Enhancing safety in conversational AI agents is a critical focus in the development of artificial intelligence technologies. As these agents become increasingly integrated into daily life, from virtual assistants to customer service bots, ensuring their safe and ethical operation is paramount. Safety in this context encompasses a range of considerations, including the prevention of harmful interactions, the protection of user privacy, and the mitigation of biases that could lead to discriminatory outcomes. By implementing robust safety measures, developers can build trust with users and ensure that conversational AI systems operate within ethical and legal boundaries. This involves employing advanced techniques in natural language processing, machine learning, and data security, as well as adhering to established guidelines and standards. Ultimately, enhancing safety in conversational AI agents not only protects users but also fosters the responsible advancement of AI technologies.
Implementing Robust Data Privacy Measures
In the rapidly evolving landscape of conversational AI, ensuring robust data privacy measures is paramount to enhancing safety and maintaining user trust. As these AI agents become increasingly integrated into our daily lives, handling tasks ranging from customer service to personal assistance, the volume of sensitive data they process grows exponentially. Consequently, implementing stringent data privacy protocols is not merely a technical necessity but a moral imperative.
To begin with, the foundation of robust data privacy in conversational AI lies in the adoption of comprehensive data encryption techniques. Encryption serves as the first line of defense against unauthorized access, ensuring that data remains secure both in transit and at rest. By employing advanced encryption standards, developers can significantly mitigate the risk of data breaches, thereby safeguarding user information from potential cyber threats. Moreover, encryption should be complemented by secure authentication mechanisms, such as multi-factor authentication, to further fortify the system against unauthorized access.
In addition to encryption, anonymization of data is a critical strategy in protecting user privacy. By stripping data of personally identifiable information, developers can ensure that even if data is intercepted, it cannot be traced back to individual users. This process not only protects user identities but also complies with stringent data protection regulations such as the General Data Protection Regulation (GDPR) and the California Consumer Privacy Act (CCPA). These regulations mandate that organizations implement measures to protect user data, and anonymization is a key component in achieving compliance.
Furthermore, transparency in data handling practices is essential for building user trust. Users must be informed about what data is being collected, how it is being used, and who has access to it. Providing clear and concise privacy policies, along with options for users to control their data, empowers individuals to make informed decisions about their interactions with AI agents. This transparency not only enhances user trust but also aligns with ethical standards in AI development.
Another crucial aspect of implementing robust data privacy measures is the regular auditing and monitoring of AI systems. Continuous monitoring allows for the early detection of potential vulnerabilities and ensures that data privacy measures are functioning as intended. Regular audits, conducted by independent third parties, provide an additional layer of assurance that the AI systems adhere to established privacy standards and regulations. These audits can identify areas for improvement and help organizations stay ahead of emerging threats.
Moreover, fostering a culture of privacy within organizations is vital for the successful implementation of data privacy measures. This involves training employees on the importance of data privacy and equipping them with the necessary skills to handle data responsibly. By cultivating an environment where privacy is prioritized, organizations can ensure that all stakeholders are aligned in their commitment to protecting user data.
In conclusion, as conversational AI agents continue to permeate various aspects of our lives, implementing robust data privacy measures is essential for enhancing safety and maintaining user trust. Through encryption, anonymization, transparency, regular audits, and a culture of privacy, organizations can effectively safeguard user data and comply with regulatory requirements. As the field of AI continues to advance, prioritizing data privacy will remain a critical component in the development of safe and trustworthy AI systems.
Utilizing Advanced Natural Language Processing Techniques
In recent years, the rapid advancement of conversational AI agents has revolutionized the way humans interact with technology. These agents, powered by sophisticated natural language processing (NLP) techniques, have become integral in various applications, from customer service to personal assistants. However, as their prevalence increases, so does the need to ensure their safety and reliability. Enhancing safety in conversational AI agents is paramount, as it directly impacts user trust and the overall effectiveness of these systems.
