Deprecated: Implicit conversion from float 16.8 to int loses precision in /home/hottech/public_html/wp-content/plugins/internal-links/core/links/text-to-link-converter-factory.php on line 35
Deprecated: Implicit conversion from float 16.1 to int loses precision in /home/hottech/public_html/wp-content/plugins/internal-links/core/links/text-to-link-converter-factory.php on line 35
**AlphaFold 3: Unveiling the Structure and Interactions of Life’s Molecules**
AlphaFold 3 represents a groundbreaking advancement in the field of computational biology, building upon the revolutionary success of its predecessors in predicting protein structures with remarkable accuracy. This latest iteration not only enhances the precision of structural predictions but also delves deeper into understanding the complex interactions and dynamics of life’s molecular machinery. By leveraging cutting-edge machine learning techniques and vast datasets, AlphaFold 3 offers unprecedented insights into the intricate world of proteins, enzymes, and other biomolecules, paving the way for transformative developments in drug discovery, disease understanding, and synthetic biology. As it continues to unravel the mysteries of molecular interactions, AlphaFold 3 stands at the forefront of a new era in biological research, promising to unlock the secrets of life at the molecular level.
Advances In Protein Structure Prediction With AlphaFold 3
The field of protein structure prediction has witnessed remarkable advancements over the past few years, with AlphaFold emerging as a groundbreaking tool in this domain. The introduction of AlphaFold 3 marks a significant leap forward, building upon the successes of its predecessors and offering unprecedented insights into the intricate world of protein structures and their interactions. This latest iteration of AlphaFold not only enhances the accuracy of protein structure predictions but also expands its capabilities to encompass a broader range of biological molecules, thereby opening new avenues for scientific exploration and discovery.
AlphaFold 3’s development is rooted in the sophisticated application of artificial intelligence and machine learning techniques. By leveraging vast datasets of known protein structures, the system has been trained to predict the three-dimensional shapes of proteins with remarkable precision. This is achieved through the integration of advanced neural networks that can discern complex patterns and relationships within the data, allowing AlphaFold 3 to generate highly accurate models of protein structures. The implications of this are profound, as understanding the precise configuration of proteins is crucial for elucidating their functions and interactions within biological systems.
One of the most notable advancements in AlphaFold 3 is its ability to predict not only individual protein structures but also the interactions between multiple proteins. This capability is essential for comprehending the dynamic nature of cellular processes, where proteins often function in concert with one another. By simulating these interactions, AlphaFold 3 provides researchers with a powerful tool to investigate the molecular mechanisms underlying various biological phenomena. This, in turn, has the potential to accelerate the development of novel therapeutics and treatments for a wide array of diseases, as many pathological conditions are linked to aberrant protein interactions.
Moreover, AlphaFold 3’s expanded scope includes the prediction of structures for other biologically relevant molecules, such as RNA and small molecules. This broadens the applicability of the tool, enabling scientists to explore the structural basis of a wider range of molecular interactions. Consequently, this advancement holds promise for enhancing our understanding of complex biological systems and facilitating the design of more effective drugs and therapies.
The impact of AlphaFold 3 extends beyond the realm of basic research, as it also offers practical benefits for industries such as pharmaceuticals and biotechnology. By providing accurate structural predictions, AlphaFold 3 can streamline the drug discovery process, reducing the time and resources required to identify potential drug candidates. Furthermore, its ability to model protein interactions can aid in the design of more targeted and efficient therapeutic interventions, ultimately improving patient outcomes.
In conclusion, AlphaFold 3 represents a monumental step forward in the field of protein structure prediction, offering unparalleled insights into the architecture and interactions of life’s molecules. Its advanced capabilities not only enhance our understanding of fundamental biological processes but also hold significant promise for transforming the landscape of drug discovery and development. As researchers continue to harness the power of AlphaFold 3, it is poised to become an indispensable tool in the quest to unravel the complexities of the molecular world and address some of the most pressing challenges in medicine and biology.
AlphaFold 3: Revolutionizing Drug Discovery And Development
AlphaFold 3 represents a significant leap forward in the field of computational biology, particularly in the realm of drug discovery and development. Building upon the groundbreaking success of its predecessors, AlphaFold 3 has further refined the ability to predict protein structures with remarkable accuracy. This advancement is not merely an academic achievement; it holds profound implications for the pharmaceutical industry and the broader scientific community. By providing detailed insights into the three-dimensional structures of proteins, AlphaFold 3 enables researchers to understand the intricate interactions that govern biological processes, thereby accelerating the identification of potential drug targets.
