Data enrichment is a critical process in the realm of data management and analytics, aimed at enhancing the quality and value of raw data by supplementing it with additional information. This process transforms basic data into a more comprehensive and insightful asset, enabling organizations to make more informed decisions, improve customer experiences, and drive business growth. Effective strategies for data enrichment involve a combination of techniques and tools designed to integrate external data sources, cleanse and validate data, and apply advanced analytics to extract meaningful insights. By leveraging these strategies, businesses can ensure their data is accurate, relevant, and actionable, ultimately leading to improved operational efficiency and competitive advantage.
Understanding Data Enrichment: Key Concepts and Benefits
Data enrichment is a critical process in the realm of data management, aimed at enhancing the quality and utility of raw data. By integrating additional information from external sources, organizations can transform basic data into a more comprehensive and insightful asset. This process not only improves the accuracy of data but also augments its relevance, thereby enabling more informed decision-making. To fully appreciate the benefits of data enrichment, it is essential to understand its key concepts and the strategies that can be employed to maximize its effectiveness.
At its core, data enrichment involves supplementing existing data with new, relevant information. This can be achieved through various methods, such as appending missing data, correcting inaccuracies, or adding contextual details. For instance, a company might enrich its customer database by appending demographic information, purchasing history, or social media activity. This enriched data can then be used to create more targeted marketing campaigns, improve customer segmentation, and enhance overall customer experience.
One of the primary benefits of data enrichment is the ability to gain deeper insights into customer behavior and preferences. By having a more complete view of the customer, businesses can tailor their products and services to better meet customer needs. This not only increases customer satisfaction but also drives customer loyalty and retention. Moreover, enriched data can help identify new market opportunities and trends, allowing companies to stay ahead of the competition.
To effectively implement data enrichment, organizations must adopt a strategic approach. First and foremost, it is crucial to identify the specific data needs and objectives. This involves understanding what information is missing or lacking in the current dataset and determining how additional data can address these gaps. Once the objectives are clear, the next step is to select appropriate data sources. These sources can be internal, such as CRM systems and transaction records, or external, such as third-party data providers and public databases.
Another important strategy is to ensure data quality and consistency. Enriched data is only valuable if it is accurate and reliable. Therefore, organizations must establish robust data validation and cleansing processes to eliminate errors and inconsistencies. This may involve using automated tools to detect and correct anomalies or implementing manual checks for more complex data issues. Additionally, maintaining data privacy and compliance is paramount, especially when dealing with sensitive information. Organizations must adhere to relevant regulations and industry standards to protect customer data and maintain trust.
Furthermore, leveraging advanced technologies can significantly enhance the data enrichment process. Machine learning algorithms, for example, can be used to identify patterns and correlations in large datasets, enabling more precise data enrichment. Similarly, natural language processing can be employed to extract valuable insights from unstructured data sources, such as social media posts and customer reviews. By integrating these technologies into their data enrichment strategies, organizations can achieve greater efficiency and accuracy.
In conclusion, data enrichment is a powerful tool that can transform raw data into a valuable asset for any organization. By understanding the key concepts and benefits of data enrichment, and by implementing effective strategies, businesses can unlock new opportunities and drive growth. As the data landscape continues to evolve, the importance of data enrichment will only increase, making it an essential component of any successful data management strategy.
Leveraging Third-Party Data Sources for Enhanced Insights
In the contemporary landscape of data-driven decision-making, organizations are increasingly recognizing the value of data enrichment as a means to gain deeper insights and enhance their strategic initiatives. One of the most effective strategies for data enrichment involves leveraging third-party data sources. By integrating external data with internal datasets, businesses can create a more comprehensive view of their operations, customers, and market trends. This approach not only augments the quality of the data but also provides a competitive edge in understanding complex business environments.
