Massachusetts Institute of Technology (MIT) has announced a groundbreaking advancement in the field of artificial intelligence with the development of a novel method for training reliable AI agents. This innovative approach addresses longstanding challenges in AI reliability and performance, promising to enhance the dependability of AI systems across various applications. By integrating cutting-edge techniques and rigorous testing protocols, MIT’s new method aims to ensure that AI agents can operate with greater accuracy and consistency, paving the way for more trustworthy and efficient AI solutions in industries ranging from healthcare to autonomous vehicles. This breakthrough represents a significant step forward in the quest to create AI systems that can be relied upon to perform critical tasks with minimal risk of error.
Understanding MIT’s Breakthrough in AI Training Methods
In recent years, the field of artificial intelligence has witnessed remarkable advancements, yet the challenge of training reliable AI agents remains a significant hurdle. MIT’s latest breakthrough in AI training methods promises to address this issue, offering a novel approach that could redefine the landscape of AI development. This innovative method focuses on enhancing the reliability and robustness of AI agents, ensuring they perform consistently across a wide range of scenarios.
Traditionally, AI training has relied heavily on large datasets and complex algorithms to teach machines how to interpret and respond to various inputs. However, this approach often results in AI systems that are highly specialized and lack the flexibility to adapt to new or unforeseen situations. Consequently, these systems can exhibit unpredictable behavior when faced with scenarios outside their training data. Recognizing this limitation, researchers at MIT have developed a new training paradigm that emphasizes adaptability and generalization.
Central to MIT’s breakthrough is the concept of “meta-learning,” a process that enables AI agents to learn how to learn. By focusing on the underlying principles of learning rather than specific tasks, meta-learning equips AI systems with the ability to quickly adapt to new environments and challenges. This approach mimics the human capacity for learning, where individuals can apply knowledge from one domain to another, seemingly unrelated, context. As a result, AI agents trained using meta-learning techniques demonstrate a higher degree of reliability and versatility.
Moreover, MIT’s method incorporates a robust feedback mechanism that continuously evaluates the performance of AI agents. This feedback loop allows the system to identify and rectify errors in real-time, thereby enhancing the overall accuracy and dependability of the AI. By integrating this dynamic feedback process, MIT’s approach ensures that AI agents are not only capable of learning from their mistakes but also improving their decision-making processes over time.
In addition to meta-learning and feedback integration, MIT’s breakthrough also leverages advanced simulation environments to train AI agents. These virtual environments provide a safe and controlled setting for AI systems to explore and experiment with different strategies without the risk of real-world consequences. By exposing AI agents to a diverse array of scenarios within these simulations, researchers can better prepare them for the complexities and unpredictabilities of real-world applications.
Furthermore, MIT’s method emphasizes the importance of collaboration between AI agents and human operators. By fostering a symbiotic relationship between humans and machines, this approach ensures that AI systems remain aligned with human values and objectives. This collaborative framework not only enhances the reliability of AI agents but also builds trust between humans and machines, a crucial factor in the widespread adoption of AI technologies.
In conclusion, MIT’s breakthrough method for training reliable AI agents represents a significant step forward in the quest for more adaptable and trustworthy AI systems. By incorporating meta-learning, dynamic feedback, advanced simulations, and human collaboration, this innovative approach addresses many of the limitations associated with traditional AI training methods. As AI continues to play an increasingly integral role in various sectors, from healthcare to autonomous vehicles, the importance of developing reliable and robust AI agents cannot be overstated. MIT’s pioneering work in this area not only advances the field of artificial intelligence but also paves the way for a future where AI systems can be trusted to operate safely and effectively in diverse and dynamic environments.
The Impact of MIT’s New AI Training Techniques on Industry
The Massachusetts Institute of Technology (MIT) has recently unveiled a groundbreaking method for training artificial intelligence (AI) agents, a development poised to significantly impact various industries. This innovative approach addresses longstanding challenges in AI reliability and efficiency, promising to enhance the performance of AI systems across multiple sectors. As industries increasingly rely on AI for critical operations, the need for dependable and robust AI agents has never been more pressing. MIT’s new training techniques offer a solution that could redefine how businesses integrate AI into their processes.
