Large Language Models (LLMs) have revolutionized the field of artificial intelligence, offering unprecedented capabilities in natural language processing and understanding. However, despite their impressive performance, these models often fall short of mimicking human behavior as expected. This discrepancy arises from several fundamental differences between how humans and LLMs process information. While humans rely on a complex interplay of cognitive processes, emotions, and contextual understanding, LLMs operate based on statistical patterns learned from vast datasets. They lack genuine comprehension, emotional intelligence, and the ability to infer meaning beyond the data they have been trained on. Additionally, LLMs do not possess consciousness or self-awareness, which are integral to human behavior. These limitations highlight the challenges in bridging the gap between artificial and human intelligence, underscoring the need for continued research and development to enhance the alignment of LLMs with human-like understanding and interaction.
Complexity Of Human Emotions
Large language models, such as those developed in recent years, have demonstrated remarkable capabilities in processing and generating human-like text. However, despite their impressive performance, these models often fall short of mimicking human behavior, particularly when it comes to the complexity of human emotions. Understanding why these models struggle in this area requires an exploration of both the intricacies of human emotions and the limitations inherent in artificial intelligence.
To begin with, human emotions are deeply complex and multifaceted, influenced by a myriad of factors including personal experiences, cultural background, and social context. Emotions are not merely responses to stimuli but are shaped by an individual’s history and environment, making them highly subjective and variable. This complexity poses a significant challenge for language models, which primarily rely on patterns in data to generate responses. While these models can identify and replicate patterns in language, they lack the ability to truly understand the underlying emotional nuances that inform human communication.
Moreover, emotions are often expressed through subtle cues such as tone, body language, and facial expressions, which are beyond the scope of text-based models. Even within text, the same words can convey different emotions depending on context, irony, or sarcasm. For instance, the phrase “I’m fine” can indicate genuine contentment or masked distress, depending on the situation. Language models, which do not possess the ability to perceive or interpret these non-verbal cues, often misinterpret or overlook the emotional depth of such expressions.
In addition to these challenges, the training data used for language models can also contribute to their limitations in understanding emotions. These models are trained on vast datasets sourced from the internet, which may not always accurately represent the full spectrum of human emotions. The data can be biased, incomplete, or lacking in diversity, leading to models that are ill-equipped to handle the rich tapestry of human emotional expression. Furthermore, the data-driven nature of these models means they are more adept at recognizing frequently occurring patterns rather than rare or unique emotional expressions.
Another factor to consider is the lack of genuine empathy in language models. While they can simulate empathetic responses by generating text that appears compassionate or understanding, they do not possess the intrinsic ability to feel or comprehend emotions. This absence of true empathy limits their capacity to engage with human emotions on a deeper level, often resulting in interactions that feel mechanical or insincere.
Despite these limitations, it is important to acknowledge the progress that has been made in the field of artificial intelligence. Researchers are continually working to improve the emotional intelligence of language models, exploring techniques such as sentiment analysis and emotion recognition to enhance their capabilities. However, achieving a level of emotional understanding comparable to that of humans remains a formidable challenge.
In conclusion, while large language models have made significant strides in natural language processing, they do not yet mimic human behavior as expected, particularly in the realm of emotions. The complexity of human emotions, coupled with the limitations of current AI technology, presents significant hurdles that have yet to be fully overcome. As research continues, it is crucial to remain mindful of these challenges and to approach the development of emotionally intelligent AI with both optimism and caution.
Contextual Understanding Limitations
Large language models, such as those developed in recent years, have demonstrated remarkable capabilities in generating human-like text, translating languages, and even engaging in complex conversations. However, despite these impressive feats, they often fall short of mimicking human behavior as expected, particularly in the realm of contextual understanding. This limitation arises from several inherent characteristics of these models, which, while sophisticated, do not fully replicate the nuanced and dynamic nature of human cognition.
To begin with, large language models are fundamentally statistical in nature. They rely on vast datasets to predict the likelihood of word sequences, thereby generating responses that appear coherent and contextually relevant. However, this statistical approach lacks the depth of understanding that humans naturally possess. Humans interpret language through a rich tapestry of experiences, emotions, and cultural contexts, which allows them to grasp subtleties and implied meanings that a language model might miss. For instance, when faced with idiomatic expressions or sarcasm, a human can draw upon personal experiences and social cues to interpret the intended meaning, whereas a language model might misinterpret or take the expression literally.
Moreover, the training data used for these models, while extensive, is inherently limited by the scope and diversity of the information it contains. Language models are trained on text from the internet, books, and other written sources, which, although vast, cannot encompass the full spectrum of human experiences and cultural nuances. Consequently, these models may struggle with context-specific understanding, particularly when dealing with niche topics or culturally specific references that are underrepresented in their training data. This limitation can lead to responses that are either overly generic or contextually inappropriate, highlighting the gap between statistical prediction and genuine understanding.
