FermiNet is a groundbreaking approach in the realm of quantum physics and chemistry, leveraging the power of neural networks to solve complex many-electron systems from first principles. Developed by researchers at DeepMind, FermiNet represents a significant advancement in computational methods, offering a novel way to approximate the wave functions of quantum systems. By utilizing deep learning techniques, FermiNet can efficiently handle the intricate interactions between electrons, providing highly accurate solutions to the Schrödinger equation. This innovative method holds the potential to transform our understanding of quantum mechanics and its applications in chemistry, enabling more precise simulations of molecular structures and reactions, and paving the way for new discoveries in material science and drug design.
Introduction To FermiNet: Revolutionizing Quantum Simulations
FermiNet represents a groundbreaking advancement in the field of quantum simulations, offering a novel approach to understanding the complex interactions within quantum systems. Developed by researchers at DeepMind, FermiNet is a neural network architecture specifically designed to tackle the challenges of quantum physics and chemistry from first principles. This innovative tool is poised to revolutionize the way scientists simulate and predict the behavior of quantum systems, providing unprecedented accuracy and efficiency.
At the heart of FermiNet’s design is its ability to model the wave functions of quantum systems. Wave functions are fundamental to quantum mechanics, encapsulating all the information about a system’s state. However, accurately representing these functions, especially for systems with many interacting particles, has long been a formidable challenge. Traditional methods, such as the Hartree-Fock approximation or density functional theory, often fall short in capturing the intricate correlations between particles. FermiNet addresses these limitations by leveraging the power of deep learning to approximate wave functions with remarkable precision.
The architecture of FermiNet is uniquely suited to the task of quantum simulation. It employs a neural network that respects the antisymmetry property of fermionic wave functions, a crucial aspect when dealing with electrons. This antisymmetry ensures that the wave function changes sign when two electrons are exchanged, a requirement dictated by the Pauli exclusion principle. By incorporating this property directly into its design, FermiNet can more accurately model the behavior of electrons in atoms and molecules, leading to more reliable predictions of their properties.
Moreover, FermiNet’s approach is rooted in the variational principle, a cornerstone of quantum mechanics. This principle states that the best approximation to the ground state of a quantum system is the one that minimizes the energy expectation value. FermiNet optimizes its parameters to achieve this minimum, effectively learning the most accurate wave function representation for a given system. This method not only enhances the accuracy of simulations but also provides insights into the underlying physics governing the system.
Transitioning from traditional methods to FermiNet offers several advantages. One significant benefit is its scalability. While conventional techniques often struggle with the exponential growth in computational complexity as the number of particles increases, FermiNet’s neural network architecture can handle larger systems more efficiently. This scalability opens new avenues for exploring complex quantum phenomena that were previously out of reach.
Furthermore, FermiNet’s ability to simulate quantum systems from first principles has profound implications for the field of chemistry. By accurately predicting molecular properties and interactions, FermiNet can aid in the design of new materials and drugs, potentially accelerating the discovery process in these areas. Its applications extend beyond theoretical research, offering practical solutions to real-world challenges.
In conclusion, FermiNet represents a significant leap forward in the realm of quantum simulations. By harnessing the power of deep learning and respecting the fundamental principles of quantum mechanics, it provides a robust framework for exploring the intricacies of quantum systems. As researchers continue to refine and expand upon this technology, FermiNet is set to play a pivotal role in advancing our understanding of the quantum world, paving the way for new discoveries and innovations in physics and chemistry.
FermiNet’s Role In Quantum Chemistry: A Deep Dive
FermiNet, a groundbreaking neural network architecture, has emerged as a pivotal tool in the exploration of quantum physics and chemistry from first principles. This innovative approach, developed by researchers at DeepMind, leverages the power of deep learning to address the complex challenges inherent in quantum systems. By focusing on the electronic structure of atoms and molecules, FermiNet offers a novel method for solving the Schrödinger equation, which is fundamental to understanding the behavior of quantum systems.
