The Massachusetts Institute of Technology (MIT) is at the forefront of a groundbreaking innovation poised to revolutionize the field of robot training. Leveraging cutting-edge advancements in artificial intelligence and machine learning, MIT researchers have developed a novel approach that significantly enhances the efficiency and effectiveness of training robotic systems. This pioneering method integrates sophisticated algorithms with real-world data, enabling robots to learn complex tasks with unprecedented speed and accuracy. By streamlining the training process, this innovation not only reduces the time and resources required to deploy functional robots but also expands the potential applications of robotics across various industries. As MIT continues to push the boundaries of technological innovation, this development marks a significant leap forward in the quest to create intelligent, adaptable, and autonomous robotic systems.
Advanced Machine Learning Techniques in Robot Training
In recent years, the field of robotics has witnessed remarkable advancements, largely driven by the integration of sophisticated machine learning techniques. Among the institutions at the forefront of this technological evolution is the Massachusetts Institute of Technology (MIT), where researchers are pioneering innovative methods to enhance robot training. These advancements are poised to revolutionize the way robots learn and interact with their environments, promising significant improvements in efficiency and adaptability.
At the core of this innovation is the application of advanced machine learning algorithms that enable robots to acquire skills through experience, much like humans do. Traditional robot training methods often rely on pre-programmed instructions, which can be limiting in dynamic and unpredictable environments. In contrast, machine learning allows robots to learn from data, adapt to new situations, and improve their performance over time. This shift from static programming to dynamic learning is a game-changer, offering the potential for robots to operate autonomously in complex settings.
One of the key techniques being explored at MIT is reinforcement learning, a type of machine learning where robots learn to make decisions by receiving feedback from their actions. This approach mimics the trial-and-error process that humans use to learn new skills. By interacting with their environment and receiving rewards or penalties based on their actions, robots can gradually develop optimal strategies for performing tasks. This method is particularly useful in scenarios where explicit programming is impractical or impossible, such as navigating unfamiliar terrain or handling delicate objects.
Moreover, MIT researchers are also investigating the use of imitation learning, where robots learn by observing and mimicking human actions. This technique leverages the natural ability of humans to demonstrate tasks, allowing robots to acquire complex skills more quickly and intuitively. By combining imitation learning with reinforcement learning, robots can benefit from both human expertise and autonomous exploration, leading to more robust and versatile training outcomes.
In addition to these learning techniques, MIT is also focusing on the development of advanced simulation environments. These virtual platforms provide a safe and controlled space for robots to practice and refine their skills before deploying them in the real world. By simulating a wide range of scenarios and conditions, researchers can expose robots to diverse challenges, accelerating their learning process and enhancing their adaptability. This approach not only reduces the risk of errors in real-world applications but also significantly cuts down on the time and resources required for training.
Furthermore, the integration of machine learning in robot training is opening new avenues for collaboration between humans and robots. By equipping robots with the ability to understand and respond to human intentions, researchers are paving the way for more seamless and intuitive human-robot interactions. This development holds great promise for industries such as healthcare, manufacturing, and logistics, where robots can assist humans in performing tasks more efficiently and safely.
In conclusion, the innovative machine learning techniques being developed at MIT are set to transform the landscape of robot training. By enabling robots to learn from experience, adapt to new environments, and collaborate with humans, these advancements are not only enhancing the capabilities of robots but also expanding the possibilities for their application across various sectors. As these technologies continue to evolve, they hold the potential to redefine the relationship between humans and machines, ushering in a new era of intelligent automation.
The Role of Simulation Environments in Enhancing Robotic Skills
In recent years, the field of robotics has witnessed remarkable advancements, largely driven by the integration of sophisticated simulation environments. These virtual platforms have become indispensable tools in the development and training of robotic systems, offering a controlled and flexible setting for experimentation. At the forefront of this innovation is the Massachusetts Institute of Technology (MIT), whose groundbreaking work in simulation technology is poised to revolutionize the way robots are trained, ultimately enhancing their skills and capabilities.
