Harnessing the power of artificial intelligence (AI) for climate change solutions represents a transformative approach to one of the most pressing challenges of our time. As the impacts of climate change become increasingly severe, innovative technologies like AI offer unprecedented opportunities to mitigate its effects and adapt to new environmental realities. AI’s ability to process vast amounts of data, identify patterns, and make predictions can enhance our understanding of climate systems, optimize energy use, and improve resource management. From developing smarter grids and enhancing renewable energy efficiency to predicting extreme weather events and optimizing agricultural practices, AI is poised to play a crucial role in driving sustainable solutions. By integrating AI into climate strategies, we can accelerate the transition to a low-carbon economy, enhance resilience, and foster a more sustainable future for generations to come.
Predictive Analytics for Climate Modeling
In the quest to address the multifaceted challenges posed by climate change, predictive analytics has emerged as a pivotal tool, offering unprecedented insights into climate modeling. As the world grapples with the escalating impacts of climate change, the integration of artificial intelligence (AI) into predictive analytics is proving to be a game-changer. This innovative approach not only enhances the accuracy of climate models but also provides a deeper understanding of the complex interactions within Earth’s systems.
Predictive analytics, at its core, involves the use of data, statistical algorithms, and machine learning techniques to identify the likelihood of future outcomes based on historical data. When applied to climate modeling, it allows scientists to simulate and predict future climate conditions with greater precision. The incorporation of AI into this process has significantly improved the ability to analyze vast datasets, which are often too complex and voluminous for traditional methods. By leveraging AI, researchers can process and interpret data from various sources, such as satellite imagery, oceanic patterns, and atmospheric conditions, to create more robust climate models.
One of the primary advantages of using AI in predictive analytics for climate modeling is its ability to handle the non-linear and dynamic nature of climate systems. Traditional models often struggle with the chaotic and interconnected variables that define climate behavior. However, AI algorithms, particularly those based on deep learning, excel at recognizing patterns and making sense of intricate data relationships. This capability allows for more accurate predictions of extreme weather events, such as hurricanes, droughts, and floods, which are becoming increasingly frequent and severe due to climate change.
Moreover, AI-driven predictive analytics facilitates the identification of long-term climate trends and potential tipping points. By analyzing historical climate data alongside current observations, AI can help scientists detect subtle changes that may indicate significant shifts in climate patterns. This foresight is crucial for policymakers and stakeholders who need to develop adaptive strategies to mitigate the adverse effects of climate change. For instance, understanding the likelihood of prolonged droughts can inform water management policies, while predictions of rising sea levels can guide coastal infrastructure planning.
In addition to enhancing the accuracy of climate models, AI also contributes to the democratization of climate science. The accessibility of AI tools and platforms enables a broader range of researchers, including those from developing countries, to participate in climate modeling efforts. This inclusivity fosters a more comprehensive understanding of global climate dynamics and encourages collaborative solutions to shared challenges. Furthermore, AI-powered predictive analytics can be used to engage the public by providing clear and relatable visualizations of climate data, thereby raising awareness and promoting informed decision-making.
Despite the promising potential of AI in predictive analytics for climate modeling, challenges remain. The quality and availability of data are critical factors that influence the effectiveness of AI models. Ensuring that data is accurate, comprehensive, and up-to-date is essential for reliable predictions. Additionally, the ethical implications of AI use, such as data privacy and algorithmic bias, must be carefully considered to maintain public trust and ensure equitable outcomes.
In conclusion, the integration of AI into predictive analytics represents a significant advancement in climate modeling, offering enhanced accuracy and deeper insights into climate systems. As the world continues to confront the realities of climate change, harnessing the power of AI will be instrumental in developing effective strategies to mitigate its impacts and build a sustainable future. Through continued innovation and collaboration, AI-driven predictive analytics holds the promise of transforming our understanding of climate change and guiding us toward a more resilient world.
AI-Driven Renewable Energy Optimization
Artificial intelligence (AI) has emerged as a transformative force across various sectors, and its potential to address climate change is particularly promising. In the realm of renewable energy, AI-driven optimization is playing a pivotal role in enhancing efficiency and effectiveness. As the world grapples with the urgent need to transition to sustainable energy sources, AI offers innovative solutions that can significantly accelerate this shift.
