Title: Study Finds Randomization Enhances Fairness in AI Resource Allocation
Introduction: In the rapidly evolving landscape of artificial intelligence, ensuring fairness in resource allocation has emerged as a critical challenge. A recent study sheds light on the potential of randomization as a tool to enhance fairness in AI-driven decision-making processes. As AI systems increasingly influence various sectors, from healthcare to finance, the need for equitable distribution of resources becomes paramount. This study explores how incorporating randomization techniques can mitigate biases and promote fairness, offering a promising avenue for developing more just and impartial AI systems. By examining the impact of randomization on resource allocation, the research provides valuable insights into creating AI models that prioritize fairness alongside efficiency.
Understanding Randomization: A Key to Fair AI Resource Allocation
In recent years, the integration of artificial intelligence (AI) into various sectors has revolutionized the way resources are allocated, promising efficiency and precision. However, as AI systems become more prevalent, concerns about fairness and bias in resource allocation have emerged. A recent study has shed light on the potential of randomization as a tool to enhance fairness in AI-driven resource allocation processes. This study underscores the importance of understanding randomization and its implications for equitable outcomes.
Randomization, a concept rooted in statistical theory, involves making decisions by chance rather than through deterministic algorithms. In the context of AI, randomization can be employed to ensure that resource allocation does not disproportionately favor certain groups over others. The study highlights that when AI systems are designed to incorporate randomization, they can mitigate biases that may arise from historical data or algorithmic design. This is particularly crucial in sectors such as healthcare, education, and finance, where biased resource allocation can have significant societal impacts.
The study’s findings suggest that randomization can serve as a counterbalance to the inherent biases present in data-driven decision-making. By introducing an element of chance, AI systems can avoid perpetuating existing inequalities. For instance, in healthcare, randomization can be used to allocate medical resources such as organ transplants or experimental treatments, ensuring that all eligible patients have an equal opportunity to receive care. This approach not only promotes fairness but also enhances the transparency of the decision-making process, as stakeholders can be assured that allocations are not influenced by hidden biases.
Moreover, the study emphasizes that randomization does not imply a lack of structure or purpose in AI systems. Instead, it can be strategically integrated into algorithms to complement other fairness-enhancing techniques. For example, randomization can be combined with fairness constraints or bias-correction methods to create a more robust framework for equitable resource distribution. This multifaceted approach allows AI systems to maintain their efficiency and effectiveness while addressing ethical concerns.
Transitioning from theory to practice, the implementation of randomization in AI systems requires careful consideration and design. The study outlines several challenges that must be addressed to harness the full potential of randomization. One key challenge is ensuring that the randomization process itself is fair and unbiased. This involves selecting appropriate randomization techniques and parameters that align with the specific context and objectives of the resource allocation task. Additionally, there is a need for ongoing monitoring and evaluation to assess the impact of randomization on fairness outcomes and make necessary adjustments.
Furthermore, the study calls for collaboration between AI researchers, policymakers, and industry stakeholders to develop guidelines and standards for the use of randomization in AI systems. Such collaboration is essential to ensure that randomization is applied consistently and effectively across different domains. By fostering a shared understanding of the benefits and limitations of randomization, stakeholders can work together to create AI systems that prioritize fairness and equity.
In conclusion, the study provides compelling evidence that randomization can enhance fairness in AI resource allocation. By incorporating randomization into AI systems, we can address biases and promote equitable outcomes across various sectors. As AI continues to shape the future of resource allocation, understanding and implementing randomization will be key to ensuring that these systems serve the broader goal of social justice and equality.
The Role of Randomization in Mitigating Bias in AI Systems
In recent years, the integration of artificial intelligence (AI) into various sectors has revolutionized the way resources are allocated, from healthcare to education and beyond. However, as AI systems become more prevalent, concerns about bias and fairness have emerged, prompting researchers to explore innovative solutions to mitigate these issues. A recent study has highlighted the potential of randomization as a tool to enhance fairness in AI-driven resource allocation, offering a promising avenue for addressing bias in these systems.
