The Positive Feedback Loop of AI Research refers to the self-reinforcing cycle that accelerates advancements in artificial intelligence. As AI technologies improve, they enhance research capabilities, leading to more efficient data processing, better algorithms, and innovative applications. This progress attracts increased investment and talent, further fueling research and development. Consequently, breakthroughs in AI lead to more sophisticated tools and methodologies, perpetuating the cycle. This loop not only accelerates technological growth but also expands AI’s impact across various sectors, driving societal and economic transformations.
Accelerating Innovation: How AI Research Fuels Its Own Growth
The field of artificial intelligence (AI) has witnessed unprecedented growth over the past few decades, driven by a positive feedback loop that continuously accelerates innovation. This self-reinforcing cycle is characterized by the interplay between technological advancements, increased research capabilities, and the expanding application of AI across various domains. As AI research progresses, it not only enhances existing technologies but also opens new avenues for exploration, thereby fueling its own growth.
To begin with, the rapid advancements in computational power and data availability have significantly contributed to the acceleration of AI research. The development of more powerful processors and the proliferation of big data have enabled researchers to train complex models with greater efficiency and accuracy. This, in turn, has led to breakthroughs in machine learning algorithms, which are the backbone of AI systems. As these algorithms become more sophisticated, they enhance the ability of AI to process and analyze vast amounts of information, leading to more accurate predictions and better decision-making capabilities.
Moreover, the iterative nature of AI research plays a crucial role in its self-sustaining growth. As researchers develop new models and techniques, they often build upon previous work, refining and improving existing methodologies. This iterative process not only leads to incremental improvements but also occasionally results in paradigm shifts that redefine the field. For instance, the transition from rule-based systems to neural networks marked a significant leap in AI capabilities, enabling machines to learn from data rather than relying solely on pre-programmed instructions.
In addition to technological advancements, the collaborative nature of AI research has been instrumental in accelerating innovation. The open-source movement, which encourages the sharing of code and data, has fostered a global community of researchers who contribute to and benefit from collective knowledge. This collaborative environment facilitates the rapid dissemination of ideas and findings, allowing researchers to build on each other’s work and avoid duplicating efforts. Consequently, the pace of innovation is accelerated as researchers can focus on pushing the boundaries of what is possible rather than reinventing the wheel.
Furthermore, the expanding application of AI across various industries has created a demand for continued research and development. As AI systems are integrated into sectors such as healthcare, finance, and transportation, they generate valuable insights and data that can be used to further refine and improve AI technologies. This real-world feedback loop not only drives the development of more robust and reliable AI systems but also highlights new challenges and opportunities for research. As a result, the application of AI in diverse fields serves as both a catalyst for innovation and a source of inspiration for future research endeavors.
Finally, the positive feedback loop of AI research is reinforced by the growing investment in the field. As AI technologies demonstrate their potential to transform industries and improve quality of life, both public and private sectors are increasingly investing in AI research and development. This influx of resources enables researchers to explore new ideas, experiment with cutting-edge technologies, and attract top talent to the field. In turn, these investments lead to further advancements in AI, perpetuating the cycle of growth and innovation.
In conclusion, the positive feedback loop of AI research is a dynamic and self-sustaining process that accelerates innovation through the interplay of technological advancements, collaborative efforts, expanding applications, and increased investment. As AI continues to evolve, this cycle will likely persist, driving the field toward new horizons and unlocking unprecedented possibilities for the future.
Collaborative Synergy: The Role of Open Source in AI Advancements
The rapid advancements in artificial intelligence (AI) over the past decade can be attributed to a multitude of factors, among which the collaborative synergy fostered by open-source platforms stands out as a pivotal element. Open-source initiatives have created a positive feedback loop in AI research, where shared knowledge and resources accelerate innovation, which in turn fuels further collaboration and development. This dynamic interplay has not only democratized access to cutting-edge technologies but also spurred a collective effort to tackle complex challenges that no single entity could address alone.
