Deprecated: Implicit conversion from float 18.9 to int loses precision in /home/hottech/public_html/wp-content/plugins/internal-links/core/links/text-to-link-converter-factory.php on line 35
Deprecated: Implicit conversion from float 18.9 to int loses precision in /home/hottech/public_html/wp-content/plugins/internal-links/core/links/text-to-link-converter-factory.php on line 35
GraphCast: Revolutionizing Global Weather Forecasting with AI Precision
In the rapidly evolving field of meteorology, GraphCast emerges as a groundbreaking innovation, harnessing the power of artificial intelligence to transform global weather forecasting. By integrating advanced machine learning algorithms with vast datasets, GraphCast offers unprecedented accuracy and precision in predicting weather patterns. This cutting-edge technology not only enhances the reliability of forecasts but also provides critical insights for industries ranging from agriculture to aviation. As climate change continues to pose significant challenges, GraphCast stands at the forefront, equipping decision-makers with the tools needed to navigate an increasingly unpredictable world.
Understanding GraphCast: The Future of Weather Forecasting
GraphCast represents a significant leap forward in the realm of weather forecasting, harnessing the power of artificial intelligence to deliver unprecedented precision and reliability. As climate change continues to alter weather patterns globally, the need for accurate and timely weather predictions has never been more critical. Traditional forecasting methods, while effective to a degree, often struggle with the complexity and variability inherent in atmospheric systems. This is where GraphCast steps in, offering a transformative approach that leverages advanced AI algorithms to enhance the accuracy of weather predictions.
At the core of GraphCast’s innovation is its use of graph neural networks, a sophisticated form of AI that excels in processing data with complex interdependencies. Unlike conventional neural networks, which operate on fixed data structures, graph neural networks can model relationships between data points in a more dynamic and flexible manner. This capability is particularly advantageous in weather forecasting, where the atmosphere’s interconnected variables—such as temperature, humidity, wind speed, and pressure—must be analyzed in concert to produce reliable forecasts. By modeling these variables as nodes in a graph, GraphCast can capture the intricate web of interactions that drive weather systems, leading to more accurate predictions.
Moreover, GraphCast’s ability to process vast amounts of data in real-time sets it apart from traditional models. Weather forecasting relies heavily on data from satellites, weather stations, and other observational tools. However, the sheer volume of this data can overwhelm conventional models, leading to delays and inaccuracies. GraphCast, with its AI-driven architecture, can efficiently handle and analyze this data, providing forecasts that are not only more accurate but also delivered with greater speed. This real-time processing capability is crucial for anticipating and responding to severe weather events, where timely information can save lives and mitigate damage.
In addition to its technical prowess, GraphCast also represents a shift towards more sustainable forecasting practices. Traditional models often require significant computational resources, contributing to their environmental footprint. In contrast, GraphCast’s AI-driven approach is more efficient, reducing the energy consumption associated with weather prediction. This efficiency aligns with broader efforts to minimize the environmental impact of technological advancements, ensuring that progress in one area does not come at the expense of another.
Furthermore, the implementation of GraphCast has the potential to democratize access to high-quality weather forecasts. By reducing the computational demands of forecasting, GraphCast can be deployed in regions with limited technological infrastructure, providing communities with the tools they need to prepare for and respond to weather-related challenges. This democratization is particularly important in developing countries, where the impacts of climate change are often felt most acutely.
In conclusion, GraphCast is poised to revolutionize global weather forecasting by combining the precision of AI with the flexibility of graph neural networks. Its ability to process complex data in real-time, coupled with its sustainable and accessible design, positions it as a critical tool in the ongoing effort to understand and adapt to our changing climate. As GraphCast continues to evolve, it promises to enhance our ability to predict and respond to weather events, ultimately contributing to a safer and more resilient world.
How AI Precision in GraphCast Enhances Weather Predictions
GraphCast, a groundbreaking innovation in the realm of meteorology, is poised to transform global weather forecasting through the application of artificial intelligence (AI). This advanced system leverages the precision of AI to enhance the accuracy and reliability of weather predictions, offering a significant leap forward from traditional forecasting methods. At the heart of GraphCast’s innovation is its ability to process vast amounts of meteorological data with unprecedented speed and accuracy. By utilizing sophisticated algorithms, GraphCast can analyze complex weather patterns and predict future conditions with remarkable precision. This capability is particularly crucial in an era where climate change is causing increasingly unpredictable weather events, necessitating more reliable forecasting tools.
