In a groundbreaking advancement, researchers have harnessed the power of deep learning to unveil millions of previously unknown materials, marking a significant leap forward in materials science. This breakthrough leverages sophisticated algorithms and vast datasets to predict the properties and potential applications of new compounds with unprecedented accuracy and speed. By transcending traditional trial-and-error methods, this innovation not only accelerates the discovery process but also opens up new avenues for technological advancements across various industries, including electronics, energy, and pharmaceuticals. The integration of deep learning into materials discovery promises to revolutionize the way scientists approach the development of new materials, paving the way for innovations that could address some of the world’s most pressing challenges.
Revolutionizing Material Science: The Role of Deep Learning in Discovering New Materials
In recent years, the field of material science has witnessed a transformative shift, largely driven by the advent of deep learning technologies. This breakthrough in deep learning has unveiled millions of new materials, offering unprecedented opportunities for innovation across various industries. The integration of artificial intelligence, particularly deep learning, into material science has revolutionized the way researchers approach the discovery and development of new materials. Traditionally, the process of discovering new materials has been labor-intensive and time-consuming, often involving trial-and-error methods that could take years, if not decades, to yield significant results. However, with the advent of deep learning, researchers can now analyze vast datasets with remarkable speed and accuracy, identifying potential new materials in a fraction of the time previously required.
Deep learning, a subset of machine learning, involves the use of neural networks with multiple layers to model complex patterns in data. This technology has proven particularly effective in material science, where the properties and behaviors of materials can be influenced by a multitude of factors. By training deep learning models on extensive datasets, researchers can predict the properties of new materials with a high degree of accuracy, thus streamlining the discovery process. Moreover, deep learning algorithms can identify patterns and relationships within data that may not be immediately apparent to human researchers, leading to the discovery of materials with novel properties and applications.
The implications of this breakthrough are far-reaching. In the realm of energy, for instance, the discovery of new materials could lead to the development of more efficient solar panels, batteries, and fuel cells, thereby advancing the transition to renewable energy sources. In the medical field, new materials could be used to create more effective drug delivery systems or biocompatible implants, improving patient outcomes and quality of life. Furthermore, in the electronics industry, the development of materials with superior conductive properties could pave the way for faster and more efficient electronic devices.
In addition to these practical applications, the use of deep learning in material science also holds promise for addressing some of the most pressing challenges facing society today. For example, the development of materials that can capture and store carbon dioxide more effectively could play a crucial role in mitigating the impacts of climate change. Similarly, the discovery of materials that can withstand extreme conditions could enhance the safety and resilience of infrastructure in disaster-prone areas.
Despite these promising developments, it is important to acknowledge the challenges that remain. The accuracy of deep learning models is heavily dependent on the quality and quantity of data available, and there is a need for continued investment in data collection and curation. Additionally, while deep learning can significantly accelerate the discovery process, the practical implementation of new materials still requires rigorous testing and validation to ensure their safety and efficacy.
In conclusion, the integration of deep learning into material science represents a significant breakthrough with the potential to revolutionize the field. By enabling the rapid discovery of millions of new materials, deep learning is opening up new possibilities for innovation and addressing some of the most critical challenges facing society today. As researchers continue to refine these technologies and expand their applications, the future of material science looks increasingly promising, heralding a new era of discovery and development.
How Deep Learning is Transforming the Search for Innovative Materials
The advent of deep learning has revolutionized numerous fields, from healthcare to finance, and now it is making significant strides in the realm of materials science. This transformation is particularly evident in the discovery and development of new materials, a process traditionally characterized by time-consuming experimentation and serendipitous findings. However, with the integration of deep learning, researchers are now able to expedite this process, unveiling millions of new materials with unprecedented efficiency and accuracy.