To begin with, open-source platforms have dismantled traditional barriers to entry in AI research. By providing free access to powerful tools, libraries, and datasets, these platforms have empowered researchers, developers, and enthusiasts from diverse backgrounds to contribute to the field. This democratization of resources has led to a more inclusive and diverse research community, which is crucial for fostering creativity and innovation. As a result, novel ideas and approaches emerge from unexpected quarters, enriching the collective knowledge pool and driving the field forward.
Moreover, the collaborative nature of open-source projects encourages transparency and reproducibility in AI research. When researchers share their code and methodologies openly, it allows others to verify results, identify potential flaws, and build upon existing work. This transparency not only enhances the credibility of research findings but also accelerates the pace of discovery, as researchers can avoid duplicating efforts and instead focus on refining and expanding upon proven concepts. Consequently, the iterative process of improvement becomes more efficient, leading to rapid advancements in AI technologies.
In addition to fostering transparency, open-source collaboration facilitates the cross-pollination of ideas across different domains. AI research is inherently interdisciplinary, drawing insights from fields such as computer science, mathematics, neuroscience, and linguistics. Open-source platforms serve as a melting pot where experts from these diverse disciplines can converge, exchange ideas, and collaborate on projects. This cross-disciplinary synergy often leads to breakthroughs that might not have been possible within the confines of a single field. For instance, techniques developed in natural language processing can inform advancements in computer vision, and vice versa, creating a virtuous cycle of innovation.
Furthermore, the open-source model encourages a culture of continuous learning and skill development. As researchers and developers engage with open-source projects, they gain exposure to a wide array of tools and techniques, enhancing their expertise and adaptability. This culture of learning is particularly beneficial in the fast-evolving field of AI, where staying abreast of the latest developments is crucial for maintaining a competitive edge. By fostering a community of lifelong learners, open-source platforms ensure that the AI research ecosystem remains vibrant and resilient in the face of emerging challenges.
In conclusion, the role of open-source collaboration in AI advancements cannot be overstated. By breaking down barriers, promoting transparency, facilitating interdisciplinary collaboration, and nurturing a culture of continuous learning, open-source platforms have created a positive feedback loop that propels the field of AI forward. As AI continues to evolve and permeate various aspects of society, the collaborative synergy enabled by open-source initiatives will remain a cornerstone of innovation, driving progress and ensuring that the benefits of AI are accessible to all.
Data Abundance: Leveraging Big Data for AI Breakthroughs
The rapid advancement of artificial intelligence (AI) in recent years can be largely attributed to the abundance of data available for research and development. This data abundance has created a positive feedback loop, where the availability of vast datasets fuels AI breakthroughs, which in turn generate more data, further propelling the cycle of innovation. As we delve into this phenomenon, it becomes evident that the interplay between data and AI is a critical driver of technological progress.
To begin with, the proliferation of digital data has provided AI researchers with unprecedented opportunities to train and refine machine learning models. The sheer volume of data generated daily—from social media interactions to sensor readings in smart devices—serves as a rich resource for developing algorithms that can learn from diverse and complex inputs. This wealth of information allows AI systems to improve their accuracy and efficiency, as they can be trained on a wide array of scenarios and conditions. Consequently, the more data these systems are exposed to, the better they become at recognizing patterns and making predictions.
Moreover, the availability of big data has facilitated the development of more sophisticated AI models, such as deep learning networks. These models require vast amounts of data to function effectively, as they rely on multiple layers of processing to extract intricate features from raw inputs. The abundance of data ensures that these models can be trained on comprehensive datasets, enabling them to achieve remarkable levels of performance in tasks such as image recognition, natural language processing, and autonomous driving. As these models become more capable, they generate even more data through their interactions with the world, thus feeding back into the cycle of data-driven innovation.
