At the International Conference on Machine Learning (ICML) 2023, Google DeepMind showcased a series of groundbreaking advancements that underscored its leadership in artificial intelligence research. These breakthroughs spanned various domains, including reinforcement learning, natural language processing, and neural network optimization, highlighting DeepMind’s commitment to pushing the boundaries of what is possible with AI. Among the notable achievements were innovative approaches to improving model efficiency and interpretability, as well as novel algorithms that enhance the ability of AI systems to learn and adapt in complex environments. These contributions not only demonstrated significant theoretical advancements but also offered practical applications that could transform industries and improve everyday technologies. DeepMind’s presentations at ICML 2023 reinforced its role as a pioneer in the AI field, driving forward the capabilities and understanding of machine learning systems.
Advancements In Reinforcement Learning Techniques
At the International Conference on Machine Learning (ICML) 2023, Google DeepMind unveiled a series of groundbreaking advancements in reinforcement learning techniques, marking a significant leap forward in the field of artificial intelligence. These developments not only highlight the innovative spirit of DeepMind but also underscore the potential of reinforcement learning to solve complex real-world problems. As the conference unfolded, it became evident that DeepMind’s contributions were poised to redefine the boundaries of what reinforcement learning can achieve.
One of the most notable breakthroughs presented by DeepMind was the introduction of a novel algorithm that significantly enhances the efficiency and scalability of reinforcement learning models. This algorithm, which leverages a more sophisticated approach to exploration and exploitation, allows models to learn optimal strategies with fewer resources and in less time. By refining the balance between exploring new strategies and exploiting known ones, DeepMind’s algorithm addresses a long-standing challenge in reinforcement learning, thereby enabling more robust and adaptable AI systems.
In addition to algorithmic improvements, DeepMind showcased advancements in the application of reinforcement learning to multi-agent systems. These systems, which involve multiple AI agents interacting within a shared environment, have traditionally been fraught with complexity due to the dynamic and often unpredictable nature of agent interactions. However, DeepMind’s latest research introduces a framework that facilitates more effective communication and cooperation among agents. This framework not only improves the overall performance of multi-agent systems but also opens new avenues for their application in areas such as autonomous vehicles and collaborative robotics.
Furthermore, DeepMind’s presentations at ICML 2023 highlighted the integration of reinforcement learning with other machine learning paradigms, such as supervised and unsupervised learning. By combining these approaches, DeepMind has developed hybrid models that leverage the strengths of each paradigm, resulting in more versatile and powerful AI systems. This integration allows for the creation of models that can learn from both labeled and unlabeled data, thereby expanding the scope of problems that reinforcement learning can address.
Another significant aspect of DeepMind’s contributions is the emphasis on ethical considerations and the responsible deployment of reinforcement learning technologies. Recognizing the potential societal impact of AI, DeepMind has been proactive in developing guidelines and frameworks to ensure that their technologies are used ethically and responsibly. This commitment to ethical AI was evident in their discussions at ICML 2023, where they emphasized the importance of transparency, fairness, and accountability in the development and deployment of reinforcement learning systems.
Moreover, DeepMind’s advancements in reinforcement learning have implications beyond the realm of technology, extending into fields such as healthcare, finance, and environmental science. By applying their cutting-edge techniques to these domains, DeepMind is paving the way for AI-driven solutions that can address some of the most pressing challenges facing society today. For instance, in healthcare, reinforcement learning models are being used to optimize treatment plans and improve patient outcomes, while in finance, they are enhancing risk management and decision-making processes.
In conclusion, the breakthroughs from Google DeepMind at ICML 2023 represent a significant milestone in the evolution of reinforcement learning techniques. Through innovative algorithms, multi-agent system advancements, integration with other learning paradigms, and a strong focus on ethical considerations, DeepMind is not only pushing the boundaries of AI research but also setting a precedent for the responsible and impactful application of these technologies. As these advancements continue to unfold, they hold the promise of transforming industries and improving lives, underscoring the transformative potential of reinforcement learning in the modern world.
Novel Approaches To Natural Language Processing
At the International Conference on Machine Learning (ICML) 2023, Google DeepMind unveiled a series of groundbreaking advancements in the field of natural language processing (NLP), marking a significant leap forward in the capabilities of artificial intelligence. These novel approaches not only enhance the understanding and generation of human language by machines but also pave the way for more sophisticated applications across various domains. Central to these advancements is the development of more efficient algorithms that improve the accuracy and speed of language models, addressing some of the longstanding challenges in NLP.