The integration of AI into weather forecasting is not merely about processing data faster; it is about understanding the intricate relationships between various meteorological variables. GraphCast employs machine learning techniques to identify patterns and correlations that might be overlooked by conventional models. This allows for a more nuanced understanding of weather systems, leading to forecasts that are not only more accurate but also more detailed. For instance, GraphCast can provide insights into localized weather phenomena, offering predictions that are tailored to specific regions. This level of detail is invaluable for industries such as agriculture, aviation, and disaster management, where precise weather information can significantly impact decision-making processes.
Moreover, GraphCast’s AI-driven approach enhances the adaptability of weather models. Traditional forecasting methods often rely on static models that may not account for sudden changes in weather patterns. In contrast, GraphCast’s dynamic algorithms can adjust in real-time, incorporating new data as it becomes available. This adaptability ensures that forecasts remain relevant and accurate, even in the face of rapidly changing weather conditions. Consequently, this capability is particularly beneficial in predicting extreme weather events, such as hurricanes and typhoons, where timely and accurate information is critical for mitigating potential damage and ensuring public safety.
In addition to improving the accuracy of weather predictions, GraphCast also contributes to the democratization of weather data. By making advanced forecasting tools accessible to a broader audience, GraphCast empowers individuals and communities to make informed decisions based on reliable weather information. This democratization is facilitated by user-friendly interfaces and platforms that present complex data in an easily understandable format. As a result, users from various sectors can leverage GraphCast’s insights to optimize their operations and enhance their resilience against adverse weather conditions.
Furthermore, the implementation of AI in weather forecasting through GraphCast has significant implications for research and development in meteorology. By providing a more accurate and comprehensive understanding of weather systems, GraphCast opens new avenues for scientific exploration and innovation. Researchers can utilize the data generated by GraphCast to study climate patterns, investigate the impacts of climate change, and develop new strategies for weather prediction and management. This potential for advancement underscores the transformative impact of AI on the field of meteorology.
In conclusion, GraphCast represents a monumental step forward in global weather forecasting, harnessing the power of AI to deliver precise and reliable predictions. By enhancing the accuracy, adaptability, and accessibility of weather forecasts, GraphCast not only improves decision-making across various sectors but also contributes to a deeper understanding of our planet’s complex weather systems. As AI continues to evolve, the potential for further advancements in weather forecasting is immense, promising a future where we are better equipped to navigate the challenges posed by an ever-changing climate.
The Role of Machine Learning in GraphCast’s Weather Models
GraphCast, a cutting-edge weather forecasting system, is transforming the landscape of meteorology through the integration of advanced machine learning techniques. At the heart of this innovation lies the application of machine learning algorithms, which have significantly enhanced the accuracy and efficiency of weather predictions. By leveraging the power of artificial intelligence, GraphCast is setting a new standard in the field, offering unprecedented precision in weather modeling.
Machine learning, a subset of artificial intelligence, involves the development of algorithms that enable computers to learn from and make predictions based on data. In the context of GraphCast, machine learning plays a pivotal role in processing vast amounts of meteorological data, which includes variables such as temperature, humidity, wind speed, and atmospheric pressure. These data points are collected from a multitude of sources, including satellites, weather stations, and ocean buoys, providing a comprehensive dataset for analysis.
One of the key advantages of using machine learning in weather forecasting is its ability to identify complex patterns and relationships within the data that may not be immediately apparent to human analysts. Traditional weather models often rely on linear equations and assumptions that can oversimplify the dynamic nature of the atmosphere. In contrast, machine learning models can capture non-linear interactions and adapt to new data, thereby improving the accuracy of forecasts.
