The Positive Feedback Loop of AI Research refers to the self-reinforcing cycle that accelerates advancements in artificial intelligence. As AI technologies improve, they enhance research capabilities, leading to more efficient data processing, better algorithms, and innovative applications. This progress, in turn, attracts increased investment and interest from academia, industry, and governments, further fueling research and development. The loop is characterized by the continuous interplay between technological breakthroughs and their subsequent impact on research methodologies, creating a dynamic environment where each advancement lays the groundwork for future innovations. This cycle not only propels AI forward but also expands its potential to transform various sectors, driving exponential growth in the field.
Accelerating Innovation Through AI Feedback Loops
The rapid advancement of artificial intelligence (AI) has been a defining feature of technological progress in recent years. Central to this acceleration is the concept of the positive feedback loop, a mechanism that is increasingly driving innovation in AI research. This feedback loop is characterized by the continuous cycle of improvement, where advancements in AI lead to further innovations, which in turn enhance AI capabilities. As AI systems become more sophisticated, they contribute to their own development, creating a self-reinforcing cycle of progress.
To understand the dynamics of this feedback loop, it is essential to consider how AI technologies are developed and refined. Initially, AI systems are designed to perform specific tasks, such as image recognition or natural language processing. As these systems are deployed, they generate vast amounts of data, which can be analyzed to identify patterns and improve performance. This data-driven approach allows researchers to refine algorithms, making them more efficient and accurate. Consequently, improved algorithms can tackle more complex problems, generating even more data and insights.
Moreover, the integration of AI into various industries has further fueled this feedback loop. In sectors such as healthcare, finance, and transportation, AI systems are being used to optimize processes, enhance decision-making, and predict outcomes. As these systems are implemented, they provide valuable feedback that informs future research and development. For instance, in healthcare, AI-driven diagnostic tools can analyze patient data to identify potential health issues, leading to more targeted and effective treatments. The insights gained from these applications not only improve patient outcomes but also contribute to the refinement of AI technologies.
In addition to industry-specific applications, the collaborative nature of AI research has played a crucial role in accelerating innovation. Researchers and developers from diverse fields are increasingly working together, sharing knowledge and resources to push the boundaries of what AI can achieve. This collaborative approach has led to the development of open-source platforms and tools, which facilitate the exchange of ideas and foster a culture of continuous improvement. As a result, breakthroughs in one area of AI research can quickly be adapted and applied to other domains, further amplifying the positive feedback loop.
Furthermore, the role of machine learning, a subset of AI, cannot be overlooked in this context. Machine learning algorithms are designed to learn from data, improving their performance over time without explicit programming. This ability to learn and adapt is a key driver of the feedback loop, as it enables AI systems to become more autonomous and efficient. As machine learning models are exposed to new data, they refine their predictions and decision-making processes, leading to more accurate and reliable outcomes. This iterative process of learning and adaptation is fundamental to the continuous cycle of innovation in AI research.
In conclusion, the positive feedback loop of AI research is a powerful mechanism that is accelerating innovation across various domains. By leveraging data-driven insights, fostering collaboration, and harnessing the capabilities of machine learning, AI systems are not only improving their own performance but also driving advancements in technology and industry. As this feedback loop continues to evolve, it holds the potential to unlock new possibilities and transform the way we live and work, underscoring the transformative impact of AI on society.
Enhancing AI Models with Continuous Learning
The field of artificial intelligence (AI) has witnessed remarkable advancements over the past few decades, largely driven by the concept of continuous learning. This approach, which allows AI models to adapt and improve over time, has created a positive feedback loop that enhances their capabilities and broadens their applications. Continuous learning, also known as lifelong learning, enables AI systems to learn from new data and experiences, thereby refining their performance and expanding their knowledge base. This process is crucial in addressing the limitations of traditional AI models, which often rely on static datasets and predefined algorithms.
One of the primary benefits of continuous learning is its ability to improve the accuracy and efficiency of AI models. As these models are exposed to new information, they can update their parameters and algorithms to better reflect the current state of the world. This adaptability is particularly important in dynamic environments where conditions change rapidly, such as in financial markets or autonomous driving. By continuously learning from real-time data, AI systems can make more informed decisions and predictions, ultimately leading to better outcomes.
Moreover, continuous learning facilitates the development of more generalized AI models. Traditional AI systems are typically designed for specific tasks and may struggle when faced with unfamiliar situations. However, by incorporating continuous learning, AI models can acquire a broader understanding of various contexts and scenarios. This generalization capability is essential for creating versatile AI systems that can perform multiple tasks and adapt to new challenges without requiring extensive retraining.