Another critical factor contributing to the contextual understanding limitations of language models is their lack of real-world grounding. Humans learn language in conjunction with sensory experiences and interactions with the physical world, which provide a rich context for understanding and using language effectively. In contrast, language models operate solely within the realm of text, devoid of sensory input or experiential learning. This absence of real-world grounding means that while a model can generate text that appears contextually appropriate, it does not truly comprehend the underlying concepts or the real-world implications of its responses.
Furthermore, the static nature of language model training presents another challenge. Once trained, these models do not dynamically update their knowledge base in response to new information or changing contexts, unlike humans who continuously learn and adapt. This static nature can result in outdated or contextually irrelevant responses, particularly in rapidly evolving fields or situations where current events play a significant role.
In conclusion, while large language models have made significant strides in natural language processing, their limitations in contextual understanding highlight the complexities of human cognition that remain beyond their reach. The statistical nature of these models, coupled with the constraints of their training data and lack of real-world grounding, contribute to their inability to fully mimic human behavior. As research in artificial intelligence continues to advance, addressing these limitations will be crucial in developing models that can more accurately and effectively understand and respond to the rich and dynamic contexts in which human language operates.
Lack Of Personal Experience
Large language models, such as those developed by leading artificial intelligence research organizations, have made significant strides in processing and generating human-like text. However, despite their impressive capabilities, these models often fall short of mimicking human behavior as expected. One of the primary reasons for this discrepancy is their lack of personal experience, which fundamentally limits their ability to fully understand and replicate the nuances of human interaction.
To begin with, it is essential to recognize that large language models are trained on vast datasets comprising text from diverse sources. These datasets provide the models with a wealth of information, enabling them to generate coherent and contextually relevant responses. However, this training process is inherently limited to the data available, which is a mere reflection of human language rather than human experience. Consequently, while these models can simulate conversations and produce text that appears human-like, they do not possess the intrinsic understanding that comes from personal experience.
Moreover, personal experience is a cornerstone of human behavior, influencing how individuals perceive the world, make decisions, and interact with others. Humans draw upon their unique experiences to navigate complex social situations, empathize with others, and adapt to new environments. In contrast, language models lack this experiential foundation, relying solely on patterns and associations learned from their training data. This absence of personal experience means that while they can mimic certain aspects of human communication, they often struggle with tasks that require genuine understanding or emotional depth.
Furthermore, the lack of personal experience in language models also affects their ability to exhibit creativity and originality. Human creativity is often driven by personal insights, emotions, and experiences, which inspire novel ideas and solutions. Language models, on the other hand, generate content by recombining existing information in novel ways, without the benefit of personal insight. This limitation can result in outputs that, although technically proficient, may lack the originality and depth that characterize human creativity.
In addition, the absence of personal experience in language models can lead to challenges in ethical decision-making and moral reasoning. Humans rely on their experiences, values, and cultural norms to navigate ethical dilemmas and make moral judgments. Language models, however, do not possess an inherent moral compass or the ability to understand the broader implications of their outputs. This can result in responses that are inappropriate or insensitive, highlighting the importance of human oversight in the deployment of these technologies.
Despite these limitations, it is important to acknowledge the significant advancements that large language models have achieved in natural language processing. They have proven to be valuable tools in various applications, from customer service to content creation, and continue to evolve rapidly. However, their lack of personal experience remains a critical factor that distinguishes them from human behavior.
In conclusion, while large language models have made remarkable progress in simulating human-like text, their lack of personal experience fundamentally limits their ability to fully mimic human behavior. This absence affects their understanding, creativity, and ethical reasoning, underscoring the importance of recognizing these limitations when integrating such models into real-world applications. As research in artificial intelligence continues to advance, addressing these challenges will be crucial in developing models that more closely align with human behavior and understanding.
Absence Of Intuition
Large language models, such as those developed by leading technology companies, have made significant strides in natural language processing and understanding. These models are capable of generating human-like text, translating languages, and even engaging in complex conversations. However, despite their impressive capabilities, they often fall short of mimicking human behavior as expected. One of the primary reasons for this discrepancy is the absence of intuition, a fundamental aspect of human cognition that these models lack.
Intuition, in the human context, refers to the ability to understand or know something immediately, without the need for conscious reasoning. It is a product of our experiences, emotions, and subconscious processing, allowing us to make quick judgments and decisions. This intuitive capability is deeply rooted in the human brain’s complex neural networks, which have evolved over millennia. In contrast, large language models are based on artificial neural networks that, while sophisticated, do not possess the same depth of experiential learning or emotional context.
