The Proactive Alert System for Emerging AI Threats is an advanced monitoring and response framework designed to identify, assess, and mitigate potential risks associated with the rapid development and deployment of artificial intelligence technologies. As AI systems become increasingly integrated into critical sectors such as finance, healthcare, and national security, the potential for misuse, unintended consequences, and vulnerabilities grows. This system leverages real-time data analytics, machine learning algorithms, and threat intelligence to provide early warnings and actionable insights to stakeholders. By proactively addressing emerging threats, the system aims to safeguard against malicious activities, ensure compliance with ethical standards, and maintain public trust in AI innovations.
Understanding Proactive Alert Systems in AI Security
In the rapidly evolving landscape of artificial intelligence, the need for robust security measures has become increasingly paramount. As AI systems become more integrated into critical sectors such as healthcare, finance, and national security, the potential threats they pose cannot be underestimated. Consequently, the development of proactive alert systems for emerging AI threats has emerged as a crucial component in safeguarding these technologies. These systems are designed to anticipate, identify, and mitigate potential risks before they can cause significant harm, thereby ensuring the safe and secure deployment of AI applications.
To understand the significance of proactive alert systems in AI security, it is essential to first recognize the nature of the threats they are designed to counteract. AI systems, by their very nature, are susceptible to a range of vulnerabilities, including adversarial attacks, data poisoning, and model inversion. Adversarial attacks, for instance, involve the manipulation of input data to deceive AI models into making incorrect predictions or classifications. Data poisoning, on the other hand, entails the introduction of malicious data into the training set, thereby compromising the integrity of the model. Model inversion attacks aim to extract sensitive information from the AI system, posing significant privacy concerns. These threats underscore the necessity for proactive measures that can detect and neutralize such risks in real-time.
Proactive alert systems operate by continuously monitoring AI systems for signs of anomalous behavior or potential vulnerabilities. By employing advanced techniques such as machine learning and anomaly detection, these systems can identify patterns indicative of an impending threat. For instance, a sudden deviation in the model’s performance or an unexpected change in data input patterns may trigger an alert, prompting further investigation. This continuous monitoring allows for the early detection of threats, enabling organizations to respond swiftly and effectively.
Moreover, the integration of proactive alert systems into AI security frameworks offers several advantages. Firstly, these systems enhance the resilience of AI applications by providing an additional layer of defense against potential attacks. By identifying threats at an early stage, organizations can implement countermeasures to prevent or mitigate the impact of an attack. Secondly, proactive alert systems contribute to the overall trustworthiness of AI technologies. As users become increasingly aware of the potential risks associated with AI, demonstrating a commitment to security through proactive measures can enhance user confidence and acceptance.
Furthermore, the implementation of proactive alert systems necessitates a collaborative approach involving various stakeholders, including AI developers, cybersecurity experts, and policymakers. By fostering collaboration, organizations can leverage diverse expertise to develop comprehensive security strategies that address the multifaceted nature of AI threats. This collaborative effort is essential in ensuring that proactive alert systems remain effective in the face of evolving threats and technological advancements.
In conclusion, the development and deployment of proactive alert systems for emerging AI threats represent a critical step in enhancing the security and reliability of AI technologies. By anticipating and addressing potential risks before they materialize, these systems play a vital role in safeguarding AI applications across various sectors. As AI continues to advance and permeate different aspects of society, the importance of proactive alert systems in AI security will only continue to grow. Through continuous monitoring, early threat detection, and collaborative efforts, these systems can help ensure that AI technologies are deployed safely and responsibly, ultimately contributing to a more secure and trustworthy digital future.
Key Features of Effective AI Threat Detection
In the rapidly evolving landscape of artificial intelligence, the development of a proactive alert system for emerging AI threats has become a critical necessity. As AI technologies continue to advance, so too do the potential risks associated with their misuse or malfunction. Therefore, an effective AI threat detection system must incorporate several key features to ensure it can identify and mitigate these risks promptly and efficiently.
