Innovative approaches to crafting realistic 3D shapes with generative AI are revolutionizing the fields of design, animation, and virtual reality. By leveraging advanced algorithms and machine learning techniques, artists and developers can create intricate and lifelike 3D models that were once time-consuming and labor-intensive to produce. These generative AI systems analyze vast datasets of existing shapes and forms, enabling them to generate new designs that adhere to aesthetic and functional criteria. This fusion of creativity and technology not only enhances the efficiency of the design process but also opens up new possibilities for customization and personalization in various applications, from gaming to industrial design. As the technology continues to evolve, the potential for creating hyper-realistic 3D shapes becomes increasingly accessible, pushing the boundaries of what is possible in digital artistry and design.
Generative AI Techniques for Realistic 3D Shape Creation
The advent of generative AI has revolutionized the field of 3D shape creation, offering innovative techniques that enhance realism and efficiency in design processes. By leveraging advanced algorithms and machine learning models, designers and artists can now produce intricate 3D shapes that closely mimic real-world objects. One of the most significant techniques employed in this domain is the use of generative adversarial networks (GANs). GANs consist of two neural networks—the generator and the discriminator—that work in tandem to create realistic outputs. The generator produces new data instances, while the discriminator evaluates them against real data, providing feedback that helps refine the generator’s output. This iterative process allows for the creation of highly detailed and realistic 3D shapes, as the generator learns to produce forms that are increasingly indistinguishable from actual objects.
In addition to GANs, variational autoencoders (VAEs) have emerged as another powerful tool for 3D shape generation. VAEs work by encoding input data into a latent space and then decoding it back into a new instance. This technique not only facilitates the generation of novel shapes but also allows for the exploration of variations within a specific category of objects. For instance, a designer can manipulate the latent space to create different iterations of a chair, experimenting with various styles and proportions while maintaining the fundamental characteristics of the object. This flexibility is particularly beneficial in industries such as gaming and film, where unique and diverse assets are essential for creating immersive environments.
Moreover, the integration of neural radiance fields (NeRF) has further enhanced the realism of 3D shape creation. NeRF utilizes deep learning to synthesize novel views of complex 3D scenes based on a sparse set of input images. By modeling the volumetric scene representation, NeRF can generate photorealistic images from different angles, allowing designers to visualize their 3D shapes in a more dynamic and realistic manner. This capability not only aids in the design process but also enhances the presentation of 3D models, making them more appealing to stakeholders and clients.
Transitioning from traditional modeling techniques to generative AI approaches also streamlines the workflow for designers. The automation of certain aspects of the design process allows for a more efficient allocation of resources, enabling artists to focus on higher-level creative decisions rather than getting bogged down in technical details. As a result, the collaboration between human creativity and machine intelligence fosters an environment where innovative ideas can flourish. Furthermore, the accessibility of generative AI tools has democratized the field of 3D design, allowing individuals with varying levels of expertise to experiment with shape creation and contribute to the evolution of design practices.
As the technology continues to evolve, the potential applications of generative AI in 3D shape creation are vast. From architecture to product design, the ability to generate realistic shapes quickly and efficiently opens new avenues for creativity and innovation. The ongoing research in this area promises to refine these techniques further, leading to even more sophisticated models that can understand and replicate the complexities of the physical world. In conclusion, the integration of generative AI techniques in 3D shape creation not only enhances realism but also transforms the design landscape, paving the way for a future where creativity and technology coexist harmoniously.
The Role of Machine Learning in 3D Shape Generation
The advent of machine learning has significantly transformed the landscape of 3D shape generation, enabling artists, designers, and engineers to create intricate models with unprecedented efficiency and realism. At the core of this transformation lies the ability of machine learning algorithms to analyze vast datasets, learning patterns and structures that define three-dimensional forms. By leveraging these capabilities, practitioners can generate complex shapes that not only meet aesthetic criteria but also adhere to functional requirements.