To begin with, one of the primary concerns in conversational AI is the potential for generating harmful or inappropriate content. This issue arises from the vast datasets used to train these models, which may inadvertently include biased or offensive language. To mitigate this risk, developers are increasingly employing advanced NLP techniques such as sentiment analysis and content filtering. By analyzing the sentiment of a conversation, AI agents can detect and respond to negative or harmful language, thereby preventing the escalation of potentially harmful interactions. Moreover, content filtering algorithms can be designed to identify and block inappropriate language, ensuring that the AI maintains a respectful and safe dialogue with users.
In addition to content moderation, another critical aspect of enhancing safety in conversational AI is ensuring data privacy and security. As these agents often handle sensitive information, it is crucial to implement robust encryption and data protection measures. Advanced NLP techniques can be leveraged to anonymize user data, stripping away personally identifiable information while retaining the context necessary for meaningful interactions. Furthermore, employing secure data transmission protocols can safeguard against unauthorized access, thereby enhancing the overall security of conversational AI systems.
Transitioning to another vital consideration, the interpretability of AI models plays a significant role in ensuring their safety. As conversational agents become more complex, understanding their decision-making processes becomes increasingly challenging. To address this, researchers are developing explainable AI techniques that provide insights into how these models generate responses. By making AI systems more transparent, developers can identify and rectify potential biases or errors, ultimately leading to safer and more reliable conversational agents.
Moreover, continuous monitoring and updating of AI models are essential for maintaining their safety over time. The dynamic nature of language and societal norms necessitates regular updates to the datasets and algorithms used in conversational AI. By employing machine learning techniques such as reinforcement learning, AI agents can adapt to new information and evolving user expectations. This adaptability not only enhances the safety of these systems but also ensures their relevance and effectiveness in a rapidly changing world.
Furthermore, collaboration between AI developers, ethicists, and regulatory bodies is crucial in establishing comprehensive safety standards for conversational AI. By fostering an interdisciplinary approach, stakeholders can address the multifaceted challenges associated with AI safety, from ethical considerations to technical constraints. This collaborative effort can lead to the development of guidelines and best practices that ensure conversational AI agents operate within safe and ethical boundaries.
In conclusion, enhancing safety in conversational AI agents is a multifaceted endeavor that requires the integration of advanced NLP techniques, robust data protection measures, and continuous model evaluation. By prioritizing these aspects, developers can create AI systems that not only perform effectively but also uphold the highest standards of safety and reliability. As conversational AI continues to evolve, maintaining a focus on safety will be essential in fostering user trust and maximizing the potential of these transformative technologies.
Incorporating Real-Time Threat Detection Systems
In the rapidly evolving landscape of artificial intelligence, conversational AI agents have become increasingly prevalent, serving as virtual assistants, customer service representatives, and even companions. As these systems become more integrated into daily life, ensuring their safety and security is paramount. One promising approach to enhancing the safety of conversational AI agents is the incorporation of real-time threat detection systems. These systems are designed to identify and mitigate potential risks as they occur, thereby safeguarding both the AI and its users.
To begin with, real-time threat detection systems operate by continuously monitoring interactions between users and AI agents. This constant vigilance allows for the immediate identification of anomalies or suspicious activities that could indicate a security threat. For instance, if a user attempts to manipulate the AI into divulging sensitive information or executing unauthorized actions, the threat detection system can recognize these patterns and intervene. By doing so, it prevents potential breaches and maintains the integrity of the AI system.
Moreover, the integration of machine learning algorithms into these detection systems enhances their ability to identify threats. Machine learning models can be trained on vast datasets to recognize patterns associated with malicious behavior. As these models are exposed to more data over time, they become increasingly adept at distinguishing between benign and harmful interactions. This adaptability is crucial, as it allows the threat detection system to evolve alongside emerging threats, ensuring that the AI remains protected against new and sophisticated attack vectors.
In addition to identifying threats, real-time detection systems can also play a crucial role in mitigating them. Once a potential threat is detected, the system can take immediate action to neutralize it. This might involve temporarily suspending the AI’s operations, alerting human supervisors, or even engaging in a dialogue with the user to verify their intentions. By taking swift and decisive action, these systems can prevent threats from escalating and causing harm.