To begin with, third-party data sources offer a wealth of information that can fill gaps in an organization’s existing data. These sources can include demographic data, social media activity, purchasing behavior, and more. By incorporating such data, companies can develop a more nuanced understanding of their customer base. For instance, demographic data can help businesses tailor their marketing strategies to specific age groups or income levels, thereby increasing the effectiveness of their campaigns. Moreover, social media data can provide real-time insights into consumer sentiment, enabling companies to respond swiftly to changing preferences and trends.
Furthermore, the integration of third-party data can significantly enhance predictive analytics. By enriching internal datasets with external information, organizations can improve the accuracy of their predictive models. This is particularly beneficial in sectors such as finance and retail, where anticipating market trends and consumer behavior is crucial. For example, a retailer might use weather data from a third-party source to predict sales patterns for seasonal products. By doing so, they can optimize inventory levels and reduce waste, ultimately leading to cost savings and increased profitability.
In addition to improving predictive capabilities, third-party data enrichment can also aid in risk management. By accessing external data related to economic indicators, geopolitical events, or industry-specific risks, companies can better assess potential threats and develop more robust contingency plans. This proactive approach to risk management not only safeguards the organization but also instills confidence among stakeholders.
However, while the benefits of leveraging third-party data sources are substantial, it is essential to address the challenges associated with this strategy. One of the primary concerns is data quality. Organizations must ensure that the external data they incorporate is accurate, reliable, and relevant. This requires a thorough vetting process and, often, the establishment of partnerships with reputable data providers. Additionally, data privacy and compliance are critical considerations. Companies must navigate complex regulations, such as the General Data Protection Regulation (GDPR), to ensure that their data enrichment practices do not infringe on individual privacy rights.
Moreover, integrating third-party data with existing systems can be technically challenging. It often requires sophisticated data management tools and expertise in data integration techniques. Organizations must invest in the necessary infrastructure and talent to effectively manage and utilize enriched datasets. Despite these challenges, the strategic use of third-party data sources remains a powerful tool for organizations seeking to enhance their insights and drive innovation.
In conclusion, leveraging third-party data sources for data enrichment offers numerous advantages, from improved customer understanding to enhanced predictive analytics and risk management. While challenges such as data quality and compliance must be addressed, the potential benefits make it a worthwhile endeavor. As organizations continue to navigate an increasingly data-centric world, the strategic integration of external data will undoubtedly play a pivotal role in shaping their success.
Implementing Machine Learning for Automated Data Enrichment
Implementing machine learning for automated data enrichment is a transformative approach that organizations are increasingly adopting to enhance the quality and value of their data. As businesses accumulate vast amounts of data, the challenge lies not only in managing this information but also in extracting meaningful insights from it. Data enrichment, the process of enhancing existing data by supplementing it with additional information, plays a crucial role in this context. Machine learning, with its ability to analyze and learn from data patterns, offers a powerful solution for automating this process, thereby improving efficiency and accuracy.
To begin with, one of the most effective strategies for implementing machine learning in data enrichment is to clearly define the objectives. Organizations must identify the specific data attributes that require enrichment and determine the additional information needed to achieve their goals. This clarity ensures that the machine learning models are trained with a focused purpose, leading to more relevant and actionable outcomes. For instance, a company aiming to enhance customer profiles might seek to enrich its data with demographic information, purchasing behavior, or social media activity.
Once the objectives are established, the next step involves selecting the appropriate machine learning algorithms. The choice of algorithm depends on the nature of the data and the enrichment goals. Supervised learning algorithms, such as decision trees or support vector machines, are often used when labeled data is available, allowing the model to learn from examples. On the other hand, unsupervised learning techniques, like clustering or association rule learning, can be employed when the data lacks labels, enabling the discovery of hidden patterns and relationships.
Data preprocessing is another critical aspect of implementing machine learning for data enrichment. This step involves cleaning and transforming the raw data to ensure it is suitable for analysis. Handling missing values, removing duplicates, and normalizing data are essential tasks that contribute to the accuracy and reliability of the machine learning models. Additionally, feature engineering, which involves creating new variables or modifying existing ones, can significantly enhance the model’s performance by providing it with more informative inputs.