One of the most prominent techniques employed in this domain is the use of generative adversarial networks (GANs). GANs consist of two neural networks—the generator and the discriminator—that work in tandem to produce realistic outputs. The generator creates new 3D shapes, while the discriminator evaluates them against real-world examples. This adversarial process fosters a continuous improvement loop, where the generator refines its outputs based on feedback from the discriminator. As a result, the generated shapes become increasingly sophisticated, often indistinguishable from those crafted by human hands.
Moreover, the integration of convolutional neural networks (CNNs) has further enhanced the capabilities of machine learning in 3D shape generation. CNNs excel at processing grid-like data, making them particularly effective for analyzing voxel representations of 3D objects. By training on extensive datasets of existing shapes, CNNs can learn to recognize and replicate intricate details, such as curvature and texture. This ability to capture fine-grained features allows for the creation of highly realistic models that can be utilized in various applications, from video game design to architectural visualization.
In addition to GANs and CNNs, other machine learning techniques, such as variational autoencoders (VAEs), have emerged as powerful tools for 3D shape generation. VAEs work by encoding input data into a compressed latent space, from which new shapes can be sampled and decoded. This approach not only facilitates the generation of diverse shapes but also allows for interpolation between different forms, enabling designers to explore a continuum of possibilities. Consequently, VAEs provide a unique avenue for creativity, allowing users to experiment with variations and combinations that might not have been conceived through traditional methods.
Furthermore, the role of reinforcement learning in 3D shape generation cannot be overlooked. By employing a reward-based system, reinforcement learning algorithms can optimize the design process by evaluating the performance of generated shapes against specific criteria. For instance, in engineering applications, these algorithms can assess structural integrity or aerodynamic efficiency, guiding the generation of shapes that fulfill both aesthetic and functional demands. This synergy between creativity and practicality exemplifies the potential of machine learning to revolutionize the design process.
As machine learning continues to evolve, the future of 3D shape generation appears increasingly promising. The ongoing development of more sophisticated algorithms and the expansion of training datasets will likely lead to even greater advancements in realism and complexity. Additionally, the integration of user feedback into the generative process will empower designers to maintain creative control while harnessing the computational power of AI. Ultimately, the collaboration between human intuition and machine learning capabilities heralds a new era in the crafting of 3D shapes, where innovation and creativity can flourish in tandem. This dynamic interplay not only enhances the efficiency of the design process but also opens up new avenues for exploration and expression in the realm of three-dimensional modeling.
Enhancing Realism in 3D Models with AI Algorithms
The advent of generative artificial intelligence has revolutionized the field of 3D modeling, particularly in enhancing the realism of digital shapes. Traditional methods of creating 3D models often relied heavily on manual input and artistic skill, which, while effective, could be time-consuming and limited by the creator’s expertise. However, with the integration of AI algorithms, the process has become more efficient and capable of producing highly realistic models that can be utilized across various industries, including gaming, film, architecture, and virtual reality.
One of the primary ways AI enhances realism in 3D models is through the use of deep learning techniques. These algorithms can analyze vast datasets of existing 3D shapes and textures, learning the intricate details that contribute to a model’s lifelike appearance. By leveraging convolutional neural networks (CNNs), AI can identify patterns and features that are often overlooked by human designers. This capability allows for the generation of complex geometries and textures that mimic real-world objects with remarkable accuracy. As a result, designers can create models that not only look realistic but also behave in a manner consistent with their real-world counterparts.
Moreover, AI algorithms can facilitate the process of texture mapping, which is crucial for achieving realism in 3D models. Texture mapping involves applying images or patterns to the surface of a 3D object, and the quality of these textures significantly impacts the overall visual fidelity. Generative AI can automate the creation of high-resolution textures by synthesizing them from existing images or generating them from scratch based on learned characteristics. This not only saves time but also ensures that the textures are seamlessly integrated with the model, enhancing the overall aesthetic appeal.
In addition to texture generation, AI can also improve the realism of lighting and shading in 3D environments. Realistic lighting is essential for creating depth and dimension, and AI algorithms can simulate how light interacts with various surfaces. By employing techniques such as ray tracing, AI can calculate the paths of light rays as they bounce off surfaces, creating shadows and highlights that mimic real-world lighting conditions. This level of detail is crucial for applications such as architectural visualization, where accurate lighting can significantly influence the perception of space and materials.