At the core of MIT’s breakthrough is a novel algorithm that enhances the learning capabilities of AI agents. Traditional AI training methods often involve extensive trial and error, requiring vast amounts of data and computational resources. However, MIT’s approach streamlines this process by enabling AI agents to learn more effectively from smaller datasets. This efficiency not only reduces the time and cost associated with training AI systems but also minimizes the environmental impact of energy-intensive computational processes. Consequently, industries can deploy AI solutions more rapidly and sustainably, aligning with global efforts to reduce carbon footprints.
Moreover, MIT’s method emphasizes the reliability of AI agents, a critical factor for industries where precision and accuracy are paramount. By incorporating advanced error-correction mechanisms, the new training techniques ensure that AI systems can adapt to unexpected scenarios and maintain high performance levels. This reliability is particularly beneficial in sectors such as healthcare, finance, and autonomous vehicles, where AI-driven decisions can have significant consequences. For instance, in healthcare, AI agents trained using MIT’s methods could improve diagnostic accuracy and patient outcomes, while in finance, they could enhance risk assessment and fraud detection.
In addition to improving reliability, MIT’s training techniques also foster greater transparency in AI decision-making processes. By enabling AI agents to provide clear explanations for their actions, these methods address one of the major concerns surrounding AI adoption: the “black box” problem. This transparency is crucial for building trust between AI systems and human users, facilitating smoother integration of AI into existing workflows. As a result, industries can leverage AI technologies with greater confidence, knowing that they can understand and verify the decisions made by these systems.
Furthermore, the implications of MIT’s breakthrough extend beyond individual industries, potentially influencing the broader AI research community. By setting a new standard for AI training, MIT’s methods could inspire further innovations and collaborations among researchers and institutions worldwide. This collective effort could accelerate the development of even more advanced AI technologies, driving progress across various fields and contributing to the global knowledge economy.
In conclusion, MIT’s unveiling of a breakthrough method for training reliable AI agents marks a significant milestone in the evolution of artificial intelligence. By addressing key challenges related to efficiency, reliability, and transparency, these new techniques have the potential to transform how industries utilize AI. As businesses increasingly integrate AI into their operations, MIT’s innovations offer a promising path forward, ensuring that AI systems are not only powerful but also trustworthy and sustainable. As the impact of these advancements unfolds, it is clear that MIT’s contributions will play a pivotal role in shaping the future of AI and its applications across diverse sectors.
How MIT’s Reliable AI Agents Could Transform Technology
MIT’s recent unveiling of a breakthrough method for training reliable AI agents marks a significant milestone in the field of artificial intelligence, promising to transform technology in profound ways. This innovative approach addresses one of the most pressing challenges in AI development: ensuring that AI systems are not only powerful but also trustworthy and dependable. As AI continues to permeate various sectors, from healthcare to finance, the need for reliable AI agents has never been more critical. MIT’s method, therefore, holds the potential to reshape the landscape of technology by enhancing the reliability and safety of AI applications.
The core of MIT’s breakthrough lies in its novel training framework, which emphasizes robustness and transparency. Traditional AI models often operate as black boxes, making it difficult to predict their behavior in unfamiliar situations. MIT’s approach, however, integrates advanced techniques that allow AI agents to explain their decision-making processes. This transparency is crucial for building trust, as it enables users to understand and verify the actions of AI systems. By fostering a deeper understanding of AI behavior, MIT’s method reduces the risk of unexpected outcomes, thereby enhancing the reliability of AI applications.
Moreover, MIT’s training method incorporates rigorous testing protocols that simulate a wide range of scenarios. This comprehensive testing ensures that AI agents can perform consistently across diverse environments, a feature that is particularly important in critical applications such as autonomous vehicles and medical diagnostics. By exposing AI systems to varied conditions during the training phase, MIT’s approach prepares them to handle real-world challenges with greater competence and reliability. Consequently, this method not only improves the performance of AI agents but also mitigates the risks associated with their deployment in high-stakes settings.