To begin with, one of the most crucial features of an effective AI threat detection system is real-time monitoring. This capability allows the system to continuously analyze data and detect anomalies as they occur. By leveraging advanced algorithms and machine learning techniques, the system can identify patterns that deviate from the norm, signaling potential threats. This real-time analysis is essential for minimizing response times and preventing threats from escalating into more significant issues.
In addition to real-time monitoring, adaptability is another vital feature. The dynamic nature of AI threats means that detection systems must be able to evolve alongside emerging technologies and tactics. This adaptability can be achieved through continuous learning mechanisms that enable the system to update its threat models based on new data and insights. By doing so, the system remains relevant and effective in identifying novel threats that may not have been previously encountered.
Furthermore, an effective AI threat detection system should incorporate a comprehensive data integration strategy. This involves aggregating data from various sources, including network traffic, user behavior, and system logs, to provide a holistic view of the environment. By correlating information from multiple channels, the system can gain a deeper understanding of potential threats and their origins. This comprehensive approach not only enhances the accuracy of threat detection but also aids in the development of more targeted and effective mitigation strategies.
Moreover, transparency and explainability are essential features that should not be overlooked. As AI systems become more complex, it is crucial for threat detection mechanisms to provide clear and understandable insights into their decision-making processes. This transparency helps build trust among stakeholders and ensures that the system’s actions can be audited and verified. Explainability also facilitates collaboration between human operators and AI systems, allowing for more informed decision-making and effective threat response.
Another key feature is the ability to prioritize threats based on their potential impact. Not all threats pose the same level of risk, and an effective detection system should be able to assess and rank threats accordingly. By employing risk assessment algorithms, the system can allocate resources and attention to the most critical threats, ensuring that they are addressed promptly. This prioritization is essential for maintaining the overall security and integrity of AI systems.
Finally, an effective AI threat detection system should include robust communication and alerting mechanisms. Once a threat is identified, the system must be able to notify relevant stakeholders quickly and efficiently. This communication should be clear and actionable, providing detailed information about the nature of the threat and recommended response measures. By facilitating timely and informed responses, these alerting mechanisms play a crucial role in mitigating the impact of potential threats.
In conclusion, the development of a proactive alert system for emerging AI threats requires a multifaceted approach that incorporates real-time monitoring, adaptability, comprehensive data integration, transparency, threat prioritization, and effective communication. By integrating these key features, organizations can enhance their ability to detect and respond to AI threats, safeguarding their systems and ensuring the continued advancement of AI technologies in a secure and responsible manner.
Implementing Proactive Measures Against AI Threats
In the rapidly evolving landscape of artificial intelligence, the potential for both innovation and risk is immense. As AI systems become increasingly sophisticated, the need for a proactive alert system to identify and mitigate emerging threats is paramount. Implementing proactive measures against AI threats requires a multifaceted approach that combines technological, regulatory, and ethical considerations. By anticipating potential risks and developing strategies to address them, stakeholders can ensure that AI technologies are harnessed for the benefit of society while minimizing potential harms.
To begin with, the development of a proactive alert system necessitates a comprehensive understanding of the various types of AI threats. These threats can range from data privacy breaches and algorithmic bias to more severe concerns such as autonomous weaponry and the manipulation of public opinion. By categorizing these threats, organizations can prioritize their efforts and allocate resources effectively. Moreover, it is essential to recognize that AI threats are not static; they evolve alongside technological advancements. Therefore, continuous monitoring and assessment are crucial components of any proactive alert system.
In addition to identifying potential threats, it is vital to establish a robust framework for risk assessment and management. This involves not only technical evaluations of AI systems but also an examination of the broader societal implications. For instance, while an AI application may perform optimally from a technical standpoint, it may still pose ethical dilemmas or exacerbate existing inequalities. Consequently, a holistic approach that incorporates diverse perspectives is necessary to ensure that AI technologies are developed and deployed responsibly.
Furthermore, collaboration between various stakeholders is essential in implementing proactive measures against AI threats. Governments, industry leaders, academic institutions, and civil society organizations must work together to share knowledge, resources, and best practices. By fostering a collaborative environment, stakeholders can develop standardized protocols and guidelines that promote transparency and accountability in AI development. Additionally, international cooperation is crucial, given the global nature of AI technologies and the cross-border implications of potential threats.