Furthermore, the use of generative adversarial networks (GANs) has emerged as a powerful tool in the realm of 3D modeling. GANs consist of two neural networks that work in opposition to each other: one generates new data while the other evaluates its authenticity. This dynamic allows for the creation of highly realistic 3D shapes that can be indistinguishable from real objects. By training on diverse datasets, GANs can produce models that not only exhibit high fidelity but also possess unique characteristics, thereby expanding the creative possibilities for designers.
As the technology continues to evolve, the potential applications of AI in enhancing realism in 3D models are vast. Industries are beginning to recognize the benefits of integrating AI into their workflows, leading to increased efficiency and innovation. For instance, in the gaming industry, developers can create immersive environments that respond dynamically to player interactions, while architects can visualize their designs with unprecedented accuracy before construction begins.
In conclusion, the integration of generative AI into the process of crafting 3D shapes marks a significant advancement in the pursuit of realism. By harnessing the power of deep learning, texture synthesis, advanced lighting techniques, and GANs, designers can create models that not only meet but exceed the expectations of realism. As these technologies continue to develop, they promise to further transform the landscape of 3D modeling, opening new avenues for creativity and innovation across various fields.
Exploring Procedural Generation for 3D Shape Design
In recent years, the field of 3D shape design has witnessed a transformative shift, largely driven by advancements in generative artificial intelligence (AI). Among the various methodologies employed, procedural generation has emerged as a particularly innovative approach, enabling designers to create complex and realistic 3D shapes with unprecedented efficiency and creativity. This technique leverages algorithms to produce content algorithmically rather than manually, allowing for the rapid generation of intricate models that would be time-consuming and labor-intensive to create by hand.
At the core of procedural generation lies the concept of using mathematical functions and rules to define the parameters of a shape. By manipulating these parameters, designers can explore a vast array of possibilities, resulting in unique and diverse outputs. This flexibility is particularly beneficial in industries such as gaming and film, where the demand for varied and detailed environments is ever-increasing. For instance, a single algorithm can generate an entire landscape, complete with mountains, rivers, and vegetation, all while maintaining a coherent aesthetic. This not only saves time but also enhances the creative potential of designers, allowing them to focus on higher-level concepts rather than getting bogged down in the minutiae of modeling.
Moreover, procedural generation facilitates the integration of randomness and variability into the design process. By incorporating stochastic elements, designers can produce shapes that exhibit natural imperfections, mimicking the organic forms found in nature. This aspect is particularly valuable in creating realistic environments, as it allows for the generation of assets that feel authentic and immersive. For example, a procedurally generated tree can vary in height, branch structure, and foliage density, resulting in a forest that appears diverse and lifelike. Such variability not only enriches the visual experience but also enhances the believability of virtual worlds.
In addition to enhancing realism, procedural generation can significantly streamline workflows. Traditional modeling techniques often require extensive manual adjustments and refinements, which can be both time-consuming and prone to human error. In contrast, procedural methods allow for rapid iterations and modifications. Designers can easily tweak parameters to achieve desired outcomes, enabling them to experiment with different styles and forms without the need for extensive rework. This iterative process fosters innovation, as designers can quickly test new ideas and concepts, ultimately leading to more creative and engaging designs.
Furthermore, the integration of machine learning with procedural generation is paving the way for even more sophisticated approaches to 3D shape design. By training AI models on vast datasets of existing shapes, designers can harness the power of generative adversarial networks (GANs) to produce entirely new forms that adhere to learned patterns and styles. This synergy between procedural generation and machine learning not only enhances the quality of the generated shapes but also opens up new avenues for exploration, as AI can suggest novel designs that may not have been conceived through traditional methods.
