Deep learning models have increasingly drawn inspiration from various domains to enhance their capabilities and understanding of complex systems. One intriguing intersection is the emulation of intuitive physics through insights derived from developmental psychology. This approach leverages the cognitive processes observed in human development to inform the design and training of artificial intelligence systems. By mimicking the way infants and young children perceive and interact with the physical world, these models aim to replicate the innate human ability to predict and understand physical phenomena. This fusion of developmental psychology and deep learning not only advances the field of AI but also provides a deeper understanding of human cognition and its potential applications in creating more sophisticated and intuitive machine learning systems.
Understanding Intuitive Physics: How Deep Learning Models Are Inspired by Developmental Psychology
In recent years, the intersection of artificial intelligence and cognitive science has led to groundbreaking advancements in understanding how machines can emulate human-like reasoning. One particularly intriguing area of study is the development of deep learning models that mimic intuitive physics, a concept rooted in developmental psychology. Intuitive physics refers to the innate ability of humans, even from a very young age, to understand and predict the behavior of physical objects in the world. This ability is not learned through formal education but rather emerges naturally as children interact with their environment. By drawing insights from developmental psychology, researchers are now able to design deep learning models that replicate this intuitive understanding, thereby enhancing the capabilities of artificial intelligence systems.
The foundation of this approach lies in the observation that infants possess a rudimentary understanding of physical principles such as gravity, object permanence, and causality. Developmental psychologists have long studied how these cognitive abilities evolve, providing a rich source of data on the stages of human cognitive development. By leveraging these insights, AI researchers can create models that simulate the learning processes observed in children. This involves training neural networks to recognize patterns and make predictions about physical interactions, much like a child learns through trial and error.
One of the key challenges in this endeavor is designing models that can generalize from limited data, a hallmark of human cognition. Unlike traditional machine learning models that require vast amounts of labeled data, humans can infer complex physical laws from relatively few observations. To address this, researchers employ techniques such as transfer learning and reinforcement learning, which allow models to apply knowledge gained from one context to new, unseen situations. This not only improves the efficiency of the learning process but also enhances the model’s ability to adapt to diverse environments.
Moreover, the integration of developmental psychology insights into deep learning models has led to the creation of more robust and flexible AI systems. For instance, by understanding how children develop an awareness of object permanence—the understanding that objects continue to exist even when out of sight—researchers can design models that better handle occlusion and partial observability in dynamic environments. This has significant implications for fields such as robotics and autonomous vehicles, where the ability to predict and respond to changes in the environment is crucial.
Furthermore, the collaboration between cognitive scientists and AI researchers has opened new avenues for exploring the ethical and philosophical dimensions of artificial intelligence. By studying how humans develop moral reasoning and social cognition, researchers can inform the design of AI systems that not only understand physical interactions but also navigate complex social dynamics. This interdisciplinary approach ensures that AI technologies are developed with a deeper understanding of human values and behaviors, ultimately leading to more harmonious integration into society.
In conclusion, the emulation of intuitive physics through deep learning models represents a promising frontier in artificial intelligence research. By drawing on insights from developmental psychology, researchers are creating systems that not only mimic human cognitive abilities but also push the boundaries of what machines can achieve. As this field continues to evolve, it holds the potential to revolutionize how we interact with technology, offering new possibilities for innovation and discovery. Through the seamless integration of cognitive science and AI, we are inching closer to a future where machines can think and learn in ways that are profoundly human-like.
Bridging AI and Human Cognition: The Role of Developmental Psychology in Deep Learning
In recent years, the field of artificial intelligence has made significant strides, particularly in the realm of deep learning. These advancements have been instrumental in enabling machines to perform tasks that were once thought to be the exclusive domain of human cognition. One of the most intriguing developments in this area is the emulation of intuitive physics by deep learning models, a feat that has been achieved by drawing insights from developmental psychology. This interdisciplinary approach not only enhances the capabilities of AI but also provides a deeper understanding of human cognitive processes.