In addition to robustness and transparency, MIT’s method emphasizes ethical considerations in AI development. The framework includes mechanisms for identifying and mitigating biases, which are often inadvertently embedded in AI systems due to skewed training data. By addressing these biases, MIT’s approach ensures that AI agents make fair and equitable decisions, thereby promoting ethical standards in AI applications. This focus on ethics is essential for fostering public confidence in AI technologies, as it aligns AI development with societal values and expectations.
The implications of MIT’s breakthrough extend beyond individual AI applications, potentially influencing the broader technological landscape. As reliable AI agents become more prevalent, industries could experience a paradigm shift in how they operate and innovate. For instance, in healthcare, AI systems trained using MIT’s method could lead to more accurate diagnoses and personalized treatment plans, ultimately improving patient outcomes. In finance, reliable AI agents could enhance risk assessment and fraud detection, contributing to more secure and efficient financial systems.
Furthermore, MIT’s method could accelerate the integration of AI into everyday life, making advanced technologies more accessible and user-friendly. As AI agents become more reliable, consumers may be more willing to adopt AI-driven solutions, leading to increased demand and further advancements in AI research and development. This cycle of innovation could drive technological progress across various domains, ultimately transforming how society interacts with and benefits from AI technologies.
In conclusion, MIT’s breakthrough method for training reliable AI agents represents a pivotal advancement in artificial intelligence, with the potential to transform technology in significant ways. By prioritizing robustness, transparency, and ethics, this approach addresses key challenges in AI development, paving the way for more trustworthy and effective AI applications. As industries and consumers alike embrace these reliable AI agents, the technological landscape is poised for a transformative shift, heralding a new era of innovation and progress.
Key Innovations in MIT’s AI Training Approach
MIT has long been at the forefront of artificial intelligence research, and its latest breakthrough in training reliable AI agents marks a significant advancement in the field. This innovative approach addresses some of the most pressing challenges in AI development, particularly the need for systems that can perform consistently and safely in real-world applications. By focusing on enhancing the reliability of AI agents, MIT’s new method promises to pave the way for more trustworthy and effective AI solutions.
One of the key innovations in MIT’s approach is the integration of robust feedback mechanisms during the training process. Traditional AI training often relies heavily on large datasets and complex algorithms to teach agents how to perform specific tasks. However, this method can sometimes lead to unpredictable behavior when the AI encounters scenarios that were not covered during training. To mitigate this issue, MIT researchers have developed a system that continuously monitors the AI’s performance and provides real-time feedback. This feedback loop allows the AI to adjust its actions dynamically, improving its ability to handle unforeseen situations with greater accuracy and reliability.
Moreover, MIT’s method emphasizes the importance of transparency in AI decision-making processes. By incorporating explainability into the training framework, the researchers aim to make AI agents more understandable to human users. This is achieved by designing algorithms that not only perform tasks efficiently but also provide clear rationales for their decisions. As a result, users can gain insights into the AI’s thought process, fostering trust and facilitating better collaboration between humans and machines.
In addition to transparency, the new training approach also prioritizes ethical considerations. MIT has implemented guidelines to ensure that AI agents are trained with a strong ethical foundation, minimizing biases and promoting fairness. This is particularly crucial in applications where AI systems have a direct impact on human lives, such as healthcare, finance, and law enforcement. By embedding ethical principles into the core of AI training, MIT aims to create agents that not only excel in technical performance but also adhere to societal values.
Furthermore, the researchers have introduced a novel technique for simulating diverse environments during training. This method exposes AI agents to a wide range of scenarios, enabling them to develop a more comprehensive understanding of the world. By simulating various conditions and challenges, the AI can learn to adapt its strategies and improve its problem-solving capabilities. This diversity in training environments is essential for preparing AI agents to operate effectively in the complex and ever-changing real world.
Another significant aspect of MIT’s breakthrough is the focus on collaboration between AI agents and human experts. The training approach encourages AI systems to work alongside humans, leveraging their expertise to enhance decision-making processes. This collaborative model not only improves the AI’s performance but also empowers human users by providing them with advanced tools to augment their capabilities. By fostering a symbiotic relationship between humans and AI, MIT’s method aims to unlock new possibilities for innovation and productivity.