Furthermore, the integration of external data sources is a key strategy in data enrichment. By incorporating data from third-party providers, public databases, or social media platforms, organizations can gain a more comprehensive view of their data subjects. Machine learning models can be trained to automatically merge and reconcile these diverse data sources, ensuring consistency and reducing the risk of errors. This integration not only enriches the data but also opens up new avenues for analysis and insight generation.
As machine learning models are deployed for data enrichment, continuous monitoring and evaluation become imperative. Organizations must regularly assess the performance of their models to ensure they are delivering the desired outcomes. This involves tracking key metrics, such as accuracy, precision, and recall, and making necessary adjustments to the models as needed. Feedback loops, where the enriched data is used to retrain and improve the models, can further enhance their effectiveness over time.
In conclusion, implementing machine learning for automated data enrichment requires a strategic approach that encompasses clear objectives, appropriate algorithm selection, thorough data preprocessing, and the integration of external data sources. By leveraging these strategies, organizations can transform their raw data into a valuable asset, driving informed decision-making and gaining a competitive edge in today’s data-driven landscape. As technology continues to evolve, the potential for machine learning in data enrichment will only grow, offering even more sophisticated and efficient solutions for businesses across various industries.
Best Practices for Data Quality and Accuracy in Enrichment
Data enrichment is a critical process in enhancing the quality and accuracy of data, which is essential for informed decision-making and strategic planning. As organizations increasingly rely on data-driven insights, the need for robust data enrichment strategies becomes paramount. To ensure data quality and accuracy, several best practices can be employed, each contributing to a more comprehensive and reliable dataset.
Firstly, it is important to establish a clear understanding of the objectives behind data enrichment. By defining the specific goals, organizations can tailor their enrichment processes to meet these objectives effectively. This involves identifying the types of data that need enrichment and the sources from which additional information will be gathered. A well-defined objective not only streamlines the enrichment process but also ensures that the data collected is relevant and useful.
Once the objectives are set, the next step is to ensure the integrity of the data sources. Reliable and credible data sources are fundamental to maintaining data quality. Organizations should conduct thorough evaluations of potential data providers, assessing their reputation, accuracy, and timeliness. By partnering with reputable sources, organizations can minimize the risk of incorporating erroneous or outdated information into their datasets.
In addition to selecting credible sources, it is crucial to implement data validation techniques. Data validation involves verifying the accuracy and consistency of data before it is integrated into the existing dataset. This can be achieved through automated validation tools that check for anomalies, duplicates, and inconsistencies. By employing these tools, organizations can significantly reduce errors and enhance the overall quality of their data.
Moreover, data enrichment should be an ongoing process rather than a one-time effort. As data is constantly evolving, regular updates and reviews are necessary to maintain its accuracy and relevance. Implementing a continuous enrichment strategy allows organizations to adapt to changes in the data landscape and ensure that their datasets remain current. This ongoing process can be facilitated by setting up automated systems that periodically refresh and validate the data.
Another best practice is to prioritize data privacy and security during the enrichment process. With the increasing amount of data being collected and shared, safeguarding sensitive information is more important than ever. Organizations must comply with data protection regulations and implement robust security measures to prevent unauthorized access and breaches. By prioritizing privacy and security, organizations can build trust with their stakeholders and protect their valuable data assets.
Furthermore, collaboration across departments can enhance the effectiveness of data enrichment efforts. By fostering a culture of collaboration, organizations can leverage the expertise and insights of different teams to enrich data more comprehensively. Cross-departmental collaboration ensures that diverse perspectives are considered, leading to a more holistic understanding of the data and its potential applications.
Finally, it is essential to measure the impact of data enrichment on business outcomes. By establishing key performance indicators (KPIs) and regularly assessing the results, organizations can determine the effectiveness of their enrichment strategies. This evaluation process provides valuable feedback, allowing organizations to refine their approaches and continuously improve data quality and accuracy.
