addressing these challenges, the AI community can move closer to creating transparent and trustworthy AI systems that align with societal values and expectations.
Case Studies: Successful Applications of Transparent AI Models
In recent years, the rapid advancement of artificial intelligence has brought about significant transformations across various sectors. However, as AI systems become increasingly complex, the need for transparency and interpretability has become more pressing. In response to this challenge, researchers at the Massachusetts Institute of Technology (MIT) have made significant strides in enhancing AI model transparency through the development of automated interpretability techniques. This case study explores the successful application of these techniques, highlighting their potential to revolutionize the way AI models are understood and trusted.
The primary concern with many AI models, particularly deep learning systems, is their “black box” nature, which makes it difficult for users to understand how decisions are made. This opacity can lead to a lack of trust, especially in critical applications such as healthcare, finance, and autonomous vehicles. Recognizing this issue, MIT researchers have focused on creating methods that automatically interpret AI models, thereby providing insights into their decision-making processes. By doing so, they aim to bridge the gap between model complexity and user comprehension.
One of the key innovations developed by the MIT team is an automated system that generates human-readable explanations for AI model predictions. This system leverages advanced algorithms to analyze the internal workings of a model and produce explanations that are both accurate and understandable. For instance, in the context of medical diagnostics, the system can elucidate why a particular diagnosis was made by highlighting relevant features in the data, such as specific patterns in medical images or key variables in patient records. This level of transparency not only enhances trust but also enables healthcare professionals to make more informed decisions.
Moreover, the MIT researchers have demonstrated the effectiveness of their approach through a series of real-world applications. In the financial sector, for example, their automated interpretability techniques have been applied to credit scoring models. By providing clear explanations for credit decisions, these techniques help financial institutions ensure fairness and compliance with regulatory standards. This is particularly important in an era where algorithmic bias is a growing concern. By making AI models more transparent, the MIT team is contributing to the development of fairer and more accountable financial systems.
In addition to healthcare and finance, the MIT researchers have also explored the application of their techniques in the realm of autonomous vehicles. Here, the ability to interpret AI models is crucial for ensuring safety and reliability. By offering insights into how autonomous systems perceive and react to their environment, the automated interpretability methods developed by MIT can help engineers identify potential weaknesses and improve system performance. This not only enhances the safety of autonomous vehicles but also fosters public confidence in their deployment.
In conclusion, the work of MIT researchers in enhancing AI model transparency through automated interpretability represents a significant advancement in the field of artificial intelligence. By providing clear and understandable explanations for complex model decisions, these techniques address the critical issue of trust in AI systems. As demonstrated through successful applications in healthcare, finance, and autonomous vehicles, the potential impact of this work is vast. As AI continues to permeate various aspects of society, the importance of transparency and interpretability cannot be overstated. The efforts of the MIT team serve as a promising step towards a future where AI systems are not only powerful but also transparent and trustworthy.
Future Prospects of AI Model Transparency in Technology
In recent years, the rapid advancement of artificial intelligence has brought about significant transformations across various sectors, from healthcare to finance. However, as AI models become increasingly complex, the need for transparency and interpretability has become more pressing. Recognizing this challenge, researchers at the Massachusetts Institute of Technology (MIT) have made significant strides in enhancing AI model transparency through the development of automated interpretability techniques. This innovation holds the potential to reshape the future of AI technology by making models more understandable and trustworthy.
The complexity of modern AI models, particularly deep learning systems, often renders them as “black boxes,” where the decision-making process is opaque to users and developers alike. This lack of transparency can lead to mistrust, especially in critical applications such as medical diagnostics or autonomous driving, where understanding the rationale behind a model’s decision is crucial. To address this issue, MIT researchers have focused on creating methods that automatically interpret the inner workings of AI models, thereby demystifying their decision-making processes.
One of the key approaches developed by the MIT team involves the use of visualization techniques that highlight which parts of the input data are most influential in the model’s predictions. By employing these techniques, users can gain insights into how specific features of the data contribute to the final output. This not only aids in understanding the model’s behavior but also helps in identifying potential biases or errors in the data that could affect the model’s performance. Consequently, this level of interpretability fosters greater confidence in AI systems, as stakeholders can verify and validate the model’s decisions.
Moreover, the MIT researchers have integrated these interpretability techniques into automated tools that can be easily applied to various AI models. This automation is crucial, as it allows for scalability and consistency in interpreting models across different applications and industries. By streamlining the interpretability process, these tools enable developers and users to focus on refining and improving AI models rather than spending excessive time deciphering their outputs. This efficiency is particularly beneficial in fast-paced environments where timely decision-making is essential.
Furthermore, the implications of enhanced AI model transparency extend beyond individual applications. As AI systems become more interpretable, they pave the way for more robust regulatory frameworks and ethical guidelines. Policymakers and regulatory bodies can leverage these interpretability tools to ensure that AI models adhere to ethical standards and do not perpetuate harmful biases. This alignment with ethical considerations is vital for fostering public trust and acceptance of AI technologies.
In addition to regulatory benefits, the advancements in AI model transparency also hold promise for collaborative innovation. By making AI models more understandable, researchers and developers from diverse fields can collaborate more effectively, sharing insights and expertise to drive further advancements in AI technology. This interdisciplinary approach can lead to the development of more sophisticated and reliable AI systems that address complex real-world challenges.
In conclusion, the work of MIT researchers in enhancing AI model transparency through automated interpretability represents a significant leap forward in the field of artificial intelligence. By making AI models more understandable and trustworthy, these innovations have the potential to transform the future of AI technology. As transparency becomes an integral part of AI development, it will not only improve the reliability and ethical standards of AI systems but also foster greater collaboration and innovation across various sectors.
Q&A
1. **What is the main focus of the MIT researchers’ work?**
– The main focus is on enhancing AI model transparency through automated interpretability techniques.
2. **What is automated interpretability?**
– Automated interpretability refers to methods and tools that automatically explain how AI models make decisions, making them more understandable to humans.
3. **Why is transparency important in AI models?**
– Transparency is crucial for building trust, ensuring ethical use, and enabling users to understand and verify AI decision-making processes.
4. **What techniques are used by MIT researchers to enhance interpretability?**
– The researchers use techniques such as feature attribution, visualization tools, and model simplification to make AI models more interpretable.
5. **How does this research benefit AI users?**
– It benefits AI users by providing clearer insights into model behavior, improving trust, and facilitating better decision-making based on AI outputs.
6. **What potential impact does this research have on the future of AI?**
– This research could lead to more widespread adoption of AI technologies by making them more transparent and accountable, thus addressing ethical and regulatory concerns.MIT researchers have made significant strides in enhancing the transparency of AI models by developing automated interpretability techniques. These advancements aim to demystify the decision-making processes of complex AI systems, making them more understandable and trustworthy for users. By automating the interpretability of AI models, the researchers have provided tools that can elucidate how these models arrive at specific conclusions, thereby fostering greater confidence and facilitating more informed deployment of AI technologies across various sectors. This progress not only addresses critical concerns about AI’s “black box” nature but also paves the way for more ethical and responsible AI applications.
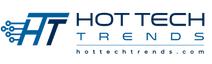