The Positive Feedback Loop of AI Research refers to the self-reinforcing cycle that accelerates advancements in artificial intelligence. As AI technologies improve, they enhance the tools and methodologies available to researchers, leading to more efficient and effective research processes. This, in turn, results in the development of even more sophisticated AI systems. The loop is fueled by increased computational power, the availability of large datasets, and the growing community of researchers and practitioners contributing to the field. As AI systems become more capable, they assist in automating and optimizing research tasks, further propelling innovation and discovery. This cycle not only accelerates the pace of AI development but also expands its applications across various domains, creating a dynamic and rapidly evolving landscape in AI research.
Accelerating Innovation Through AI Feedback Loops
The rapid advancement of artificial intelligence (AI) has been a defining feature of technological progress in recent years. Central to this acceleration is the concept of the positive feedback loop, a mechanism by which AI research and development continuously enhance each other, leading to exponential growth in innovation. This phenomenon is not only reshaping the landscape of technology but also redefining the boundaries of what is possible in various fields.
At the core of this feedback loop is the iterative nature of AI development. As researchers develop more sophisticated algorithms, these algorithms, in turn, facilitate the creation of even more advanced AI systems. For instance, machine learning models, which are a subset of AI, are designed to improve their performance as they are exposed to more data. This self-improvement capability allows AI systems to refine their algorithms autonomously, thereby accelerating the pace of innovation. Consequently, the more data these systems process, the more efficient and effective they become, creating a cycle of continuous enhancement.
Moreover, the integration of AI into research processes has significantly increased the speed and efficiency of scientific discovery. AI tools are now capable of analyzing vast datasets far more quickly than human researchers, identifying patterns and insights that might otherwise remain hidden. This capability not only expedites the research process but also opens up new avenues for exploration that were previously deemed too complex or time-consuming. As a result, AI is not merely a tool for research but a catalyst for innovation, driving breakthroughs across disciplines ranging from medicine to materials science.
In addition to accelerating research, AI is also transforming the way innovations are implemented and scaled. The automation of routine tasks through AI technologies allows researchers and developers to focus on more complex and creative aspects of their work. This shift not only enhances productivity but also fosters an environment where innovative ideas can be rapidly prototyped and tested. Furthermore, AI-driven platforms facilitate collaboration across geographical and disciplinary boundaries, enabling a more diverse range of perspectives and expertise to contribute to the innovation process.
The positive feedback loop of AI research is further amplified by the democratization of AI technologies. Open-source platforms and collaborative initiatives have made cutting-edge AI tools accessible to a broader audience, empowering individuals and organizations to contribute to the field. This inclusivity not only accelerates the pace of innovation but also ensures that a wider array of challenges and opportunities are addressed. As more people engage with AI technologies, the collective knowledge and experience grow, feeding back into the development of even more advanced systems.
However, it is important to acknowledge the challenges that accompany this rapid advancement. Ethical considerations, such as data privacy and algorithmic bias, must be addressed to ensure that the benefits of AI are equitably distributed. Moreover, as AI systems become more complex, ensuring their transparency and accountability becomes increasingly crucial. Addressing these challenges requires a concerted effort from researchers, policymakers, and industry leaders to establish frameworks that guide the responsible development and deployment of AI technologies.
In conclusion, the positive feedback loop of AI research is a powerful driver of innovation, propelling advancements at an unprecedented pace. By continuously enhancing the capabilities of AI systems and expanding their applications, this feedback loop is not only transforming the technological landscape but also unlocking new possibilities for addressing some of the world’s most pressing challenges. As we navigate this rapidly evolving field, it is imperative to balance the pursuit of innovation with a commitment to ethical and responsible AI development.
Enhancing AI Models with Continuous Learning
The field of artificial intelligence (AI) has witnessed remarkable advancements over the past few decades, largely driven by the concept of continuous learning. This approach, which involves the ongoing improvement and adaptation of AI models, has created a positive feedback loop that enhances the capabilities of these systems. Continuous learning allows AI models to evolve by integrating new data and experiences, thereby refining their performance and expanding their applicability across various domains.