The process of drug discovery has traditionally been a time-consuming and costly endeavor, often taking years of research and billions of dollars to bring a new drug to market. A significant portion of this effort is dedicated to understanding the structure and function of proteins, which are the primary targets for most therapeutic agents. Proteins are complex molecules that perform a vast array of functions within living organisms, and their activity is largely determined by their three-dimensional shape. Misfolded proteins can lead to diseases, making the ability to predict their structure crucial for developing effective treatments. AlphaFold 3 addresses this challenge by providing highly accurate predictions of protein structures, thus reducing the need for labor-intensive experimental methods such as X-ray crystallography and nuclear magnetic resonance spectroscopy.
Moreover, AlphaFold 3’s capabilities extend beyond static structure prediction. It offers insights into the dynamic nature of proteins, capturing the subtle conformational changes that occur as proteins interact with other molecules. This dynamic perspective is essential for understanding how drugs bind to their targets and exert their effects. By simulating these interactions, AlphaFold 3 allows researchers to identify potential binding sites and optimize drug candidates with greater precision. This capability not only enhances the efficiency of the drug design process but also increases the likelihood of developing more effective and safer therapeutics.
In addition to its impact on drug discovery, AlphaFold 3 is poised to revolutionize the development of personalized medicine. By analyzing the unique protein structures associated with an individual’s genetic makeup, researchers can tailor treatments to target specific molecular pathways involved in disease. This personalized approach promises to improve treatment outcomes and reduce adverse effects, marking a significant shift from the traditional one-size-fits-all model of medicine.
Furthermore, the open-access nature of AlphaFold 3’s predictions democratizes scientific research, allowing researchers worldwide to access and utilize this powerful tool. This collaborative approach fosters innovation and accelerates the pace of discovery, as scientists can build upon each other’s work and share insights across disciplines. The implications of this are far-reaching, potentially leading to breakthroughs in areas such as cancer research, neurodegenerative diseases, and infectious diseases.
In conclusion, AlphaFold 3 is not just a technological marvel; it is a catalyst for change in the field of drug discovery and development. By providing unprecedented insights into the structure and interactions of life’s molecules, it empowers researchers to tackle some of the most pressing challenges in medicine today. As we continue to explore the potential of this transformative tool, the future of drug discovery looks increasingly promising, with the potential to improve the lives of millions around the world.
Understanding Molecular Interactions Through AlphaFold 3
AlphaFold 3 represents a significant leap forward in the field of computational biology, offering unprecedented insights into the structure and interactions of life’s molecules. Building upon the groundbreaking success of its predecessors, AlphaFold 3 continues to refine our understanding of protein folding, a complex process that is fundamental to virtually all biological functions. Proteins, composed of long chains of amino acids, must fold into specific three-dimensional shapes to perform their roles effectively. Misfolded proteins can lead to diseases, making the accurate prediction of protein structures a critical area of research.
The development of AlphaFold 3 has been driven by the need to address the limitations of previous models, which, while revolutionary, still faced challenges in predicting the interactions between proteins and other molecules. By leveraging advanced machine learning techniques and vast datasets, AlphaFold 3 has achieved remarkable accuracy in predicting not only the static structures of proteins but also their dynamic interactions with other biomolecules. This capability is crucial for understanding how proteins function within the complex networks of living organisms.
One of the most significant advancements in AlphaFold 3 is its ability to model protein-protein interactions with high precision. Proteins rarely act in isolation; they often form complexes with other proteins to carry out their functions. Understanding these interactions is essential for elucidating cellular processes and developing therapeutic interventions. AlphaFold 3’s enhanced algorithms allow researchers to predict how proteins interact with each other, providing insights into the mechanisms of diseases and identifying potential targets for drug development.
Moreover, AlphaFold 3 extends its predictive power to protein-ligand interactions, which are central to drug discovery. Ligands, which can be small molecules or ions, bind to proteins and modulate their activity. Accurate prediction of these interactions can accelerate the identification of new drugs and improve the design of existing ones. By simulating how ligands bind to their target proteins, AlphaFold 3 offers a powerful tool for pharmaceutical research, potentially reducing the time and cost associated with drug development.
