FACTS Grounding is a novel framework designed to enhance the evaluation of factual accuracy in large language models (LLMs). As the reliance on LLMs for information dissemination grows, ensuring the reliability and truthfulness of their outputs becomes paramount. FACTS Grounding introduces a systematic approach to assess how well these models align with verified facts, providing a standardized methodology for researchers and developers. By focusing on the grounding of information in credible sources, FACTS Grounding aims to mitigate the risks of misinformation and improve the overall trustworthiness of LLMs in various applications, from education to content creation. This initiative represents a significant step towards establishing rigorous benchmarks for factual accuracy, ultimately fostering greater accountability in the deployment of AI technologies.
Understanding FACTS Grounding: A New Approach to Factual Accuracy
In recent years, the proliferation of large language models (LLMs) has transformed the landscape of natural language processing, enabling unprecedented capabilities in text generation, translation, and summarization. However, alongside these advancements, concerns regarding the factual accuracy of the information produced by these models have emerged. This has led to the development of FACTS Grounding, a novel framework designed to establish a new standard for assessing the factual accuracy of LLM outputs. By focusing on the grounding of facts within a structured context, FACTS Grounding aims to enhance the reliability of information generated by these models.
At its core, FACTS Grounding emphasizes the importance of verifying the factual basis of the content produced by LLMs. Traditional evaluation methods often rely on subjective assessments or anecdotal evidence, which can lead to inconsistencies and biases in determining accuracy. In contrast, FACTS Grounding introduces a systematic approach that incorporates verifiable sources and established criteria for factual validation. This method not only enhances the credibility of the information but also provides a clear framework for users to understand the basis of the claims made by the models.
One of the key components of FACTS Grounding is its focus on source attribution. By requiring LLMs to cite credible sources for the information they generate, this framework encourages transparency and accountability. Users can then trace the origins of the information, allowing them to assess its reliability independently. This is particularly crucial in an era where misinformation can spread rapidly, and the ability to discern fact from fiction is paramount. By grounding outputs in verifiable sources, FACTS Grounding seeks to mitigate the risks associated with the dissemination of false or misleading information.
Moreover, FACTS Grounding incorporates a multi-faceted evaluation process that considers various dimensions of factual accuracy. This includes not only the correctness of the information but also its relevance and contextual appropriateness. For instance, an LLM may generate a factually correct statement that, when placed in a specific context, may not align with the user’s intent or the surrounding discourse. By evaluating outputs through this comprehensive lens, FACTS Grounding ensures that the information is not only accurate but also meaningful and applicable to the user’s needs.
In addition to enhancing the assessment of factual accuracy, FACTS Grounding also serves as a guiding principle for the development of future LLMs. By establishing clear standards for factual validation, developers can create models that prioritize accuracy and reliability from the outset. This proactive approach not only improves the quality of the outputs but also fosters user trust in the technology. As users become more discerning about the information they consume, the demand for LLMs that adhere to rigorous standards of factual accuracy will likely increase.
In conclusion, FACTS Grounding represents a significant advancement in the quest for reliable information generated by large language models. By emphasizing source attribution, implementing a multi-dimensional evaluation process, and guiding future model development, this framework sets a new standard for assessing factual accuracy. As the reliance on LLMs continues to grow across various sectors, the importance of ensuring the integrity of the information they produce cannot be overstated. Through the adoption of FACTS Grounding, stakeholders can work towards a future where the outputs of language models are not only innovative but also trustworthy and grounded in factual reality.
The Importance of Factual Accuracy in Large Language Models
In recent years, large language models (LLMs) have gained significant traction across various sectors, from education to healthcare, due to their ability to generate human-like text and assist in complex tasks. However, as these models become increasingly integrated into decision-making processes, the importance of factual accuracy cannot be overstated. Factual accuracy serves as a cornerstone for the reliability and trustworthiness of information generated by LLMs, which, if compromised, can lead to misinformation and potentially harmful consequences.
The proliferation of LLMs has raised critical questions about the integrity of the information they produce. As these models are trained on vast datasets that encompass a wide range of topics, they can inadvertently propagate inaccuracies present in the training data. This phenomenon is particularly concerning in contexts where precise information is paramount, such as medical advice or legal guidance. Consequently, the need for a robust framework to assess and ensure the factual accuracy of LLM outputs has become increasingly urgent.