Intuitive physics refers to the innate ability of humans, even from a very young age, to understand and predict the behavior of objects in the physical world. This includes concepts such as gravity, object permanence, and causality. Developmental psychology has long studied how infants and children develop these intuitive understandings, providing a rich source of insights into the cognitive processes that underpin them. By leveraging these insights, researchers in AI have been able to design deep learning models that mimic this aspect of human cognition.
The integration of developmental psychology into AI research involves the use of cognitive models that simulate the learning processes observed in children. These models are designed to learn from experience, much like a child does, by interacting with their environment and gradually building an understanding of physical laws. This approach contrasts with traditional AI models, which often rely on large datasets and predefined rules. By adopting a more human-like learning process, these deep learning models can achieve a more nuanced understanding of the physical world.
One of the key benefits of this approach is the ability of AI systems to generalize from limited data. Just as children can infer the properties of objects they have never encountered before, these models can make predictions about new situations based on their learned understanding of physics. This capability is particularly valuable in dynamic and unpredictable environments, where pre-programmed rules may fall short. Moreover, this method of learning aligns more closely with how humans naturally acquire knowledge, making AI systems more adaptable and robust.
Furthermore, the collaboration between AI and developmental psychology has the potential to shed light on the nature of human cognition itself. By modeling the learning processes of children, researchers can test hypotheses about how intuitive physics is acquired and refined over time. This not only advances the field of AI but also contributes to our understanding of cognitive development in humans. The insights gained from this research could have far-reaching implications, influencing educational strategies and therapeutic interventions for cognitive impairments.
In addition to these cognitive benefits, the emulation of intuitive physics by AI systems has practical applications across various industries. For instance, in robotics, machines equipped with this capability can navigate complex environments more effectively, improving their utility in tasks such as search and rescue operations or autonomous driving. Similarly, in the field of virtual reality, more realistic simulations can be created, enhancing user experiences in gaming and training applications.
In conclusion, the intersection of deep learning and developmental psychology represents a promising frontier in the quest to bridge AI and human cognition. By emulating the intuitive physics that humans naturally possess, AI systems can achieve a level of understanding and adaptability that was previously unattainable. This interdisciplinary approach not only advances the capabilities of artificial intelligence but also enriches our understanding of the human mind, paving the way for future innovations in both fields. As research continues to evolve, the collaboration between these disciplines will undoubtedly yield further insights and breakthroughs, shaping the future of intelligent systems.
Emulating Human Intuition: Deep Learning Models and Their Psychological Foundations
In recent years, the field of artificial intelligence has made significant strides in developing models that emulate human-like intuition, particularly in understanding the physical world. One of the most promising approaches in this endeavor is the integration of insights from developmental psychology into deep learning models. This interdisciplinary approach seeks to replicate the intuitive grasp of physics that humans naturally develop from a young age. By examining how infants and children learn to navigate their environment, researchers aim to create AI systems that can similarly understand and predict physical interactions.
The concept of intuitive physics refers to the innate ability of humans to predict and understand the behavior of objects in the physical world. This includes anticipating how objects will move, interact, and respond to various forces. Developmental psychology has long studied how these abilities emerge in humans, revealing that even infants possess a rudimentary understanding of physical principles such as gravity, solidity, and continuity. These insights have inspired AI researchers to incorporate similar learning mechanisms into deep learning models, allowing machines to develop a comparable intuitive understanding of physics.
One of the key strategies in this pursuit is the use of unsupervised learning techniques, which enable models to learn from raw sensory data without explicit labels or instructions. This approach mirrors the way infants learn about the world through observation and interaction, gradually building a mental model of their environment. By training deep learning models on large datasets of videos depicting physical interactions, researchers aim to imbue these systems with the ability to predict future states of objects based on their current configurations. This capability is crucial for applications such as robotics, where machines must navigate complex environments and manipulate objects with precision.