To begin with, AI’s ability to process vast amounts of data with remarkable speed and accuracy is a key asset in optimizing renewable energy systems. For instance, in the context of solar and wind energy, AI algorithms can analyze weather patterns, historical data, and real-time information to predict energy production levels with high precision. This predictive capability allows for better integration of renewable energy into the grid, minimizing wastage and ensuring a more stable energy supply. Consequently, energy providers can make informed decisions about when to store energy, when to release it, and how to balance supply with demand.
Moreover, AI-driven optimization extends to the maintenance and operation of renewable energy infrastructure. Machine learning models can be employed to monitor the health of solar panels and wind turbines, identifying potential faults before they lead to significant downtime. By predicting maintenance needs, AI helps in reducing operational costs and prolonging the lifespan of renewable energy assets. This proactive approach not only enhances the reliability of renewable energy systems but also contributes to their economic viability, making them more attractive to investors and stakeholders.
In addition to operational efficiencies, AI is instrumental in advancing the design and development of renewable energy technologies. Through simulations and modeling, AI can explore a multitude of design configurations and material compositions, identifying the most efficient and cost-effective solutions. This capability accelerates the innovation cycle, enabling the rapid deployment of cutting-edge technologies that can harness renewable energy more effectively. As a result, the transition to a low-carbon economy is expedited, supporting global efforts to mitigate climate change.
Furthermore, AI-driven optimization is crucial in addressing the intermittency challenges associated with renewable energy sources. By integrating AI with energy storage systems, such as batteries, it is possible to optimize the charging and discharging cycles, ensuring that energy is available when needed. This integration enhances the reliability of renewable energy, making it a more dependable component of the energy mix. Additionally, AI can facilitate the development of smart grids, which are capable of dynamically adjusting to fluctuations in energy supply and demand, further stabilizing the energy system.
While the benefits of AI-driven renewable energy optimization are substantial, it is important to acknowledge the challenges that accompany its implementation. Issues such as data privacy, cybersecurity, and the need for substantial computational resources must be addressed to fully realize AI’s potential in this domain. Nevertheless, ongoing advancements in AI technology and increased collaboration between stakeholders are paving the way for overcoming these hurdles.
In conclusion, AI-driven optimization is a powerful tool in the quest for sustainable energy solutions. By enhancing the efficiency, reliability, and economic viability of renewable energy systems, AI is playing a crucial role in the global effort to combat climate change. As technology continues to evolve, the integration of AI in renewable energy will undoubtedly become more sophisticated, offering even greater opportunities for innovation and progress. Through strategic implementation and continued investment, AI has the potential to transform the renewable energy landscape, driving us closer to a sustainable future.
Machine Learning in Carbon Footprint Reduction
The integration of artificial intelligence (AI) into efforts to combat climate change has opened new avenues for reducing carbon footprints across various sectors. Machine learning, a subset of AI, plays a pivotal role in this endeavor by offering innovative solutions that enhance efficiency and sustainability. As the world grapples with the escalating impacts of climate change, the application of machine learning in carbon footprint reduction emerges as a promising strategy to mitigate environmental degradation.
To begin with, machine learning algorithms are adept at analyzing vast datasets, which is crucial for understanding and managing carbon emissions. By processing and interpreting complex data, these algorithms can identify patterns and trends that might otherwise go unnoticed. For instance, in the energy sector, machine learning models can predict energy consumption patterns, enabling more efficient energy distribution and reducing waste. This predictive capability allows for the optimization of energy grids, ensuring that energy is used more efficiently and sustainably.
Moreover, machine learning facilitates the development of smart grids, which are instrumental in integrating renewable energy sources into existing energy systems. By analyzing data from various sources, such as weather forecasts and energy consumption patterns, machine learning algorithms can optimize the use of renewable energy, thereby reducing reliance on fossil fuels. This not only decreases carbon emissions but also enhances the resilience of energy systems against climate-related disruptions.
In addition to optimizing energy use, machine learning is also transforming the transportation sector, which is a significant contributor to global carbon emissions. Through the development of intelligent transportation systems, machine learning can improve traffic management, reduce congestion, and promote the use of electric vehicles. For example, AI-powered navigation systems can provide real-time traffic updates and suggest the most efficient routes, thereby minimizing fuel consumption and emissions. Furthermore, machine learning algorithms can optimize the charging infrastructure for electric vehicles, ensuring that charging stations are strategically located and efficiently utilized.