Moreover, the implications of disseminating inaccurate information extend beyond individual users; they can affect entire communities and industries. For instance, in the realm of journalism, the reliance on LLMs for content generation can lead to the spread of false narratives if the underlying data is flawed. This not only undermines public trust in media but also poses a risk to democratic processes by skewing public perception. Therefore, establishing a standard for evaluating the factual accuracy of LLMs is essential to mitigate these risks and uphold the integrity of information.
In addition to the societal implications, the economic ramifications of inaccurate information generated by LLMs cannot be ignored. Businesses that rely on these models for customer service, marketing, or data analysis may find themselves at a competitive disadvantage if they disseminate erroneous information. This can lead to financial losses, reputational damage, and a decline in customer trust. As such, organizations must prioritize the implementation of rigorous assessment mechanisms to ensure that the outputs of LLMs align with factual accuracy.
Furthermore, the challenge of ensuring factual accuracy is compounded by the rapid evolution of knowledge across various fields. As new discoveries are made and existing information is updated, LLMs must be capable of adapting to these changes. This necessitates a dynamic approach to training and evaluating these models, one that incorporates ongoing assessments of factual accuracy. By doing so, developers can create LLMs that not only generate coherent and contextually relevant text but also reflect the most current and accurate information available.
In light of these considerations, the establishment of a standardized framework for assessing the factual accuracy of LLMs, such as FACTS Grounding, is a critical step forward. This framework aims to provide a systematic approach to evaluating the reliability of information generated by these models, thereby fostering greater accountability among developers and users alike. By prioritizing factual accuracy, stakeholders can work towards creating a more informed society, where the benefits of LLMs can be harnessed without compromising the quality of information.
In conclusion, the importance of factual accuracy in large language models cannot be overstated. As these models continue to shape the way we access and interact with information, it is imperative that we establish rigorous standards for evaluating their outputs. By doing so, we can ensure that LLMs serve as reliable tools that enhance our understanding of the world, rather than sources of misinformation that undermine it.
How FACTS Grounding Enhances Model Reliability
In the rapidly evolving landscape of artificial intelligence, particularly in the realm of large language models (LLMs), the need for reliable and accurate outputs has never been more critical. As these models are increasingly integrated into various applications, from customer service to content generation, the potential for misinformation and inaccuracies poses significant challenges. This is where FACTS Grounding emerges as a pivotal framework, enhancing the reliability of LLMs by establishing a new standard for assessing factual accuracy. By implementing FACTS Grounding, developers and researchers can ensure that the information generated by these models is not only coherent but also verifiable and trustworthy.
One of the primary ways FACTS Grounding enhances model reliability is through its emphasis on evidence-based responses. Traditional LLMs often generate text based on patterns learned from vast datasets, which can lead to the propagation of inaccuracies if the underlying data contains misleading or false information. In contrast, FACTS Grounding requires models to substantiate their claims with credible sources. This shift towards an evidence-based approach not only improves the factual accuracy of the outputs but also instills a sense of accountability in the model’s responses. By demanding that models cite their sources, users can critically evaluate the information presented, fostering a more informed interaction between humans and machines.
Moreover, FACTS Grounding introduces a systematic methodology for evaluating the factual accuracy of model outputs. This methodology involves rigorous testing against established benchmarks and datasets, allowing for a more nuanced understanding of a model’s performance in real-world scenarios. By employing a standardized assessment framework, researchers can identify specific areas where a model may falter, enabling targeted improvements. This iterative process of evaluation and refinement is essential for developing LLMs that not only meet but exceed user expectations in terms of reliability and accuracy.
In addition to enhancing factual accuracy, FACTS Grounding also addresses the issue of bias in language models. Bias can manifest in various forms, often leading to skewed or misleading outputs that do not reflect a balanced perspective. By grounding responses in verified facts and diverse sources, FACTS Grounding mitigates the risk of perpetuating biases that may exist within the training data. This commitment to diversity and accuracy not only enriches the model’s outputs but also promotes ethical considerations in AI development, ensuring that the technology serves a broader audience without marginalizing any group.
Furthermore, the implementation of FACTS Grounding can significantly improve user trust in LLMs. As users become more aware of the potential for misinformation, their skepticism towards AI-generated content increases. By providing a framework that prioritizes factual accuracy and transparency, FACTS Grounding can help bridge the trust gap between users and AI systems. When users can see the sources behind the information presented, they are more likely to engage with the content and rely on it for decision-making processes.