Traditionally, quantum chemistry has relied on approximations and computational methods such as Hartree-Fock and Density Functional Theory (DFT) to predict molecular properties and interactions. While these methods have been successful to a certain extent, they often fall short in accurately describing systems with strong electron correlation or those involving transition metals. This is where FermiNet distinguishes itself by providing a more precise and flexible framework for modeling quantum systems.
FermiNet’s architecture is designed to represent the wave function of a quantum system, which is a complex mathematical function that encodes all the information about the system’s state. Unlike conventional methods that use fixed basis sets, FermiNet employs a neural network to learn the wave function directly from the principles of quantum mechanics. This approach allows for a more accurate representation of electron correlation, a critical factor in determining the properties of molecules.
One of the key advantages of FermiNet is its ability to scale with system size. As the number of electrons in a system increases, the complexity of solving the Schrödinger equation grows exponentially. FermiNet addresses this challenge by using a neural network that can adapt to different system sizes without a significant loss in accuracy. This scalability is crucial for studying larger and more complex molecules, which are often beyond the reach of traditional quantum chemistry methods.
Moreover, FermiNet’s flexibility extends to its ability to incorporate different types of physical constraints and symmetries. By embedding these constraints into the neural network architecture, FermiNet ensures that the solutions it generates are physically meaningful and adhere to the fundamental laws of quantum mechanics. This capability not only enhances the accuracy of the results but also provides insights into the underlying physics of the systems being studied.
In addition to its applications in quantum chemistry, FermiNet holds promise for advancing our understanding of condensed matter physics and materials science. By providing a more accurate description of electronic interactions, FermiNet can aid in the design of new materials with tailored properties, such as superconductors and catalysts. Furthermore, its ability to model complex quantum systems could lead to breakthroughs in fields such as quantum computing and nanotechnology.
In conclusion, FermiNet represents a significant advancement in the field of quantum chemistry, offering a powerful tool for exploring the intricacies of quantum systems from first principles. By harnessing the capabilities of deep learning, FermiNet provides a more accurate and scalable approach to solving the Schrödinger equation, paving the way for new discoveries in chemistry and physics. As researchers continue to refine and expand upon this innovative architecture, FermiNet is poised to play a crucial role in shaping the future of quantum science and technology.
Understanding The Architecture Of FermiNet
FermiNet, a groundbreaking neural network architecture, has emerged as a pivotal tool in the exploration of quantum physics and chemistry from first principles. This innovative approach, developed by researchers at DeepMind, leverages the power of machine learning to solve the Schrödinger equation, a fundamental equation in quantum mechanics that describes how quantum systems evolve over time. By understanding the architecture of FermiNet, one can appreciate its potential to revolutionize the way scientists approach complex quantum systems.
At its core, FermiNet is designed to approximate the wave function of a quantum system. The wave function is a mathematical description that encapsulates all the information about a system’s quantum state. Traditionally, solving the Schrödinger equation for many-electron systems has been a formidable challenge due to the exponential growth in complexity as the number of particles increases. FermiNet addresses this challenge by employing a neural network architecture that is specifically tailored to respect the antisymmetry property of fermionic wave functions, which is a crucial aspect of quantum mechanics.
The architecture of FermiNet is built upon several key components that work in harmony to model the wave function accurately. One of the fundamental features of FermiNet is its use of permutation-equivariant layers. These layers ensure that the network’s output remains consistent under permutations of identical particles, a property that is essential for modeling systems of indistinguishable fermions such as electrons. By incorporating this symmetry directly into the architecture, FermiNet is able to capture the complex interactions between particles more effectively than traditional methods.