One of the key innovations presented by DeepMind is a new architecture that significantly reduces the computational resources required for training large language models. This architecture, which leverages a more efficient use of attention mechanisms, allows for the processing of longer sequences of text without a proportional increase in computational cost. By optimizing the way models attend to different parts of the input data, DeepMind has managed to enhance the scalability of NLP systems, making them more accessible for a wider range of applications. This breakthrough is particularly important as it addresses the growing demand for models that can handle increasingly complex language tasks without necessitating prohibitive computational power.
In addition to architectural improvements, DeepMind introduced a novel training paradigm that emphasizes the importance of context in language understanding. This approach involves a more nuanced method of pre-training models on diverse datasets that better capture the intricacies of human language. By incorporating a broader spectrum of linguistic contexts, these models demonstrate a marked improvement in their ability to comprehend and generate text that is contextually relevant and coherent. This advancement is crucial for applications such as machine translation, sentiment analysis, and conversational agents, where understanding the subtleties of language is paramount.
Furthermore, DeepMind’s research at ICML 2023 highlighted the integration of reinforcement learning techniques into NLP models. By employing reinforcement learning, these models can be fine-tuned to optimize specific language tasks, thereby improving their performance in real-world applications. This approach allows models to learn from feedback and adapt their strategies accordingly, leading to more robust and versatile language systems. The incorporation of reinforcement learning not only enhances the adaptability of NLP models but also opens up new possibilities for interactive AI systems that can learn and evolve over time.
Another significant contribution from DeepMind is the development of models that exhibit a deeper understanding of semantics and pragmatics in language. By focusing on these aspects, the models are better equipped to grasp the intended meaning behind words and phrases, rather than merely processing them at a syntactic level. This advancement is particularly beneficial for applications that require a high degree of language comprehension, such as legal document analysis and automated content generation. By improving the semantic and pragmatic understanding of language, DeepMind’s models are poised to deliver more accurate and meaningful outputs.
In conclusion, the breakthroughs presented by Google DeepMind at ICML 2023 represent a substantial advancement in the field of natural language processing. Through innovative architectures, enhanced training paradigms, the integration of reinforcement learning, and a focus on semantics and pragmatics, DeepMind has set a new benchmark for what is possible in NLP. These developments not only improve the efficiency and effectiveness of language models but also expand the potential applications of AI in understanding and generating human language. As these technologies continue to evolve, they promise to transform the way we interact with machines, making them more intuitive and capable partners in a wide array of tasks.
Innovations In Quantum Computing Algorithms
At the International Conference on Machine Learning (ICML) 2023, Google DeepMind unveiled a series of groundbreaking innovations in the realm of quantum computing algorithms, marking a significant leap forward in the field. These advancements are poised to redefine the landscape of computational capabilities, offering unprecedented opportunities for solving complex problems that were previously deemed intractable. As quantum computing continues to evolve, the contributions from Google DeepMind are particularly noteworthy, given their potential to accelerate the development of more efficient and powerful algorithms.
One of the most significant breakthroughs presented by Google DeepMind at ICML 2023 is the development of a novel quantum algorithm that dramatically enhances the speed and accuracy of quantum computations. This algorithm leverages the principles of quantum superposition and entanglement to perform calculations at a scale and speed that classical computers cannot match. By optimizing the way quantum bits, or qubits, interact and process information, this innovation promises to reduce computational errors and improve the reliability of quantum systems. Consequently, this advancement could pave the way for more practical applications of quantum computing in various fields, including cryptography, materials science, and complex system simulations.
In addition to improving computational efficiency, Google DeepMind’s research also addresses one of the most pressing challenges in quantum computing: error correction. Quantum systems are notoriously susceptible to errors due to environmental interference and the inherent instability of qubits. To tackle this issue, DeepMind has introduced a sophisticated error-correction algorithm that utilizes machine learning techniques to predict and mitigate errors in real-time. This approach not only enhances the stability of quantum computations but also extends the coherence time of qubits, thereby allowing for longer and more complex calculations. The integration of machine learning with quantum error correction represents a significant step forward in making quantum computing more robust and reliable.
