**Introduction:**
In the rapidly evolving landscape of artificial intelligence, the intersection of machine learning and mathematics has given rise to groundbreaking methodologies for uncovering new mathematical insights. One such innovative approach is FunSearch, a pioneering framework that leverages the capabilities of large language models to explore and unveil novel mathematical discoveries. By harnessing the power of advanced AI, FunSearch transcends traditional boundaries, offering a unique platform where complex mathematical concepts are not only analyzed but also expanded upon. This cutting-edge tool is designed to assist mathematicians and researchers in navigating the intricate world of mathematical theories, providing a fresh perspective and fostering a deeper understanding of the mathematical universe. Through FunSearch, the potential for discovering new mathematical phenomena is not just a possibility but a tangible reality, marking a significant step forward in the symbiotic relationship between artificial intelligence and mathematics.
Exploring FunSearch: Revolutionizing Mathematical Discoveries with AI
In recent years, the intersection of artificial intelligence and mathematics has opened new avenues for exploration and discovery. One of the most exciting developments in this domain is FunSearch, a groundbreaking initiative that leverages large language models to revolutionize mathematical discoveries. As we delve into the intricacies of FunSearch, it becomes evident that this innovative approach is reshaping the landscape of mathematical research and problem-solving.
At its core, FunSearch utilizes the capabilities of large language models, which are trained on vast datasets encompassing a wide range of mathematical concepts, theories, and problems. These models, powered by advanced machine learning algorithms, are designed to understand and generate human-like text. By harnessing this ability, FunSearch aims to assist mathematicians in uncovering new insights, formulating conjectures, and even proving theorems. The potential of such a tool is immense, as it can process and analyze information at a speed and scale that far surpasses human capabilities.
One of the primary advantages of FunSearch is its ability to identify patterns and connections within complex mathematical data. Traditional methods of mathematical research often rely on human intuition and experience, which, while invaluable, can be limited by cognitive biases and the sheer volume of information. FunSearch, on the other hand, can sift through extensive datasets, recognizing subtle patterns that might elude even the most seasoned mathematicians. This capability not only accelerates the discovery process but also opens up new avenues for exploration that were previously unimaginable.
Moreover, FunSearch serves as a collaborative tool, bridging the gap between human intuition and machine precision. By providing mathematicians with suggestions and potential pathways to explore, it enhances their ability to tackle complex problems. For instance, when faced with a particularly challenging theorem, a mathematician can input relevant data into FunSearch, which then generates possible approaches or even partial solutions. This symbiotic relationship between human and machine fosters a more dynamic and efficient research environment, where ideas can be tested and refined with unprecedented speed.
In addition to its practical applications, FunSearch also holds promise for educational purposes. By making advanced mathematical concepts more accessible, it has the potential to inspire a new generation of mathematicians. Students and educators can use FunSearch to explore mathematical theories interactively, gaining insights that might otherwise remain out of reach. This democratization of knowledge not only enriches the learning experience but also encourages a broader range of individuals to engage with mathematics.
However, the integration of AI into mathematical research is not without its challenges. Concerns about the reliability and interpretability of AI-generated results persist, as do questions about the ethical implications of relying on machines for intellectual pursuits. It is crucial for the mathematical community to address these issues, ensuring that FunSearch and similar tools are used responsibly and transparently.
In conclusion, FunSearch represents a significant leap forward in the field of mathematical research. By harnessing the power of large language models, it offers a novel approach to discovery, collaboration, and education. As we continue to explore the potential of this innovative tool, it is clear that the future of mathematics is being reshaped by the synergy between human intellect and artificial intelligence. Through careful consideration and responsible implementation, FunSearch has the potential to unlock new frontiers in mathematical understanding, paving the way for discoveries that were once beyond our reach.
The Role of Large Language Models in FunSearch’s Mathematical Breakthroughs
In recent years, the advent of large language models has revolutionized various fields, including natural language processing, creative writing, and even scientific research. Among the most intriguing applications of these models is their role in mathematical discovery, as exemplified by FunSearch, a pioneering initiative that leverages the capabilities of large language models to uncover new mathematical insights. The integration of these models into mathematical research has opened up unprecedented avenues for exploration, enabling researchers to tackle complex problems with enhanced efficiency and creativity.