The study, conducted by a team of researchers from leading institutions, delves into the intricacies of AI algorithms and their decision-making processes. It reveals that, despite the sophistication of these systems, inherent biases can still manifest, often reflecting the prejudices present in the data on which they are trained. These biases can lead to unequal distribution of resources, disproportionately affecting marginalized groups and perpetuating existing inequalities. In response to this challenge, the researchers propose the incorporation of randomization techniques as a means to counteract bias and promote fairness.
Randomization, a concept borrowed from statistical methods, involves introducing an element of chance into the decision-making process. By doing so, it can disrupt patterns of bias that may otherwise influence the outcomes of AI systems. The study demonstrates that when randomization is applied judiciously, it can serve as a powerful tool to level the playing field, ensuring that resources are allocated more equitably across different demographic groups. This approach not only addresses the immediate issue of bias but also fosters trust in AI systems by making their operations more transparent and understandable.
Moreover, the study emphasizes the importance of carefully designing randomization protocols to maximize their effectiveness. It suggests that randomization should be strategically integrated into the AI system’s framework, taking into account the specific context and objectives of the resource allocation task. For instance, in healthcare, randomization could be used to ensure that patients from diverse backgrounds have equal access to experimental treatments or clinical trials. In education, it might be employed to distribute scholarships or learning resources more equitably among students from various socioeconomic backgrounds.
While the potential benefits of randomization are significant, the study also acknowledges the challenges associated with its implementation. One of the primary concerns is the balance between randomness and precision. Excessive randomization could lead to inefficiencies or unintended consequences, undermining the overall effectiveness of the AI system. Therefore, it is crucial to strike a balance that maintains fairness without compromising the system’s ability to achieve its intended goals.
Furthermore, the study highlights the need for ongoing evaluation and refinement of randomization techniques. As AI systems continue to evolve, so too must the methods used to ensure their fairness. This requires a commitment to continuous research and collaboration among stakeholders, including AI developers, policymakers, and affected communities. By working together, these groups can develop and implement randomization strategies that are both effective and adaptable to changing circumstances.
In conclusion, the study underscores the potential of randomization as a valuable tool in the quest for fairness in AI resource allocation. By introducing an element of chance into decision-making processes, randomization can help mitigate bias and promote equitable outcomes. However, its successful implementation requires careful design, ongoing evaluation, and collaboration among diverse stakeholders. As AI systems become increasingly integral to our lives, embracing such innovative approaches will be essential to ensuring that they serve the interests of all members of society.
How Randomization Enhances Equity in AI Decision-Making
In recent years, the integration of artificial intelligence (AI) into various sectors has revolutionized decision-making processes, offering unprecedented efficiency and accuracy. However, as AI systems increasingly influence critical areas such as healthcare, finance, and criminal justice, concerns about fairness and equity have emerged. A recent study has shed light on an innovative approach to addressing these concerns: the use of randomization in AI resource allocation. This method, while seemingly counterintuitive, has been shown to enhance fairness in decision-making processes, thereby promoting equity across diverse populations.
To understand the significance of randomization in AI, it is essential to first consider the challenges associated with traditional AI systems. These systems often rely on historical data to make predictions and allocate resources. Unfortunately, historical data can be fraught with biases that reflect existing societal inequalities. Consequently, AI systems may inadvertently perpetuate or even exacerbate these biases, leading to unfair outcomes for marginalized groups. For instance, in the realm of healthcare, AI algorithms trained on biased data might allocate fewer resources to minority communities, thereby widening health disparities.
In response to these challenges, researchers have explored the potential of randomization as a tool to mitigate bias in AI systems. Randomization involves introducing an element of chance into the decision-making process, thereby reducing the influence of biased data. By doing so, it can help ensure that resources are distributed more equitably among different groups. The recent study highlights how randomization can be effectively integrated into AI systems to enhance fairness without compromising efficiency.
