In an era where artificial intelligence plays a pivotal role in decision-making across various sectors, the need for transparency and comprehensibility in AI predictions has never been more critical. “Making AI’s Predictions Understandable: A Guide to Plain Language Explanations” aims to bridge the gap between complex algorithms and user comprehension. This guide provides practical strategies for translating technical jargon into accessible language, empowering users to grasp the rationale behind AI-driven outcomes. By fostering a clearer understanding of AI predictions, we can enhance trust, facilitate informed decision-making, and promote ethical AI practices.
Importance Of Plain Language In AI Predictions
In the rapidly evolving landscape of artificial intelligence, the ability to interpret and understand AI predictions has become increasingly crucial. As AI systems are integrated into various sectors, from healthcare to finance, the complexity of their algorithms often obscures the rationale behind their predictions. This complexity can lead to a significant gap between the technology and its users, resulting in mistrust and misinterpretation. Therefore, the importance of plain language in explaining AI predictions cannot be overstated. By employing clear and accessible language, stakeholders can bridge this gap, fostering a more informed and engaged user base.
First and foremost, plain language serves to demystify the intricate workings of AI systems. Many users, including decision-makers and end-users, may not possess a technical background. Consequently, when faced with complex jargon or convoluted explanations, they may struggle to grasp the implications of AI predictions. This lack of understanding can hinder effective decision-making, as users may be unable to evaluate the reliability or relevance of the predictions presented to them. By utilizing plain language, AI developers can ensure that their explanations are comprehensible, allowing users to make informed choices based on the insights provided.
Moreover, the use of plain language enhances transparency in AI systems. Transparency is a fundamental principle in building trust between AI developers and users. When predictions are articulated in straightforward terms, users can better understand the factors influencing the outcomes. This understanding is particularly vital in high-stakes environments, such as healthcare, where AI predictions can impact patient care and treatment decisions. By clearly outlining the reasoning behind predictions, developers can foster a sense of accountability and reliability, which is essential for user confidence.
In addition to promoting transparency, plain language explanations can facilitate collaboration among interdisciplinary teams. In many cases, AI projects involve professionals from diverse fields, including data scientists, domain experts, and business stakeholders. Each group may possess its own terminology and jargon, which can create barriers to effective communication. By adopting a common language that is accessible to all parties, teams can work more cohesively, ensuring that everyone is aligned on the objectives and implications of the AI predictions. This collaborative approach not only enhances the quality of the project but also leads to more robust and well-rounded outcomes.
Furthermore, the importance of plain language extends to regulatory compliance and ethical considerations. As governments and organizations increasingly scrutinize AI technologies, clear communication becomes essential in demonstrating adherence to ethical standards and regulations. By providing explanations that are easily understood, organizations can better articulate their commitment to responsible AI practices. This transparency not only satisfies regulatory requirements but also reassures users that ethical considerations are at the forefront of AI development.
In conclusion, the significance of plain language in explaining AI predictions is multifaceted. It serves to demystify complex algorithms, enhance transparency, facilitate collaboration, and ensure compliance with ethical standards. As AI continues to permeate various aspects of society, the need for clear and accessible communication will only grow. By prioritizing plain language in their explanations, AI developers can empower users, foster trust, and ultimately contribute to the responsible and effective use of artificial intelligence. In doing so, they will not only enhance user understanding but also pave the way for a more inclusive and informed dialogue surrounding AI technologies.
Techniques For Simplifying AI Explanations
In the rapidly evolving landscape of artificial intelligence, the ability to interpret and understand AI predictions has become increasingly crucial. As AI systems are integrated into various sectors, from healthcare to finance, the need for clear and accessible explanations of their decision-making processes is paramount. Simplifying AI explanations not only enhances user trust but also fosters informed decision-making. To achieve this, several techniques can be employed to distill complex AI outputs into plain language that is easily comprehensible.