At the core of FunSearch’s success is the ability of large language models to process and analyze vast amounts of mathematical data. These models, trained on extensive datasets, possess a remarkable capacity to recognize patterns and generate hypotheses that might elude traditional analytical methods. By harnessing this computational power, FunSearch has been able to identify novel relationships and conjectures, thereby pushing the boundaries of mathematical knowledge. This approach not only accelerates the pace of discovery but also democratizes access to advanced mathematical tools, allowing a broader range of researchers to contribute to the field.
Moreover, large language models facilitate a more intuitive exploration of mathematical concepts. Unlike conventional computational methods that often require explicit programming and algorithmic design, language models can interpret and generate mathematical expressions in a more human-like manner. This capability enables researchers to interact with mathematical problems in a more natural and fluid way, fostering a deeper understanding of the underlying principles. Consequently, FunSearch has been able to bridge the gap between abstract mathematical theory and practical application, making complex ideas more accessible to both experts and novices alike.
In addition to enhancing the discovery process, large language models also play a crucial role in verifying and validating mathematical findings. The models’ ability to cross-reference and synthesize information from diverse sources ensures that new conjectures and theorems are rigorously tested against existing knowledge. This comprehensive approach minimizes the risk of errors and inconsistencies, thereby bolstering the credibility of FunSearch’s contributions to the mathematical community. Furthermore, the collaborative nature of these models encourages interdisciplinary dialogue, as insights from different fields can be seamlessly integrated into the research process.
Despite these advancements, the use of large language models in mathematical research is not without challenges. One of the primary concerns is the interpretability of the models’ outputs. While these models can generate highly accurate predictions and suggestions, understanding the rationale behind their conclusions remains a complex task. This opacity can hinder the acceptance of new findings, as researchers may be reluctant to trust results that cannot be easily explained. To address this issue, FunSearch is actively exploring methods to enhance the transparency and interpretability of language model outputs, ensuring that their contributions are both reliable and comprehensible.
In conclusion, the role of large language models in FunSearch’s mathematical breakthroughs underscores the transformative potential of artificial intelligence in scientific research. By enabling more efficient exploration, intuitive interaction, and rigorous validation, these models are reshaping the landscape of mathematical discovery. As FunSearch continues to refine its methodologies and address existing challenges, the integration of large language models promises to unlock even greater possibilities for innovation and collaboration in the field of mathematics. Through this synergy of human ingenuity and machine intelligence, the future of mathematical research holds exciting prospects for uncovering the mysteries of the universe.
How FunSearch is Transforming the Landscape of Mathematical Research
FunSearch is revolutionizing the field of mathematical research by leveraging the capabilities of large language models to uncover new mathematical discoveries. This innovative approach is transforming how researchers explore complex mathematical problems, offering unprecedented insights and solutions. At the heart of FunSearch is the integration of advanced computational techniques with traditional mathematical inquiry, creating a synergy that enhances the depth and breadth of research possibilities.
The advent of large language models has opened new avenues for mathematical exploration. These models, trained on vast datasets, possess the ability to understand and generate human-like text, making them invaluable tools for researchers. By utilizing these models, FunSearch can process and analyze extensive mathematical literature, identifying patterns and connections that may elude human researchers. This capability not only accelerates the research process but also broadens the scope of inquiry, allowing for the exploration of previously uncharted territories in mathematics.
One of the key advantages of FunSearch is its ability to facilitate collaboration among researchers. By providing a platform where ideas can be shared and developed collectively, FunSearch fosters a collaborative environment that encourages the exchange of knowledge and expertise. This collaborative approach is particularly beneficial in tackling complex mathematical problems that require interdisciplinary solutions. By bringing together researchers from diverse fields, FunSearch enables the synthesis of different perspectives, leading to more comprehensive and innovative solutions.