One of the key findings of the study is that randomization can serve as a powerful equalizer in situations where data-driven predictions are inherently uncertain. In such cases, relying solely on algorithmic predictions can lead to skewed outcomes that favor certain groups over others. By incorporating randomization, AI systems can distribute resources in a manner that accounts for this uncertainty, thereby promoting a more equitable allocation. This approach is particularly beneficial in scenarios where the stakes are high, and the cost of unfair resource distribution is significant.
Moreover, the study emphasizes that randomization does not necessarily imply a complete departure from data-driven decision-making. Instead, it can be strategically applied in conjunction with traditional AI methods to balance efficiency and fairness. For example, in a healthcare setting, an AI system might use data-driven predictions to identify a pool of eligible patients for a particular treatment. Randomization can then be employed to select patients from this pool, ensuring that the allocation process is not solely dictated by potentially biased data.
While the integration of randomization into AI systems presents a promising avenue for enhancing equity, it is not without its challenges. Implementing randomization requires careful consideration of ethical and practical implications, as well as ongoing evaluation to ensure that it effectively addresses bias without introducing new forms of inequity. Nevertheless, the study underscores the potential of randomization as a valuable tool in the quest for fairer AI systems.
In conclusion, as AI continues to play an increasingly prominent role in decision-making across various sectors, the importance of ensuring fairness and equity cannot be overstated. The recent study provides compelling evidence that randomization can enhance fairness in AI resource allocation, offering a novel approach to addressing the biases inherent in traditional AI systems. By embracing randomization, stakeholders can work towards creating AI systems that not only optimize efficiency but also uphold the principles of equity and justice.
Case Studies: Randomization Improving Fairness in AI Applications
In recent years, the integration of artificial intelligence (AI) into various sectors has sparked both enthusiasm and concern. While AI systems have the potential to revolutionize industries by improving efficiency and decision-making, they also pose significant challenges, particularly in terms of fairness and bias. A recent study has shed light on an innovative approach to addressing these challenges: the use of randomization in AI resource allocation. This study provides compelling evidence that randomization can enhance fairness in AI applications, offering a promising avenue for mitigating bias and ensuring equitable outcomes.
The study, conducted by a team of researchers from leading institutions, explored the impact of randomization on fairness in AI systems across several case studies. These case studies spanned diverse fields, including healthcare, finance, and education, where AI is increasingly being used to allocate resources and make critical decisions. By introducing randomization into the decision-making process, the researchers aimed to reduce the influence of inherent biases that often plague AI algorithms.
One of the key findings of the study was that randomization can serve as an effective tool for leveling the playing field. In the healthcare sector, for instance, AI systems are frequently used to prioritize patients for treatments or allocate medical resources. However, these systems can inadvertently perpetuate existing disparities if they rely solely on historical data, which may reflect biased practices. By incorporating randomization, the researchers found that AI systems were able to distribute resources more equitably, ensuring that patients from marginalized groups received fair consideration.
Similarly, in the financial sector, AI-driven credit scoring models often determine individuals’ access to loans and financial services. These models can be biased against certain demographic groups, leading to unequal opportunities. The study demonstrated that by introducing a degree of randomness into the credit scoring process, financial institutions could mitigate these biases, resulting in fairer outcomes for all applicants. This approach not only promotes equity but also enhances the overall trustworthiness of AI systems in sensitive applications.
Furthermore, the study highlighted the potential of randomization to improve fairness in educational settings. AI systems are increasingly used to allocate resources such as scholarships, admissions, and personalized learning tools. However, these systems can inadvertently favor students from privileged backgrounds if they rely on biased data. By incorporating randomization, educational institutions can ensure that all students have an equal chance of benefiting from these resources, thereby promoting diversity and inclusion.
While the study’s findings are promising, it is important to acknowledge the limitations and challenges associated with implementing randomization in AI systems. Critics argue that randomization may introduce uncertainty and reduce the efficiency of decision-making processes. However, the researchers contend that the benefits of enhanced fairness outweigh these potential drawbacks, particularly in contexts where equity is a primary concern.