In conclusion, the exploration of procedural generation for 3D shape design represents a significant advancement in the field, offering a powerful tool for artists and designers alike. By combining algorithmic processes with the creative potential of AI, this approach enables the efficient creation of realistic and diverse shapes, while also fostering innovation and experimentation. As technology continues to evolve, the possibilities for procedural generation will undoubtedly expand, further enriching the landscape of 3D design and opening new frontiers for creative expression.
Integrating Generative Adversarial Networks in 3D Modeling
The integration of Generative Adversarial Networks (GANs) into 3D modeling represents a significant advancement in the field of computer graphics and design. GANs, which consist of two neural networks—the generator and the discriminator—work in tandem to produce high-quality outputs by learning from a dataset. This innovative approach has transformed the way artists and designers create realistic 3D shapes, enabling them to explore new realms of creativity and efficiency.
To begin with, the generator network is responsible for creating new data instances, while the discriminator evaluates them against real data, providing feedback that helps the generator improve its outputs. This adversarial process fosters a competitive environment that ultimately leads to the generation of highly realistic 3D shapes. By leveraging this technology, designers can produce intricate models that closely resemble real-world objects, thereby enhancing the visual fidelity of their projects.
One of the most compelling aspects of using GANs in 3D modeling is their ability to learn from vast datasets. Traditional modeling techniques often require extensive manual input and expertise, which can be time-consuming and labor-intensive. In contrast, GANs can analyze and synthesize information from numerous examples, allowing them to generate complex shapes and structures with minimal human intervention. This capability not only accelerates the design process but also opens up opportunities for experimentation and innovation, as designers can quickly iterate on ideas and concepts.
Moreover, the application of GANs extends beyond mere shape generation. They can also be employed to enhance texture and detail, which are crucial for achieving realism in 3D models. By training on diverse datasets that include various materials and surfaces, GANs can produce textures that mimic the intricacies of real-world objects. This integration of texture generation with shape modeling results in a more holistic approach to 3D design, where both form and surface are considered in tandem.
In addition to improving the quality of 3D shapes, GANs also facilitate the customization of models. Designers can input specific parameters or constraints, allowing the generator to create tailored outputs that meet particular requirements. This level of customization is particularly beneficial in industries such as gaming, film, and virtual reality, where unique and diverse assets are essential for creating immersive experiences. By harnessing the power of GANs, designers can efficiently produce a wide array of models that cater to different artistic visions and project needs.
Furthermore, the integration of GANs in 3D modeling encourages collaboration between artists and artificial intelligence. As designers become more familiar with these tools, they can leverage AI-generated outputs as a foundation for their creative processes. This symbiotic relationship allows for a fusion of human intuition and machine learning, resulting in innovative designs that may not have been possible through traditional methods alone. The potential for collaboration between human creativity and AI capabilities is vast, paving the way for new artistic expressions and design paradigms.
In conclusion, the incorporation of Generative Adversarial Networks into 3D modeling is revolutionizing the way realistic shapes are crafted. By enabling rapid generation, enhancing texture, facilitating customization, and fostering collaboration, GANs are not only streamlining the design process but also expanding the horizons of what is achievable in the realm of 3D graphics. As this technology continues to evolve, it promises to unlock even greater possibilities for artists and designers, ultimately reshaping the landscape of digital creation.
Future Trends in AI-Driven 3D Shape Innovation
As we look toward the future of AI-driven 3D shape innovation, it becomes increasingly clear that the integration of generative AI into design processes is poised to revolutionize various industries. This transformation is not merely a continuation of existing practices but rather a significant leap forward, characterized by enhanced creativity, efficiency, and accessibility. One of the most promising trends is the emergence of advanced algorithms that can learn from vast datasets, enabling them to generate complex 3D shapes that were previously unimaginable. By leveraging deep learning techniques, these algorithms can analyze and synthesize design elements, resulting in unique forms that blend aesthetics with functionality.
Moreover, the democratization of design tools is another critical trend shaping the future of 3D shape innovation. As generative AI becomes more accessible, designers and artists from diverse backgrounds can harness its capabilities without requiring extensive technical expertise. This shift is likely to foster a new wave of creativity, as individuals can experiment with AI-generated designs and iterate on them in real-time. Consequently, we may witness a surge in collaborative projects that merge human intuition with machine-generated insights, leading to groundbreaking creations that reflect a fusion of artistic vision and computational power.