In addition to improving model performance, continuous learning also contributes to the ethical and responsible deployment of AI technologies. As AI systems learn from diverse datasets, they can become more aware of potential biases and inaccuracies in their training data. This awareness allows developers to identify and mitigate these issues, leading to fairer and more equitable AI solutions. Furthermore, continuous learning can help AI systems recognize and respond to ethical dilemmas in real-time, ensuring that they align with societal values and norms.
The positive feedback loop of AI research is further amplified by the collaborative nature of the field. Researchers and developers from various disciplines contribute to the continuous learning process by sharing their findings and insights. This collaboration fosters innovation and accelerates the development of new techniques and methodologies. As a result, AI models become more sophisticated and capable, driving further research and exploration.
However, the implementation of continuous learning in AI models is not without its challenges. One significant concern is the potential for catastrophic forgetting, where new information overwrites previously learned knowledge. To address this issue, researchers are exploring techniques such as memory replay and regularization, which help maintain a balance between retaining old knowledge and acquiring new information. Additionally, the computational resources required for continuous learning can be substantial, necessitating advancements in hardware and software to support these demands.
In conclusion, the positive feedback loop of AI research, driven by continuous learning, is transforming the landscape of artificial intelligence. By enabling AI models to adapt and improve over time, continuous learning enhances their accuracy, generalization, and ethical considerations. This ongoing process not only advances the capabilities of AI systems but also fosters collaboration and innovation within the research community. As the field continues to evolve, the integration of continuous learning will undoubtedly play a pivotal role in shaping the future of artificial intelligence.
The Role of Data in AI Feedback Mechanisms
In the rapidly evolving field of artificial intelligence, the role of data in feedback mechanisms is pivotal, serving as the backbone for the development and refinement of AI models. As AI systems are designed to learn from data, the quality and quantity of this data significantly influence the effectiveness of these systems. The concept of a positive feedback loop in AI research is intrinsically linked to how data is utilized, processed, and fed back into the system to enhance performance and accuracy.
To begin with, data serves as the foundational element upon which AI models are built. The initial dataset used to train an AI model determines its baseline capabilities. As the model processes this data, it begins to identify patterns and make predictions. However, the true power of AI is unleashed when these predictions are evaluated and the results are fed back into the system. This feedback loop allows the model to adjust its parameters, thereby improving its accuracy over time. The iterative nature of this process means that with each cycle, the AI system becomes more adept at understanding and interpreting complex data sets.
Moreover, the feedback loop is not solely about improving accuracy; it also plays a crucial role in identifying and mitigating biases within AI models. As data is fed back into the system, discrepancies and biases can be detected and addressed. This is particularly important in ensuring that AI systems are fair and equitable, as biased data can lead to skewed outcomes. By continuously refining the data and the model, researchers can work towards creating AI systems that are not only more accurate but also more just.
In addition to improving model performance and reducing bias, the feedback loop facilitated by data also accelerates the pace of AI research. As models become more sophisticated, they require increasingly complex data to continue improving. This demand for high-quality data drives innovation in data collection and processing techniques. Consequently, as researchers develop new methods for gathering and analyzing data, these advancements feed back into the AI models, creating a cycle of continuous improvement and innovation.
Furthermore, the positive feedback loop of AI research is amplified by the collaborative nature of the field. As researchers share data and findings, the collective knowledge base expands, providing more resources for refining AI models. This collaboration not only enhances individual models but also contributes to the overall advancement of AI technology. The shared data and insights act as a catalyst, propelling the field forward at an unprecedented rate.
In conclusion, the role of data in AI feedback mechanisms is a critical component of the positive feedback loop that drives AI research. By serving as the foundation for model training, enabling bias detection and correction, and fostering innovation through collaboration, data is at the heart of AI development. As the field continues to evolve, the importance of high-quality data and effective feedback mechanisms will only grow, ensuring that AI systems become increasingly sophisticated, accurate, and equitable. This ongoing cycle of improvement underscores the transformative potential of AI and highlights the essential role that data plays in shaping the future of technology.
Collaborative AI Research and Its Compounding Benefits
The field of artificial intelligence (AI) has witnessed unprecedented growth over the past few decades, largely due to the collaborative nature of research and development within the community. This collaborative approach has created a positive feedback loop, where shared knowledge and resources lead to accelerated advancements, which in turn foster further collaboration. As researchers and institutions work together, they not only enhance the capabilities of AI technologies but also address complex challenges that would be insurmountable in isolation.
One of the primary drivers of this positive feedback loop is the open sharing of data and algorithms. By making datasets and code publicly available, researchers enable others to build upon their work, leading to rapid iterations and improvements. This openness not only democratizes access to cutting-edge tools but also encourages a diverse range of perspectives to contribute to problem-solving. Consequently, the collective intelligence of the global research community is harnessed, resulting in innovative solutions that push the boundaries of what AI can achieve.