Furthermore, the implementation of real-time threat detection systems can also enhance user trust in conversational AI agents. Users are more likely to engage with AI systems that they perceive as secure and reliable. By demonstrating a commitment to safety through the use of advanced threat detection technologies, developers can foster greater confidence among users. This, in turn, can lead to increased adoption and utilization of AI agents across various sectors.
However, it is important to acknowledge the challenges associated with incorporating real-time threat detection systems into conversational AI. One significant challenge is the potential for false positives, where benign interactions are mistakenly flagged as threats. This can lead to unnecessary disruptions and frustrate users. To address this issue, developers must strike a balance between sensitivity and specificity in their detection algorithms, ensuring that they are both accurate and efficient.
In conclusion, the incorporation of real-time threat detection systems represents a significant advancement in enhancing the safety of conversational AI agents. By continuously monitoring interactions, leveraging machine learning, and taking swift action to mitigate threats, these systems can protect both the AI and its users from potential harm. While challenges remain, particularly in minimizing false positives, the benefits of real-time threat detection are clear. As AI continues to play an increasingly prominent role in society, ensuring its safety through such innovative measures will be essential for fostering trust and promoting widespread adoption.
Ensuring Compliance with Regulatory Standards
In the rapidly evolving landscape of artificial intelligence, conversational AI agents have emerged as pivotal tools in various sectors, from customer service to healthcare. As these technologies become increasingly integrated into daily life, ensuring their compliance with regulatory standards is paramount. This compliance not only safeguards user privacy and data security but also enhances the overall safety and reliability of AI systems. To achieve this, developers and organizations must navigate a complex web of regulations that vary across jurisdictions, necessitating a comprehensive understanding of both local and international standards.
One of the primary regulatory frameworks that conversational AI agents must adhere to is data protection legislation. The General Data Protection Regulation (GDPR) in the European Union, for instance, sets stringent requirements for data handling, emphasizing user consent and the right to access and erase personal data. Similarly, the California Consumer Privacy Act (CCPA) in the United States mandates transparency in data collection and usage. Compliance with these regulations is crucial, as failure to do so can result in hefty fines and damage to an organization’s reputation. Therefore, AI developers must implement robust data management practices, ensuring that personal information is collected, stored, and processed in a manner that aligns with these legal requirements.
In addition to data protection, conversational AI agents must also comply with industry-specific regulations. In the healthcare sector, for example, AI systems must adhere to the Health Insurance Portability and Accountability Act (HIPAA) in the United States, which governs the privacy and security of health information. This involves implementing stringent access controls and encryption measures to protect sensitive patient data. Similarly, in the financial sector, AI agents must comply with regulations such as the Payment Card Industry Data Security Standard (PCI DSS), which ensures the secure handling of credit card information. By aligning with these industry-specific standards, organizations can mitigate risks and enhance the trustworthiness of their AI solutions.
Moreover, ethical considerations play a crucial role in ensuring compliance with regulatory standards. As conversational AI agents interact with users, they must be designed to avoid biases and discriminatory behavior. This requires developers to implement fairness and transparency in AI algorithms, ensuring that decisions made by these systems are explainable and justifiable. Regulatory bodies are increasingly focusing on these ethical aspects, with guidelines such as the European Commission’s Ethics Guidelines for Trustworthy AI providing a framework for responsible AI development. By adhering to these guidelines, organizations can foster public trust and acceptance of AI technologies.
Furthermore, ongoing monitoring and auditing of conversational AI systems are essential to maintain compliance with regulatory standards. As regulations evolve and new threats emerge, organizations must continuously assess and update their AI systems to address potential vulnerabilities. This involves conducting regular audits, implementing feedback mechanisms, and staying informed about changes in the regulatory landscape. By adopting a proactive approach to compliance, organizations can ensure that their AI agents remain safe and effective over time.