Transitioning to the industrial sector, machine learning offers substantial opportunities for reducing carbon footprints by enhancing manufacturing processes. By analyzing production data, machine learning models can identify inefficiencies and suggest improvements that lead to reduced energy consumption and waste. Predictive maintenance, powered by machine learning, can also play a crucial role in minimizing downtime and extending the lifespan of machinery, further contributing to sustainability efforts.
Furthermore, machine learning is instrumental in advancing carbon capture and storage technologies. By modeling and simulating various scenarios, machine learning can optimize the design and operation of carbon capture systems, making them more efficient and cost-effective. This is particularly important as industries seek to reduce their carbon emissions while maintaining economic viability.
In conclusion, the application of machine learning in carbon footprint reduction is a testament to the transformative potential of AI in addressing climate change. By enhancing efficiency across various sectors, from energy and transportation to industry, machine learning not only reduces carbon emissions but also promotes sustainable practices. As technology continues to evolve, the integration of machine learning into climate change solutions will undoubtedly play a critical role in shaping a more sustainable future. The ongoing collaboration between AI experts, policymakers, and industry leaders will be essential in harnessing the full potential of machine learning to combat climate change effectively.
Smart Agriculture for Sustainable Farming
In the quest to mitigate the impacts of climate change, smart agriculture emerges as a pivotal component of sustainable farming practices. As the global population continues to rise, the demand for food production intensifies, placing unprecedented pressure on agricultural systems. Consequently, the integration of artificial intelligence (AI) into farming practices offers a promising avenue to enhance productivity while minimizing environmental impact. By leveraging AI technologies, farmers can optimize resource use, improve crop yields, and reduce greenhouse gas emissions, thereby contributing to a more sustainable agricultural future.
To begin with, AI-driven precision agriculture is revolutionizing the way farmers manage their crops. Through the use of advanced sensors, drones, and satellite imagery, AI systems can collect and analyze vast amounts of data related to soil health, weather patterns, and crop conditions. This data-driven approach enables farmers to make informed decisions about when and where to plant, irrigate, and apply fertilizers or pesticides. For instance, AI algorithms can predict the optimal time for planting based on weather forecasts, ensuring that crops receive the right amount of water and nutrients at the right time. This precision not only enhances crop yields but also reduces the overuse of water and chemicals, which are significant contributors to environmental degradation.
Moreover, AI technologies facilitate the development of climate-resilient crops. By analyzing genetic data and environmental conditions, AI can assist in breeding crops that are more resistant to extreme weather events such as droughts, floods, and heatwaves. These resilient crops are crucial in maintaining food security in the face of climate change, as they can withstand adverse conditions that would otherwise devastate traditional crop varieties. Furthermore, AI can help identify and monitor pest and disease outbreaks, allowing for timely interventions that prevent widespread crop damage. This proactive approach not only safeguards food production but also reduces the need for chemical interventions, promoting a healthier ecosystem.
In addition to crop management, AI plays a significant role in optimizing livestock farming. Through machine learning algorithms, farmers can monitor the health and well-being of their animals in real-time. Sensors and wearable devices collect data on animal behavior, feeding patterns, and environmental conditions, which AI systems analyze to detect signs of illness or stress. Early detection of health issues enables farmers to take preventive measures, reducing the need for antibiotics and improving animal welfare. Furthermore, AI can optimize feed formulations to ensure that livestock receive the necessary nutrients while minimizing waste and emissions. This not only enhances productivity but also reduces the carbon footprint of livestock farming.
Transitioning to smart agriculture requires overcoming several challenges, including the need for significant investment in technology and infrastructure. However, the long-term benefits of AI integration in agriculture are substantial. By improving resource efficiency, enhancing crop resilience, and reducing environmental impact, AI-driven smart agriculture holds the potential to transform farming into a more sustainable and climate-friendly industry. As policymakers and stakeholders recognize the importance of sustainable agriculture in addressing climate change, the adoption of AI technologies is likely to accelerate, paving the way for a more resilient and sustainable food system.
In conclusion, harnessing AI for smart agriculture presents a viable solution to the challenges posed by climate change. By optimizing resource use, improving crop and livestock management, and enhancing resilience to environmental stressors, AI technologies offer a pathway to sustainable farming practices. As the world grapples with the urgent need to address climate change, the integration of AI into agriculture stands as a beacon of hope, promising a future where food production is both efficient and environmentally responsible.