Another critical aspect of a proactive alert system is the integration of advanced technologies for threat detection and response. Machine learning algorithms, for example, can be employed to analyze vast amounts of data and identify patterns indicative of emerging threats. By leveraging these technologies, organizations can detect anomalies and respond swiftly to mitigate potential risks. However, it is important to ensure that these systems are themselves transparent and free from bias, as reliance on flawed algorithms could exacerbate the very threats they are designed to prevent.
Moreover, regulatory frameworks play a pivotal role in shaping the development and deployment of AI technologies. Policymakers must strike a balance between fostering innovation and ensuring public safety. This involves crafting regulations that are flexible enough to accommodate technological advancements while providing clear guidelines for ethical AI use. Regular reviews and updates of these regulations are necessary to keep pace with the rapidly changing AI landscape.
In conclusion, implementing proactive measures against AI threats is a complex but essential endeavor. By understanding the nature of these threats, establishing a comprehensive risk management framework, fostering collaboration among stakeholders, integrating advanced technologies, and developing robust regulatory frameworks, society can effectively navigate the challenges posed by AI. Through these efforts, we can harness the transformative potential of AI while safeguarding against its risks, ensuring a future where technology serves the greater good.
The Role of Machine Learning in Proactive Alert Systems
In the rapidly evolving landscape of artificial intelligence, the development of proactive alert systems has become increasingly crucial to mitigate emerging threats. At the heart of these systems lies machine learning, a subset of AI that enables computers to learn from data and make decisions with minimal human intervention. Machine learning plays a pivotal role in enhancing the capabilities of proactive alert systems, allowing them to identify potential threats before they manifest into significant issues. By leveraging vast amounts of data, machine learning algorithms can detect patterns and anomalies that may indicate the presence of a threat, thus providing an early warning system that is both efficient and effective.
To understand the role of machine learning in proactive alert systems, it is essential to consider how these systems operate. Proactive alert systems are designed to continuously monitor data streams, analyzing them in real-time to identify any deviations from the norm. Machine learning algorithms are particularly adept at this task, as they can process large volumes of data at high speeds, making them ideal for applications where time is of the essence. Furthermore, these algorithms can be trained to recognize specific patterns associated with known threats, as well as to adapt to new and evolving threats by learning from new data inputs.
One of the key advantages of using machine learning in proactive alert systems is its ability to improve over time. As more data is collected and analyzed, machine learning models can refine their predictions, becoming more accurate and reliable. This continuous learning process is vital in the context of AI threats, which are often dynamic and unpredictable. By constantly updating their models, proactive alert systems can stay ahead of potential threats, providing organizations with the information they need to take preemptive action.
Moreover, machine learning enables proactive alert systems to prioritize alerts based on the severity and likelihood of a threat. This is achieved through the use of sophisticated algorithms that assess the risk associated with each detected anomaly. By assigning a risk score to each potential threat, these systems can help organizations focus their resources on the most pressing issues, thereby enhancing their overall security posture. This prioritization is particularly important in environments where resources are limited, and the cost of responding to false positives can be high.
In addition to improving threat detection and prioritization, machine learning also facilitates the integration of proactive alert systems with other security measures. By providing a comprehensive view of the threat landscape, these systems can inform and enhance other security protocols, such as intrusion detection systems and firewalls. This integration creates a multi-layered defense strategy that is more resilient to sophisticated attacks, thereby reducing the likelihood of a successful breach.
In conclusion, the role of machine learning in proactive alert systems is indispensable in the fight against emerging AI threats. By harnessing the power of machine learning, these systems can detect, prioritize, and respond to potential threats with unprecedented speed and accuracy. As AI continues to advance, the importance of proactive alert systems will only grow, making the integration of machine learning technologies a critical component of any robust security strategy. Through continuous learning and adaptation, machine learning ensures that proactive alert systems remain at the forefront of threat detection, safeguarding organizations against the ever-evolving landscape of AI threats.