Simulation environments provide a unique opportunity for researchers and developers to test robotic algorithms and behaviors without the constraints and risks associated with real-world testing. By creating detailed virtual replicas of physical environments, these platforms allow for the meticulous examination of robotic performance under a variety of conditions. This capability is particularly crucial in scenarios where real-world testing may be impractical or hazardous, such as in disaster response or space exploration. Consequently, simulation environments have become a cornerstone in the iterative process of refining robotic systems, enabling rapid prototyping and testing.
MIT’s contribution to this field is particularly noteworthy. The institution has developed advanced simulation tools that incorporate cutting-edge technologies such as machine learning and artificial intelligence. These tools are designed to mimic complex real-world interactions with a high degree of accuracy, providing robots with a rich and diverse set of experiences from which to learn. By leveraging these sophisticated simulations, robots can be trained to adapt to dynamic environments, recognize and respond to unforeseen obstacles, and execute tasks with greater precision and efficiency.
Moreover, the use of simulation environments in robotic training offers significant advantages in terms of cost and scalability. Traditional methods of training robots often require expensive physical prototypes and extensive trial-and-error testing, which can be both time-consuming and resource-intensive. In contrast, virtual simulations allow for the simultaneous training of multiple robotic systems, significantly reducing the time and cost associated with development. This scalability is particularly beneficial for industries that rely on large fleets of robots, such as manufacturing and logistics, where efficiency and consistency are paramount.
Furthermore, simulation environments facilitate the integration of collaborative learning among robots. By sharing data and experiences within a simulated setting, robots can collectively improve their performance and develop more sophisticated strategies for problem-solving. This collaborative approach not only accelerates the learning process but also fosters innovation, as robots can experiment with novel solutions in a risk-free environment. As a result, the potential for robots to tackle increasingly complex tasks is greatly enhanced.
In addition to these practical benefits, the use of simulation environments in robotic training also has profound implications for the future of human-robot interaction. As robots become more adept at navigating and understanding their surroundings, they are better equipped to work alongside humans in a variety of settings, from healthcare to hospitality. This improved interaction is likely to lead to greater acceptance and integration of robots into everyday life, ultimately transforming the way we live and work.
In conclusion, the role of simulation environments in enhancing robotic skills cannot be overstated. Through the pioneering efforts of institutions like MIT, these virtual platforms are set to redefine the landscape of robotic training, offering unprecedented opportunities for innovation and advancement. As we continue to explore the potential of these technologies, the future of robotics promises to be both exciting and transformative.
Human-Robot Interaction: Bridging the Gap with Innovative Technologies
In recent years, the field of human-robot interaction has witnessed remarkable advancements, with researchers striving to bridge the gap between human capabilities and robotic functionalities. A groundbreaking innovation from the Massachusetts Institute of Technology (MIT) is poised to revolutionize the way robots are trained, offering promising implications for various industries. This innovation, which leverages advanced machine learning algorithms and intuitive human-robot interfaces, aims to enhance the efficiency and effectiveness of robot training processes.
Traditionally, training robots to perform complex tasks has been a time-consuming and resource-intensive endeavor. Engineers and programmers have had to meticulously code each action, often requiring extensive trial and error to achieve the desired outcomes. However, MIT’s novel approach introduces a paradigm shift by enabling robots to learn from human demonstrations in a more natural and intuitive manner. This is achieved through the integration of sophisticated sensors and machine learning models that allow robots to observe and mimic human actions with remarkable precision.
One of the key components of this innovation is the use of advanced computer vision systems. These systems enable robots to perceive their environment in a manner akin to human vision, allowing them to recognize objects, interpret gestures, and understand spatial relationships. By processing visual data in real-time, robots can adapt to dynamic environments and perform tasks with a level of dexterity that was previously unattainable. This capability is particularly beneficial in industries such as manufacturing and logistics, where robots are required to handle a diverse array of objects and navigate complex settings.