GraphCast employs sophisticated machine learning techniques, such as neural networks and ensemble learning, to enhance its predictive capabilities. Neural networks, inspired by the human brain’s structure, consist of interconnected nodes that process information in layers. These networks are particularly adept at handling large datasets and can be trained to recognize intricate patterns in weather data. Ensemble learning, on the other hand, involves combining multiple models to produce a more robust and reliable forecast. By integrating these approaches, GraphCast can generate predictions that are not only more accurate but also more resilient to uncertainties.
Furthermore, machine learning enables GraphCast to perform real-time data assimilation, a process that continuously updates the model with the latest observations. This capability is crucial for maintaining the relevance and accuracy of forecasts, especially in rapidly changing weather conditions. By incorporating new data as it becomes available, GraphCast can adjust its predictions accordingly, providing timely and precise information to users.
The implementation of machine learning in GraphCast’s weather models also facilitates the generation of probabilistic forecasts. Unlike deterministic forecasts, which provide a single predicted outcome, probabilistic forecasts offer a range of possible scenarios along with their associated probabilities. This approach allows users to better understand the uncertainty inherent in weather predictions and make more informed decisions based on the likelihood of various outcomes.
In conclusion, the role of machine learning in GraphCast’s weather models is instrumental in revolutionizing global weather forecasting. By harnessing the capabilities of artificial intelligence, GraphCast is able to process vast amounts of data, identify complex patterns, and generate highly accurate and timely forecasts. As machine learning technology continues to advance, it is likely that its application in meteorology will expand further, paving the way for even more precise and reliable weather predictions. Through its innovative use of machine learning, GraphCast is not only enhancing our understanding of atmospheric dynamics but also empowering individuals and organizations to better prepare for and respond to weather-related challenges.
GraphCast vs. Traditional Weather Forecasting: A Comparative Analysis
In the realm of meteorology, the advent of artificial intelligence has heralded a new era of precision and efficiency, with GraphCast emerging as a groundbreaking innovation in global weather forecasting. This AI-driven system represents a significant departure from traditional forecasting methods, offering a comparative analysis that highlights its transformative potential. Traditional weather forecasting relies heavily on numerical weather prediction (NWP) models, which use mathematical equations to simulate atmospheric processes. These models require vast computational resources and are often limited by their resolution and the complexity of the equations involved. Despite their sophistication, traditional models can struggle with accurately predicting short-term weather events and are often updated only a few times a day, which can lead to delays in disseminating critical information.
In contrast, GraphCast leverages the power of machine learning and graph neural networks to enhance forecasting accuracy and timeliness. By processing vast amounts of historical weather data, GraphCast can identify patterns and relationships that might be overlooked by conventional models. This ability to learn from data allows GraphCast to make predictions with remarkable speed and precision, often outperforming traditional methods in both short-term and long-term forecasts. Moreover, GraphCast’s use of graph neural networks enables it to model complex spatial relationships more effectively, providing a more nuanced understanding of atmospheric dynamics.
One of the most significant advantages of GraphCast over traditional forecasting methods is its ability to update predictions in real-time. This continuous updating process ensures that the most current data is always being used, allowing for more accurate and timely forecasts. This capability is particularly crucial in the context of extreme weather events, where timely and precise information can be the difference between safety and disaster. Furthermore, GraphCast’s efficiency in processing data means that it can provide forecasts at a much higher resolution than traditional models, offering more localized and detailed predictions that are invaluable for decision-makers in various sectors.
Another critical aspect of GraphCast’s superiority lies in its scalability. Traditional models often require significant computational power and infrastructure, which can be a limiting factor for many meteorological agencies, especially in developing regions. GraphCast, on the other hand, is designed to be more resource-efficient, making it accessible to a broader range of users. This democratization of advanced forecasting technology has the potential to improve weather prediction capabilities globally, particularly in areas that have historically been underserved by traditional methods.
Despite these advantages, it is essential to acknowledge that GraphCast is not without its challenges. The reliance on historical data means that the system’s accuracy is contingent upon the quality and comprehensiveness of the data it is trained on. Additionally, while GraphCast excels in pattern recognition, it may still require integration with traditional models to fully understand the underlying physical processes driving weather phenomena. This hybrid approach could potentially offer the best of both worlds, combining the strengths of AI with the foundational principles of meteorology.