At the heart of continuous learning is the ability of AI systems to learn from new information without forgetting previously acquired knowledge. This is achieved through techniques such as transfer learning, where models leverage pre-existing knowledge to understand new tasks more efficiently. By doing so, AI models can quickly adapt to changes in their environment, making them more robust and versatile. This adaptability is crucial in dynamic fields such as healthcare, finance, and autonomous systems, where the ability to respond to new data can significantly impact outcomes.
Moreover, continuous learning fosters innovation by encouraging researchers to develop novel algorithms and architectures that can handle the complexities of real-world data. As AI models are exposed to diverse datasets, they encounter a wide range of scenarios that challenge their existing capabilities. This necessitates the creation of more sophisticated models that can generalize better and make more accurate predictions. Consequently, the pursuit of continuous learning drives the development of cutting-edge technologies that push the boundaries of what AI can achieve.
In addition to enhancing model performance, continuous learning also contributes to the democratization of AI. As models become more adept at learning from smaller datasets, the barrier to entry for developing AI applications is lowered. This enables a broader range of individuals and organizations to harness the power of AI, fostering a more inclusive and diverse ecosystem. By making AI more accessible, continuous learning empowers a wider array of stakeholders to contribute to the field, leading to a richer tapestry of ideas and innovations.
Furthermore, the positive feedback loop created by continuous learning extends beyond technical improvements. It also has significant implications for the ethical development of AI. As models learn from a broader spectrum of data, they become more attuned to the nuances of human behavior and societal norms. This awareness can help mitigate biases and ensure that AI systems operate in a manner that aligns with ethical standards. By continuously refining their understanding of the world, AI models can contribute to more equitable and fair outcomes.
The integration of continuous learning into AI research also facilitates collaboration across disciplines. As AI models become more sophisticated, they require insights from fields such as neuroscience, cognitive science, and linguistics to enhance their learning processes. This interdisciplinary approach not only enriches the field of AI but also leads to breakthroughs that can be applied across various scientific domains. By fostering collaboration, continuous learning accelerates the pace of discovery and innovation.
In conclusion, the positive feedback loop of AI research, driven by continuous learning, is a powerful force that enhances the capabilities of AI models. By enabling these systems to adapt and improve over time, continuous learning not only advances the technical frontiers of AI but also promotes ethical development and interdisciplinary collaboration. As the field continues to evolve, the principles of continuous learning will remain central to unlocking the full potential of artificial intelligence.
The Role of Data in AI Feedback Mechanisms
In the rapidly evolving field of artificial intelligence, the role of data in feedback mechanisms is pivotal, serving as the backbone for the development and refinement of AI models. As AI systems are designed to learn from data, the quality and quantity of this data significantly influence the effectiveness of these systems. The concept of a positive feedback loop in AI research is particularly relevant, as it describes a cycle where data collection and AI model improvement mutually reinforce each other, leading to continuous advancements in the field.
Initially, AI models are trained on datasets that provide the foundational knowledge necessary for them to perform specific tasks. These datasets are meticulously curated to ensure they are representative of the real-world scenarios the AI will encounter. As the AI system processes this data, it begins to identify patterns and make predictions. However, the true power of AI is unleashed when these systems are exposed to new data, which allows them to refine their algorithms and improve their accuracy over time. This iterative process is at the heart of the positive feedback loop, where each cycle of learning enhances the model’s performance, thereby increasing its ability to handle more complex tasks.
Moreover, the feedback loop is further strengthened by the continuous influx of data generated by AI systems themselves. As these systems are deployed in various applications, they generate vast amounts of new data through their interactions with users and environments. This new data, in turn, becomes a valuable resource for further training and refining AI models. For instance, in natural language processing, AI systems like chatbots and virtual assistants learn from user interactions, which helps them to better understand and respond to human language. This self-improving mechanism ensures that AI systems remain relevant and effective in dynamic environments.