AI-Powered Climate Risk Assessment
Artificial Intelligence (AI) has emerged as a transformative force across various sectors, and its application in climate risk assessment is proving to be particularly promising. As the world grapples with the escalating impacts of climate change, the need for precise and comprehensive risk assessment tools has never been more critical. AI, with its ability to process vast amounts of data and identify patterns, offers a powerful means to enhance our understanding of climate risks and inform effective mitigation strategies.
To begin with, AI-powered climate risk assessment tools are capable of analyzing complex datasets that include historical climate data, satellite imagery, and real-time weather information. By leveraging machine learning algorithms, these tools can identify trends and anomalies that might be imperceptible to human analysts. For instance, AI can predict the likelihood of extreme weather events such as hurricanes, floods, and droughts with greater accuracy. This predictive capability is invaluable for governments and organizations seeking to implement proactive measures to protect vulnerable communities and infrastructure.
Moreover, AI facilitates the integration of diverse data sources, enabling a more holistic view of climate risks. Traditional risk assessment methods often rely on limited datasets, which can result in incomplete or skewed analyses. In contrast, AI systems can synthesize information from a wide array of sources, including social media, economic reports, and environmental studies. This comprehensive approach allows for a more nuanced understanding of how climate change impacts different regions and sectors, thereby supporting more targeted and effective interventions.
In addition to enhancing predictive accuracy, AI also plays a crucial role in optimizing resource allocation for climate adaptation and mitigation efforts. By analyzing data on resource availability, environmental conditions, and socio-economic factors, AI can help decision-makers prioritize actions that yield the greatest benefits. For example, AI can identify areas where investments in renewable energy infrastructure would be most effective, or where conservation efforts could have the highest impact on biodiversity preservation. This strategic allocation of resources is essential for maximizing the efficacy of climate change initiatives, particularly in resource-constrained settings.
Furthermore, AI-powered climate risk assessment tools can facilitate real-time monitoring and response to climate-related threats. With the advent of the Internet of Things (IoT) and advanced sensor technologies, AI systems can continuously collect and analyze data from the environment. This capability enables the rapid detection of emerging risks, such as rising sea levels or increasing temperatures, allowing for timely interventions. For instance, AI can support early warning systems that alert communities to impending natural disasters, thereby reducing the potential for loss of life and property.
Despite the significant advantages offered by AI in climate risk assessment, it is important to acknowledge the challenges associated with its implementation. Issues such as data privacy, algorithmic bias, and the need for substantial computational resources must be addressed to ensure the ethical and equitable use of AI technologies. Additionally, collaboration between AI experts, climate scientists, policymakers, and local communities is essential to develop solutions that are both technically sound and socially acceptable.
In conclusion, AI-powered climate risk assessment represents a promising frontier in the fight against climate change. By enhancing our ability to predict, understand, and respond to climate risks, AI can play a pivotal role in safeguarding our planet for future generations. As we continue to refine these technologies and address associated challenges, the potential for AI to drive meaningful progress in climate resilience becomes increasingly apparent.
Enhancing Climate Resilience with AI Technologies
Artificial Intelligence (AI) has emerged as a transformative force across various sectors, and its potential to address climate change is increasingly being recognized. As the world grapples with the escalating impacts of climate change, AI technologies offer innovative solutions to enhance climate resilience. By leveraging AI, we can improve our ability to predict, mitigate, and adapt to the adverse effects of climate change, thereby safeguarding ecosystems and human communities.
To begin with, AI’s capacity for processing vast amounts of data with speed and accuracy is instrumental in climate modeling and prediction. Traditional climate models, while effective, often struggle with the complexity and scale of data required to make precise forecasts. AI algorithms, particularly machine learning models, can analyze extensive datasets from satellites, weather stations, and ocean buoys to identify patterns and trends that might elude conventional methods. This enhanced predictive capability allows for more accurate forecasting of extreme weather events, such as hurricanes, floods, and droughts, enabling communities to prepare and respond more effectively.
Moreover, AI technologies are pivotal in optimizing energy systems, which is crucial for reducing greenhouse gas emissions. Smart grids, powered by AI, can efficiently manage energy distribution by predicting demand and supply fluctuations. This not only minimizes energy waste but also integrates renewable energy sources more effectively into the grid. For instance, AI can forecast solar and wind energy production based on weather conditions, ensuring that these intermittent energy sources are utilized optimally. Consequently, this leads to a more sustainable energy infrastructure that supports climate resilience.