Moreover, collaborative AI research often involves interdisciplinary partnerships, bringing together experts from various fields such as computer science, neuroscience, linguistics, and ethics. These collaborations are crucial because they allow for a more holistic understanding of AI’s potential and limitations. For instance, insights from neuroscience can inform the development of more efficient neural networks, while ethical considerations ensure that AI systems are designed with societal values in mind. By integrating knowledge from different domains, researchers can create AI systems that are not only more powerful but also more aligned with human needs and values.
In addition to interdisciplinary collaboration, international cooperation plays a significant role in the compounding benefits of AI research. As AI challenges are global in nature, solutions often require input from diverse cultural and regulatory perspectives. International partnerships facilitate the exchange of ideas and best practices, enabling researchers to address issues such as bias, privacy, and security more effectively. Furthermore, these collaborations can lead to the establishment of global standards and frameworks that ensure the responsible development and deployment of AI technologies.
The positive feedback loop of AI research is also fueled by the increasing availability of computational resources. Cloud computing and high-performance computing infrastructures have made it possible for researchers to conduct experiments at a scale that was previously unimaginable. This access to powerful computational tools allows for the testing and validation of complex AI models, accelerating the pace of discovery and innovation. As a result, researchers can tackle more ambitious projects, further contributing to the cycle of advancement.
Finally, the compounding benefits of collaborative AI research are evident in the real-world applications that emerge from these efforts. From healthcare and education to transportation and environmental sustainability, AI technologies are being deployed to address some of the most pressing challenges facing society today. The success of these applications not only demonstrates the potential of AI to drive positive change but also inspires further investment and interest in the field. This, in turn, attracts new talent and resources, perpetuating the cycle of growth and innovation.
In conclusion, the collaborative nature of AI research has created a positive feedback loop that continues to propel the field forward. Through the open sharing of knowledge, interdisciplinary and international partnerships, and access to advanced computational resources, researchers are able to achieve breakthroughs that benefit society as a whole. As this cycle of collaboration and innovation continues, the potential for AI to transform our world becomes increasingly within reach.
Feedback Loops in AI: Driving Efficiency and Accuracy
The field of artificial intelligence (AI) has witnessed remarkable advancements over the past few decades, largely driven by the concept of feedback loops. These loops, integral to the development and refinement of AI systems, play a crucial role in enhancing both efficiency and accuracy. At the core of AI research, feedback loops facilitate continuous learning and adaptation, enabling systems to improve their performance over time. By examining the mechanisms of these loops, one can appreciate their significance in propelling AI technologies forward.
To begin with, feedback loops in AI are processes where the output of a system is fed back into the system as input, creating a cycle of continuous improvement. This iterative process allows AI models to learn from their mistakes and successes, refining their algorithms to produce more accurate results. For instance, in supervised learning, a common approach in AI, models are trained on labeled data. As they make predictions, the discrepancies between predicted and actual outcomes are used to adjust the model’s parameters. This adjustment process, repeated over numerous iterations, enhances the model’s predictive accuracy.
Moreover, feedback loops are not limited to supervised learning. They are also pivotal in reinforcement learning, where agents learn optimal behaviors through trial and error interactions with their environment. In this context, feedback loops manifest as reward signals that guide the agent’s learning process. Positive rewards reinforce successful actions, while negative feedback discourages undesirable behaviors. Over time, the agent develops strategies that maximize cumulative rewards, demonstrating the power of feedback loops in shaping intelligent behavior.
In addition to improving accuracy, feedback loops contribute significantly to the efficiency of AI systems. By continuously refining algorithms, these loops reduce the computational resources required for training and inference. This efficiency is particularly evident in the development of neural networks, where feedback loops help in optimizing network architectures and hyperparameters. Techniques such as backpropagation leverage feedback loops to minimize errors in neural networks, ensuring that models converge faster and with greater precision.
Furthermore, feedback loops extend beyond individual AI models to encompass entire research ecosystems. The collaborative nature of AI research fosters a global feedback loop, where researchers build upon each other’s work, share insights, and refine methodologies. This collective effort accelerates the pace of innovation, as breakthroughs in one area often lead to advancements in others. For example, improvements in natural language processing can enhance machine translation systems, which in turn can benefit from advancements in computer vision for multimodal applications.
As AI systems become more sophisticated, the importance of feedback loops continues to grow. They are essential for addressing challenges such as bias and fairness, as they enable models to learn from diverse datasets and adapt to changing conditions. By incorporating feedback from real-world deployments, AI systems can become more robust and equitable, ensuring that they serve a broader range of users effectively.