In addition to the self-generated data, the integration of diverse data sources also plays a crucial role in enhancing AI feedback mechanisms. By incorporating data from different domains, AI models can gain a more comprehensive understanding of the world, leading to more robust and versatile systems. This diversity in data sources not only improves the generalization capabilities of AI models but also helps in mitigating biases that may arise from limited or skewed datasets. Consequently, the positive feedback loop is not only about increasing the volume of data but also about enriching its variety and quality.
Furthermore, advancements in data processing technologies have significantly contributed to the efficiency of AI feedback mechanisms. With the advent of powerful computing resources and sophisticated algorithms, AI systems can now process and analyze vast datasets at unprecedented speeds. This capability allows for real-time feedback and rapid iteration, accelerating the pace of AI research and development. As a result, the positive feedback loop is continuously fueled by technological innovations that enhance data processing and analysis.
In conclusion, the role of data in AI feedback mechanisms is integral to the positive feedback loop that drives AI research forward. By leveraging high-quality, diverse datasets and harnessing the power of advanced data processing technologies, AI systems can continuously learn and improve. This self-reinforcing cycle not only propels the development of more sophisticated AI models but also ensures their adaptability and relevance in an ever-changing world. As AI continues to evolve, the interplay between data and feedback mechanisms will remain a cornerstone of its progress, shaping the future of technology and its applications.
Collaborative AI Research and Its Compounding Benefits
The field of artificial intelligence (AI) has witnessed unprecedented growth over the past few decades, driven largely by collaborative research efforts that have created a positive feedback loop, propelling the discipline forward. This collaborative nature of AI research not only accelerates technological advancements but also fosters an environment where knowledge is shared, leading to compounding benefits for the scientific community and society at large.
To begin with, the collaborative approach in AI research allows for the pooling of diverse expertise and resources, which is crucial in tackling complex problems that no single entity could address alone. Researchers from various disciplines, such as computer science, neuroscience, and cognitive psychology, come together to contribute their unique perspectives and skills. This interdisciplinary collaboration enriches the research process, leading to more innovative solutions and breakthroughs. For instance, the development of neural networks, which are inspired by the human brain, has been significantly enhanced by insights from neuroscientists working alongside computer scientists.
Moreover, the open sharing of data and research findings is a hallmark of collaborative AI research. Open-source platforms and repositories, such as GitHub and arXiv, have become instrumental in disseminating knowledge and facilitating collaboration across geographical boundaries. This openness not only democratizes access to cutting-edge research but also enables researchers to build upon each other’s work, thereby accelerating the pace of innovation. As a result, the AI community can rapidly iterate on ideas, test new hypotheses, and refine algorithms, leading to more robust and efficient AI systems.
In addition to fostering innovation, collaborative AI research also plays a critical role in addressing ethical and societal challenges associated with AI technologies. By bringing together diverse stakeholders, including ethicists, policymakers, and industry leaders, collaborative efforts ensure that AI development is aligned with societal values and ethical standards. This collective approach helps in identifying potential biases in AI systems, developing fair and transparent algorithms, and creating guidelines for responsible AI deployment. Consequently, the positive feedback loop of collaboration not only advances technological capabilities but also ensures that these advancements are beneficial and equitable for all.
Furthermore, the compounding benefits of collaborative AI research extend to education and workforce development. As researchers share their findings and methodologies, educational institutions can incorporate the latest advancements into their curricula, preparing the next generation of AI professionals. This continuous flow of knowledge from research to education creates a well-informed workforce capable of driving further innovation. Additionally, industry-academia partnerships facilitate the transfer of knowledge and technology, enabling companies to leverage cutting-edge research for commercial applications, thereby contributing to economic growth.