Deep learning, a subset of artificial intelligence, involves training neural networks to recognize patterns and make predictions based on vast datasets. In the context of materials science, these datasets comprise chemical compositions, structural properties, and performance metrics of known materials. By analyzing these datasets, deep learning models can identify potential new materials that exhibit desired properties, such as enhanced strength, conductivity, or thermal resistance. This capability is particularly valuable in industries ranging from electronics to aerospace, where the demand for innovative materials is ever-increasing.
One of the most significant breakthroughs facilitated by deep learning is the ability to predict the properties of materials before they are synthesized. Traditionally, researchers relied on trial-and-error methods, which were not only labor-intensive but also limited by human intuition. In contrast, deep learning models can process and analyze data at a scale and speed far beyond human capabilities, enabling the identification of promising materials that might otherwise remain undiscovered. This predictive power is transforming the way scientists approach materials discovery, shifting the focus from empirical experimentation to data-driven exploration.
Moreover, deep learning is enhancing the efficiency of materials design by optimizing the synthesis process. By predicting the optimal conditions for creating a material with specific properties, these models reduce the need for extensive laboratory testing. This not only accelerates the development timeline but also minimizes resource consumption, making the process more sustainable. Furthermore, the ability to simulate various environmental conditions allows researchers to assess the durability and performance of materials in real-world applications, ensuring that only the most viable candidates proceed to the production stage.
In addition to accelerating discovery and design, deep learning is fostering collaboration across disciplines. The complexity of materials science often necessitates expertise from chemistry, physics, and engineering. Deep learning models serve as a common platform where insights from these diverse fields can be integrated and leveraged. This interdisciplinary approach is crucial for tackling complex challenges, such as developing materials for renewable energy solutions or creating biodegradable alternatives to plastics.
Despite these advancements, the integration of deep learning in materials science is not without challenges. The accuracy of predictions depends heavily on the quality and diversity of the input data. Incomplete or biased datasets can lead to erroneous conclusions, underscoring the importance of comprehensive data collection and curation. Additionally, the interpretability of deep learning models remains a concern, as the “black box” nature of these algorithms can obscure the rationale behind their predictions. Addressing these issues is essential for building trust and ensuring the reliability of deep learning applications in materials science.
In conclusion, deep learning is transforming the search for innovative materials by enhancing the speed, efficiency, and scope of discovery and design processes. As researchers continue to refine these models and overcome existing challenges, the potential for uncovering new materials with groundbreaking properties is immense. This technological synergy not only promises to advance scientific understanding but also holds the potential to drive significant industrial and societal progress.
The Impact of AI-Driven Discoveries on Material Innovation
The advent of deep learning has ushered in a new era of material innovation, fundamentally transforming the landscape of scientific discovery. Recent breakthroughs in artificial intelligence have enabled researchers to identify millions of new materials, a feat that was previously unimaginable. This remarkable development is poised to revolutionize various industries, from electronics to pharmaceuticals, by providing unprecedented access to novel materials with unique properties.
At the heart of this transformation is the ability of deep learning algorithms to process vast amounts of data with remarkable speed and accuracy. These algorithms, inspired by the neural networks of the human brain, are capable of identifying patterns and making predictions that were once the exclusive domain of human experts. By leveraging these capabilities, scientists can now explore the vast chemical space more efficiently, uncovering materials that exhibit desirable characteristics such as enhanced conductivity, improved strength, or increased thermal stability.
One of the most significant impacts of AI-driven discoveries in material science is the acceleration of the research and development process. Traditionally, the discovery of new materials has been a time-consuming and labor-intensive endeavor, often involving trial-and-error experimentation. However, with the integration of deep learning, researchers can now simulate and predict the properties of potential materials before they are synthesized in the laboratory. This predictive capability not only reduces the time and cost associated with material development but also allows for the exploration of a broader range of possibilities.
Moreover, the application of deep learning in material science is not limited to the discovery of new materials. It also plays a crucial role in optimizing existing materials for specific applications. For instance, by analyzing the performance data of materials under various conditions, AI algorithms can suggest modifications that enhance their functionality or durability. This ability to fine-tune materials to meet specific requirements is particularly valuable in industries such as aerospace and automotive, where performance and safety are paramount.