Moreover, FunSearch enhances the accessibility of mathematical research. Traditionally, access to cutting-edge research has been limited to those within academic institutions or specialized fields. However, FunSearch democratizes access to mathematical knowledge by making research findings and methodologies available to a broader audience. This increased accessibility not only promotes inclusivity within the mathematical community but also encourages the participation of amateur mathematicians and enthusiasts, who can contribute fresh ideas and perspectives.
In addition to fostering collaboration and accessibility, FunSearch also plays a crucial role in education. By integrating large language models into educational platforms, FunSearch provides students with interactive learning experiences that enhance their understanding of complex mathematical concepts. These models can generate personalized learning materials, offer real-time feedback, and simulate problem-solving scenarios, making mathematics more engaging and accessible to learners at all levels. This educational aspect of FunSearch is instrumental in nurturing the next generation of mathematicians, equipping them with the tools and knowledge needed to tackle future challenges.
Furthermore, FunSearch is instrumental in advancing the frontiers of mathematical research by enabling the discovery of new theorems and conjectures. The ability of large language models to process and analyze vast amounts of data allows researchers to identify novel patterns and relationships, leading to the formulation of new mathematical theories. This capability not only expands the existing body of mathematical knowledge but also inspires further research and exploration.
In conclusion, FunSearch is transforming the landscape of mathematical research by harnessing the power of large language models. Through its ability to facilitate collaboration, enhance accessibility, and advance education, FunSearch is paving the way for new mathematical discoveries and innovations. As researchers continue to explore the potential of this groundbreaking approach, the future of mathematics looks promising, with FunSearch at the forefront of this exciting transformation.
FunSearch and AI: A New Era of Mathematical Exploration
In recent years, the intersection of artificial intelligence and mathematics has opened up new avenues for exploration and discovery. Among the most exciting developments in this field is FunSearch, a groundbreaking initiative that leverages large language models to uncover novel mathematical insights. As we delve into this new era of mathematical exploration, it is essential to understand how FunSearch operates and the implications it holds for the future of mathematics.
FunSearch represents a significant shift in how mathematical research is conducted. Traditionally, mathematical discoveries have relied heavily on human intuition and the meticulous work of mathematicians. However, with the advent of large language models, such as those developed by OpenAI and other leading tech companies, there is now the potential to automate and accelerate the discovery process. These models, trained on vast datasets, possess the ability to recognize patterns and generate hypotheses that might elude even the most seasoned mathematicians.
The core of FunSearch’s approach lies in its ability to process and analyze enormous amounts of mathematical data. By utilizing the computational power of large language models, FunSearch can sift through complex equations, theorems, and proofs, identifying connections and suggesting new lines of inquiry. This capability is particularly valuable in fields where the sheer volume of existing literature can be overwhelming for human researchers. Moreover, FunSearch’s algorithms are designed to learn and adapt, continuously improving their performance as they are exposed to more data.
One of the most intriguing aspects of FunSearch is its potential to democratize mathematical research. By making advanced computational tools accessible to a broader audience, FunSearch empowers not only professional mathematicians but also students, educators, and amateur enthusiasts. This democratization could lead to a more diverse range of perspectives and ideas, fostering innovation and creativity in mathematical exploration. Furthermore, FunSearch’s user-friendly interface allows individuals with varying levels of expertise to engage with complex mathematical concepts, thereby broadening the scope of participation in the field.
In addition to democratizing research, FunSearch also holds promise for interdisciplinary collaboration. Mathematics is often described as the language of science, and its principles underpin many other disciplines, including physics, computer science, and economics. By facilitating the discovery of new mathematical insights, FunSearch can contribute to advancements in these related fields. For instance, novel mathematical models generated by FunSearch could lead to breakthroughs in understanding complex systems, optimizing algorithms, or developing new technologies.
Despite its potential, the integration of large language models into mathematical research is not without challenges. Concerns about the accuracy and reliability of AI-generated insights must be addressed, as errors in mathematical reasoning can have significant consequences. Additionally, there is the question of interpretability; while FunSearch can generate hypotheses, human mathematicians are still needed to verify and contextualize these findings. Ensuring that AI complements rather than replaces human expertise is crucial for the responsible advancement of this technology.