One effective technique is the use of analogies. By drawing parallels between AI processes and familiar concepts, practitioners can bridge the gap between technical jargon and everyday understanding. For instance, comparing a neural network to a human brain can help users grasp the idea of interconnected nodes processing information. This method not only makes the explanation relatable but also engages the audience by linking new information to existing knowledge.
Another valuable approach is the implementation of visual aids. Graphs, charts, and infographics can serve as powerful tools to convey complex data in a more digestible format. Visual representations can highlight key trends and relationships that might be obscured in textual explanations. For example, a decision tree diagram can illustrate how an AI model arrives at a particular conclusion, allowing users to visualize the decision-making process step by step. By complementing verbal explanations with visual elements, the overall understanding of AI predictions can be significantly enhanced.
Furthermore, breaking down explanations into smaller, manageable components is essential for clarity. Instead of presenting a comprehensive overview all at once, it is beneficial to dissect the information into bite-sized pieces. This technique allows users to absorb each part of the explanation before moving on to the next, thereby reducing cognitive overload. For instance, when explaining a predictive model, one might first describe the input data, followed by the model’s processing steps, and finally the output results. This sequential approach not only aids comprehension but also encourages users to engage with the material more actively.
In addition to these techniques, employing plain language is critical in making AI explanations accessible. Avoiding technical jargon and using straightforward vocabulary can significantly lower barriers to understanding. It is important to consider the audience’s background and tailor the language accordingly. For instance, when addressing a non-technical audience, it may be more effective to use everyday terms rather than specialized terminology. This practice not only democratizes access to AI insights but also empowers users to make informed decisions based on the information provided.
Moreover, providing context is essential for meaningful interpretation. Users are more likely to understand AI predictions when they are given background information about the model’s purpose and the significance of its outputs. By framing the explanation within a relevant context, practitioners can help users appreciate the implications of the AI’s predictions. For example, explaining how a predictive model for patient outcomes can influence treatment decisions in healthcare settings can enhance the perceived value of the AI’s insights.
In conclusion, simplifying AI explanations is a multifaceted endeavor that requires the integration of various techniques. By utilizing analogies, visual aids, and plain language, while also breaking down complex information and providing context, practitioners can make AI predictions more understandable. As the reliance on AI continues to grow, ensuring that its outputs are accessible and comprehensible will be vital in fostering trust and facilitating informed decision-making across diverse fields.
Case Studies: Successful AI Communication
In recent years, the integration of artificial intelligence into various sectors has prompted a growing need for clear communication regarding AI’s predictions and decisions. This necessity has led to the development of effective strategies that enhance the understanding of AI outputs, particularly through the use of plain language explanations. Several case studies illustrate how organizations have successfully implemented these strategies, thereby improving user trust and engagement.
One notable example can be found in the healthcare sector, where AI systems are increasingly utilized for diagnostic purposes. A prominent hospital implemented an AI-driven tool designed to analyze medical imaging data. Initially, the tool provided complex statistical outputs that were difficult for medical professionals to interpret. Recognizing the potential for miscommunication, the hospital collaborated with AI developers to create a user-friendly interface that translated the AI’s predictions into plain language. By employing straightforward terminology and visual aids, the hospital enabled doctors to grasp the AI’s recommendations quickly. This approach not only facilitated better decision-making but also fostered a sense of confidence among healthcare providers, who felt more empowered to discuss AI-generated insights with their patients.
Similarly, in the financial services industry, a leading investment firm adopted an AI system to analyze market trends and provide investment recommendations. Initially, the firm faced challenges in conveying the rationale behind the AI’s predictions to its clients, many of whom lacked a technical background. To address this issue, the firm developed a series of educational materials that broke down complex financial concepts into accessible language. By using analogies and relatable examples, the firm was able to demystify the AI’s processes, allowing clients to understand the reasoning behind investment strategies. This initiative not only improved client satisfaction but also increased client retention, as individuals felt more informed and engaged in their financial decisions.