The absence of intuition in language models can be attributed to their reliance on data-driven learning. These models are trained on vast datasets, absorbing patterns and structures from the text they process. They excel at identifying correlations and generating responses based on statistical probabilities. However, this approach lacks the nuanced understanding that intuition provides. For instance, when faced with ambiguous or context-dependent situations, humans can draw upon their intuition to navigate the uncertainty. Language models, on the other hand, may struggle to produce coherent or contextually appropriate responses, as they lack the intrinsic ability to “feel” or “sense” the subtleties involved.
Moreover, intuition often involves an emotional component, which is inherently absent in machine learning models. Human intuition is frequently informed by emotions, which guide our perceptions and decisions. Emotions can influence how we interpret language, inferring meaning beyond the literal words. Language models, however, process text in a purely logical manner, devoid of emotional influence. This can lead to responses that, while technically correct, may seem detached or inappropriate in emotionally charged situations.
Furthermore, the absence of intuition in language models is evident in their handling of novel or unexpected scenarios. Humans can rely on their intuitive understanding to adapt to new situations, drawing parallels from past experiences to inform their actions. In contrast, language models are limited by their training data and may falter when confronted with unfamiliar contexts. They lack the ability to generalize from limited information in the way humans can, often resulting in responses that are either overly generic or entirely off the mark.
In addition, the absence of intuition affects the models’ ability to grasp cultural nuances and social dynamics. Human intuition is shaped by cultural norms and social interactions, enabling us to navigate complex social landscapes. Language models, however, may not fully comprehend these subtleties, leading to misunderstandings or culturally insensitive outputs. This limitation underscores the importance of incorporating diverse and representative data in training these models, yet it also highlights the inherent challenge of replicating human intuition.
In conclusion, while large language models have achieved remarkable feats in language processing, their inability to mimic human behavior as expected is largely due to the absence of intuition. This fundamental difference underscores the complexity of human cognition and the challenges of replicating it in artificial systems. As research in artificial intelligence continues to advance, addressing the gap between data-driven learning and intuitive understanding remains a critical area of exploration.
Inability To Grasp Nuance
Large language models, such as those developed in recent years, have demonstrated remarkable capabilities in processing and generating human-like text. However, despite their impressive performance, these models often fall short of mimicking human behavior as expected, particularly when it comes to grasping nuance. This limitation can be attributed to several factors inherent in the design and functioning of these models.
To begin with, large language models are fundamentally statistical in nature. They rely on vast datasets to learn patterns and correlations between words and phrases. While this approach allows them to generate coherent and contextually relevant text, it does not equip them with an understanding of the subtleties and complexities that characterize human communication. Humans, on the other hand, interpret language through a rich tapestry of experiences, emotions, and cultural contexts, which are difficult to encapsulate in a purely data-driven model.
Moreover, the training data used for these models often lacks the depth and diversity necessary to capture the full spectrum of human nuance. Language is not just a collection of words and grammar rules; it is a dynamic and evolving entity shaped by social, cultural, and historical influences. Consequently, language models trained on static datasets may miss out on the evolving nature of language and the subtle shifts in meaning that occur over time. This can lead to outputs that are technically correct but lack the depth and insight that a human might provide.
In addition, large language models do not possess the ability to understand context in the same way humans do. While they can process context within a given text, they lack the broader contextual awareness that humans naturally apply when interpreting language. For instance, humans can easily discern sarcasm, irony, or humor based on contextual cues and shared knowledge, whereas language models may struggle to identify these nuances without explicit indicators. This limitation is further compounded by the fact that language models do not have access to non-verbal cues, such as tone of voice or body language, which play a crucial role in human communication.
Furthermore, the inability of language models to grasp nuance is also linked to their lack of true understanding or consciousness. These models do not possess beliefs, desires, or intentions; they merely generate text based on learned patterns. As a result, they may produce responses that are superficially appropriate but lack the depth of understanding that comes from genuine human cognition. This absence of true comprehension means that language models are unable to appreciate the subtleties of meaning that arise from personal experiences or emotional connections.
Despite these challenges, ongoing research and development in the field of artificial intelligence continue to explore ways to enhance the ability of language models to grasp nuance. Efforts to incorporate more diverse and representative datasets, as well as advancements in contextual understanding, hold promise for improving the performance of these models. However, it is important to recognize that while language models can be powerful tools for processing and generating text, they are not a substitute for the rich and multifaceted nature of human communication.
In conclusion, the inability of large language models to fully grasp nuance is a reflection of their inherent limitations as statistical tools. While they excel in certain tasks, their lack of true understanding and contextual awareness means that they cannot yet mimic human behavior as expected. As research progresses, it will be crucial to address these limitations to create models that better align with the complexities of human language and communication.