In addition to these practical benefits, the integration of AI in material science also fosters a more sustainable approach to innovation. By enabling the discovery of materials with superior properties, deep learning can contribute to the development of more efficient and environmentally friendly technologies. For example, the identification of new catalysts can lead to more efficient chemical reactions, reducing energy consumption and minimizing waste. Similarly, the discovery of lightweight yet strong materials can result in more fuel-efficient vehicles, thereby decreasing carbon emissions.
Despite these promising advancements, the integration of AI in material science is not without its challenges. The complexity of chemical interactions and the vastness of the chemical space present significant hurdles that require sophisticated algorithms and high computational power. Furthermore, the interpretability of AI models remains a critical issue, as understanding the rationale behind AI-driven predictions is essential for gaining scientific insights and ensuring the reliability of the results.
Nevertheless, the potential benefits of AI-driven discoveries in material science far outweigh these challenges. As deep learning algorithms continue to evolve and improve, they will undoubtedly unlock even more possibilities, paving the way for innovations that were once beyond our reach. In conclusion, the breakthrough in deep learning that has unveiled millions of new materials marks a pivotal moment in the field of material science, offering a glimpse into a future where AI-driven discoveries drive unprecedented advancements across a multitude of industries.
Exploring the Intersection of Deep Learning and Material Science
The intersection of deep learning and material science has recently witnessed a groundbreaking development, unveiling millions of new materials with potential applications across various industries. This breakthrough is a testament to the transformative power of artificial intelligence in scientific research, offering unprecedented opportunities for innovation and discovery. As researchers continue to explore the vast landscape of material science, deep learning emerges as a pivotal tool, enabling the identification and synthesis of novel materials with remarkable efficiency and accuracy.
Traditionally, the discovery of new materials has been a labor-intensive and time-consuming process, often relying on trial and error. Scientists would painstakingly experiment with different combinations of elements, hoping to stumble upon a material with desirable properties. However, the advent of deep learning has revolutionized this approach, allowing researchers to predict the properties of materials before they are physically synthesized. By training algorithms on vast datasets of known materials, deep learning models can now identify patterns and relationships that were previously hidden, thus accelerating the discovery process.
One of the most significant advantages of using deep learning in material science is its ability to handle complex, high-dimensional data. Materials are characterized by a multitude of properties, such as electrical conductivity, thermal stability, and mechanical strength, which are influenced by their atomic structure and composition. Deep learning models, particularly neural networks, excel at processing such intricate data, enabling them to predict how changes in composition or structure might affect a material’s properties. This capability not only speeds up the discovery of new materials but also enhances the understanding of existing ones.
Moreover, the integration of deep learning with high-throughput computational techniques has further amplified its impact on material science. High-throughput methods allow researchers to simulate thousands of material combinations simultaneously, generating vast amounts of data that can be fed into deep learning models. This synergy between computational power and artificial intelligence has led to the identification of millions of new materials, each with unique properties and potential applications. From superconductors and semiconductors to lightweight alloys and advanced polymers, the possibilities are virtually limitless.
In addition to accelerating material discovery, deep learning also plays a crucial role in optimizing the synthesis and processing of materials. By analyzing data from previous experiments, deep learning models can suggest optimal conditions for synthesizing a material, such as temperature, pressure, and reaction time. This predictive capability reduces the need for costly and time-consuming experimental trials, making the development of new materials more efficient and sustainable.
Furthermore, the implications of this breakthrough extend beyond the realm of material science. The ability to rapidly discover and develop new materials has far-reaching consequences for industries such as electronics, energy, and healthcare. For instance, the development of new semiconductors could lead to more efficient electronic devices, while advanced materials for energy storage could revolutionize battery technology. In healthcare, biocompatible materials could pave the way for innovative medical implants and devices.