Moreover, the integration of natural language processing technologies further enhances the human-robot interaction experience. By enabling robots to comprehend and respond to verbal instructions, this innovation facilitates seamless communication between humans and machines. This is especially advantageous in collaborative settings, where human workers and robots must work in tandem to achieve common goals. The ability to communicate effectively not only improves task efficiency but also fosters a sense of trust and cooperation between human operators and their robotic counterparts.
In addition to these technological advancements, MIT’s innovation emphasizes the importance of user-friendly interfaces. By designing intuitive control systems, researchers aim to democratize robot training, making it accessible to individuals without extensive technical expertise. This democratization has the potential to accelerate the adoption of robotic technologies across various sectors, from healthcare to agriculture, where specialized knowledge is often a barrier to entry.
Furthermore, the implications of this innovation extend beyond immediate practical applications. By streamlining the robot training process, MIT’s approach paves the way for more rapid prototyping and deployment of robotic solutions. This agility is crucial in addressing emerging challenges and opportunities in a rapidly evolving technological landscape. As industries continue to grapple with issues such as labor shortages and increasing demand for automation, the ability to quickly and efficiently train robots becomes a significant competitive advantage.
In conclusion, MIT’s innovative approach to robot training represents a significant leap forward in the field of human-robot interaction. By harnessing the power of advanced machine learning, computer vision, and natural language processing, this innovation not only enhances the capabilities of robots but also fosters a more harmonious and productive relationship between humans and machines. As this technology continues to evolve, it holds the promise of transforming industries and redefining the possibilities of human-robot collaboration.
Real-Time Data Processing for Adaptive Robot Learning
In recent years, the field of robotics has witnessed remarkable advancements, with innovations that continue to push the boundaries of what machines can achieve. Among these groundbreaking developments, a new approach pioneered by researchers at the Massachusetts Institute of Technology (MIT) is poised to revolutionize the way robots are trained. This innovation centers around real-time data processing for adaptive robot learning, a method that promises to enhance the efficiency and effectiveness of robotic systems across various applications.
Traditionally, robot training has relied heavily on pre-programmed instructions and extensive datasets, which are used to teach machines how to perform specific tasks. However, this approach often lacks the flexibility needed to adapt to dynamic environments or unforeseen challenges. Recognizing this limitation, MIT researchers have developed a novel system that leverages real-time data processing to enable robots to learn and adapt on the fly. By integrating advanced algorithms and machine learning techniques, this system allows robots to process and analyze data as it is collected, facilitating immediate adjustments to their actions and strategies.
One of the key components of this innovative approach is the use of sophisticated sensors that continuously gather information from the robot’s surroundings. These sensors capture a wide array of data, including visual, auditory, and tactile inputs, which are then processed in real-time by the robot’s onboard computing system. This immediate processing capability is crucial, as it enables the robot to make informed decisions based on the most current information available, rather than relying on outdated or static data.
Moreover, the integration of machine learning algorithms allows the robot to identify patterns and correlations within the data, leading to more nuanced and effective responses. For instance, a robot equipped with this technology could navigate a cluttered environment by recognizing obstacles and adjusting its path accordingly, all while continuously learning from its interactions with the environment. This adaptability is particularly valuable in scenarios where conditions are constantly changing, such as in search and rescue operations or autonomous vehicle navigation.
In addition to enhancing the robot’s ability to adapt, real-time data processing also significantly reduces the time required for training. Traditional methods often involve lengthy training periods, during which robots must be exposed to vast amounts of data to achieve a satisfactory level of proficiency. In contrast, the MIT system allows robots to learn more efficiently by focusing on relevant data and discarding extraneous information. This streamlined approach not only accelerates the training process but also reduces the computational resources needed, making it a more sustainable and cost-effective solution.
Furthermore, the implications of this technology extend beyond individual robots. By enabling machines to learn and adapt in real-time, this approach opens up new possibilities for collaborative robotics, where multiple robots work together to achieve a common goal. In such scenarios, robots can share data and insights with one another, leading to a collective intelligence that enhances their overall performance.