In addition to energy optimization, AI plays a significant role in monitoring and managing natural resources. Deforestation, a major contributor to climate change, can be mitigated through AI-driven satellite imagery analysis. By detecting illegal logging activities in real-time, AI systems enable authorities to take swift action to protect forests. Similarly, AI can monitor water resources, predicting shortages and optimizing irrigation systems to conserve water in agriculture. These applications not only help in preserving vital ecosystems but also ensure that communities dependent on these resources are better equipped to face climate challenges.
Transitioning to urban environments, AI technologies are being harnessed to create smarter, more resilient cities. Urban areas are particularly vulnerable to climate change due to their high population density and infrastructure demands. AI can enhance urban resilience by optimizing traffic flow to reduce emissions, managing waste more efficiently, and designing buildings that adapt to changing climate conditions. For example, AI can analyze data from sensors embedded in infrastructure to predict maintenance needs, thereby preventing failures that could be exacerbated by extreme weather.
Furthermore, AI’s role in climate resilience extends to agriculture, where it aids in developing climate-smart farming practices. Machine learning models can analyze soil health, weather patterns, and crop performance to provide farmers with actionable insights. This enables the adoption of precision agriculture techniques that increase yield while minimizing environmental impact. By ensuring food security in the face of climate change, AI contributes to the resilience of agricultural communities.
In conclusion, the integration of AI technologies into climate change strategies offers a promising pathway to enhance resilience. By improving predictive capabilities, optimizing resource management, and fostering sustainable practices, AI empowers societies to better withstand and adapt to the challenges posed by a changing climate. As we continue to innovate and refine these technologies, the potential for AI to drive meaningful progress in climate resilience becomes increasingly attainable.
Q&A
1. **Question:** How can AI improve climate change modeling and predictions?
**Answer:** AI can enhance climate change modeling by processing vast datasets to identify patterns and trends, improving the accuracy of climate predictions and enabling more precise forecasting of weather events and long-term climate shifts.
2. **Question:** In what ways can AI optimize energy consumption to combat climate change?
**Answer:** AI can optimize energy consumption by managing smart grids, predicting energy demand, and improving the efficiency of renewable energy sources, thereby reducing waste and lowering carbon emissions.
3. **Question:** How does AI contribute to monitoring deforestation and biodiversity loss?
**Answer:** AI contributes by analyzing satellite imagery and sensor data to detect changes in land use, track deforestation rates, and monitor biodiversity, enabling timely interventions and conservation efforts.
4. **Question:** What role does AI play in enhancing carbon capture and storage technologies?
**Answer:** AI aids in optimizing carbon capture processes by modeling chemical reactions, improving the efficiency of capture materials, and predicting the best storage sites, thus enhancing the overall effectiveness of carbon sequestration.
5. **Question:** How can AI assist in developing sustainable agriculture practices?
**Answer:** AI can assist by analyzing soil health, predicting crop yields, optimizing irrigation, and reducing pesticide use, leading to more sustainable and climate-resilient agricultural practices.
6. **Question:** In what ways can AI facilitate climate change adaptation strategies?
**Answer:** AI can facilitate adaptation by providing data-driven insights for infrastructure planning, disaster response, and resource management, helping communities to better prepare for and respond to climate impacts.Harnessing AI for climate change solutions presents a transformative opportunity to address one of the most pressing challenges of our time. AI technologies can enhance climate modeling, optimize energy systems, improve resource efficiency, and facilitate the transition to renewable energy sources. By enabling more accurate predictions and real-time data analysis, AI can help policymakers and businesses make informed decisions to mitigate the impacts of climate change. Furthermore, AI-driven innovations in agriculture, transportation, and urban planning can significantly reduce carbon footprints and promote sustainable practices. However, the deployment of AI must be approached with caution, ensuring ethical considerations and equitable access to technology. Collaborative efforts between governments, industries, and research institutions are essential to maximize the potential of AI in combating climate change. Ultimately, while AI is not a panacea, it is a powerful tool that, when integrated thoughtfully, can significantly contribute to global climate resilience and sustainability efforts.
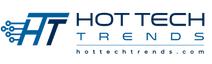