In addition to enhancing creativity, generative AI is also streamlining the design process, significantly reducing the time and resources required to produce high-quality 3D shapes. Traditional design methods often involve labor-intensive modeling and prototyping, which can be both time-consuming and costly. However, with the advent of AI-driven tools, designers can quickly generate multiple iterations of a shape, allowing for rapid prototyping and testing. This efficiency not only accelerates the development cycle but also enables designers to explore a broader range of possibilities, ultimately leading to more innovative outcomes.
Furthermore, the application of generative AI in 3D shape innovation extends beyond the realm of aesthetics; it also encompasses functional design. For instance, industries such as architecture and engineering are increasingly utilizing AI to optimize structural integrity and material usage. By simulating various environmental conditions and stress factors, generative algorithms can propose designs that maximize performance while minimizing waste. This approach not only enhances the sustainability of projects but also aligns with the growing demand for environmentally conscious design practices.
As we consider the implications of these advancements, it is essential to acknowledge the ethical considerations that accompany the rise of AI in design. The potential for bias in AI-generated outputs raises questions about the inclusivity of design processes. Therefore, it is crucial for developers and designers to remain vigilant and ensure that the datasets used to train generative models are diverse and representative. By doing so, we can mitigate the risk of perpetuating existing biases and foster a more equitable design landscape.
In conclusion, the future of AI-driven 3D shape innovation is marked by a convergence of creativity, efficiency, and ethical responsibility. As generative AI continues to evolve, it will undoubtedly unlock new avenues for exploration and expression in design. By embracing these innovative approaches, we can anticipate a future where the boundaries of creativity are continually expanded, leading to a richer and more diverse array of 3D shapes that reflect the complexities of our world. Ultimately, the integration of generative AI into the design process promises not only to enhance our creative capabilities but also to redefine the very nature of how we conceive and construct the objects that populate our environment.
Q&A
1. **Question:** What is generative AI in the context of 3D shape creation?
**Answer:** Generative AI refers to algorithms that can create new content, including 3D shapes, by learning patterns from existing data, allowing for the design of complex and realistic models.
2. **Question:** How can generative adversarial networks (GANs) be used in 3D modeling?
**Answer:** GANs can generate realistic 3D shapes by training on a dataset of existing models, where one network generates shapes and another evaluates their realism, leading to improved quality over iterations.
3. **Question:** What role does procedural generation play in crafting 3D shapes?
**Answer:** Procedural generation uses algorithms to create 3D shapes based on defined rules and parameters, allowing for the rapid creation of diverse and complex models without manual design.
4. **Question:** How does machine learning enhance the realism of 3D shapes?
**Answer:** Machine learning techniques can analyze and learn from vast datasets of 3D models, enabling the generation of shapes that closely mimic real-world objects in terms of proportions, textures, and details.
5. **Question:** What are some applications of realistic 3D shape generation using generative AI?
**Answer:** Applications include video game design, virtual reality environments, architectural visualization, product design, and film production, where realistic models enhance user experience and storytelling.
6. **Question:** What challenges exist in using generative AI for 3D shape creation?
**Answer:** Challenges include ensuring the quality and diversity of generated shapes, managing computational resources, addressing biases in training data, and integrating generated models into existing workflows.Innovative approaches to crafting realistic 3D shapes with generative AI leverage advanced algorithms and machine learning techniques to enhance design efficiency and creativity. By integrating neural networks and procedural generation methods, designers can produce complex geometries and textures that were previously time-consuming or impossible to achieve. These technologies not only streamline the modeling process but also enable real-time adjustments and iterations, fostering a more dynamic and interactive design environment. Ultimately, the fusion of generative AI with 3D modeling represents a significant advancement in the field, offering unprecedented opportunities for innovation and artistic expression in various industries, including gaming, film, and product design.
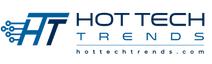