In conclusion, the real-time data processing system developed by MIT represents a significant leap forward in the field of robotics. By allowing robots to learn and adapt in real-time, this innovation addresses many of the limitations associated with traditional training methods, offering a more flexible, efficient, and effective solution. As this technology continues to evolve, it holds the potential to transform a wide range of industries, from manufacturing and logistics to healthcare and beyond, ultimately paving the way for a new era of intelligent and adaptive robotic systems.
The Impact of Quantum Computing on Robotic Training Efficiency
The advent of quantum computing is set to transform numerous fields, and one of the most promising areas of impact is robotic training efficiency. At the forefront of this technological revolution is the Massachusetts Institute of Technology (MIT), where researchers are pioneering innovative methods to harness the power of quantum computing to enhance the capabilities of robots. This development is not merely an incremental improvement but a potential paradigm shift in how robots are trained, offering unprecedented speed and efficiency.
Traditionally, robotic training has relied heavily on classical computing methods, which, while effective, are limited by the constraints of binary processing. These methods require extensive computational resources and time, especially when dealing with complex tasks that involve large datasets or intricate algorithms. However, quantum computing introduces a new dimension to this process by leveraging the principles of superposition and entanglement, allowing for the simultaneous processing of vast amounts of information. This capability significantly reduces the time required for training robots, enabling them to learn and adapt at a pace previously deemed impossible.
MIT’s research into quantum computing for robotic training is particularly focused on optimizing machine learning algorithms. Machine learning, a subset of artificial intelligence, is crucial for enabling robots to perform tasks autonomously by learning from data. Quantum computing can enhance these algorithms by providing more efficient ways to process and analyze data, leading to faster and more accurate learning outcomes. For instance, quantum algorithms can solve complex optimization problems more efficiently than classical algorithms, which is essential for training robots to navigate dynamic environments or perform intricate tasks.
Moreover, the integration of quantum computing into robotic training is expected to have a profound impact on the development of autonomous systems. As robots become more adept at learning from their environments, they can operate with greater autonomy and reliability. This advancement is particularly significant in fields such as healthcare, manufacturing, and logistics, where robots are increasingly deployed to perform tasks that require precision and adaptability. By improving the efficiency of robotic training, quantum computing can accelerate the deployment of these autonomous systems, leading to enhanced productivity and safety.
In addition to improving training efficiency, quantum computing also offers the potential for more robust and secure robotic systems. Quantum algorithms can enhance the security of data transmission and storage, which is critical for protecting sensitive information in applications such as autonomous vehicles or medical robots. This added layer of security ensures that robots can operate safely and effectively in environments where data integrity is paramount.
While the potential benefits of quantum computing for robotic training are immense, it is important to acknowledge the challenges that lie ahead. The field of quantum computing is still in its nascent stages, and significant research and development are required to fully realize its potential. Issues such as error rates, scalability, and the development of quantum hardware must be addressed to ensure the practical application of quantum computing in robotic training.
In conclusion, the integration of quantum computing into robotic training represents a significant leap forward in the field of robotics. MIT’s pioneering research in this area is poised to revolutionize how robots are trained, offering faster, more efficient, and more secure methods of learning. As this technology continues to evolve, it holds the promise of transforming industries and enhancing the capabilities of robots in ways that were once thought to be the realm of science fiction.
Collaborative Robotics: Innovations in Team-Based Learning Approaches
In recent years, the field of robotics has witnessed remarkable advancements, with collaborative robotics emerging as a particularly promising area. At the forefront of this innovation is the Massachusetts Institute of Technology (MIT), where researchers are pioneering new methods to enhance robot training through team-based learning approaches. This groundbreaking work is poised to revolutionize how robots are trained, ultimately leading to more efficient and effective robotic systems.