In addition to its applications in medicine, AlphaFold 3 holds promise for advancing our understanding of fundamental biological processes. For instance, it can shed light on the molecular basis of enzyme catalysis, signal transduction, and gene regulation. By providing detailed models of protein structures and their interactions, AlphaFold 3 enables researchers to explore the intricate molecular machinery that underpins life itself.
Furthermore, the insights gained from AlphaFold 3 have implications beyond biology and medicine. In the field of biotechnology, for example, the ability to predict protein interactions can inform the design of novel enzymes for industrial applications, such as biofuel production and waste management. Similarly, in agriculture, understanding protein interactions can lead to the development of crops with improved resistance to pests and environmental stresses.
In conclusion, AlphaFold 3 represents a transformative tool in the quest to understand the molecular interactions that govern life. Its ability to accurately predict protein structures and interactions opens new avenues for research and innovation across multiple disciplines. As scientists continue to explore the capabilities of AlphaFold 3, it is poised to drive significant advancements in our understanding of biology, with far-reaching implications for health, industry, and the environment. Through its sophisticated algorithms and comprehensive datasets, AlphaFold 3 is not only unveiling the structures of life’s molecules but also illuminating the complex web of interactions that sustain life on Earth.
The Impact Of AlphaFold 3 On Genomic Research
The advent of AlphaFold 3 marks a significant milestone in the field of genomic research, offering unprecedented insights into the structure and interactions of life’s molecules. Building upon the groundbreaking achievements of its predecessors, AlphaFold 3 has further refined the ability to predict protein structures with remarkable accuracy. This advancement is not merely a technical achievement; it represents a transformative tool that is reshaping our understanding of biology at a molecular level. As researchers delve deeper into the complexities of genomics, the implications of AlphaFold 3 are becoming increasingly evident, heralding a new era of discovery and innovation.
To appreciate the impact of AlphaFold 3, it is essential to consider the challenges that have historically plagued protein structure prediction. Proteins, composed of long chains of amino acids, fold into intricate three-dimensional shapes that determine their function. Understanding these structures is crucial for elucidating the roles proteins play in various biological processes. However, traditional methods of determining protein structures, such as X-ray crystallography and nuclear magnetic resonance spectroscopy, are time-consuming and resource-intensive. AlphaFold 3 addresses these limitations by leveraging advanced machine learning algorithms to predict protein structures with a level of precision that rivals experimental methods.
The implications of this capability are profound. With AlphaFold 3, researchers can now predict the structures of proteins that were previously inaccessible, opening new avenues for exploration in genomic research. This ability to model proteins at scale accelerates the identification of potential drug targets, thereby expediting the drug discovery process. Moreover, AlphaFold 3’s predictions facilitate a deeper understanding of genetic diseases by revealing how mutations can alter protein structures and disrupt their functions. Consequently, this knowledge paves the way for the development of targeted therapies that can correct these molecular aberrations.
Furthermore, AlphaFold 3’s impact extends beyond individual proteins to encompass the complex networks of interactions that underpin cellular function. Proteins rarely act in isolation; they interact with other molecules to form intricate pathways that regulate biological processes. By accurately predicting protein structures, AlphaFold 3 enables researchers to map these interactions with greater clarity, providing insights into the molecular mechanisms that drive health and disease. This comprehensive understanding of protein networks is instrumental in advancing personalized medicine, where treatments can be tailored to an individual’s unique genetic makeup.
In addition to its direct applications in research and medicine, AlphaFold 3 is fostering collaboration across scientific disciplines. Its open-access model encourages the sharing of data and resources, promoting a culture of transparency and cooperation. This collaborative spirit is essential for tackling the complex challenges that lie ahead in genomic research, as it enables scientists from diverse fields to pool their expertise and accelerate the pace of discovery.
In conclusion, AlphaFold 3 is revolutionizing genomic research by providing a powerful tool for understanding the structure and interactions of proteins. Its ability to predict protein structures with high accuracy is transforming the landscape of drug discovery, genetic disease research, and personalized medicine. As researchers continue to harness the potential of AlphaFold 3, the insights gained will undoubtedly lead to breakthroughs that enhance our understanding of biology and improve human health. The journey of discovery is far from over, but with AlphaFold 3, we are equipped with a formidable ally in the quest to unravel the mysteries of life at the molecular level.