Case Studies: Successful Proactive Alert Systems in AI
In recent years, the rapid advancement of artificial intelligence has brought about significant benefits across various sectors, from healthcare to finance. However, with these advancements come potential threats that necessitate the development of proactive alert systems to mitigate risks associated with AI technologies. Several case studies highlight the successful implementation of such systems, demonstrating their effectiveness in identifying and addressing emerging AI threats before they escalate into significant issues.
One notable example is the proactive alert system developed by a leading cybersecurity firm to monitor AI-driven cyber threats. This system employs machine learning algorithms to analyze vast amounts of data in real-time, identifying patterns and anomalies that may indicate a potential cyber attack. By continuously learning from new data, the system can adapt to evolving threats, providing early warnings to cybersecurity teams. This proactive approach has proven instrumental in preventing data breaches and minimizing the impact of cyber attacks on organizations.
Transitioning to another sector, the financial industry has also benefited from proactive alert systems designed to detect fraudulent activities. Financial institutions have implemented AI-driven systems that monitor transactions for unusual patterns indicative of fraud. These systems utilize advanced analytics to assess the risk associated with each transaction, flagging suspicious activities for further investigation. By alerting financial institutions to potential fraud in real-time, these systems have significantly reduced financial losses and enhanced the security of financial transactions.
In the healthcare sector, proactive alert systems have been employed to improve patient safety and care. Hospitals and healthcare providers have integrated AI systems that monitor patient data for signs of deterioration or potential complications. For instance, these systems can analyze vital signs, lab results, and other clinical data to predict the likelihood of adverse events, such as sepsis or cardiac arrest. By providing early warnings to healthcare professionals, these systems enable timely interventions, ultimately improving patient outcomes and reducing the burden on healthcare resources.
Moreover, the transportation industry has seen the successful implementation of proactive alert systems in autonomous vehicles. These systems are designed to detect and respond to potential hazards on the road, such as pedestrians, other vehicles, or obstacles. By continuously analyzing data from sensors and cameras, the AI systems can anticipate and react to potential threats, ensuring the safety of passengers and other road users. This proactive approach has been crucial in advancing the development and deployment of autonomous vehicles, addressing safety concerns and building public trust in this emerging technology.
Furthermore, proactive alert systems have been instrumental in addressing ethical concerns related to AI technologies. For instance, AI systems used in hiring processes have been scrutinized for potential biases. To address this, companies have developed alert systems that monitor AI decision-making processes for signs of bias or discrimination. By identifying and rectifying these issues early, organizations can ensure fair and unbiased outcomes, promoting ethical AI practices.
In conclusion, the successful implementation of proactive alert systems across various sectors underscores their importance in mitigating emerging AI threats. By leveraging advanced technologies and continuous learning, these systems provide early warnings and enable timely interventions, safeguarding against potential risks. As AI continues to evolve, the development and refinement of proactive alert systems will be crucial in ensuring the safe and ethical deployment of AI technologies, ultimately fostering trust and confidence in their use.
Future Trends in Proactive AI Threat Management
In the rapidly evolving landscape of artificial intelligence, the emergence of new threats necessitates a proactive approach to threat management. As AI systems become increasingly integrated into critical infrastructure, the potential for misuse or unintended consequences grows. This has led to the development of proactive alert systems designed to identify and mitigate emerging AI threats before they can cause significant harm. These systems are becoming an essential component of future AI threat management strategies, offering a promising solution to the challenges posed by advanced AI technologies.
One of the key trends in proactive AI threat management is the integration of machine learning algorithms that can predict potential threats based on historical data and current trends. By analyzing vast amounts of data, these algorithms can identify patterns and anomalies that may indicate the presence of a threat. This predictive capability allows organizations to take preemptive action, thereby reducing the risk of an AI-related incident. Moreover, the continuous improvement of these algorithms through machine learning ensures that they remain effective even as new threats emerge.