In conclusion, the positive feedback loop of AI research is a driving force behind the field’s rapid progress. By facilitating continuous learning and adaptation, feedback loops enhance the efficiency and accuracy of AI systems. They not only optimize individual models but also contribute to the collective advancement of AI technologies. As researchers continue to explore and refine these loops, the potential for AI to transform industries and improve lives becomes increasingly attainable. Through the lens of feedback loops, one can appreciate the dynamic and evolving nature of AI research, underscoring its pivotal role in shaping the future.
The Future of AI: Harnessing Positive Feedback for Growth
The field of artificial intelligence (AI) is experiencing an unprecedented era of growth, driven by a positive feedback loop that continually accelerates advancements and applications. This phenomenon is characterized by the interplay between technological progress, increased investment, and expanding applications, each reinforcing the other in a cycle of continuous improvement. As AI technologies become more sophisticated, they attract greater interest and investment, which in turn fuels further research and development. This cycle not only propels the field forward but also broadens the scope of AI’s impact across various sectors.
To begin with, technological advancements in AI have been remarkable, with breakthroughs in machine learning, natural language processing, and computer vision leading the charge. These innovations have enabled AI systems to perform tasks with increasing accuracy and efficiency, making them more appealing to businesses and researchers alike. As AI systems demonstrate their potential to solve complex problems, they attract more funding from both public and private sectors. This influx of resources allows for more extensive research and experimentation, leading to further technological advancements. Consequently, the cycle of innovation and investment continues to gain momentum.
Moreover, the expanding applications of AI across diverse industries further contribute to this positive feedback loop. In healthcare, for instance, AI is being used to enhance diagnostic accuracy, personalize treatment plans, and streamline administrative processes. In finance, AI algorithms are employed to detect fraudulent activities, optimize trading strategies, and improve customer service. The automotive industry is leveraging AI to develop autonomous vehicles, while the retail sector uses it to enhance customer experiences through personalized recommendations and efficient inventory management. As AI proves its value in these and other areas, more industries are motivated to explore its potential, leading to a broader adoption of AI technologies.
This widespread adoption, in turn, generates a wealth of data that is crucial for the continued development of AI systems. The more data available, the better AI models can be trained to understand and predict complex patterns. This data-driven approach not only improves the performance of existing AI systems but also opens up new avenues for research and innovation. As AI systems become more adept at handling large datasets, they can tackle increasingly complex challenges, further demonstrating their value and attracting additional investment.
Furthermore, the positive feedback loop of AI research is bolstered by the collaborative nature of the global research community. Researchers from academia, industry, and government institutions are increasingly working together to share knowledge, resources, and expertise. This collaboration accelerates the pace of innovation and ensures that advancements in AI are disseminated widely, benefiting a broader range of applications and industries. As a result, the collective efforts of the research community contribute to the continuous growth and evolution of AI technologies.
In conclusion, the positive feedback loop of AI research is a powerful driver of growth and innovation in the field. The interplay between technological advancements, increased investment, and expanding applications creates a self-reinforcing cycle that propels AI forward. As AI continues to demonstrate its potential across various sectors, it attracts more resources and attention, leading to further advancements and applications. This cycle not only ensures the continued evolution of AI technologies but also expands their impact, paving the way for a future where AI plays an increasingly integral role in society.
Q&A
1. **What is a positive feedback loop in AI research?**
A positive feedback loop in AI research refers to the cycle where advancements in AI technology lead to improved tools and methods, which in turn accelerate further research and development in the field.
2. **How does increased computational power contribute to the positive feedback loop?**
Increased computational power allows researchers to train more complex models and process larger datasets, leading to breakthroughs that further enhance AI capabilities and research efficiency.
3. **What role does data availability play in the positive feedback loop of AI research?**
Greater data availability enables the training of more accurate and robust AI models, which can lead to new applications and insights, driving further data collection and research efforts.
4. **How do improved AI models impact research productivity?**
Improved AI models can automate and optimize various research tasks, increasing productivity and allowing researchers to focus on more complex problems, thus accelerating the pace of innovation.
5. **What is the effect of AI research advancements on industry applications?**
Advancements in AI research lead to more effective and efficient industry applications, which can generate economic benefits and further investment in AI research and development.
6. **How does collaboration between academia and industry influence the positive feedback loop?**
Collaboration between academia and industry facilitates the exchange of knowledge and resources, leading to faster innovation and the practical application of research findings, which in turn fuels further research efforts.The positive feedback loop of AI research refers to the self-reinforcing cycle where advancements in AI technology lead to improved tools and methodologies, which in turn accelerate further research and development. As AI systems become more sophisticated, they enhance data analysis, optimize research processes, and enable the creation of more powerful algorithms. This progress attracts increased investment and talent, fostering a thriving ecosystem that propels innovation. Consequently, the rapid evolution of AI capabilities continues to drive breakthroughs across various fields, creating a dynamic and exponential growth pattern in AI research and its applications.
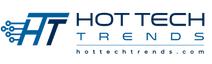