As we stand on the cusp of a new era in mathematical exploration, FunSearch exemplifies the transformative power of artificial intelligence. By harnessing the capabilities of large language models, FunSearch is poised to revolutionize the way we approach mathematical research, offering unprecedented opportunities for discovery and collaboration. As this technology continues to evolve, it will undoubtedly reshape our understanding of mathematics and its role in the broader scientific landscape, paving the way for a future where human and artificial intelligence work hand in hand to unlock the mysteries of the universe.
Unveiling Hidden Patterns: FunSearch’s Impact on Mathematics
In recent years, the intersection of artificial intelligence and mathematics has opened new avenues for exploration and discovery. Among the most intriguing developments in this domain is FunSearch, a groundbreaking initiative that leverages large language models to uncover hidden patterns in mathematical data. This innovative approach has the potential to revolutionize the way mathematicians approach problem-solving, offering fresh insights and facilitating the discovery of previously unnoticed relationships within complex mathematical structures.
At the core of FunSearch is the utilization of large language models, which have been trained on vast datasets encompassing a wide range of mathematical concepts and problems. These models, renowned for their ability to process and generate human-like text, are now being harnessed to analyze mathematical data in unprecedented ways. By examining patterns and relationships within this data, FunSearch aims to identify novel mathematical insights that might elude traditional analytical methods.
One of the most significant impacts of FunSearch is its ability to reveal hidden patterns that are not immediately apparent to human researchers. For instance, in the realm of number theory, FunSearch has been instrumental in identifying previously unknown relationships between prime numbers. By analyzing extensive datasets, the language models can detect subtle patterns and correlations that might take human mathematicians years to uncover. This capability not only accelerates the pace of discovery but also broadens the scope of mathematical inquiry, allowing researchers to explore new territories with greater confidence.
Moreover, FunSearch’s impact extends beyond the discovery of new patterns. It also plays a crucial role in hypothesis generation and testing. By providing mathematicians with a wealth of data-driven insights, FunSearch enables researchers to formulate and test hypotheses more efficiently. This iterative process of hypothesis generation and validation is essential for advancing mathematical knowledge, and FunSearch’s contributions in this area are invaluable. The ability to quickly generate and test hypotheses allows mathematicians to focus their efforts on the most promising avenues of research, thereby optimizing the allocation of resources and time.
In addition to its contributions to pure mathematics, FunSearch has practical applications in various fields that rely on mathematical modeling and analysis. For example, in cryptography, the discovery of new patterns in number theory can lead to the development of more secure encryption algorithms. Similarly, in fields such as physics and engineering, FunSearch’s insights can enhance the accuracy of mathematical models, leading to more precise predictions and improved technological innovations.
Despite its many advantages, the integration of large language models into mathematical research is not without challenges. One of the primary concerns is the interpretability of the models’ outputs. While FunSearch can identify patterns and generate hypotheses, understanding the underlying reasons for these patterns remains a complex task. This necessitates a collaborative effort between AI researchers and mathematicians to ensure that the insights generated by FunSearch are both meaningful and actionable.
In conclusion, FunSearch represents a significant advancement in the field of mathematics, offering a novel approach to uncovering hidden patterns and facilitating the discovery of new mathematical insights. By harnessing the power of large language models, FunSearch not only accelerates the pace of mathematical discovery but also broadens the scope of inquiry, enabling researchers to explore new frontiers with greater confidence. As this technology continues to evolve, it holds the promise of transforming the landscape of mathematical research, paving the way for a deeper understanding of the intricate patterns that underpin the mathematical universe.
FunSearch’s Innovative Approach to Solving Complex Mathematical Problems
FunSearch, a pioneering initiative in the realm of mathematical research, is leveraging the power of large language models to tackle complex mathematical problems. This innovative approach is transforming the landscape of mathematical discovery, offering new insights and solutions that were previously unattainable. By harnessing the capabilities of advanced artificial intelligence, FunSearch is not only accelerating the pace of mathematical research but also expanding the boundaries of what is possible in this field.