In conclusion, the integration of deep learning into material science represents a paradigm shift in how new materials are discovered and developed. By harnessing the power of artificial intelligence, researchers can explore the vast potential of material science with unprecedented speed and precision. As this field continues to evolve, it promises to unlock new frontiers of innovation, driving progress across a multitude of industries and ultimately enhancing the quality of life for people around the world.
Unveiling New Materials: The Power of Deep Learning Algorithms
The recent breakthrough in deep learning has ushered in a new era of material discovery, revealing millions of previously unknown materials with potential applications across various industries. This advancement is largely attributed to the development of sophisticated deep learning algorithms, which have significantly enhanced our ability to predict and identify new materials with desirable properties. As researchers continue to explore the vast landscape of material science, the integration of deep learning techniques has proven to be a game-changer, offering unprecedented insights and accelerating the pace of discovery.
Traditionally, the discovery of new materials has been a labor-intensive and time-consuming process, often involving trial-and-error experimentation and extensive computational modeling. However, the advent of deep learning has transformed this landscape by enabling the analysis of vast datasets with remarkable speed and accuracy. By training algorithms on existing material databases, researchers can now predict the properties of unknown compounds, thereby streamlining the discovery process. This capability not only reduces the time required to identify promising materials but also expands the scope of exploration to include a broader range of chemical compositions and structures.
One of the key advantages of deep learning in material discovery is its ability to identify complex patterns and relationships within data that may not be immediately apparent to human researchers. By leveraging neural networks, these algorithms can process and analyze multidimensional data, uncovering hidden correlations that can lead to the identification of novel materials. This ability to discern intricate patterns is particularly valuable in the field of material science, where the properties of a material are often influenced by a multitude of factors, including atomic structure, bonding, and electronic configuration.
Moreover, deep learning algorithms have demonstrated remarkable versatility in their application to various domains within material science. For instance, they have been employed to predict the mechanical properties of materials, such as strength and elasticity, as well as their thermal and electrical conductivities. This versatility extends to the discovery of materials with specific functionalities, such as superconductors, catalysts, and energy storage materials. By tailoring the algorithms to target specific properties, researchers can efficiently identify materials that meet the desired criteria, thereby facilitating the development of innovative technologies.
In addition to accelerating the discovery process, deep learning also holds promise for optimizing existing materials. By analyzing the properties of known materials, algorithms can suggest modifications to enhance their performance or reduce their environmental impact. This capability is particularly relevant in the context of sustainable development, where the demand for eco-friendly materials is on the rise. By optimizing materials for sustainability, deep learning can contribute to the development of greener technologies and more efficient resource utilization.
As the field of deep learning continues to evolve, its integration into material science is expected to yield even more significant breakthroughs. The ongoing refinement of algorithms and the expansion of material databases will likely enhance the accuracy and predictive power of these tools, further accelerating the pace of discovery. Moreover, the collaboration between material scientists and computer scientists will be crucial in harnessing the full potential of deep learning, ensuring that these advancements translate into tangible benefits for society.
In conclusion, the breakthrough in deep learning has revolutionized the field of material discovery, unveiling millions of new materials with potential applications across various industries. By leveraging the power of deep learning algorithms, researchers can efficiently predict and identify materials with desirable properties, thereby accelerating the pace of innovation. As this technology continues to advance, it holds the promise of transforming material science and contributing to the development of sustainable and cutting-edge technologies.
The Future of Material Discovery: Deep Learning’s Breakthroughs and Implications
In recent years, the field of material science has witnessed a transformative shift, largely driven by the advent of deep learning technologies. This breakthrough has unveiled millions of new materials, promising to revolutionize industries ranging from electronics to pharmaceuticals. Deep learning, a subset of artificial intelligence, involves training neural networks to recognize patterns and make predictions based on vast datasets. Its application in material discovery is proving to be a game-changer, as it allows researchers to explore the vast chemical space more efficiently than traditional methods.