In addition to predictive analytics, the use of real-time monitoring systems is becoming increasingly prevalent. These systems provide continuous oversight of AI operations, enabling the rapid detection of any deviations from expected behavior. By employing sophisticated sensors and data collection techniques, real-time monitoring systems can quickly identify potential threats and alert relevant stakeholders. This immediate response capability is crucial in minimizing the impact of any AI-related incidents, as it allows for swift intervention and resolution.
Furthermore, the development of collaborative frameworks for threat intelligence sharing is another significant trend in proactive AI threat management. By fostering cooperation between organizations, industries, and governments, these frameworks facilitate the exchange of information regarding emerging threats and best practices for mitigation. This collective approach not only enhances the overall effectiveness of threat management efforts but also helps to build a more resilient AI ecosystem. As a result, organizations are better equipped to anticipate and respond to potential threats, thereby safeguarding their operations and assets.
Another important aspect of proactive AI threat management is the implementation of robust governance and regulatory frameworks. These frameworks establish clear guidelines and standards for the development and deployment of AI systems, ensuring that they are designed with security and ethical considerations in mind. By promoting transparency and accountability, governance frameworks help to mitigate the risks associated with AI technologies and foster public trust in their use. As AI continues to advance, the importance of strong governance and regulatory measures will only increase, making them a critical component of any comprehensive threat management strategy.
Finally, the role of human oversight in proactive AI threat management cannot be overstated. While automated systems and algorithms play a crucial role in identifying and mitigating threats, human expertise is essential in interpreting data, making informed decisions, and implementing effective solutions. By combining the strengths of both human and machine intelligence, organizations can create a more robust and adaptive threat management system that is capable of addressing the complex challenges posed by emerging AI threats.
In conclusion, the future of proactive AI threat management lies in the integration of advanced technologies, collaborative frameworks, and strong governance measures. By adopting a proactive approach, organizations can effectively anticipate and mitigate emerging threats, ensuring the safe and responsible use of AI technologies. As the field of artificial intelligence continues to evolve, the development and implementation of proactive alert systems will be essential in safeguarding against potential risks and maximizing the benefits of AI innovations.
Q&A
1. **What is a Proactive Alert System for Emerging AI Threats?**
A Proactive Alert System for Emerging AI Threats is a framework designed to identify, monitor, and alert stakeholders about potential risks and threats posed by AI technologies before they manifest into significant issues.
2. **How does the system identify emerging AI threats?**
The system uses advanced algorithms, machine learning models, and data analytics to continuously scan and analyze data from various sources, including research papers, news articles, and cybersecurity reports, to detect patterns indicative of emerging AI threats.
3. **What are the key components of a Proactive Alert System?**
Key components include threat detection algorithms, real-time data monitoring, a comprehensive database of known threats, a user-friendly interface for alerts, and integration capabilities with existing security infrastructure.
4. **Who benefits from using a Proactive Alert System for AI threats?**
Organizations across various sectors, including cybersecurity firms, government agencies, tech companies, and any entity relying on AI technologies, benefit by staying informed and prepared against potential AI-related risks.
5. **What types of threats can the system detect?**
The system can detect a range of threats, including AI model vulnerabilities, adversarial attacks, data poisoning, unauthorized AI usage, and ethical concerns related to AI deployment.
6. **How does the system alert stakeholders about threats?**
The system sends real-time alerts through various channels such as emails, SMS, or integrated dashboards, providing detailed information about the threat, its potential impact, and recommended mitigation strategies.A Proactive Alert System for Emerging AI Threats is essential in today’s rapidly evolving technological landscape to ensure the safe and ethical deployment of artificial intelligence. Such a system would continuously monitor AI developments, identify potential risks, and provide timely alerts to stakeholders, enabling them to take preventive measures. By leveraging advanced analytics, machine learning, and real-time data processing, the system can detect anomalies and predict threats before they materialize. This proactive approach not only mitigates risks associated with AI but also fosters trust and transparency in AI applications. Ultimately, a well-designed alert system is a critical component in safeguarding against the unintended consequences of AI, ensuring that its benefits are maximized while minimizing potential harms.
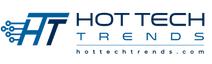