In conclusion, GraphCast represents a significant leap forward in the field of weather forecasting, offering a compelling alternative to traditional methods. Its ability to provide real-time, high-resolution forecasts with greater accuracy and efficiency positions it as a vital tool in the ongoing quest to better understand and predict the complexities of our planet’s weather systems. As technology continues to evolve, the integration of AI-driven systems like GraphCast with traditional meteorological practices promises to revolutionize the way we approach weather forecasting, ultimately leading to safer and more informed communities worldwide.
Real-World Applications of GraphCast in Meteorology
GraphCast, an innovative artificial intelligence (AI) system, is transforming the field of meteorology by enhancing the accuracy and efficiency of global weather forecasting. This cutting-edge technology leverages the power of AI to process vast amounts of meteorological data, offering precise and timely weather predictions that are crucial for various sectors, including agriculture, aviation, and disaster management. As traditional weather forecasting methods often struggle with the complexity and variability of atmospheric conditions, GraphCast emerges as a formidable tool that addresses these challenges with remarkable precision.
At the core of GraphCast’s functionality is its ability to analyze complex data sets through advanced machine learning algorithms. By utilizing graph neural networks, the system can model the intricate relationships between different meteorological variables, such as temperature, humidity, wind speed, and atmospheric pressure. This approach allows GraphCast to capture the dynamic interactions within the Earth’s atmosphere, providing a more comprehensive understanding of weather patterns. Consequently, the system can generate forecasts that are not only more accurate but also extend further into the future compared to traditional models.
Moreover, GraphCast’s real-world applications are vast and varied, significantly impacting several industries. In agriculture, for instance, accurate weather forecasts are essential for optimizing planting schedules, managing irrigation, and mitigating the risks of extreme weather events. By providing farmers with reliable predictions, GraphCast enables them to make informed decisions that enhance crop yield and reduce losses. Similarly, in the aviation industry, precise weather forecasts are critical for ensuring flight safety and efficiency. GraphCast’s ability to predict turbulence, storms, and other adverse weather conditions allows airlines to optimize flight routes, minimize delays, and enhance passenger safety.
In addition to its applications in agriculture and aviation, GraphCast plays a pivotal role in disaster management. Natural disasters such as hurricanes, floods, and wildfires pose significant threats to human life and property. Accurate and timely weather forecasts are crucial for emergency response teams to prepare and respond effectively to such events. GraphCast’s advanced predictive capabilities enable authorities to issue early warnings, plan evacuations, and allocate resources more efficiently, ultimately saving lives and reducing the impact of disasters.
Furthermore, the integration of GraphCast into existing meteorological frameworks is seamless, as it complements rather than replaces traditional forecasting methods. By enhancing the accuracy of numerical weather prediction models, GraphCast provides meteorologists with a powerful tool to refine their forecasts. This collaborative approach ensures that the expertise of human forecasters is combined with the computational prowess of AI, resulting in a more robust and reliable forecasting system.
As the climate continues to change and weather patterns become increasingly unpredictable, the need for accurate and reliable weather forecasting has never been more critical. GraphCast represents a significant advancement in meteorology, offering a solution that addresses the limitations of traditional forecasting methods. By harnessing the power of AI, GraphCast not only improves the accuracy of weather predictions but also extends their applicability across various sectors. As a result, this revolutionary technology is poised to play a crucial role in shaping the future of global weather forecasting, providing societies with the tools they need to adapt and thrive in an ever-changing world.
The Impact of GraphCast on Climate Research and Environmental Planning
GraphCast, an innovative artificial intelligence system, is poised to transform the landscape of global weather forecasting, offering unprecedented precision and reliability. This cutting-edge technology leverages advanced machine learning algorithms to analyze vast datasets, providing insights that are crucial for climate research and environmental planning. As the world grapples with the escalating impacts of climate change, the need for accurate and timely weather predictions has never been more critical. GraphCast emerges as a pivotal tool in this context, offering capabilities that extend far beyond traditional forecasting methods.