In the realm of autonomous vehicles, a leading technology company faced the challenge of explaining the decision-making processes of its AI systems to the public. Given the potential safety implications, it was crucial for the company to communicate effectively about how its vehicles made driving decisions. To achieve this, the company launched a public awareness campaign that included interactive demonstrations and simplified explanations of the AI’s algorithms. By utilizing visual storytelling techniques, the company illustrated how the AI assessed various driving scenarios and made real-time decisions. This transparency not only alleviated public concerns about safety but also built trust in the technology, encouraging wider acceptance of autonomous vehicles.
Moreover, in the realm of customer service, a major retail corporation implemented an AI chatbot to assist customers with inquiries. Initially, the chatbot’s responses were often technical and jargon-heavy, leading to confusion among users. To enhance the user experience, the corporation reprogrammed the chatbot to provide responses in plain language, incorporating friendly and conversational tones. This adjustment significantly improved customer interactions, as users felt more comfortable engaging with the AI. The corporation also gathered feedback to continuously refine the chatbot’s communication style, ensuring that it remained user-centric.
These case studies exemplify the importance of clear communication in AI applications across various industries. By prioritizing plain language explanations, organizations can bridge the gap between complex AI systems and end-users, fostering trust and understanding. As AI continues to evolve, the commitment to effective communication will be essential in ensuring that its benefits are accessible to all, ultimately leading to more informed decision-making and enhanced user experiences.
Tools For Creating Understandable AI Outputs
As artificial intelligence (AI) continues to permeate various sectors, the need for transparency and comprehensibility in its outputs has become increasingly critical. One of the primary challenges faced by developers and users alike is the inherent complexity of AI algorithms, which often produce results that are difficult for the average person to interpret. To address this issue, several tools and methodologies have emerged, aimed at transforming intricate AI predictions into plain language explanations that are accessible to a broader audience.
One of the most effective tools for creating understandable AI outputs is the use of natural language generation (NLG) systems. These systems are designed to convert structured data into human-readable text, thereby facilitating a clearer understanding of AI-generated insights. By employing NLG, organizations can present complex data analyses in a format that is not only digestible but also engaging for users. For instance, rather than presenting raw statistical outputs, an NLG system can articulate findings in a narrative format, highlighting key trends and implications in a way that resonates with stakeholders.
In addition to NLG, visualization tools play a crucial role in enhancing the interpretability of AI outputs. Visual representations, such as charts, graphs, and infographics, can simplify complex information and make it more relatable. By translating numerical data into visual formats, these tools allow users to grasp patterns and relationships that might otherwise remain obscured in textual descriptions. Furthermore, interactive visualizations enable users to explore data dynamically, fostering a deeper understanding of the underlying AI processes and outcomes.
Moreover, explainable AI (XAI) frameworks have gained traction as a means of demystifying AI decision-making processes. These frameworks are designed to provide insights into how AI models arrive at specific predictions, thereby promoting accountability and trust. By incorporating techniques such as feature importance analysis and model-agnostic explanations, XAI tools can elucidate the factors that influence AI outputs. This transparency not only empowers users to make informed decisions based on AI recommendations but also encourages developers to refine their models for greater accuracy and fairness.
Another significant aspect of creating understandable AI outputs is the emphasis on user-centric design. By prioritizing the needs and preferences of end-users, developers can tailor explanations to suit diverse audiences. This approach involves conducting user research to identify common pain points and areas of confusion, which can then inform the design of explanatory interfaces. For example, a healthcare AI system might provide different levels of detail depending on whether the user is a medical professional or a patient, ensuring that each group receives information that is relevant and comprehensible.
Furthermore, the integration of feedback mechanisms can enhance the effectiveness of AI explanations. By allowing users to provide input on the clarity and usefulness of the explanations they receive, developers can continuously improve their systems. This iterative process not only fosters a culture of collaboration between users and developers but also ensures that AI outputs evolve in response to real-world needs.