In conclusion, MIT’s breakthrough method for training reliable AI agents represents a major step forward in the development of trustworthy and effective artificial intelligence systems. By integrating robust feedback mechanisms, promoting transparency, prioritizing ethical considerations, simulating diverse environments, and encouraging human-AI collaboration, this approach addresses key challenges in the field. As AI continues to play an increasingly important role in society, MIT’s innovations offer a promising path toward creating systems that are not only technically proficient but also aligned with human values and needs.
Comparing Traditional AI Training with MIT’s New Method
In recent years, the field of artificial intelligence has witnessed remarkable advancements, yet the quest for developing reliable AI agents remains a formidable challenge. Traditional AI training methods, which have been the cornerstone of AI development, often rely on vast datasets and extensive computational resources. These methods typically involve supervised learning, where AI models are trained on labeled data, or reinforcement learning, where agents learn through trial and error by interacting with their environment. While these approaches have yielded impressive results, they are not without limitations. Issues such as overfitting, where models perform well on training data but poorly on unseen data, and the requirement for large amounts of labeled data, which can be both time-consuming and costly to obtain, have prompted researchers to seek more efficient and reliable training methodologies.
In this context, MIT’s recent unveiling of a breakthrough method for training AI agents marks a significant milestone. This novel approach, which diverges from traditional paradigms, emphasizes the importance of robustness and adaptability in AI systems. Unlike conventional methods that often focus on optimizing performance metrics, MIT’s method prioritizes the development of AI agents that can generalize well across diverse scenarios. This is achieved through a combination of innovative techniques that reduce the dependency on large datasets and enhance the agents’ ability to learn from limited information.
One of the key differentiators of MIT’s method is its emphasis on unsupervised and self-supervised learning. By leveraging these techniques, AI agents can learn from unstructured data without the need for explicit labels. This not only alleviates the burden of data labeling but also enables the agents to discover patterns and relationships within the data autonomously. Furthermore, MIT’s approach incorporates elements of transfer learning, allowing AI agents to apply knowledge gained from one domain to another, thereby improving their adaptability and efficiency.
Another critical aspect of MIT’s method is its focus on interpretability and transparency. Traditional AI models, particularly deep learning systems, are often criticized for being “black boxes” due to their complex and opaque decision-making processes. MIT addresses this concern by integrating explainability into the training process, ensuring that AI agents can provide insights into their reasoning and decision-making. This not only enhances trust in AI systems but also facilitates their deployment in sensitive applications where accountability is paramount.
Moreover, MIT’s method introduces a novel framework for continuous learning, enabling AI agents to evolve and improve over time. This is particularly advantageous in dynamic environments where conditions and requirements may change rapidly. By incorporating mechanisms for ongoing learning and adaptation, MIT’s approach ensures that AI agents remain relevant and effective in the face of new challenges.
In conclusion, while traditional AI training methods have laid the groundwork for the development of intelligent systems, MIT’s breakthrough method offers a promising alternative that addresses many of the limitations associated with conventional approaches. By prioritizing robustness, adaptability, and interpretability, MIT’s method paves the way for the creation of more reliable AI agents capable of operating effectively in a wide range of environments. As the field of artificial intelligence continues to evolve, such innovative training methodologies will undoubtedly play a crucial role in shaping the future of AI technology.
Future Implications of MIT’s AI Training Breakthrough
The recent announcement from the Massachusetts Institute of Technology (MIT) regarding their breakthrough method for training reliable AI agents has sparked considerable interest in the field of artificial intelligence. This development holds significant promise for the future, as it addresses one of the most pressing challenges in AI: ensuring that these systems can be trusted to perform consistently and safely in real-world applications. As AI continues to integrate into various sectors, from healthcare to autonomous vehicles, the reliability of these systems becomes paramount. MIT’s innovative approach could potentially redefine how AI agents are developed and deployed, offering a new standard for reliability and performance.