In conclusion, enhancing safety in conversational AI agents through compliance with regulatory standards is a multifaceted endeavor that requires a thorough understanding of data protection laws, industry-specific regulations, and ethical considerations. By implementing robust data management practices, aligning with industry standards, addressing ethical concerns, and maintaining ongoing monitoring, organizations can ensure that their AI systems are not only compliant but also trustworthy and reliable. As the field of AI continues to advance, prioritizing compliance will be essential in fostering a safe and secure environment for users and stakeholders alike.
Developing User-Friendly Safety Features
In the rapidly evolving landscape of artificial intelligence, conversational AI agents have become increasingly prevalent, offering users a seamless and interactive experience across various platforms. As these agents become more integrated into daily life, ensuring their safety and reliability has emerged as a paramount concern. Developing user-friendly safety features is essential to fostering trust and enhancing the overall user experience. This involves a multifaceted approach that combines technological innovation with a deep understanding of user needs and behaviors.
To begin with, one of the primary considerations in enhancing safety is the implementation of robust data privacy measures. Users interacting with conversational AI agents often share sensitive information, whether consciously or inadvertently. Therefore, it is crucial to establish stringent data protection protocols that safeguard user data from unauthorized access and misuse. Employing advanced encryption techniques and ensuring compliance with data protection regulations, such as the General Data Protection Regulation (GDPR), can significantly mitigate privacy risks. Moreover, transparency in data handling practices can further bolster user confidence, as individuals are more likely to trust systems that clearly communicate how their data is being used and protected.
In addition to data privacy, another critical aspect of safety in conversational AI is the prevention of harmful interactions. AI agents must be equipped with the ability to recognize and respond appropriately to potentially harmful or abusive language. This requires the integration of sophisticated natural language processing algorithms that can detect and filter out inappropriate content. By doing so, AI agents can maintain a respectful and safe environment for all users. Furthermore, incorporating user feedback mechanisms allows individuals to report any concerning interactions, enabling continuous improvement of the system’s safety features.
Transitioning from the technical to the human-centric aspect, user education plays a pivotal role in enhancing safety. Educating users about the capabilities and limitations of conversational AI agents can empower them to make informed decisions during interactions. Providing clear guidelines on how to engage with AI agents safely, as well as highlighting potential risks, can help users navigate these technologies more effectively. Additionally, offering tutorials or interactive demonstrations can demystify the technology, making it more accessible and less intimidating for users of varying technical proficiencies.
Moreover, fostering inclusivity in the design of conversational AI agents is essential for ensuring safety across diverse user groups. This involves considering the unique needs and preferences of different demographics, including those with disabilities or language barriers. By incorporating features such as voice recognition for various accents and dialects, as well as offering multilingual support, AI agents can provide a more inclusive and equitable user experience. This not only enhances safety by reducing misunderstandings but also broadens the reach and utility of these technologies.
Finally, continuous monitoring and evaluation of AI systems are indispensable for maintaining and improving safety standards. Regular audits and updates can help identify potential vulnerabilities and ensure that safety features remain effective in the face of evolving threats. Engaging with a community of developers, researchers, and users can facilitate the sharing of best practices and innovative solutions, driving the collective advancement of safety in conversational AI.
In conclusion, developing user-friendly safety features in conversational AI agents is a complex yet essential endeavor. By prioritizing data privacy, preventing harmful interactions, educating users, fostering inclusivity, and committing to ongoing evaluation, developers can create AI systems that are not only safe but also trusted and valued by users worldwide. As the field continues to advance, these efforts will be crucial in shaping a future where conversational AI agents are seamlessly integrated into society, enhancing rather than compromising user safety.
Conducting Regular Security Audits and Updates
In the rapidly evolving landscape of artificial intelligence, conversational AI agents have become integral to various sectors, from customer service to healthcare. As these systems become more sophisticated, ensuring their safety and security is paramount. One of the most effective strategies to enhance the safety of conversational AI agents is conducting regular security audits and updates. This approach not only safeguards sensitive data but also fortifies the AI systems against potential threats.