Traditionally, the discovery of new materials has been a labor-intensive and time-consuming process, often involving trial and error. Researchers would synthesize and test materials in the lab, a method that, while effective, is limited by human capacity and the constraints of physical experimentation. However, with the integration of deep learning, this process is being accelerated exponentially. By training algorithms on existing material databases, scientists can predict the properties of unknown compounds, thus identifying promising candidates for further study without the need for immediate physical synthesis.
Moreover, deep learning models can handle complex, multidimensional data, making them particularly suited for material science applications. These models can analyze the relationships between a material’s structure and its properties, providing insights that were previously unattainable. For instance, they can predict how a change in molecular structure might affect a material’s conductivity or strength, allowing researchers to tailor materials for specific applications. This capability not only speeds up the discovery process but also opens up possibilities for creating materials with unprecedented properties.
The implications of this breakthrough are far-reaching. In the electronics industry, for example, the discovery of new semiconductors could lead to more efficient and powerful devices. Similarly, in the field of renewable energy, novel materials could enhance the efficiency of solar panels or improve the storage capacity of batteries. The pharmaceutical industry also stands to benefit, as deep learning can aid in the identification of new compounds with potential therapeutic effects, thus accelerating drug discovery and development.
Furthermore, the environmental impact of material production is a growing concern, and deep learning offers a pathway to more sustainable practices. By predicting the environmental footprint of new materials before they are synthesized, researchers can prioritize those with lower ecological impacts. This approach aligns with the global push towards sustainability and responsible innovation, ensuring that technological advancements do not come at the expense of the planet.
Despite these promising developments, challenges remain. The accuracy of deep learning models depends heavily on the quality and quantity of data available. Incomplete or biased datasets can lead to erroneous predictions, underscoring the need for comprehensive and diverse data collection. Additionally, the interpretability of deep learning models is a topic of ongoing research. While these models can make accurate predictions, understanding the underlying reasons for these predictions is crucial for gaining scientific insights and ensuring trust in the technology.
In conclusion, the integration of deep learning into material discovery marks a significant milestone in the field of material science. By unveiling millions of new materials, this technology holds the potential to drive innovation across various industries, paving the way for advancements that were once thought to be out of reach. As researchers continue to refine these models and address existing challenges, the future of material discovery looks promising, heralding a new era of scientific exploration and technological progress.
Q&A
1. **What is the breakthrough in deep learning related to materials?**
The breakthrough involves using advanced deep learning algorithms to predict and discover millions of new materials with unique properties, significantly accelerating the materials discovery process.
2. **How does deep learning contribute to materials discovery?**
Deep learning models can analyze vast datasets of known materials and their properties, learning complex patterns and relationships that allow them to predict the properties of hypothetical new materials.
3. **What are the potential applications of these newly discovered materials?**
These materials could be used in various fields, including electronics, energy storage, pharmaceuticals, and construction, leading to innovations like more efficient batteries, stronger building materials, and new drug compounds.
4. **What challenges does this breakthrough address in traditional materials science?**
It addresses the time-consuming and costly trial-and-error process of traditional materials discovery by providing a faster, more efficient method to identify promising new materials.
5. **Who are the key players involved in this breakthrough?**
The breakthrough typically involves collaborations between academic institutions, research labs, and technology companies specializing in artificial intelligence and materials science.
6. **What is the future impact of this breakthrough on industry and research?**
The ability to rapidly discover new materials could lead to significant advancements in technology and industry, fostering innovation and potentially leading to new products and solutions to global challenges.The breakthrough in deep learning that has unveiled millions of new materials represents a significant advancement in materials science and artificial intelligence. By leveraging sophisticated algorithms and vast datasets, researchers can now predict and discover novel materials with unprecedented speed and accuracy. This development holds the potential to revolutionize various industries, including electronics, energy, and pharmaceuticals, by enabling the creation of materials with tailored properties and enhanced performance. The integration of deep learning into materials discovery not only accelerates the innovation process but also opens new avenues for sustainable and efficient solutions to global challenges.
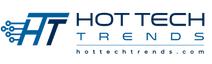