Moreover, the integration of developmental psychology insights into AI models has led to the development of more sophisticated neural architectures. These architectures are designed to mimic the hierarchical and modular nature of the human brain, allowing for the efficient processing of sensory information and the formation of abstract representations. By incorporating mechanisms such as attention and memory, these models can focus on relevant aspects of a scene and retain important information over time, much like humans do when observing their surroundings.
In addition to enhancing the predictive capabilities of AI systems, this interdisciplinary approach also holds promise for improving their generalization abilities. Human intuition is characterized by its flexibility and adaptability, enabling individuals to apply their understanding of physics to novel situations. By emulating the learning processes observed in children, deep learning models can potentially achieve a similar level of generalization, allowing them to perform well in unfamiliar environments and tasks.
Despite these advancements, challenges remain in fully replicating human-like intuition in AI systems. The complexity of the physical world and the vast array of variables involved in any given interaction pose significant hurdles for model development. However, ongoing research continues to refine these models, drawing on the latest findings from both AI and psychology to bridge the gap between human and machine understanding.
In conclusion, the integration of developmental psychology insights into deep learning models represents a promising avenue for emulating human intuition in AI systems. By leveraging the natural learning processes observed in infants and children, researchers are developing models that can intuitively understand and predict physical interactions. This interdisciplinary approach not only enhances the capabilities of AI systems but also brings us closer to creating machines that can seamlessly interact with the world in a human-like manner. As research progresses, the potential applications of these intuitive AI systems are vast, ranging from robotics to autonomous vehicles, and hold the promise of transforming how machines perceive and engage with their environments.
The Intersection of AI and Psychology: Insights into Intuitive Physics Modeling
In recent years, the intersection of artificial intelligence and psychology has opened new avenues for understanding and modeling human cognition. One particularly intriguing area of study is the emulation of intuitive physics by deep learning models, inspired by insights from developmental psychology. Intuitive physics refers to the innate ability of humans, even from a very young age, to understand and predict the behavior of physical objects in the world. This capability, which includes predicting trajectories, understanding object permanence, and recognizing causal relationships, is fundamental to human interaction with the environment. By leveraging insights from developmental psychology, researchers aim to enhance the ability of AI systems to mimic this human-like understanding of physical phenomena.
Developmental psychology provides a rich framework for understanding how humans acquire intuitive physics. Studies have shown that infants possess a rudimentary understanding of physical principles, which becomes more sophisticated as they grow. This developmental trajectory suggests that intuitive physics is not solely a product of learning from the environment but also involves innate cognitive structures. By examining these developmental processes, researchers can identify key principles and mechanisms that can be translated into computational models. This approach not only aids in the creation of more robust AI systems but also offers a deeper understanding of human cognition.
Deep learning models, particularly those employing neural networks, have shown promise in emulating aspects of intuitive physics. These models can be trained on large datasets to recognize patterns and make predictions about physical interactions. However, traditional deep learning approaches often require vast amounts of data and computational resources, and they may struggle to generalize beyond the specific scenarios they have been trained on. By incorporating insights from developmental psychology, researchers aim to overcome these limitations. For instance, by mimicking the way infants learn about the world through exploration and interaction, AI models can be designed to learn more efficiently and generalize better to new situations.
One promising approach involves the use of reinforcement learning, where models learn by interacting with their environment and receiving feedback. This method mirrors the trial-and-error learning process observed in young children. By integrating reinforcement learning with principles derived from developmental psychology, AI systems can develop a more nuanced understanding of physical interactions. Additionally, incorporating concepts such as object permanence and causality into the training process can further enhance the model’s ability to predict and reason about physical events.
The implications of successfully modeling intuitive physics in AI systems are far-reaching. In robotics, for example, machines equipped with an understanding of intuitive physics could navigate and manipulate their environment more effectively, leading to advancements in automation and human-robot interaction. In virtual reality and gaming, more realistic simulations of physical interactions could be achieved, enhancing user experience. Furthermore, these advancements could contribute to the development of AI systems capable of more sophisticated reasoning and problem-solving, with applications in fields ranging from autonomous vehicles to scientific research.