To begin with, regular security audits serve as a critical tool in identifying vulnerabilities within AI systems. These audits involve a comprehensive examination of the AI’s architecture, data handling processes, and interaction protocols. By systematically evaluating these components, organizations can detect weaknesses that could be exploited by malicious actors. For instance, an audit might reveal inadequate encryption methods or insufficient access controls, both of which could compromise the integrity of the AI system. Consequently, addressing these vulnerabilities promptly is essential to maintaining the security of conversational AI agents.
Moreover, security audits provide valuable insights into the AI’s compliance with industry standards and regulations. As data privacy laws become increasingly stringent, ensuring that AI systems adhere to these regulations is crucial. Regular audits help organizations verify that their AI agents are operating within legal frameworks, thereby avoiding potential legal repercussions. Furthermore, these audits can highlight areas where the AI system may need to be updated to align with new regulatory requirements, ensuring ongoing compliance and enhancing the system’s overall safety.
In addition to audits, implementing regular updates is vital for maintaining the security of conversational AI agents. The dynamic nature of cybersecurity threats necessitates that AI systems are continually updated to counteract new risks. These updates often include patches for known vulnerabilities, enhancements to existing security features, and the integration of advanced security technologies. By keeping the AI system up-to-date, organizations can protect against emerging threats and ensure that their AI agents remain resilient in the face of evolving challenges.
Transitioning from the technical aspects, it is also important to consider the human element in conducting security audits and updates. Training personnel to recognize and respond to security threats is an essential component of a comprehensive security strategy. By fostering a culture of security awareness, organizations can empower their teams to proactively identify potential risks and take appropriate action. This human-centric approach complements the technical measures, creating a robust defense against security breaches.
Furthermore, collaboration between different departments within an organization can enhance the effectiveness of security audits and updates. By involving IT, legal, and operational teams in the audit process, organizations can ensure a holistic approach to security. Each department brings unique insights and expertise, contributing to a more thorough understanding of the AI system’s security posture. This collaborative effort not only strengthens the security framework but also promotes a unified organizational commitment to safeguarding AI systems.
In conclusion, conducting regular security audits and updates is a fundamental practice for enhancing the safety of conversational AI agents. By identifying vulnerabilities, ensuring compliance with regulations, and implementing timely updates, organizations can protect their AI systems from potential threats. Additionally, fostering a culture of security awareness and encouraging interdepartmental collaboration further bolsters the security measures in place. As conversational AI continues to advance, prioritizing these practices will be essential in maintaining the trust and reliability of these intelligent systems.
Q&A
1. **What is a key method to enhance safety in conversational AI agents?**
Implementing robust content filtering and moderation systems to detect and prevent harmful or inappropriate language.
2. **How can user privacy be protected in conversational AI interactions?**
By employing strong data encryption and anonymization techniques to safeguard personal information.
3. **What role does user feedback play in improving AI safety?**
User feedback helps identify potential safety issues and biases, allowing developers to make necessary adjustments and improvements.
4. **Why is it important to regularly update conversational AI models?**
Regular updates ensure that the AI can adapt to new safety standards, language changes, and emerging threats.
5. **How can bias be reduced in conversational AI systems?**
By training models on diverse datasets and continuously monitoring for biased outputs to make corrective adjustments.
6. **What is a proactive approach to prevent AI from generating harmful content?**
Implementing preemptive safety layers, such as ethical guidelines and scenario-based testing, to anticipate and mitigate potential risks.Enhancing safety in conversational AI agents is crucial to ensure user trust, privacy, and security. As these agents become increasingly integrated into daily life, they must be designed to handle sensitive information responsibly, avoid harmful interactions, and provide accurate responses. Implementing robust data protection measures, continuous monitoring for inappropriate content, and incorporating ethical guidelines are essential steps. Additionally, fostering transparency in AI decision-making processes and enabling user control over data usage can further bolster safety. By prioritizing these aspects, developers can create conversational AI agents that are not only effective but also safe and reliable for users.
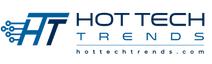