At the core of MIT’s breakthrough is a novel training methodology that emphasizes robustness and adaptability. Traditional AI training methods often rely on vast amounts of data to teach systems how to recognize patterns and make decisions. However, these methods can fall short when AI agents encounter scenarios that differ from their training data. MIT’s approach, in contrast, focuses on equipping AI agents with the ability to generalize from limited data and adapt to new situations. This is achieved through a combination of advanced algorithms and a unique training environment that simulates a wide range of potential real-world conditions. Consequently, AI agents trained using this method are better prepared to handle unexpected challenges, reducing the risk of errors and enhancing their overall reliability.
Moreover, the implications of this breakthrough extend beyond just improving the performance of AI systems. By fostering greater trust in AI, MIT’s method could accelerate the adoption of these technologies across various industries. For instance, in the healthcare sector, reliable AI agents could assist in diagnosing diseases with greater accuracy, leading to improved patient outcomes. Similarly, in the realm of autonomous vehicles, enhanced AI reliability could pave the way for safer and more efficient transportation systems. As these technologies become more prevalent, the societal benefits could be substantial, ranging from increased productivity to enhanced quality of life.
Furthermore, MIT’s breakthrough also raises important considerations regarding the ethical deployment of AI. As AI systems become more reliable, the potential for their misuse or unintended consequences also grows. It is crucial that the development of these technologies is accompanied by robust ethical guidelines and regulatory frameworks to ensure they are used responsibly. MIT’s work highlights the need for ongoing dialogue between researchers, policymakers, and the public to address these challenges and ensure that the benefits of AI are realized in a manner that aligns with societal values.
In addition to its immediate applications, MIT’s breakthrough could also influence future research directions in the field of AI. By demonstrating the feasibility of training reliable AI agents, this development may inspire further innovations in AI training methodologies and algorithms. Researchers may explore new ways to enhance the adaptability and robustness of AI systems, potentially leading to even more advanced capabilities. As the field continues to evolve, the insights gained from MIT’s work could serve as a foundation for future advancements, driving the next wave of AI innovation.
In conclusion, MIT’s breakthrough method for training reliable AI agents represents a significant milestone in the field of artificial intelligence. By addressing key challenges related to reliability and adaptability, this development has the potential to transform how AI systems are designed and deployed. As these technologies become increasingly integrated into our daily lives, the implications of this breakthrough will likely be far-reaching, shaping the future of AI and its role in society.
Q&A
1. **What is the breakthrough method introduced by MIT for training AI agents?**
MIT has introduced a novel approach that focuses on enhancing the reliability and robustness of AI agents through a combination of advanced machine learning techniques and rigorous testing protocols.
2. **How does this method improve the reliability of AI agents?**
The method improves reliability by incorporating diverse datasets, simulating real-world scenarios, and implementing continuous learning mechanisms that allow AI agents to adapt and respond accurately to new and unforeseen situations.
3. **What are the key components of this training method?**
Key components include the use of synthetic data generation, reinforcement learning frameworks, and a feedback loop system that enables AI agents to learn from their mistakes and improve over time.
4. **What potential applications could benefit from this breakthrough?**
Potential applications include autonomous vehicles, healthcare diagnostics, financial modeling, and any domain where decision-making accuracy and reliability are critical.
5. **What challenges does this method address in AI training?**
This method addresses challenges such as data scarcity, overfitting, and the inability of AI models to generalize well across different environments and tasks.
6. **What are the implications of this breakthrough for the future of AI development?**
The implications include the possibility of creating more trustworthy and dependable AI systems, which could accelerate the integration of AI into critical sectors and enhance human-AI collaboration.The recent breakthrough by MIT in developing a novel method for training reliable AI agents marks a significant advancement in the field of artificial intelligence. By focusing on enhancing the robustness and dependability of AI systems, this method addresses critical challenges related to AI reliability and trustworthiness. The approach likely incorporates innovative techniques in machine learning, data processing, and algorithmic design, ensuring that AI agents can perform consistently across various scenarios and applications. This development not only paves the way for more secure and efficient AI deployment in diverse sectors but also sets a new standard for future research and development in AI technologies. As a result, MIT’s breakthrough holds the potential to accelerate the integration of AI into everyday life, fostering greater confidence in AI-driven solutions.
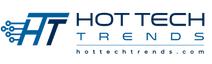