Furthermore, Google DeepMind’s innovations extend to the realm of quantum machine learning, where they have developed algorithms that can process and analyze vast amounts of data more efficiently than ever before. By harnessing the power of quantum computing, these algorithms can identify patterns and correlations in data sets that are too complex for classical algorithms to discern. This capability holds immense potential for advancing fields such as drug discovery, financial modeling, and artificial intelligence, where the ability to process and interpret large data sets is crucial.
Moreover, the implications of these advancements are not limited to theoretical research; they also have practical applications that could transform industries. For instance, in the field of cryptography, the enhanced computational power of quantum algorithms could lead to the development of more secure encryption methods, safeguarding sensitive information against increasingly sophisticated cyber threats. Similarly, in materials science, the ability to simulate molecular interactions at a quantum level could accelerate the discovery of new materials with unique properties, driving innovation in sectors ranging from electronics to renewable energy.
In conclusion, the breakthroughs from Google DeepMind at ICML 2023 represent a pivotal moment in the evolution of quantum computing algorithms. By addressing key challenges such as computational efficiency and error correction, and by exploring new frontiers in quantum machine learning, these innovations have the potential to unlock new possibilities across a wide range of disciplines. As the field of quantum computing continues to advance, the contributions from Google DeepMind will undoubtedly play a crucial role in shaping the future of technology and its applications.
Breakthroughs In AI Safety And Ethics
At the International Conference on Machine Learning (ICML) 2023, Google DeepMind unveiled a series of groundbreaking advancements in the realm of AI safety and ethics, marking a significant stride in addressing the complex challenges associated with artificial intelligence. As AI systems become increasingly integrated into various aspects of society, ensuring their safe and ethical deployment has become a paramount concern. DeepMind’s latest contributions underscore the importance of developing robust frameworks that prioritize these aspects, thereby fostering trust and reliability in AI technologies.
One of the most notable breakthroughs presented by DeepMind at the conference was the development of advanced algorithms designed to enhance the interpretability of AI models. These algorithms aim to provide clearer insights into the decision-making processes of complex AI systems, thereby addressing one of the longstanding challenges in AI safety. By making AI systems more transparent, these algorithms help mitigate risks associated with opaque decision-making, which can lead to unintended consequences. This advancement not only aids researchers and developers in understanding AI behavior but also empowers stakeholders to make informed decisions regarding AI deployment.
In addition to interpretability, DeepMind has made significant progress in the area of AI alignment, which involves ensuring that AI systems’ goals and actions are in harmony with human values and intentions. At ICML 2023, DeepMind introduced novel techniques that leverage reinforcement learning to align AI behavior with ethical guidelines. These techniques are designed to minimize the risk of AI systems acting in ways that are misaligned with human interests, thereby enhancing their safety and reliability. By focusing on alignment, DeepMind is addressing a critical aspect of AI ethics, ensuring that AI systems contribute positively to society.
Furthermore, DeepMind’s research at ICML 2023 highlighted the importance of fairness in AI systems. Recognizing that biased AI models can perpetuate and even exacerbate societal inequalities, DeepMind has developed innovative methods to detect and mitigate bias in AI algorithms. These methods involve rigorous testing and validation processes that identify potential sources of bias and implement corrective measures. By prioritizing fairness, DeepMind is taking proactive steps to ensure that AI technologies are equitable and just, thereby promoting their ethical use across diverse applications.
Another significant contribution from DeepMind at the conference was the emphasis on collaborative approaches to AI safety and ethics. DeepMind advocates for a multidisciplinary approach, bringing together experts from fields such as computer science, ethics, law, and social sciences to address the multifaceted challenges of AI safety. This collaborative effort is crucial in developing comprehensive solutions that consider the diverse perspectives and implications of AI technologies. By fostering collaboration, DeepMind is paving the way for more holistic and inclusive approaches to AI safety and ethics.
In conclusion, the breakthroughs presented by Google DeepMind at ICML 2023 represent a significant leap forward in the pursuit of safe and ethical AI systems. Through advancements in interpretability, alignment, fairness, and collaboration, DeepMind is addressing some of the most pressing challenges in the field. As AI continues to evolve and permeate various sectors, these contributions are instrumental in ensuring that AI technologies are developed and deployed in ways that are beneficial, equitable, and aligned with human values. The work of DeepMind serves as a beacon for the AI community, guiding future research and development efforts towards a more responsible and ethical AI landscape.