In addition to enhancing the capabilities of AI systems, data abundance also plays a crucial role in democratizing AI research. With access to large datasets, researchers from diverse backgrounds and institutions can contribute to the field, fostering a collaborative environment that accelerates progress. Open data initiatives and shared repositories have made it easier for researchers to access the information they need, breaking down barriers to entry and encouraging a wider range of perspectives in AI development. This inclusivity not only enriches the research landscape but also leads to more robust and versatile AI solutions.
Furthermore, the positive feedback loop of data and AI is not limited to technological advancements; it also has significant implications for various industries. In healthcare, for instance, the integration of AI with big data has led to breakthroughs in personalized medicine, where algorithms analyze patient data to tailor treatments to individual needs. Similarly, in finance, AI-driven data analysis has improved risk assessment and fraud detection, enhancing the security and efficiency of financial transactions. These applications demonstrate how the synergy between data and AI can drive innovation across sectors, ultimately benefiting society as a whole.
In conclusion, the abundance of data has created a fertile ground for AI research, establishing a positive feedback loop that continues to drive technological progress. As AI systems become more advanced, they generate additional data, which in turn fuels further breakthroughs. This cycle not only enhances the capabilities of AI but also democratizes research and fosters innovation across various industries. As we move forward, it is essential to recognize the pivotal role of data in shaping the future of AI and to continue leveraging this resource to unlock new possibilities.
Educational Impact: Training the Next Generation of AI Researchers
The field of artificial intelligence (AI) has experienced unprecedented growth over the past few decades, driven by rapid technological advancements and an increasing demand for intelligent systems. This growth has created a positive feedback loop in AI research, particularly in the realm of education, where the focus is on training the next generation of AI researchers. As AI technologies continue to evolve, educational institutions are adapting their curricula to equip students with the necessary skills and knowledge to thrive in this dynamic field. This adaptation, in turn, fuels further advancements in AI, creating a cycle of continuous improvement and innovation.
To begin with, the integration of AI into educational programs has become a priority for many universities and research institutions worldwide. By offering specialized courses and degree programs in AI and machine learning, these institutions are preparing students to tackle complex problems and contribute to cutting-edge research. This educational emphasis not only enhances students’ technical skills but also fosters critical thinking and problem-solving abilities, which are essential for innovation in AI. Moreover, the inclusion of interdisciplinary approaches in AI education, such as combining computer science with fields like neuroscience, linguistics, and ethics, provides students with a holistic understanding of AI’s potential and challenges.
Furthermore, the availability of online resources and platforms has democratized access to AI education, allowing a broader audience to engage with AI research. Massive open online courses (MOOCs), webinars, and virtual workshops have made it possible for individuals from diverse backgrounds to learn about AI, regardless of their geographical location. This widespread access to AI education not only increases the pool of potential researchers but also brings diverse perspectives to the field, which is crucial for addressing the multifaceted challenges posed by AI technologies.
In addition to formal education, hands-on experience plays a vital role in training future AI researchers. Internships, research assistantships, and collaborative projects with industry partners provide students with practical experience and exposure to real-world applications of AI. These opportunities enable students to apply theoretical knowledge to practical problems, thereby deepening their understanding and sparking innovative ideas. Moreover, collaboration between academia and industry fosters a symbiotic relationship where academic research informs industry practices, and industry challenges inspire academic inquiry.
As the next generation of AI researchers emerges, they are not only contributing to technological advancements but also shaping the ethical and societal implications of AI. Educational programs are increasingly incorporating discussions on AI ethics, bias, and fairness, ensuring that future researchers are equipped to develop responsible and equitable AI systems. This focus on ethical considerations is essential for building public trust in AI technologies and ensuring that their benefits are distributed fairly across society.
In conclusion, the positive feedback loop of AI research is significantly influenced by the educational impact of training the next generation of AI researchers. By adapting curricula, providing access to diverse learning resources, and emphasizing hands-on experience, educational institutions are playing a crucial role in advancing AI research. This, in turn, leads to further technological innovations and a deeper understanding of AI’s potential and challenges. As this cycle continues, it promises to drive the field of AI forward, ensuring that future researchers are well-equipped to navigate the complexities of this rapidly evolving domain.