In conclusion, effective data enrichment requires a strategic approach that encompasses clear objectives, reliable sources, validation techniques, ongoing updates, privacy considerations, cross-departmental collaboration, and impact assessment. By adhering to these best practices, organizations can enhance the quality and accuracy of their data, ultimately driving better decision-making and achieving their business goals.
Integrating Data Enrichment with CRM Systems for Better Customer Engagement
Integrating data enrichment with Customer Relationship Management (CRM) systems is a pivotal strategy for enhancing customer engagement. As businesses strive to understand their customers better, data enrichment emerges as a crucial tool, providing deeper insights and more personalized interactions. By augmenting existing customer data with additional information, companies can create a more comprehensive view of their clientele, thereby facilitating more effective engagement strategies.
To begin with, data enrichment involves the process of enhancing existing data by adding new information from external sources. This can include demographic details, social media profiles, purchase history, and more. When integrated with CRM systems, enriched data can significantly improve the quality of customer insights. For instance, a CRM system enriched with social media data can help businesses understand customer preferences and behaviors more accurately. This, in turn, allows for the creation of targeted marketing campaigns that resonate more effectively with the intended audience.
Moreover, the integration of data enrichment with CRM systems can lead to improved customer segmentation. By having access to a broader range of data points, businesses can segment their customers more precisely. This enables the development of tailored marketing strategies that address the specific needs and preferences of different customer groups. Consequently, businesses can engage with their customers in a more meaningful way, fostering stronger relationships and enhancing customer loyalty.
In addition to improved segmentation, data enrichment can also enhance predictive analytics within CRM systems. By incorporating enriched data, businesses can better anticipate customer needs and behaviors. This predictive capability allows companies to proactively address customer concerns and offer solutions before issues arise. As a result, customer satisfaction is likely to increase, as customers feel understood and valued by the business.
Furthermore, data enrichment can streamline the personalization of customer interactions. With a more comprehensive understanding of each customer, businesses can tailor their communications and offers to align with individual preferences. This level of personalization is increasingly expected by customers, who are more likely to engage with brands that demonstrate an understanding of their unique needs. By leveraging enriched data, businesses can deliver personalized experiences that enhance customer engagement and drive brand loyalty.
However, integrating data enrichment with CRM systems is not without its challenges. One of the primary concerns is data privacy. As businesses collect and integrate more data, they must ensure compliance with data protection regulations. This requires implementing robust data governance frameworks to safeguard customer information and maintain trust. Additionally, businesses must ensure that the data they integrate is accurate and relevant. Poor-quality data can lead to misguided strategies and ineffective customer engagement efforts.
To overcome these challenges, businesses should adopt a strategic approach to data enrichment. This involves selecting reliable data sources, implementing data validation processes, and continuously monitoring data quality. By doing so, businesses can ensure that their CRM systems are equipped with high-quality, relevant data that enhances customer engagement efforts.
In conclusion, integrating data enrichment with CRM systems offers significant benefits for businesses seeking to improve customer engagement. By providing deeper insights, enabling precise segmentation, enhancing predictive analytics, and facilitating personalized interactions, enriched data empowers businesses to connect with their customers more effectively. However, to fully realize these benefits, businesses must address challenges related to data privacy and quality. By adopting a strategic approach to data enrichment, companies can harness the power of enriched data to foster stronger customer relationships and drive business success.
Measuring the ROI of Data Enrichment Initiatives
Measuring the return on investment (ROI) of data enrichment initiatives is a critical component for organizations seeking to enhance their data-driven decision-making processes. As businesses increasingly rely on data to inform strategies and operations, the quality and comprehensiveness of this data become paramount. Data enrichment, which involves enhancing existing data by adding additional information from external sources, can significantly improve the value of data assets. However, to justify the investment in such initiatives, it is essential to accurately measure their ROI.
To begin with, understanding the objectives of data enrichment is crucial. Organizations typically pursue data enrichment to achieve more accurate customer profiling, improved segmentation, and enhanced personalization. These objectives, in turn, lead to better-targeted marketing campaigns, increased customer satisfaction, and ultimately, higher revenue. Therefore, the first step in measuring ROI is to clearly define the goals of the data enrichment initiative. By establishing specific, measurable objectives, organizations can create a baseline against which the success of the initiative can be evaluated.