At the core of FunSearch’s methodology is the use of large language models, which have been trained on vast datasets encompassing a wide range of mathematical concepts and theories. These models are capable of understanding and generating human-like text, making them invaluable tools for exploring intricate mathematical problems. By processing and analyzing large volumes of mathematical data, these models can identify patterns and relationships that may not be immediately apparent to human researchers. This ability to uncover hidden connections is one of the key advantages of using language models in mathematical research.
Moreover, FunSearch’s approach is characterized by its collaborative nature. By integrating the expertise of mathematicians with the computational power of language models, FunSearch is creating a synergistic environment where human intuition and machine intelligence complement each other. This collaboration allows for a more comprehensive exploration of mathematical problems, as researchers can leverage the strengths of both human and machine to arrive at novel solutions. Furthermore, this partnership fosters a deeper understanding of complex mathematical concepts, as language models can provide explanations and insights that enhance human comprehension.
In addition to facilitating collaboration, FunSearch’s use of language models also democratizes access to mathematical research. Traditionally, advanced mathematical problem-solving has been the domain of highly specialized experts. However, with the assistance of language models, a broader range of individuals can engage with complex mathematical concepts. This democratization of knowledge not only broadens the pool of potential contributors to mathematical research but also encourages diverse perspectives and innovative approaches to problem-solving.
Another significant aspect of FunSearch’s approach is its emphasis on transparency and reproducibility. By utilizing language models, FunSearch ensures that the processes and methodologies used in solving mathematical problems are well-documented and easily accessible. This transparency is crucial for the validation and verification of results, as it allows other researchers to replicate and build upon FunSearch’s findings. In this way, FunSearch is contributing to the creation of a more open and collaborative scientific community, where knowledge is shared and progress is collectively achieved.
As FunSearch continues to explore the potential of large language models in mathematical research, it is also addressing the challenges associated with this approach. One such challenge is ensuring the accuracy and reliability of the models’ outputs. To mitigate this, FunSearch employs rigorous testing and validation procedures, ensuring that the solutions generated by language models are both accurate and meaningful. Additionally, FunSearch is committed to continuously refining and improving its models, incorporating feedback from researchers and advancements in AI technology.
In conclusion, FunSearch’s innovative approach to solving complex mathematical problems is reshaping the field of mathematical research. By leveraging the power of large language models, FunSearch is not only accelerating the discovery of new mathematical insights but also fostering a more collaborative and inclusive research environment. As this initiative continues to evolve, it holds the promise of unlocking even greater mathematical discoveries, paving the way for a deeper understanding of the mathematical universe.
Q&A
1. **What is FunSearch?**
FunSearch is a research initiative that leverages large language models to explore and discover new mathematical functions and relationships.
2. **How do large language models contribute to FunSearch?**
Large language models assist in FunSearch by analyzing vast amounts of mathematical data, identifying patterns, and suggesting potential new functions or theorems.
3. **What are the primary goals of FunSearch?**
The primary goals of FunSearch are to uncover novel mathematical insights, enhance our understanding of existing mathematical concepts, and potentially solve longstanding mathematical problems.
4. **What types of mathematical discoveries has FunSearch made?**
FunSearch has made discoveries in areas such as number theory, algebra, and calculus, including new functions, conjectures, and proofs.
5. **Who can benefit from the findings of FunSearch?**
Mathematicians, researchers, educators, and students can benefit from FunSearch’s findings, as they provide new tools and perspectives for mathematical exploration and education.
6. **What are the future prospects of FunSearch?**
The future prospects of FunSearch include expanding its database, refining its algorithms, and collaborating with mathematicians to validate and apply its discoveries in various fields.FunSearch leverages large language models to explore and uncover new mathematical discoveries, demonstrating the potential of AI in advancing mathematical research. By utilizing the computational power and pattern recognition capabilities of these models, FunSearch can identify novel mathematical relationships and conjectures that may not be immediately apparent to human researchers. This approach not only accelerates the discovery process but also opens up new avenues for collaboration between AI and mathematicians, ultimately enriching the field with innovative insights and expanding the boundaries of mathematical knowledge.
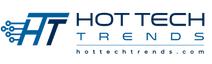