Industry Partnerships: Driving AI Progress Through Collaboration
The rapid advancement of artificial intelligence (AI) technology has been significantly propelled by strategic industry partnerships, which have become a cornerstone in driving AI progress through collaboration. These partnerships, often formed between academic institutions, technology companies, and industry leaders, create a positive feedback loop that accelerates innovation and application in the AI field. By pooling resources, expertise, and data, these collaborations enable the development of more sophisticated AI models and solutions, which in turn attract further investment and interest, perpetuating a cycle of continuous improvement and discovery.
One of the primary benefits of industry partnerships in AI research is the ability to leverage diverse expertise and perspectives. Academic institutions bring a wealth of theoretical knowledge and cutting-edge research capabilities, while industry partners contribute practical insights and real-world applications. This synergy allows for the translation of theoretical models into practical solutions that can be deployed across various sectors, from healthcare to finance. For instance, collaborations between universities and healthcare companies have led to the development of AI systems capable of early disease detection, significantly improving patient outcomes and reducing healthcare costs.
Moreover, these partnerships facilitate access to vast amounts of data, which is crucial for training and refining AI algorithms. Industry partners often possess extensive datasets that are not readily available to academic researchers. By sharing this data, companies enable researchers to develop more accurate and robust AI models. In return, these companies benefit from the cutting-edge research and innovations that emerge from these collaborations, which can be integrated into their products and services, enhancing their competitive edge in the market.
In addition to data sharing, industry partnerships also provide financial support that is essential for sustaining long-term AI research projects. Developing advanced AI technologies requires significant investment in infrastructure, talent, and resources. Industry partners, with their substantial financial capabilities, can fund research initiatives that might otherwise be unattainable for academic institutions alone. This financial backing not only supports the immediate research goals but also helps in building a sustainable ecosystem for future AI advancements.
Furthermore, these collaborations often lead to the establishment of joint research labs and innovation hubs, where interdisciplinary teams work together to tackle complex AI challenges. These environments foster creativity and innovation, as researchers from different backgrounds and areas of expertise collaborate to push the boundaries of what AI can achieve. The cross-pollination of ideas in such settings often results in breakthroughs that might not have been possible in isolated research environments.
The positive feedback loop created by industry partnerships in AI research is also evident in the way these collaborations attract top talent. As AI becomes an increasingly attractive field, the opportunity to work on cutting-edge projects with leading industry players draws skilled researchers and engineers. This influx of talent further accelerates the pace of innovation, as fresh ideas and perspectives are continuously introduced into the research process.
In conclusion, industry partnerships play a pivotal role in driving AI progress through collaboration. By combining the strengths of academic research and industry expertise, these partnerships create a dynamic environment that fosters innovation and accelerates the development of AI technologies. The positive feedback loop generated by these collaborations not only advances the field of AI but also ensures that its benefits are realized across various sectors, ultimately contributing to societal progress and economic growth. As AI continues to evolve, the importance of these partnerships will only grow, underscoring the need for continued collaboration and investment in this transformative field.
Technological Convergence: How AI Research Benefits from Interdisciplinary Approaches
The rapid advancement of artificial intelligence (AI) research is not occurring in isolation; rather, it is deeply intertwined with developments in various other scientific and technological fields. This convergence of disciplines creates a positive feedback loop, where progress in one area fuels advancements in another, ultimately accelerating the overall pace of innovation. By examining the interdisciplinary nature of AI research, we can better understand how this synergy is driving transformative changes across multiple domains.
To begin with, the integration of AI with fields such as neuroscience and cognitive science has been particularly fruitful. Insights into the human brain’s functioning have inspired the development of neural networks, which are foundational to many AI systems. Conversely, AI models have provided neuroscientists with new tools to simulate and understand complex brain processes. This bidirectional exchange of knowledge not only enhances our understanding of human cognition but also leads to the creation of more sophisticated AI systems that can mimic human-like learning and decision-making processes.