Moreover, FermiNet employs a deep neural network structure with multiple hidden layers, allowing it to learn intricate patterns and relationships within the data. Each layer in the network is designed to process information in a way that mimics the hierarchical nature of quantum interactions. This hierarchical processing enables FermiNet to build a comprehensive representation of the wave function, capturing both short-range and long-range correlations between particles. As a result, the network can model the behavior of quantum systems with remarkable accuracy.
In addition to its architectural innovations, FermiNet utilizes advanced optimization techniques to train the network. The training process involves minimizing the energy of the system, which is calculated using the variational principle. By iteratively adjusting the network’s parameters to find the lowest possible energy state, FermiNet effectively learns the optimal wave function for the given system. This approach not only ensures that the network produces physically meaningful results but also allows it to generalize to a wide range of quantum systems.
Furthermore, FermiNet’s architecture is designed to be highly scalable, making it suitable for tackling large and complex quantum systems that were previously intractable. The network’s ability to handle many-electron systems with high accuracy opens up new possibilities for research in quantum chemistry and materials science. By providing insights into the electronic structure of molecules and materials, FermiNet has the potential to accelerate the discovery of new compounds and the development of novel technologies.
In conclusion, the architecture of FermiNet represents a significant advancement in the application of machine learning to quantum physics and chemistry. By incorporating principles of quantum mechanics directly into its design, FermiNet offers a powerful tool for solving the Schrödinger equation and exploring the behavior of complex quantum systems. As researchers continue to refine and expand upon this architecture, FermiNet is poised to play a crucial role in advancing our understanding of the quantum world.
Comparing FermiNet With Traditional Quantum Methods
FermiNet, a neural network-based approach developed by researchers at DeepMind, represents a significant advancement in the field of quantum physics and chemistry. It offers a novel method for solving the Schrödinger equation, which is fundamental to understanding the behavior of quantum systems. Traditional quantum methods, such as the Hartree-Fock and Density Functional Theory (DFT), have long been the cornerstone of computational quantum chemistry. However, these methods often involve approximations that can limit their accuracy and applicability, particularly for complex systems. In contrast, FermiNet aims to address these limitations by leveraging the power of machine learning to provide more accurate solutions from first principles.
To begin with, traditional methods like Hartree-Fock rely on approximating the wave function of a quantum system as a single Slater determinant. This approach simplifies the problem but can lead to inaccuracies, especially in systems with strong electron correlation. DFT, on the other hand, uses electron density rather than wave functions to describe a system, which can be computationally efficient but often requires empirical functionals that may not be universally applicable. These methods, while effective for many systems, can struggle with accurately predicting properties of complex molecules or materials where electron interactions play a crucial role.
FermiNet, however, approaches the problem differently by using a neural network to directly approximate the wave function of a quantum system. This method allows for a more flexible and accurate representation of electron correlations. The neural network is trained to minimize the energy of the system, effectively solving the Schrödinger equation without relying on the approximations inherent in traditional methods. This approach not only enhances accuracy but also provides a more general framework that can be applied to a wider range of systems.
Moreover, FermiNet’s ability to learn from data and improve over time offers a distinct advantage over traditional methods. While methods like Hartree-Fock and DFT are based on fixed mathematical frameworks, FermiNet can adapt and refine its predictions as more data becomes available. This adaptability is particularly valuable in the study of complex quantum systems, where traditional methods may require significant modifications or extensions to achieve similar levels of accuracy.
In addition to its adaptability, FermiNet also benefits from the computational power of modern machine learning techniques. The use of neural networks allows for parallel processing and efficient handling of large datasets, which can significantly reduce computation times compared to traditional methods. This efficiency makes FermiNet a promising tool for tackling large-scale quantum problems that were previously intractable.
However, it is important to note that FermiNet is not without its challenges. The training of neural networks requires substantial computational resources and expertise in machine learning, which may limit its accessibility to some researchers. Furthermore, while FermiNet has shown great promise, it is still a relatively new approach and ongoing research is needed to fully understand its capabilities and limitations.