In conclusion, the study provides valuable insights into the role of randomization in improving fairness in AI applications. By reducing the impact of biases and ensuring equitable resource allocation, randomization offers a viable solution to one of the most pressing challenges facing AI today. As AI continues to permeate various aspects of society, it is imperative that stakeholders consider innovative approaches like randomization to promote fairness and build trust in these powerful technologies. The findings of this study serve as a call to action for researchers, policymakers, and industry leaders to explore and implement randomization as a means of achieving more equitable AI systems.
The Science Behind Randomization and Fairness in AI
In recent years, the integration of artificial intelligence (AI) into various sectors has revolutionized the way resources are allocated, from healthcare to finance. However, the fairness of these AI-driven systems has been a subject of intense scrutiny and debate. A recent study has shed light on the potential of randomization as a tool to enhance fairness in AI resource allocation, offering a promising avenue for addressing biases that have long plagued algorithmic decision-making.
The concept of fairness in AI is multifaceted, encompassing notions of equity, impartiality, and justice. Traditional AI systems often rely on historical data to make predictions and allocate resources. Unfortunately, this data can be tainted with biases that reflect societal inequalities, leading to unfair outcomes. For instance, in the context of loan approvals, an AI system trained on biased data might disproportionately deny loans to certain demographic groups. This is where randomization comes into play as a potential solution.
Randomization, in the context of AI, involves introducing an element of chance into the decision-making process. By doing so, it can help mitigate the impact of biased data and ensure a more equitable distribution of resources. The recent study highlights how randomization can be strategically employed to enhance fairness without compromising the efficiency of AI systems. By randomly selecting from a pool of equally qualified candidates or options, AI systems can prevent the perpetuation of existing biases and promote a more balanced allocation of resources.
Moreover, the study emphasizes that randomization does not imply a complete abandonment of data-driven decision-making. Instead, it suggests a hybrid approach where randomization is used in conjunction with traditional AI methods. This approach allows for the retention of the predictive power of AI while simultaneously addressing fairness concerns. For example, in a healthcare setting, an AI system could use randomization to decide which patients receive additional resources when multiple patients have similar needs and prognoses. This ensures that no particular group is systematically favored or disadvantaged.
Transitioning from theory to practice, the implementation of randomization in AI systems requires careful consideration and design. The study outlines several key factors that must be addressed to effectively integrate randomization into AI resource allocation. These include determining the appropriate level of randomization, ensuring transparency in the decision-making process, and continuously monitoring outcomes to assess the impact on fairness. By addressing these factors, organizations can harness the benefits of randomization while minimizing potential drawbacks.
Furthermore, the study acknowledges that randomization is not a panacea for all fairness issues in AI. It is one of many tools that can be employed to create more equitable systems. Other approaches, such as bias correction algorithms and diverse training datasets, should also be considered as part of a comprehensive strategy to enhance fairness. Nevertheless, the introduction of randomization offers a novel perspective and a valuable addition to the toolkit for addressing bias in AI.
In conclusion, the study provides compelling evidence that randomization can play a crucial role in enhancing fairness in AI resource allocation. By introducing an element of chance, AI systems can mitigate the impact of biased data and promote more equitable outcomes. As AI continues to permeate various aspects of society, it is imperative that fairness remains a central consideration in its development and deployment. Randomization, alongside other fairness-enhancing strategies, offers a promising path forward in the quest for just and impartial AI systems.
Future Implications of Randomization in AI Resource Distribution
A recent study has shed light on the potential benefits of incorporating randomization into artificial intelligence (AI) systems, particularly in the context of resource allocation. As AI continues to play an increasingly pivotal role in decision-making processes across various sectors, ensuring fairness in these systems has become a pressing concern. The study, conducted by a team of researchers from leading institutions, suggests that randomization could serve as a valuable tool in enhancing fairness, thereby addressing some of the ethical challenges associated with AI.