In conclusion, the positive feedback loop of collaborative AI research is a powerful engine driving the field forward. By fostering interdisciplinary collaboration, promoting open sharing of knowledge, addressing ethical challenges, and enhancing education and workforce development, this collaborative approach generates compounding benefits that extend beyond the scientific community. As AI continues to evolve, maintaining and strengthening these collaborative efforts will be essential in ensuring that the technology develops in a way that is both innovative and aligned with societal needs. Through continued collaboration, the potential of AI to transform industries, improve lives, and solve global challenges can be fully realized, creating a future where technology and humanity thrive together.
Feedback Loops in AI: Driving Efficiency and Accuracy
The field of artificial intelligence (AI) has witnessed remarkable advancements over the past few decades, largely driven by the concept of feedback loops. These loops, integral to the development and refinement of AI systems, play a crucial role in enhancing both efficiency and accuracy. At the core of AI research, feedback loops facilitate continuous learning and adaptation, enabling systems to improve their performance over time. By understanding the dynamics of these loops, researchers and developers can harness their potential to create more sophisticated and reliable AI models.
Initially, feedback loops in AI research are established through the process of training models on large datasets. During this phase, AI systems are exposed to vast amounts of information, allowing them to identify patterns and make predictions. As these systems process data, they generate outputs that are compared against known results. This comparison forms the basis of the feedback loop, as discrepancies between predicted and actual outcomes are used to adjust the model’s parameters. Consequently, the system learns from its mistakes, gradually improving its accuracy and efficiency.
Moreover, the iterative nature of feedback loops ensures that AI systems remain adaptable in dynamic environments. As new data becomes available, these systems can incorporate it into their learning process, refining their models to better reflect current realities. This adaptability is particularly valuable in fields such as healthcare, finance, and autonomous vehicles, where conditions can change rapidly and unpredictably. By continuously updating their knowledge base, AI systems can maintain high levels of performance, even in the face of evolving challenges.
In addition to enhancing adaptability, feedback loops also contribute to the optimization of AI algorithms. Through repeated cycles of evaluation and adjustment, these loops help identify inefficiencies and areas for improvement within the system. For instance, by analyzing the feedback from previous iterations, researchers can pinpoint bottlenecks or redundancies in the algorithm’s structure. Addressing these issues not only boosts the system’s efficiency but also reduces computational costs, making AI technologies more accessible and sustainable.
Furthermore, feedback loops foster collaboration between AI systems and human experts. In many applications, AI models are used to augment human decision-making, providing insights and recommendations based on data analysis. By incorporating human feedback into the loop, these systems can align their outputs more closely with human values and preferences. This synergy between AI and human intelligence enhances the overall decision-making process, leading to more informed and effective outcomes.
As AI research continues to evolve, the importance of feedback loops is likely to grow even further. Emerging technologies, such as reinforcement learning and generative adversarial networks, rely heavily on these loops to achieve their full potential. In reinforcement learning, for example, agents learn by interacting with their environment and receiving feedback in the form of rewards or penalties. This feedback loop enables the agent to develop strategies that maximize its long-term success. Similarly, generative adversarial networks use feedback loops to refine their ability to generate realistic data, pushing the boundaries of what AI can achieve.
In conclusion, feedback loops are a fundamental component of AI research, driving improvements in efficiency and accuracy. By facilitating continuous learning and adaptation, these loops enable AI systems to thrive in complex and changing environments. As researchers continue to explore the possibilities of AI, the role of feedback loops will remain central to the development of more advanced and reliable technologies. Through their iterative nature, these loops not only enhance the capabilities of AI systems but also promote collaboration between machines and humans, ultimately leading to a more intelligent and interconnected world.
The Future of AI: Harnessing Positive Feedback for Growth
The field of artificial intelligence (AI) has witnessed unprecedented growth over the past few decades, driven by a positive feedback loop that continues to propel advancements in technology and research. This self-reinforcing cycle is characterized by the interplay between technological innovation, increased investment, and the expanding application of AI across various sectors. As AI systems become more sophisticated, they generate more data, which in turn fuels further research and development, creating a virtuous cycle of progress.