AlphaFold 3 And Its Role In Personalized Medicine
AlphaFold 3, the latest iteration of the groundbreaking protein structure prediction tool developed by DeepMind, is poised to revolutionize the field of personalized medicine. Building on the success of its predecessors, AlphaFold 3 offers unprecedented accuracy in predicting the three-dimensional structures of proteins, which are crucial for understanding biological processes and developing targeted therapies. As we delve into the implications of this technological advancement, it becomes evident that AlphaFold 3 holds the potential to transform how we approach the diagnosis and treatment of diseases on an individual level.
To appreciate the significance of AlphaFold 3 in personalized medicine, it is essential to understand the role of proteins in the human body. Proteins are the workhorses of cells, responsible for a myriad of functions ranging from catalyzing biochemical reactions to providing structural support. The function of a protein is intricately linked to its three-dimensional shape, which is determined by the sequence of amino acids encoded in the corresponding gene. Misfolded proteins or aberrations in protein structure can lead to a host of diseases, including cancer, neurodegenerative disorders, and rare genetic conditions. Therefore, accurately predicting protein structures is a critical step in understanding disease mechanisms and developing effective treatments.
AlphaFold 3’s ability to predict protein structures with remarkable precision opens new avenues for personalized medicine. By integrating this tool into clinical practice, healthcare providers can gain insights into the molecular underpinnings of a patient’s condition, allowing for more accurate diagnoses and tailored treatment plans. For instance, in the realm of oncology, AlphaFold 3 can be used to identify specific mutations in cancer-related proteins, enabling the development of targeted therapies that directly address the molecular abnormalities driving tumor growth. This approach not only enhances treatment efficacy but also minimizes adverse effects, as therapies can be designed to selectively target cancer cells while sparing healthy tissue.
Moreover, AlphaFold 3’s contributions extend beyond cancer treatment. In the field of rare genetic disorders, where traditional drug development approaches often fall short due to the small patient populations, AlphaFold 3 offers a promising solution. By elucidating the structures of proteins affected by rare mutations, researchers can identify potential drug targets and design molecules that correct or compensate for the structural defects. This capability accelerates the drug discovery process and brings hope to patients who previously had limited treatment options.
Furthermore, AlphaFold 3’s integration into personalized medicine is facilitated by its compatibility with other cutting-edge technologies. For example, when combined with genomic sequencing data, AlphaFold 3 can provide a comprehensive view of an individual’s molecular landscape, enabling a holistic approach to disease management. Additionally, the tool’s ability to predict protein-protein interactions enhances our understanding of complex biological networks, paving the way for the development of combination therapies that target multiple pathways simultaneously.
In conclusion, AlphaFold 3 represents a significant leap forward in the quest for personalized medicine. Its unparalleled accuracy in predicting protein structures and interactions holds the promise of transforming how we diagnose and treat diseases, offering tailored solutions that address the unique molecular profiles of individual patients. As this technology continues to evolve, it is poised to become an indispensable tool in the arsenal of healthcare providers, ultimately improving patient outcomes and advancing the frontiers of medical science.
Challenges And Future Directions For AlphaFold 3
AlphaFold 3 represents a significant leap forward in the field of computational biology, building upon the groundbreaking achievements of its predecessors. While AlphaFold 2 revolutionized our understanding of protein structures by predicting their 3D configurations with remarkable accuracy, AlphaFold 3 aims to delve deeper into the intricate web of molecular interactions that govern biological processes. However, as with any pioneering technology, AlphaFold 3 faces a myriad of challenges that must be addressed to fully realize its potential and pave the way for future advancements.
One of the primary challenges confronting AlphaFold 3 is the sheer complexity of biological systems. Proteins rarely function in isolation; they interact with a multitude of other molecules, including nucleic acids, lipids, and small metabolites. These interactions are dynamic and context-dependent, often influenced by the cellular environment and external stimuli. Accurately modeling these interactions requires not only a comprehensive understanding of individual protein structures but also an ability to predict how these structures change in response to various factors. This necessitates the integration of vast amounts of data from diverse sources, including experimental studies and high-throughput sequencing technologies.