Once the objectives are defined, the next step is to identify the key performance indicators (KPIs) that will be used to measure success. These KPIs should align with the goals of the data enrichment initiative and may include metrics such as increased conversion rates, improved customer retention, or higher average transaction values. By tracking these KPIs over time, organizations can assess the impact of data enrichment on their business outcomes. Additionally, it is important to consider both quantitative and qualitative measures. While quantitative metrics provide concrete evidence of success, qualitative feedback from customers and stakeholders can offer valuable insights into the perceived value of enriched data.
Furthermore, it is essential to account for the costs associated with data enrichment initiatives. These costs may include expenses related to acquiring external data sources, investing in technology and tools for data integration, and allocating resources for data management and analysis. By comparing these costs against the benefits realized through improved business performance, organizations can calculate the ROI of their data enrichment efforts. It is important to note that ROI should be evaluated over a reasonable time frame, as the benefits of data enrichment may not be immediately apparent.
In addition to direct financial benefits, data enrichment can also yield intangible advantages that contribute to long-term success. For instance, enriched data can enhance an organization’s ability to innovate by providing deeper insights into market trends and customer preferences. This, in turn, can lead to the development of new products and services that better meet customer needs. Moreover, data enrichment can improve operational efficiency by streamlining processes and reducing the time and effort required to gather and analyze information.
To ensure the ongoing success of data enrichment initiatives, organizations should adopt a continuous improvement approach. This involves regularly reviewing and refining data enrichment strategies based on the insights gained from measuring ROI. By doing so, organizations can adapt to changing market conditions and evolving customer expectations, thereby maximizing the value of their data assets.
In conclusion, measuring the ROI of data enrichment initiatives is a multifaceted process that requires careful planning and execution. By clearly defining objectives, identifying relevant KPIs, and considering both costs and benefits, organizations can effectively assess the impact of data enrichment on their business performance. Ultimately, a well-executed data enrichment strategy can provide significant competitive advantages, driving growth and innovation in an increasingly data-driven world.
Q&A
1. **What is data enrichment?**
Data enrichment is the process of enhancing existing data by adding additional information from external sources, improving its quality, accuracy, and value.
2. **Why is data enrichment important?**
Data enrichment is crucial because it provides a more comprehensive view of customers, improves decision-making, enhances personalization, and increases the effectiveness of marketing and sales strategies.
3. **What are some common data enrichment techniques?**
Common techniques include demographic enrichment, firmographic enrichment, behavioral data integration, and geographic data enhancement.
4. **How can machine learning be used in data enrichment?**
Machine learning can automate the process of identifying patterns and correlations in data, predict missing values, and classify data into meaningful categories, thus enhancing the enrichment process.
5. **What are the challenges of data enrichment?**
Challenges include data privacy concerns, ensuring data accuracy and consistency, integrating data from multiple sources, and managing the cost and complexity of enrichment processes.
6. **What tools are commonly used for data enrichment?**
Tools like Clearbit, ZoomInfo, InsideView, and Salesforce Data.com are commonly used for data enrichment, providing access to vast databases and integration capabilities for enhancing data quality.Effective data enrichment strategies are crucial for enhancing the value and utility of data within an organization. These strategies involve augmenting existing data with additional information to provide deeper insights and improve decision-making processes. Key strategies include leveraging third-party data sources to fill gaps and enhance data quality, employing machine learning algorithms to identify patterns and predict trends, and utilizing data integration tools to ensure seamless data flow across platforms. Additionally, maintaining data accuracy and privacy through regular audits and compliance with data protection regulations is essential. By implementing these strategies, organizations can achieve a more comprehensive understanding of their data, leading to improved customer experiences, optimized operations, and a competitive advantage in the market.
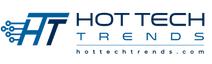