Static Knowledge Base
Large language models, such as those developed by leading artificial intelligence research organizations, have garnered significant attention for their ability to process and generate human-like text. These models, trained on vast datasets, are often perceived as possessing an understanding akin to human cognition. However, despite their impressive capabilities, they do not mimic human behavior as one might expect. This discrepancy arises from several fundamental differences between how these models operate and how humans think and learn.
To begin with, large language models are essentially static knowledge bases. They are trained on a fixed dataset, which means their knowledge is limited to the information available at the time of training. Unlike humans, who continuously learn and adapt to new information, these models do not have the ability to update their knowledge in real-time. This static nature can lead to outdated or incorrect responses, especially in rapidly changing fields where new developments occur frequently. Consequently, while a language model might generate text that appears knowledgeable, it lacks the dynamic adaptability that characterizes human learning.
Moreover, the way language models process information is fundamentally different from human cognition. Humans rely on a complex interplay of emotions, experiences, and contextual understanding to interpret and generate language. In contrast, language models use statistical patterns derived from their training data to predict the next word in a sequence. This approach, while effective in generating coherent text, lacks the depth of understanding that comes from human experiences and emotions. As a result, language models may produce text that is contextually appropriate but devoid of genuine insight or empathy.
Furthermore, the absence of true comprehension in language models is evident in their inability to grasp nuanced meanings or cultural subtleties. Humans draw upon a rich tapestry of cultural knowledge and personal experiences to understand and convey meaning. Language models, however, are limited to the patterns they have learned from their training data. This limitation can lead to misunderstandings or inappropriate responses, particularly in situations that require cultural sensitivity or emotional intelligence. Thus, while language models can mimic certain aspects of human language, they fall short in replicating the depth and richness of human communication.
In addition to these cognitive differences, ethical considerations also play a role in why language models do not mimic human behavior as expected. Developers of these models must carefully balance the need for powerful language generation with the potential for misuse or harm. This involves implementing safeguards to prevent the generation of harmful or biased content. However, these safeguards can also limit the model’s ability to fully replicate human-like behavior, as they may restrict certain types of responses or interactions.
In conclusion, while large language models represent a significant advancement in artificial intelligence, they do not mimic human behavior as one might expect due to their static knowledge base, lack of true comprehension, and ethical constraints. These models, though capable of generating impressive text, are ultimately limited by their design and training. Understanding these limitations is crucial for effectively utilizing language models and setting realistic expectations for their capabilities. As research in artificial intelligence continues to evolve, it is essential to recognize the distinct differences between machine-generated language and human communication, appreciating each for its unique strengths and limitations.
Q&A
1. **Question:** Why do large language models struggle with understanding context like humans do?
**Answer:** Large language models process text based on patterns in data rather than genuine comprehension, leading to difficulties in grasping nuanced context as humans do.
2. **Question:** How do large language models handle ambiguity compared to humans?
**Answer:** Unlike humans, who use intuition and experience to resolve ambiguity, large language models rely on statistical correlations, which can result in misinterpretations.
3. **Question:** Why do large language models sometimes produce nonsensical or incorrect answers?
**Answer:** These models generate responses based on probability rather than factual accuracy, which can lead to plausible-sounding but incorrect or nonsensical outputs.
4. **Question:** What limits large language models’ ability to mimic human emotional understanding?
**Answer:** Large language models lack genuine emotional intelligence and empathy, as they do not experience emotions and only simulate responses based on learned data patterns.
5. **Question:** How do large language models’ training data affect their behavior?
**Answer:** The behavior of large language models is heavily influenced by the biases and limitations present in their training data, which can lead to unexpected or undesirable outputs.
6. **Question:** Why can’t large language models exhibit true creativity like humans?
**Answer:** Large language models generate content by recombining existing data patterns, lacking the ability to create genuinely novel ideas or concepts as humans do through imagination and inspiration.Large Language Models (LLMs) like GPT-3 and others are designed to process and generate human-like text based on patterns learned from vast datasets. However, they often fail to mimic human behavior as expected due to several reasons. Firstly, LLMs lack genuine understanding and consciousness; they process information statistically rather than contextually or emotionally, which limits their ability to grasp nuanced human experiences. Secondly, these models are trained on data that may contain biases, inaccuracies, or outdated information, leading to outputs that do not always align with current human values or knowledge. Thirdly, LLMs do not possess the ability to learn from real-time interactions or adapt to new information in the way humans do, resulting in static and sometimes inappropriate responses. Lastly, human behavior is influenced by a complex interplay of emotions, social dynamics, and personal experiences, which LLMs cannot replicate due to their fundamentally different nature as algorithmic constructs. Therefore, while LLMs can simulate certain aspects of human language, they fall short of truly mimicking human behavior due to their inherent limitations in understanding, adaptability, and emotional intelligence.
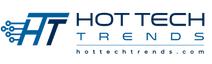