Moreover, the computational demands of simulating such complex systems are immense. While AlphaFold 2 demonstrated the power of deep learning in predicting static protein structures, AlphaFold 3 must extend this capability to dynamic simulations. This involves not only predicting the most stable conformations of proteins but also capturing the transient states that are crucial for understanding their function. Achieving this level of detail requires significant advancements in computational power and algorithmic efficiency, as well as the development of novel machine learning techniques capable of handling the intricacies of molecular dynamics.
In addition to these technical challenges, there are also significant data-related hurdles to overcome. The accuracy of AlphaFold 3’s predictions is heavily reliant on the quality and quantity of available data. While the Protein Data Bank provides a wealth of structural information, it is far from comprehensive. Many proteins, particularly those that are membrane-bound or intrinsically disordered, remain underrepresented in structural databases. To address this, there is a pressing need for more experimental data, particularly from techniques such as cryo-electron microscopy and nuclear magnetic resonance spectroscopy, which can provide insights into the structures of challenging proteins.
Furthermore, the ethical and societal implications of AlphaFold 3’s capabilities cannot be overlooked. As the technology advances, it holds the potential to revolutionize drug discovery and personalized medicine by enabling the rapid identification of novel therapeutic targets and the design of tailored interventions. However, this also raises concerns about data privacy, intellectual property, and the equitable distribution of benefits. Ensuring that the advancements made possible by AlphaFold 3 are accessible to all and used responsibly will require careful consideration and collaboration among scientists, policymakers, and industry stakeholders.
Looking ahead, the future directions for AlphaFold 3 are both exciting and daunting. Continued progress will likely involve a multidisciplinary approach, combining insights from biology, chemistry, physics, and computer science. Collaborative efforts between academia, industry, and government will be essential to overcome the challenges and harness the full potential of this transformative technology. As AlphaFold 3 continues to evolve, it promises to deepen our understanding of the molecular machinery of life, offering unprecedented opportunities to address some of the most pressing challenges in health and disease.
Q&A
1. **What is AlphaFold 3?**
AlphaFold 3 is the anticipated next iteration of DeepMind’s AlphaFold, a groundbreaking AI system designed to predict protein structures with high accuracy, potentially offering enhanced capabilities in understanding molecular interactions and dynamics.
2. **How does AlphaFold 3 improve upon its predecessors?**
AlphaFold 3 is expected to offer improvements in prediction accuracy, speed, and the ability to model more complex protein interactions and dynamics, possibly incorporating more advanced machine learning techniques and larger datasets.
3. **What are the potential applications of AlphaFold 3?**
Potential applications include drug discovery, understanding disease mechanisms, designing novel proteins for industrial and therapeutic purposes, and advancing research in structural biology.
4. **How does AlphaFold 3 impact the field of structural biology?**
AlphaFold 3 could significantly accelerate research by providing more accurate and comprehensive protein structure predictions, reducing the need for time-consuming experimental methods like X-ray crystallography and cryo-electron microscopy.
5. **What challenges does AlphaFold 3 address in protein structure prediction?**
AlphaFold 3 aims to address challenges such as predicting the structures of protein complexes, modeling protein flexibility and dynamics, and improving predictions for proteins with limited experimental data.
6. **What are the implications of AlphaFold 3 for drug discovery?**
AlphaFold 3 could streamline drug discovery by providing detailed insights into protein targets, facilitating the design of more effective and specific drugs, and potentially reducing the time and cost associated with bringing new therapeutics to market.AlphaFold 3 represents a significant advancement in computational biology, building upon its predecessors to further enhance the accuracy and efficiency of protein structure prediction. By integrating more sophisticated algorithms and leveraging vast datasets, AlphaFold 3 not only predicts the three-dimensional structures of proteins with unprecedented precision but also provides insights into their interactions and functions within biological systems. This breakthrough has the potential to revolutionize fields such as drug discovery, molecular biology, and bioengineering by enabling researchers to understand complex biological processes at a molecular level. As a result, AlphaFold 3 stands as a pivotal tool in the quest to unravel the mysteries of life’s molecular machinery, paving the way for innovative solutions to some of the most pressing challenges in medicine and biotechnology.
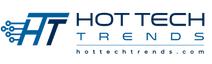