The concept of fairness in AI is multifaceted, often involving the equitable distribution of resources, opportunities, or outcomes among different groups. Traditional AI systems, which rely heavily on historical data and deterministic algorithms, can inadvertently perpetuate existing biases. This is because the data used to train these systems often reflect societal inequalities, leading to skewed outcomes that favor certain groups over others. In contrast, randomization introduces an element of chance, which can help mitigate these biases by ensuring that decisions are not solely based on potentially flawed data.
Moreover, the study highlights that randomization can be particularly effective in scenarios where resources are limited and demand exceeds supply. In such cases, deterministic algorithms may struggle to allocate resources equitably, as they often prioritize efficiency over fairness. By incorporating randomization, AI systems can distribute resources in a manner that is perceived as more impartial, thereby enhancing public trust in these technologies. This is especially important in sectors such as healthcare, where the stakes are high and the consequences of biased decision-making can be severe.
Furthermore, the researchers emphasize that randomization should not be viewed as a standalone solution but rather as a complementary approach to existing fairness-enhancing techniques. For instance, combining randomization with methods such as fairness constraints or bias detection algorithms can lead to more robust outcomes. This integrated approach allows for a more nuanced understanding of fairness, taking into account both the need for equitable treatment and the practical constraints of resource allocation.
In addition to its potential benefits, the study also acknowledges the challenges associated with implementing randomization in AI systems. One of the primary concerns is the potential for randomness to introduce variability in outcomes, which could be perceived as unfair by some stakeholders. To address this issue, the researchers propose the use of controlled randomization techniques, which balance the need for fairness with the requirement for consistency. By carefully calibrating the degree of randomness, AI systems can achieve a desirable trade-off between equity and predictability.
Looking ahead, the implications of this study are significant for the future of AI resource distribution. As AI continues to evolve and permeate various aspects of society, the demand for fair and transparent decision-making processes will only grow. By embracing randomization as a tool for enhancing fairness, policymakers and developers can work towards creating AI systems that are not only efficient but also equitable. This, in turn, can help foster greater public confidence in AI technologies, paving the way for their broader acceptance and integration into everyday life.
In conclusion, the study underscores the importance of exploring innovative approaches to fairness in AI, with randomization emerging as a promising avenue. While challenges remain, the potential benefits of incorporating randomization into AI systems are substantial, offering a pathway towards more equitable resource allocation. As the field of AI continues to advance, ongoing research and collaboration will be crucial in ensuring that these technologies serve the best interests of all members of society.
Q&A
1. **What is the main finding of the study?**
The study finds that incorporating randomization into AI algorithms can enhance fairness in resource allocation by reducing biases and ensuring more equitable distribution.
2. **How does randomization improve fairness in AI?**
Randomization helps mitigate biases inherent in data and decision-making processes, leading to more balanced outcomes and preventing systematic favoritism or discrimination.
3. **What are the potential applications of this finding?**
This approach can be applied in various fields such as healthcare, education, and finance, where fair resource allocation is critical, ensuring that AI systems do not perpetuate existing inequalities.
4. **What challenges might arise from implementing randomization in AI systems?**
Challenges include maintaining efficiency and effectiveness of AI systems while integrating randomness, as well as ensuring transparency and understanding of the randomization process by stakeholders.
5. **Did the study address any limitations of using randomization?**
Yes, the study acknowledges that while randomization can enhance fairness, it may also introduce variability in outcomes, which needs to be carefully managed to avoid unintended consequences.
6. **What future research directions does the study suggest?**
The study suggests exploring hybrid models that combine randomization with other fairness-enhancing techniques, as well as investigating the long-term impacts of randomization on systemic fairness in AI-driven systems.The study concludes that incorporating randomization into AI-driven resource allocation processes can significantly enhance fairness. By introducing elements of chance, randomization helps mitigate biases inherent in algorithmic decision-making, ensuring a more equitable distribution of resources. This approach can prevent the systematic favoring of certain groups over others, promoting inclusivity and fairness. The findings suggest that randomization can be a valuable tool in addressing ethical concerns and improving the overall trustworthiness of AI systems in resource allocation tasks.
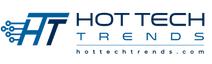