In conclusion, FermiNet represents a groundbreaking approach to solving quantum problems from first principles, offering significant advantages over traditional methods in terms of accuracy, adaptability, and computational efficiency. As research in this area continues to evolve, FermiNet has the potential to transform our understanding of quantum systems and open new avenues for exploration in quantum physics and chemistry.
Applications Of FermiNet In Modern Quantum Research
FermiNet, a neural network architecture developed by DeepMind, has emerged as a groundbreaking tool in the realm of quantum physics and chemistry, offering unprecedented insights into the behavior of quantum systems from first principles. This innovative approach leverages the power of machine learning to solve the Schrödinger equation, a fundamental equation in quantum mechanics that describes how the quantum state of a physical system changes over time. By doing so, FermiNet provides a novel method for understanding complex quantum systems, which has significant implications for modern quantum research.
One of the primary applications of FermiNet is in the field of quantum chemistry, where it is used to predict the electronic structure of molecules. Traditional methods for solving the Schrödinger equation, such as the Hartree-Fock method and density functional theory, often involve approximations that can limit their accuracy. In contrast, FermiNet employs a deep neural network to directly approximate the wave function of a quantum system, allowing for more precise calculations. This capability is particularly valuable for studying molecules with strong electron correlations, where conventional methods struggle to provide accurate results. Consequently, FermiNet has the potential to revolutionize the design of new materials and drugs by enabling researchers to predict molecular properties with greater accuracy.
Moreover, FermiNet’s ability to model quantum systems extends beyond chemistry to the broader field of condensed matter physics. In this domain, understanding the behavior of electrons in solids is crucial for developing new technologies, such as superconductors and quantum computers. FermiNet’s approach allows researchers to explore the properties of complex materials at a fundamental level, providing insights that could lead to the discovery of novel materials with unique electronic properties. By offering a more detailed understanding of electron interactions in solids, FermiNet contributes to the advancement of technologies that rely on quantum mechanical principles.
In addition to its applications in chemistry and condensed matter physics, FermiNet also holds promise for advancing our understanding of fundamental quantum phenomena. For instance, it can be used to study quantum entanglement, a phenomenon where particles become interconnected in such a way that the state of one particle cannot be described independently of the state of the other. Understanding entanglement is essential for the development of quantum computing and quantum communication technologies. FermiNet’s ability to model entangled states with high accuracy provides researchers with a powerful tool for exploring these complex quantum phenomena.
Furthermore, FermiNet’s impact on quantum research is amplified by its potential to reduce computational costs. Traditional quantum mechanical calculations can be computationally expensive, often requiring significant resources and time. By utilizing machine learning techniques, FermiNet can perform these calculations more efficiently, making it accessible to a broader range of researchers and applications. This efficiency not only accelerates the pace of research but also democratizes access to advanced quantum modeling tools.
In conclusion, FermiNet represents a significant advancement in the application of machine learning to quantum research. Its ability to accurately model quantum systems from first principles opens new avenues for exploration in quantum chemistry, condensed matter physics, and fundamental quantum mechanics. As researchers continue to refine and expand upon this innovative approach, FermiNet is poised to play a pivotal role in shaping the future of quantum science and technology, offering insights that were previously beyond reach.
Future Prospects Of FermiNet In Quantum Computing
FermiNet, a neural network architecture developed by DeepMind, represents a significant advancement in the field of quantum computing, particularly in the simulation of quantum systems. As researchers continue to explore the potential of FermiNet, its future prospects in quantum computing appear promising. This innovative approach leverages the principles of quantum mechanics to solve complex problems in physics and chemistry, offering a glimpse into a future where computational power can be harnessed to tackle challenges that were previously insurmountable.
One of the most compelling aspects of FermiNet is its ability to model quantum systems from first principles. Unlike traditional methods that rely on approximations, FermiNet utilizes a neural network to directly represent the wave functions of quantum systems. This capability allows for more accurate simulations of molecular systems, which is crucial for understanding chemical reactions and material properties at a fundamental level. As a result, FermiNet has the potential to revolutionize fields such as drug discovery and materials science by providing insights that were previously beyond reach.