At the heart of GraphCast’s revolutionary approach is its ability to process and interpret complex data from a multitude of sources. By integrating information from satellites, weather stations, and ocean buoys, among others, GraphCast constructs a comprehensive picture of atmospheric conditions. This holistic view enables the system to generate forecasts with remarkable accuracy, even for regions that have historically been challenging to predict. Consequently, researchers and policymakers can rely on GraphCast to make informed decisions, enhancing their ability to respond to environmental challenges.
Moreover, GraphCast’s impact on climate research is profound. By providing detailed and reliable forecasts, it allows scientists to better understand weather patterns and their long-term implications. This understanding is crucial for developing strategies to mitigate the effects of climate change. For instance, accurate predictions of extreme weather events, such as hurricanes and droughts, enable communities to prepare more effectively, potentially saving lives and reducing economic losses. Furthermore, GraphCast’s data-driven insights contribute to refining climate models, which are essential for projecting future climate scenarios and informing global policy decisions.
In addition to its contributions to climate research, GraphCast plays a significant role in environmental planning. Urban planners and environmental managers can utilize its forecasts to design infrastructure that is resilient to changing weather conditions. For example, cities can optimize drainage systems to handle increased rainfall, while agricultural sectors can adjust planting schedules to align with expected weather patterns. This proactive approach not only minimizes the adverse effects of climate change but also promotes sustainable development.
Transitioning from traditional forecasting methods to AI-driven systems like GraphCast also presents opportunities for collaboration across various sectors. By fostering partnerships between technology developers, meteorologists, and policymakers, GraphCast encourages a multidisciplinary approach to tackling climate-related challenges. This collaboration is essential for ensuring that the insights generated by GraphCast are effectively translated into actionable strategies.
However, the integration of AI in weather forecasting is not without its challenges. Ensuring the accuracy and reliability of AI-generated forecasts requires continuous validation and refinement of algorithms. Additionally, there is a need to address concerns related to data privacy and security, as the system relies on vast amounts of information. Despite these challenges, the potential benefits of GraphCast far outweigh the drawbacks, making it a valuable asset in the global effort to combat climate change.
In conclusion, GraphCast represents a significant advancement in the field of weather forecasting, with far-reaching implications for climate research and environmental planning. Its ability to deliver precise and reliable forecasts empowers researchers, policymakers, and communities to make informed decisions, ultimately enhancing resilience to climate change. As the world continues to face environmental challenges, the adoption of innovative technologies like GraphCast will be crucial in shaping a sustainable future.
Q&A
1. **What is GraphCast?**
GraphCast is an AI-based system designed to enhance global weather forecasting by leveraging advanced machine learning techniques to improve prediction accuracy and efficiency.
2. **How does GraphCast improve weather forecasting?**
GraphCast utilizes graph neural networks to model complex atmospheric patterns, allowing it to process vast amounts of meteorological data and generate precise weather forecasts.
3. **What are the key features of GraphCast?**
Key features include high-resolution forecasts, rapid processing times, and the ability to integrate diverse data sources, such as satellite imagery and sensor data, to produce comprehensive weather models.
4. **Who developed GraphCast?**
GraphCast was developed by a team of researchers and engineers specializing in artificial intelligence and meteorology, often involving collaborations between tech companies and academic institutions.
5. **What are the benefits of using GraphCast over traditional methods?**
GraphCast offers improved accuracy, faster updates, and the ability to predict extreme weather events more reliably, which can lead to better preparedness and response strategies.
6. **What challenges does GraphCast face?**
Challenges include ensuring data quality, managing computational demands, and integrating AI forecasts with existing meteorological systems and practices to gain trust and adoption from the weather forecasting community.GraphCast represents a significant advancement in global weather forecasting by leveraging AI precision to enhance prediction accuracy and efficiency. By utilizing advanced machine learning algorithms and vast datasets, GraphCast can model complex atmospheric patterns and provide more reliable forecasts. This innovation not only improves the timeliness and accuracy of weather predictions but also has the potential to transform industries reliant on weather data, such as agriculture, aviation, and disaster management. As a result, GraphCast stands as a revolutionary tool in meteorology, offering a new level of precision and insight into weather phenomena, ultimately contributing to better-informed decision-making and increased safety worldwide.
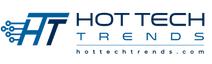