Cutting-edge Developments In Machine Learning Interpretability
At the International Conference on Machine Learning (ICML) 2023, Google DeepMind unveiled a series of groundbreaking advancements in the realm of machine learning interpretability, a field that has long grappled with the challenge of demystifying the decision-making processes of complex models. As machine learning systems become increasingly integral to various sectors, from healthcare to finance, the need for transparency and understanding of these models’ inner workings has never been more critical. DeepMind’s latest contributions promise to enhance our ability to interpret and trust these sophisticated systems.
One of the most notable breakthroughs presented by DeepMind is a novel framework that significantly improves the interpretability of deep neural networks. This framework leverages a combination of feature attribution methods and visualization techniques to provide a more comprehensive understanding of how models arrive at specific decisions. By offering a clearer picture of which features are most influential in a model’s predictions, this approach not only aids researchers and practitioners in debugging and refining models but also helps in building trust with end-users who rely on these systems for critical decision-making.
In addition to feature attribution, DeepMind has also introduced innovative methods for understanding the dynamics of model training. Traditionally, the training process of deep learning models has been viewed as a black box, with limited insights into how models evolve over time. DeepMind’s new techniques employ advanced statistical tools to track and visualize the learning trajectory of models, shedding light on how they adapt and optimize during training. This enhanced visibility into the training process allows for more informed adjustments and improvements, ultimately leading to more robust and reliable models.
Furthermore, DeepMind has made strides in the interpretability of reinforcement learning systems, which are particularly challenging due to their complex decision-making environments. By developing new algorithms that can decompose and analyze the decision policies of reinforcement learning agents, DeepMind has provided a means to better understand the rationale behind their actions. This advancement is crucial for applications where safety and accountability are paramount, such as autonomous vehicles and robotic systems.
Transitioning from technical innovations to practical applications, DeepMind’s work at ICML 2023 also highlighted the importance of interpretability in real-world scenarios. For instance, in the healthcare sector, where machine learning models are increasingly used for diagnostic and prognostic purposes, the ability to interpret model outputs can significantly impact patient outcomes. DeepMind’s advancements offer healthcare professionals the tools to better understand model predictions, thereby facilitating more informed clinical decisions and improving patient trust in AI-driven healthcare solutions.
Moreover, DeepMind’s contributions extend beyond individual models to encompass broader system-level interpretability. By developing frameworks that allow for the analysis of interactions between multiple models within a system, DeepMind is paving the way for more transparent and accountable AI ecosystems. This holistic approach to interpretability is essential as AI systems become more interconnected and complex, ensuring that stakeholders can maintain oversight and control over these powerful technologies.
In conclusion, the breakthroughs from Google DeepMind at ICML 2023 represent a significant leap forward in the field of machine learning interpretability. By enhancing our ability to understand and trust complex models, these advancements not only address longstanding challenges but also open new avenues for the responsible deployment of AI technologies across various domains. As the field continues to evolve, the insights and innovations presented by DeepMind will undoubtedly serve as a foundation for future research and development in machine learning interpretability.
Pioneering Research In Neural Network Architectures
At the International Conference on Machine Learning (ICML) 2023, Google DeepMind unveiled a series of groundbreaking advancements in neural network architectures, marking a significant leap forward in the field of artificial intelligence. These innovations not only demonstrate the potential for more efficient and powerful AI systems but also pave the way for new applications across various domains. The research presented by DeepMind at the conference highlighted several key areas of development, each contributing to the overarching goal of enhancing the capabilities and understanding of neural networks.
One of the most notable breakthroughs was the introduction of a novel architecture that significantly improves the efficiency of training deep neural networks. This new design, which DeepMind researchers have termed “SparseNet,” leverages sparsity in neural connections to reduce computational overhead without sacrificing performance. By selectively activating only a subset of neurons during training, SparseNet achieves faster convergence rates and requires less computational power, making it particularly advantageous for large-scale models. This innovation addresses one of the longstanding challenges in AI research: the trade-off between model complexity and computational efficiency.