In conclusion, the tools and methodologies available for creating understandable AI outputs are diverse and continually evolving. By leveraging natural language generation, visualization techniques, explainable AI frameworks, user-centric design, and feedback mechanisms, organizations can bridge the gap between complex AI predictions and user comprehension. As the demand for transparency in AI grows, these tools will play an essential role in fostering trust and facilitating informed decision-making across various domains.
Engaging Stakeholders With Clear AI Insights
In the rapidly evolving landscape of artificial intelligence, the ability to communicate AI predictions in a clear and comprehensible manner is paramount. Engaging stakeholders effectively hinges on the clarity of insights derived from AI systems. As organizations increasingly rely on AI to inform decision-making processes, the necessity for plain language explanations becomes evident. Stakeholders, ranging from executives to end-users, must grasp the implications of AI predictions to foster trust and facilitate informed choices.
To begin with, it is essential to recognize that stakeholders often possess varying levels of technical expertise. Consequently, the language used to convey AI insights must be tailored to accommodate this diversity. By employing straightforward terminology and avoiding jargon, organizations can ensure that all stakeholders, regardless of their background, can engage with the information presented. This approach not only enhances understanding but also encourages active participation in discussions surrounding AI-driven insights.
Moreover, the use of analogies and relatable examples can significantly aid in demystifying complex AI concepts. By drawing parallels between AI predictions and familiar scenarios, stakeholders can better visualize the implications of the data. For instance, comparing a predictive model’s output to weather forecasting can help stakeholders appreciate the probabilistic nature of AI predictions. Such relatable comparisons serve to bridge the gap between technical intricacies and practical understanding, thereby fostering a more inclusive dialogue.
In addition to simplifying language, it is crucial to present AI insights in a structured and logical manner. A well-organized presentation of information allows stakeholders to follow the narrative seamlessly, enhancing their ability to absorb and retain the insights shared. Utilizing visual aids, such as charts and graphs, can further augment comprehension by providing a visual representation of the data. These tools not only make the information more accessible but also highlight key trends and patterns that may otherwise be overlooked in a purely textual format.
Furthermore, engaging stakeholders in a two-way dialogue is vital for ensuring that their concerns and questions are addressed. By encouraging feedback and inviting inquiries, organizations can create an environment where stakeholders feel valued and heard. This participatory approach not only enhances understanding but also fosters a sense of ownership among stakeholders regarding the AI insights presented. When stakeholders are actively involved in the discussion, they are more likely to embrace the findings and integrate them into their decision-making processes.
It is also important to contextualize AI predictions within the broader organizational goals and strategies. By linking insights to specific objectives, stakeholders can better appreciate the relevance and potential impact of the predictions. This alignment not only reinforces the importance of the insights but also encourages stakeholders to consider how they can leverage the information to drive positive outcomes within their respective domains.
In conclusion, engaging stakeholders with clear AI insights is a multifaceted endeavor that requires careful consideration of language, structure, and context. By prioritizing plain language explanations, utilizing relatable analogies, and fostering open dialogue, organizations can enhance stakeholder understanding and trust in AI predictions. Ultimately, this approach not only empowers stakeholders to make informed decisions but also paves the way for a more collaborative and effective integration of AI into organizational practices. As the reliance on AI continues to grow, the ability to communicate insights clearly will remain a critical factor in the successful adoption and implementation of these transformative technologies.
Future Trends In AI Explanation Methods
As artificial intelligence continues to evolve and permeate various sectors, the need for transparency in AI predictions has become increasingly critical. Future trends in AI explanation methods are poised to address this necessity by enhancing the interpretability of complex algorithms. One of the most promising directions is the development of more sophisticated natural language processing techniques. These advancements will enable AI systems to generate explanations that are not only accurate but also accessible to a broader audience. By leveraging natural language, AI can articulate its reasoning in a manner that resonates with users, thereby demystifying the decision-making processes behind its predictions.