Traditionally, robot training has been a solitary endeavor, with each robot learning tasks independently. However, MIT’s novel approach leverages the power of collaboration, drawing inspiration from human team dynamics. By enabling robots to learn in groups, researchers aim to replicate the benefits of human teamwork, such as shared knowledge, diverse perspectives, and collective problem-solving. This shift from individual to collaborative learning marks a significant departure from conventional methods and holds the potential to accelerate the development of intelligent robotic systems.
One of the key innovations driving this transformation is the use of advanced machine learning algorithms that facilitate communication and cooperation among robots. These algorithms allow robots to share information and learn from each other’s experiences, thereby enhancing their collective intelligence. For instance, when one robot encounters a novel obstacle, it can relay this information to its peers, enabling them to adapt and respond more effectively in similar situations. This collaborative learning process not only improves the robots’ performance but also reduces the time and resources required for training.
Moreover, MIT’s approach emphasizes the importance of diversity within robotic teams. By incorporating robots with varying capabilities and specializations, researchers can simulate real-world scenarios where diverse teams often outperform homogeneous ones. This diversity fosters a richer learning environment, as robots can draw on each other’s strengths and compensate for individual weaknesses. Consequently, this leads to more robust and versatile robotic systems capable of tackling complex tasks that would be challenging for a single robot to handle alone.
In addition to enhancing robot performance, collaborative learning approaches also have significant implications for human-robot interaction. As robots become more adept at working in teams, they can better integrate into human environments, where collaboration and communication are essential. This paves the way for more seamless and intuitive interactions between humans and robots, ultimately expanding the potential applications of robotic technology across various industries.
Furthermore, the insights gained from MIT’s research have the potential to influence other areas of artificial intelligence and machine learning. By demonstrating the efficacy of team-based learning, these innovations could inspire new approaches to training AI systems in fields such as autonomous vehicles, healthcare, and logistics. The principles of collaboration and diversity, which are central to MIT’s work, may prove invaluable in developing AI systems that are not only more capable but also more adaptable to the complexities of the real world.
In conclusion, MIT’s pioneering efforts in collaborative robotics represent a significant leap forward in the field of robot training. By harnessing the power of teamwork and diversity, researchers are laying the groundwork for a new era of intelligent robotic systems. As these innovations continue to evolve, they hold the promise of transforming not only the capabilities of robots but also their role in society, ultimately leading to a future where humans and robots work together in harmony to achieve shared goals.
Q&A
1. **What is the MIT innovation in robot training?**
MIT researchers have developed a new machine learning technique that allows robots to learn tasks more efficiently by using a combination of simulation and real-world data.
2. **How does this innovation improve robot training?**
The innovation accelerates the training process by enabling robots to learn from a smaller set of real-world examples, reducing the time and resources needed for training.
3. **What is the key technology behind this innovation?**
The key technology is a hybrid learning model that integrates reinforcement learning with advanced simulation environments, allowing robots to generalize from simulated experiences to real-world applications.
4. **What are the potential applications of this innovation?**
Potential applications include autonomous vehicles, industrial automation, healthcare robotics, and any field where robots need to adapt to complex, dynamic environments.
5. **How does this innovation impact the cost of robot training?**
By reducing the reliance on extensive real-world trials, the innovation significantly lowers the cost associated with robot training, making it more accessible for various industries.
6. **What challenges does this innovation address in current robot training methods?**
It addresses challenges such as the high cost, time consumption, and limited adaptability of robots trained solely on real-world data, providing a more efficient and scalable solution.The MIT innovation in robot training is set to revolutionize the field by introducing advanced methodologies that significantly enhance the efficiency and effectiveness of robotic learning processes. By leveraging cutting-edge technologies and novel algorithms, this innovation allows robots to acquire skills and adapt to new tasks with unprecedented speed and accuracy. This breakthrough not only reduces the time and resources required for robot training but also expands the potential applications of robotics across various industries. As a result, the MIT innovation is poised to drive significant advancements in automation, leading to increased productivity, improved performance, and the potential for transformative impacts on sectors ranging from manufacturing to healthcare.
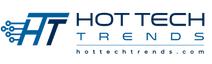