In addition to SparseNet, DeepMind also showcased advancements in the interpretability of neural networks. Understanding how these complex models arrive at their decisions has been a persistent challenge, often described as the “black box” problem. To tackle this, DeepMind introduced a new framework called “Explainable Neural Networks” (XNNs), which incorporates interpretability directly into the model architecture. XNNs provide insights into the decision-making process by highlighting the most influential features and pathways within the network. This development not only enhances trust in AI systems but also facilitates their deployment in critical areas such as healthcare and autonomous driving, where understanding the rationale behind decisions is paramount.
Furthermore, DeepMind’s research at ICML 2023 explored the integration of reinforcement learning with neural network architectures. By combining these two powerful approaches, the researchers developed a hybrid model that excels in dynamic and uncertain environments. This model, referred to as “Adaptive Neural Reinforcement” (ANR), dynamically adjusts its learning strategy based on real-time feedback, allowing it to perform optimally in a wide range of scenarios. The implications of ANR are vast, with potential applications in robotics, game playing, and real-time decision-making systems.
Another significant contribution from DeepMind was in the realm of unsupervised learning. The team introduced a new method for training neural networks without labeled data, a technique known as “Self-Supervised Neural Networks” (SSNNs). This approach enables models to learn from vast amounts of unstructured data by identifying patterns and relationships within the data itself. SSNNs have the potential to revolutionize fields where labeled data is scarce or expensive to obtain, such as natural language processing and computer vision.
In conclusion, the pioneering research presented by Google DeepMind at ICML 2023 represents a substantial advancement in neural network architectures. Through innovations such as SparseNet, Explainable Neural Networks, Adaptive Neural Reinforcement, and Self-Supervised Neural Networks, DeepMind is pushing the boundaries of what is possible with AI. These developments not only enhance the efficiency, interpretability, and adaptability of neural networks but also open new avenues for their application across diverse fields. As these technologies continue to evolve, they hold the promise of transforming industries and improving the quality of life on a global scale.
Q&A
1. **Question:** What was a significant breakthrough presented by Google DeepMind at ICML 2023 related to reinforcement learning?
**Answer:** Google DeepMind introduced a novel reinforcement learning algorithm that significantly improves sample efficiency and stability in training, leveraging advanced exploration strategies.
2. **Question:** How did Google DeepMind address the challenge of interpretability in machine learning models at ICML 2023?
**Answer:** They presented a new framework for model interpretability that uses counterfactual explanations, allowing users to understand model decisions by exploring hypothetical scenarios.
3. **Question:** What advancement did Google DeepMind showcase in the field of unsupervised learning at ICML 2023?
**Answer:** DeepMind unveiled a cutting-edge unsupervised learning technique that enhances the ability of models to learn from unlabelled data by utilizing a self-supervised approach with contrastive learning.
4. **Question:** What was Google DeepMind’s contribution to the optimization of neural networks discussed at ICML 2023?
**Answer:** They introduced an innovative optimization algorithm that accelerates convergence rates in training deep neural networks, reducing computational costs and improving scalability.
5. **Question:** How did Google DeepMind tackle the issue of fairness in AI systems at ICML 2023?
**Answer:** DeepMind proposed a new fairness-aware training method that ensures equitable outcomes across different demographic groups by incorporating fairness constraints directly into the learning process.
6. **Question:** What was a key development from Google DeepMind in the area of AI safety presented at ICML 2023?
**Answer:** They showcased a robust AI safety mechanism that enhances the reliability of AI systems by integrating adversarial training techniques to defend against potential security threats and biases.At ICML 2023, Google DeepMind showcased several groundbreaking advancements in machine learning and artificial intelligence. Key breakthroughs included novel architectures for deep learning models that significantly improved efficiency and performance across various tasks. They introduced innovative techniques for reinforcement learning, enhancing the ability of AI systems to learn from complex environments with minimal supervision. Additionally, DeepMind presented advancements in interpretability and fairness, addressing critical challenges in AI ethics and transparency. These contributions not only pushed the boundaries of current AI capabilities but also set new directions for future research in the field. Overall, DeepMind’s work at ICML 2023 underscored its leadership in driving forward the development of more powerful, efficient, and ethical AI technologies.
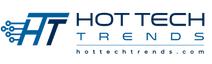