Moreover, the integration of visual aids is expected to play a significant role in the future of AI explanations. Visual representations, such as graphs, charts, and interactive models, can complement textual explanations by providing intuitive insights into the data and algorithms at play. This dual approach—combining natural language with visual elements—will facilitate a deeper understanding of AI outputs, allowing users to grasp complex concepts more readily. As a result, stakeholders, including policymakers and end-users, will be better equipped to make informed decisions based on AI-generated insights.
In addition to these advancements, the trend towards user-centered design in AI explanation methods is gaining traction. This approach emphasizes tailoring explanations to the specific needs and backgrounds of users. By considering factors such as the user’s expertise, context, and preferences, AI systems can deliver personalized explanations that enhance comprehension. For instance, a medical AI might provide different levels of detail in its explanations depending on whether it is addressing a healthcare professional or a patient. This adaptability not only improves user experience but also fosters trust in AI systems, as users feel more engaged and informed.
Furthermore, the rise of explainable AI (XAI) frameworks is set to revolutionize how AI systems communicate their reasoning. These frameworks prioritize transparency and accountability, ensuring that AI predictions are accompanied by clear, understandable explanations. As regulatory bodies increasingly emphasize the importance of explainability, organizations will be compelled to adopt XAI principles in their AI deployments. This shift will not only enhance compliance with emerging regulations but also promote ethical AI practices, ultimately benefiting society as a whole.
Another noteworthy trend is the collaboration between AI developers and domain experts. By working together, these two groups can create explanations that are both technically sound and contextually relevant. This collaboration will lead to the development of domain-specific explanation methods that cater to the unique challenges and requirements of various fields, such as finance, healthcare, and education. As a result, users will receive explanations that are not only accurate but also meaningful within their specific contexts.
Finally, the future of AI explanation methods will likely see an increased emphasis on user feedback. By actively soliciting input from users regarding the clarity and usefulness of explanations, AI systems can continuously improve their communication strategies. This iterative process will ensure that explanations evolve in tandem with user needs, fostering a culture of transparency and collaboration.
In conclusion, the future trends in AI explanation methods are geared towards making AI’s predictions more understandable and accessible. Through advancements in natural language processing, the integration of visual aids, user-centered design, the adoption of explainable AI frameworks, collaboration with domain experts, and a focus on user feedback, the landscape of AI explanations is set to transform. As these trends unfold, they will not only enhance user comprehension but also build trust in AI systems, paving the way for a more informed and engaged society.
Q&A
1. **Question:** What is the main goal of making AI predictions understandable?
**Answer:** The main goal is to provide clear, plain language explanations of AI predictions to enhance transparency and trust among users.
2. **Question:** Why is it important to use plain language in AI explanations?
**Answer:** Plain language is important because it makes complex AI concepts accessible to a broader audience, including those without technical backgrounds.
3. **Question:** What are some common techniques for explaining AI predictions?
**Answer:** Common techniques include using visualizations, analogies, and step-by-step breakdowns of the decision-making process.
4. **Question:** How can user feedback improve AI explanations?
**Answer:** User feedback can identify areas of confusion, allowing developers to refine explanations and make them more intuitive and relevant.
5. **Question:** What role does context play in understanding AI predictions?
**Answer:** Context helps users relate the AI’s predictions to their specific situation, making the explanations more meaningful and applicable.
6. **Question:** What are the potential benefits of understandable AI predictions?
**Answer:** Benefits include increased user trust, better decision-making, and enhanced collaboration between humans and AI systems.In conclusion, making AI’s predictions understandable through plain language explanations is essential for fostering trust, enhancing user engagement, and promoting informed decision-making. By simplifying complex algorithms and presenting results in an accessible manner, stakeholders can better grasp the implications of AI outputs, leading to more effective collaboration and responsible use of technology. This approach not only demystifies AI but also empowers users to critically evaluate and utilize AI-driven insights in their respective fields.
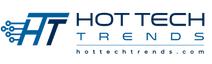