In conclusion, the integration of insights from developmental psychology into the design of deep learning models represents a promising frontier in the quest to emulate human-like intuitive physics. By bridging the gap between AI and psychology, researchers are not only advancing the capabilities of artificial systems but also gaining valuable insights into the nature of human cognition. As this interdisciplinary field continues to evolve, it holds the potential to transform our understanding of both artificial and human intelligence.
From Infants to AI: How Developmental Psychology Shapes Deep Learning Approaches
In recent years, the intersection of developmental psychology and artificial intelligence has opened new avenues for understanding how machines can emulate human-like cognitive processes. One of the most intriguing areas of this interdisciplinary research is the application of insights from developmental psychology to enhance deep learning models, particularly in the realm of intuitive physics. Intuitive physics refers to the innate human ability to understand and predict the physical properties and behaviors of objects in the environment, a skill that even infants display at a remarkably early age. By examining how infants develop this understanding, researchers are gaining valuable insights into how to design AI systems that can similarly grasp and predict physical interactions in the world.
The foundation of this approach lies in the observation that infants learn about the world through a combination of sensory experiences and innate cognitive frameworks. From a young age, they are able to recognize that objects are solid, cannot pass through each other, and will fall if unsupported. These basic principles of physics are not taught explicitly but are instead acquired through interaction with the environment. This developmental process has inspired AI researchers to create models that learn in a similar fashion, using data-driven approaches to develop an understanding of physical laws without explicit programming.
One of the key strategies in this endeavor is the use of deep learning architectures that mimic the hierarchical learning processes observed in human cognition. Convolutional neural networks (CNNs), for instance, have been employed to process visual information in a manner akin to the human visual cortex. By training these networks on large datasets of videos depicting physical interactions, researchers aim to enable AI systems to predict outcomes based on learned patterns. This method mirrors how infants learn to anticipate the consequences of actions, such as predicting the trajectory of a rolling ball or understanding that a stack of blocks will topple if not balanced.
Moreover, the integration of reinforcement learning techniques has further enhanced the ability of AI models to emulate intuitive physics. Reinforcement learning allows models to learn through trial and error, much like infants experimenting with their surroundings. By receiving feedback on their predictions, these models can iteratively refine their understanding of physical principles. This approach not only improves the accuracy of predictions but also fosters a more flexible and adaptive learning process, akin to the way humans adjust their understanding based on new experiences.
In addition to these technical advancements, the collaboration between developmental psychologists and AI researchers has led to a deeper appreciation of the role of curiosity and exploration in learning. Infants are naturally curious, driven to explore their environment and test their hypotheses about how the world works. By incorporating mechanisms that simulate curiosity-driven exploration, AI models can be encouraged to seek out new information and refine their understanding of physical interactions. This not only accelerates the learning process but also enhances the model’s ability to generalize from limited data, a crucial capability for real-world applications.
In conclusion, the fusion of developmental psychology insights with deep learning techniques is paving the way for AI systems that can emulate human-like intuitive physics. By drawing parallels between infant learning and machine learning, researchers are developing models that not only predict physical interactions with greater accuracy but also exhibit a more human-like adaptability and curiosity. As this interdisciplinary field continues to evolve, it holds the promise of creating AI systems that better understand and interact with the world, ultimately bridging the gap between human cognition and artificial intelligence.
Intuitive Physics in AI: Leveraging Developmental Psychology for Advanced Modeling
In recent years, the field of artificial intelligence has witnessed remarkable advancements, particularly in the realm of deep learning. One intriguing area of exploration is the emulation of intuitive physics, a concept that refers to the innate human ability to understand and predict physical interactions in the world. This capability, often taken for granted, is a fundamental aspect of human cognition, allowing individuals to navigate their environment effectively. By leveraging insights from developmental psychology, researchers are now making strides in developing AI models that can mimic this intuitive understanding of physics.
Developmental psychology provides a rich framework for understanding how humans acquire intuitive physics. From a young age, children demonstrate an impressive ability to grasp basic physical principles, such as object permanence, gravity, and causality. These cognitive skills develop through interaction with the environment, suggesting that learning through experience is crucial. By studying these developmental processes, AI researchers can gain valuable insights into how to structure deep learning models that emulate similar capabilities.