Moreover, the intersection of AI with data science and big data analytics has been instrumental in the evolution of machine learning algorithms. The vast amounts of data generated in today’s digital world serve as the raw material for training AI models. As data science techniques become more refined, they enable the extraction of meaningful patterns and insights from these large datasets, which in turn improves the accuracy and efficiency of AI systems. This symbiotic relationship ensures that as data science advances, AI systems become more capable, and as AI systems improve, they can handle even larger and more complex datasets.
In addition to these fields, the collaboration between AI research and robotics has led to significant breakthroughs in automation and intelligent systems. Robotics provides a tangible platform for implementing AI algorithms, allowing researchers to test and refine their models in real-world environments. This practical application not only validates theoretical research but also drives further innovation in both AI and robotics. As robots become more autonomous and capable, they open up new possibilities for AI applications in industries ranging from manufacturing to healthcare.
Furthermore, the convergence of AI with fields like ethics and philosophy is shaping the development of responsible AI systems. As AI technologies become more pervasive, ethical considerations are increasingly important to ensure that these systems are used for the benefit of society. Interdisciplinary research in this area is crucial for developing frameworks and guidelines that address issues such as bias, privacy, and accountability. By incorporating diverse perspectives from the humanities and social sciences, AI researchers can create systems that are not only technically advanced but also socially responsible.
Finally, the collaboration between AI and other technological domains, such as quantum computing, holds the potential to revolutionize the field. Quantum computing promises to exponentially increase computational power, which could enable the development of AI models that are currently beyond our reach. As researchers explore the intersection of these two cutting-edge fields, they are likely to uncover new algorithms and techniques that will redefine the capabilities of AI.
In conclusion, the positive feedback loop of AI research is driven by its interdisciplinary nature, where advancements in one field catalyze progress in others. This convergence not only accelerates technological innovation but also ensures that AI systems are developed in a holistic and responsible manner. As researchers continue to collaborate across disciplines, the potential for AI to transform society in profound ways becomes increasingly attainable.
Q&A
1. **What is the Positive Feedback Loop in AI Research?**
The Positive Feedback Loop in AI Research refers to the cycle where advancements in AI technology lead to improved tools and methods, which in turn accelerate further AI research and development.
2. **How does increased computational power contribute to the loop?**
Increased computational power allows researchers to train more complex models faster, leading to breakthroughs that further enhance computational techniques and hardware, perpetuating the cycle.
3. **What role does data play in the Positive Feedback Loop?**
As AI systems improve, they can process and analyze larger datasets more effectively, leading to better insights and models, which then attract more data collection and usage.
4. **How do improved AI models impact research productivity?**
Improved AI models can automate and optimize various research tasks, increasing productivity and allowing researchers to focus on more complex problems, thus accelerating the pace of innovation.
5. **What is the impact of AI research on industry applications?**
Breakthroughs in AI research often lead to new applications and solutions in various industries, which generate economic incentives and funding that are reinvested into further AI research.
6. **How does collaboration enhance the Positive Feedback Loop?**
Collaboration among researchers, institutions, and industries facilitates the sharing of knowledge, resources, and data, which accelerates innovation and strengthens the feedback loop.The positive feedback loop of AI research refers to the self-reinforcing cycle where advancements in artificial intelligence lead to improved tools and methodologies, which in turn accelerate further research and development. As AI systems become more sophisticated, they enhance data analysis, optimize research processes, and enable the creation of more powerful algorithms. This progress attracts increased investment and talent, fostering a thriving ecosystem that propels innovation. Consequently, the rapid evolution of AI technologies continues to drive breakthroughs across various fields, creating a dynamic and exponential growth pattern in AI research and its applications.
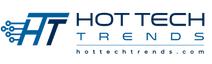