To begin with, the rapid advancements in AI technology have been largely facilitated by the exponential growth in computational power and the availability of vast amounts of data. These resources have enabled researchers to develop more complex algorithms and models, which are capable of performing tasks that were once thought to be the exclusive domain of human intelligence. As these AI systems demonstrate their utility and effectiveness, they attract more attention from both the public and private sectors, leading to increased funding and investment in AI research. This influx of resources allows for the exploration of new ideas and the refinement of existing technologies, further accelerating the pace of innovation.
Moreover, the expanding application of AI across various industries has created a demand for more specialized and advanced AI solutions. From healthcare and finance to transportation and entertainment, AI is being integrated into a wide range of fields, each with its own unique challenges and requirements. This diversification of AI applications not only drives the development of new technologies but also provides valuable insights and data that can be used to improve existing systems. As AI continues to permeate different sectors, it generates a wealth of information that researchers can leverage to enhance the capabilities of AI models, thereby contributing to the positive feedback loop.
In addition to technological advancements and increased investment, the collaborative nature of AI research has played a crucial role in sustaining this positive feedback loop. The open exchange of ideas and information among researchers, institutions, and companies has fostered a culture of innovation and collaboration that has been instrumental in driving progress. By sharing their findings and building upon each other’s work, researchers can accelerate the development of new technologies and overcome challenges more efficiently. This collaborative approach not only enhances the quality of AI research but also ensures that the benefits of AI are more widely distributed across society.
Furthermore, the positive feedback loop of AI research is reinforced by the growing recognition of the potential benefits and transformative impact of AI on society. As AI systems continue to demonstrate their ability to improve efficiency, reduce costs, and enhance decision-making processes, there is an increasing awareness of the value that AI can bring to various aspects of life. This recognition has led to greater public and governmental support for AI research, which in turn fuels further investment and innovation.
In conclusion, the positive feedback loop of AI research is a powerful driver of growth and development in the field of artificial intelligence. By harnessing the interplay between technological advancements, increased investment, and the expanding application of AI, researchers can continue to push the boundaries of what is possible and unlock new opportunities for innovation. As this cycle of progress continues, it holds the potential to transform industries, improve lives, and shape the future of society in profound ways.
Q&A
1. **What is the Positive Feedback Loop in AI Research?**
The Positive Feedback Loop in AI Research refers to the cycle where advancements in AI technology lead to improved tools and methods, which in turn accelerate further AI research and development.
2. **How does increased computational power contribute to the loop?**
Increased computational power allows researchers to train more complex models faster, leading to breakthroughs that further enhance computational techniques and hardware, perpetuating the cycle.
3. **What role does data play in the feedback loop?**
Access to larger and more diverse datasets improves AI model training, resulting in better performance and insights, which drive the demand for more data collection and sharing.
4. **How do improved AI models impact research productivity?**
Improved AI models automate and optimize research processes, increasing productivity and enabling researchers to tackle more complex problems, thus fueling further AI advancements.
5. **What is the effect of AI research on interdisciplinary fields?**
AI research contributes tools and methodologies to interdisciplinary fields, enhancing their capabilities and leading to innovations that feed back into AI research with new challenges and data.
6. **How does funding influence the positive feedback loop?**
Successful AI research attracts more funding, which supports further research and development, leading to more breakthroughs and attracting even more investment, sustaining the loop.The positive feedback loop of AI research refers to the self-reinforcing cycle where advancements in artificial intelligence lead to improved tools and methodologies, which in turn accelerate further research and development. As AI systems become more sophisticated, they enhance data analysis, optimize research processes, and enable the creation of more powerful algorithms. This progress attracts increased investment and talent, fostering a thriving ecosystem that propels innovation. Consequently, the rapid evolution of AI technologies continues to drive breakthroughs across various fields, creating a dynamic and exponential growth pattern in AI research and its applications.
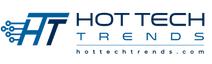