Moreover, the scalability of FermiNet is a key factor in its future prospects. As quantum systems increase in complexity, the computational resources required to simulate them grow exponentially. FermiNet addresses this challenge by efficiently scaling with the size of the system, making it feasible to study larger and more intricate quantum systems. This scalability is particularly important as researchers aim to simulate complex molecules and materials that are relevant to real-world applications. By enabling the study of these systems, FermiNet could accelerate the development of new technologies and materials that have a profound impact on various industries.
In addition to its scalability, FermiNet’s adaptability is another promising feature. The architecture can be tailored to different types of quantum systems, allowing researchers to apply it to a wide range of problems. This flexibility is essential as the field of quantum computing continues to evolve, with new challenges and opportunities emerging regularly. By adapting to these changes, FermiNet can remain at the forefront of quantum research, driving innovation and discovery in the years to come.
Furthermore, the integration of FermiNet with existing quantum computing technologies could enhance its capabilities even further. As quantum hardware continues to advance, the combination of FermiNet’s powerful simulation capabilities with cutting-edge quantum processors could unlock new possibilities for solving complex problems. This synergy between software and hardware is likely to be a critical factor in the future success of quantum computing, and FermiNet is well-positioned to play a central role in this development.
Looking ahead, the potential applications of FermiNet extend beyond traditional domains of physics and chemistry. As researchers continue to refine and expand its capabilities, FermiNet could be applied to a broader range of fields, including cryptography, optimization, and artificial intelligence. By providing a deeper understanding of quantum systems, FermiNet could contribute to breakthroughs in these areas, further solidifying its place as a transformative tool in the realm of quantum computing.
In conclusion, the future prospects of FermiNet in quantum computing are both exciting and expansive. Its ability to model quantum systems from first principles, combined with its scalability and adaptability, positions it as a powerful tool for advancing our understanding of the quantum world. As researchers continue to explore its potential, FermiNet is poised to make significant contributions to science and technology, paving the way for a new era of discovery and innovation.
Q&A
1. **What is FermiNet?**
FermiNet is a neural network architecture designed to solve the electronic Schrödinger equation for quantum systems, enabling the exploration of quantum physics and chemistry from first principles.
2. **Who developed FermiNet?**
FermiNet was developed by researchers at DeepMind, a leading artificial intelligence research lab.
3. **What problem does FermiNet address?**
FermiNet addresses the challenge of accurately simulating quantum systems, particularly the electronic structure of atoms and molecules, which is crucial for understanding chemical properties and reactions.
4. **How does FermiNet work?**
FermiNet uses a neural network to represent the wave function of a quantum system, optimizing it to minimize the energy of the system, thereby solving the Schrödinger equation.
5. **What are the advantages of using FermiNet?**
FermiNet offers improved accuracy and scalability over traditional quantum chemistry methods, allowing for more precise simulations of complex quantum systems.
6. **What are potential applications of FermiNet?**
Potential applications of FermiNet include drug discovery, materials science, and the development of new chemical processes, as it provides insights into the fundamental behavior of molecules and materials.FermiNet represents a significant advancement in the application of deep learning to quantum physics and chemistry, offering a novel approach to solving the electronic Schrödinger equation from first principles. By leveraging neural networks to approximate wave functions, FermiNet provides a scalable and flexible framework that can handle complex many-electron systems with high accuracy. This method not only improves upon traditional quantum Monte Carlo techniques but also opens new avenues for exploring quantum systems that were previously computationally prohibitive. As a result, FermiNet holds promise for advancing our understanding of quantum phenomena and facilitating the discovery of new materials and molecules.
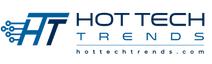