One approach to integrating developmental psychology insights into AI is through the use of neural networks that mimic the learning processes observed in children. These networks are designed to learn from vast amounts of data, gradually refining their understanding of physical interactions. By incorporating principles such as reinforcement learning and unsupervised learning, these models can develop a more nuanced understanding of the physical world. This approach not only enhances the model’s predictive capabilities but also allows it to generalize its knowledge to novel situations, much like a human would.
Moreover, the incorporation of developmental psychology into AI models offers the potential for more robust and adaptable systems. Traditional AI models often rely on predefined rules and algorithms, which can limit their flexibility in dynamic environments. In contrast, models inspired by human cognitive development can adapt to new information and adjust their understanding accordingly. This adaptability is particularly valuable in applications such as robotics, where machines must interact with unpredictable and ever-changing environments.
Furthermore, the integration of intuitive physics into AI has significant implications for human-computer interaction. As AI systems become more adept at understanding and predicting physical interactions, they can provide more intuitive and seamless user experiences. For instance, virtual assistants and augmented reality applications can leverage these capabilities to offer more natural and context-aware interactions, enhancing user satisfaction and engagement.
However, despite these promising developments, challenges remain in fully replicating human-like intuitive physics in AI. The complexity of human cognition, shaped by millions of years of evolution, presents a formidable challenge for researchers. Additionally, ethical considerations must be addressed, particularly in ensuring that AI systems do not perpetuate biases or make erroneous predictions that could have real-world consequences.
In conclusion, the emulation of intuitive physics in AI through insights from developmental psychology represents a significant step forward in the quest for more advanced and human-like artificial intelligence. By drawing inspiration from the way humans learn and understand the physical world, researchers are developing models that are not only more capable but also more adaptable and intuitive. As this field continues to evolve, it holds the promise of transforming how AI systems interact with the world and with us, paving the way for a future where machines can seamlessly integrate into our daily lives.
Q&A
1. **What is the main focus of the research on deep learning models emulating intuitive physics?**
The research focuses on developing deep learning models that can mimic human-like intuitive physics by incorporating insights from developmental psychology, aiming to understand and predict physical interactions in the environment as humans do.
2. **How do developmental psychology insights contribute to these models?**
Developmental psychology provides insights into how humans, especially infants, develop an understanding of physical properties and interactions, which can be used to inform the design and training of deep learning models to improve their intuitive physics capabilities.
3. **What are some key features of intuitive physics that these models aim to replicate?**
Key features include understanding object permanence, causality, and the ability to predict the outcomes of physical interactions, such as collisions or gravitational effects, in a manner similar to human intuition.
4. **Why is emulating intuitive physics important for AI development?**
Emulating intuitive physics is crucial for creating AI systems that can interact with the physical world more naturally and effectively, improving their ability to perform tasks in dynamic and unpredictable environments.
5. **What challenges do researchers face in developing these models?**
Challenges include capturing the complexity and variability of real-world physics, ensuring models generalize well across different scenarios, and integrating the nuanced understanding that humans naturally develop over time.
6. **What potential applications could benefit from these advancements in intuitive physics modeling?**
Potential applications include robotics, where understanding and predicting physical interactions is essential, as well as virtual reality, gaming, and any domain requiring realistic simulation of physical environments.The integration of developmental psychology insights into deep learning models to emulate intuitive physics represents a significant advancement in artificial intelligence. By mimicking the cognitive development processes observed in humans, these models can better understand and predict physical interactions in the environment. This approach not only enhances the model’s ability to generalize from limited data but also aligns its learning trajectory with human-like reasoning patterns. Consequently, this fusion of psychology and AI fosters the creation of more robust, adaptable, and efficient systems capable of performing complex tasks with a level of intuition akin to human understanding.
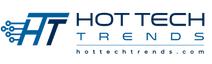