In conclusion, FACTS Grounding represents a transformative approach to enhancing the reliability of large language models. By prioritizing evidence-based responses, establishing systematic evaluation methodologies, addressing bias, and fostering user trust, this framework sets a new standard for assessing the factual accuracy of AI-generated content. As the reliance on LLMs continues to grow across various sectors, the adoption of FACTS Grounding will be crucial in ensuring that these models not only perform effectively but also uphold the integrity and reliability that users expect. Through this commitment to factual accuracy, the future of AI can be shaped into one that is both innovative and responsible.
Comparing Traditional Assessment Methods with FACTS Grounding
In the rapidly evolving landscape of artificial intelligence, particularly in the realm of large language models (LLMs), the need for robust assessment methods has become increasingly critical. Traditional assessment methods, which often rely on subjective evaluations or simplistic metrics, have proven inadequate in capturing the nuanced capabilities and limitations of these sophisticated systems. As a response to this challenge, FACTS Grounding emerges as a pioneering framework designed to establish a new standard for evaluating the factual accuracy of LLMs. By comparing traditional assessment methods with FACTS Grounding, we can better understand the advantages and implications of this innovative approach.
Traditional assessment methods typically involve human evaluators who assess the outputs of LLMs based on criteria such as coherence, relevance, and fluency. While these criteria are essential for determining the overall quality of generated text, they often overlook the critical aspect of factual accuracy. Consequently, an LLM may produce text that is coherent and fluent yet contains significant factual inaccuracies. This limitation highlights the need for a more rigorous and systematic approach to evaluation, one that prioritizes factual correctness alongside other qualitative measures.
In contrast, FACTS Grounding introduces a structured methodology that emphasizes the verification of factual claims made by LLMs. This framework incorporates a multi-faceted approach, utilizing external knowledge sources and verification tools to assess the accuracy of information presented in generated text. By grounding the evaluation process in verifiable facts, FACTS Grounding not only enhances the reliability of assessments but also provides a clearer understanding of an LLM’s performance in real-world applications. This shift from subjective evaluation to fact-based assessment represents a significant advancement in the field.
Moreover, traditional methods often rely on small, curated datasets for evaluation, which can lead to biased results that do not accurately reflect an LLM’s capabilities across diverse contexts. In contrast, FACTS Grounding advocates for the use of larger, more representative datasets that encompass a wide range of topics and factual claims. This broader approach allows for a more comprehensive evaluation of an LLM’s performance, ensuring that it can reliably generate accurate information across various domains. By addressing the limitations of traditional methods, FACTS Grounding paves the way for more meaningful assessments that can inform the development and deployment of LLMs.
Another critical distinction between traditional assessment methods and FACTS Grounding lies in the feedback loop established by the latter. Traditional evaluations often conclude with a one-time assessment, leaving little room for iterative improvement. In contrast, FACTS Grounding fosters a continuous feedback mechanism, enabling developers to refine their models based on factual accuracy assessments. This iterative process not only enhances the quality of LLMs over time but also encourages a culture of accountability within the AI development community.
Furthermore, the implications of adopting FACTS Grounding extend beyond mere assessment. By prioritizing factual accuracy, this framework encourages developers to focus on the integrity of the information their models generate. This shift in focus is particularly crucial in an era where misinformation can spread rapidly, leading to significant societal consequences. By establishing a new standard for evaluating LLMs, FACTS Grounding not only enhances the reliability of these systems but also contributes to the broader goal of promoting responsible AI development.
In conclusion, the comparison between traditional assessment methods and FACTS Grounding reveals a clear need for a more rigorous and fact-based approach to evaluating large language models. By emphasizing factual accuracy, utilizing diverse datasets, and fostering continuous improvement, FACTS Grounding sets a new standard that promises to enhance the reliability and integrity of LLMs in an increasingly complex information landscape. As the field of artificial intelligence continues to advance, embracing such innovative frameworks will be essential for ensuring that these powerful tools serve society effectively and responsibly.
Implementing FACTS Grounding in AI Development
The implementation of FACTS Grounding in AI development represents a significant advancement in the pursuit of ensuring factual accuracy in large language models. As the reliance on these models grows across various sectors, the need for a robust framework to assess and enhance their reliability becomes increasingly critical. FACTS Grounding provides a structured approach that not only evaluates the factual correctness of the information generated by these models but also establishes a new standard for their development and deployment.
To begin with, the integration of FACTS Grounding into the AI development process necessitates a comprehensive understanding of the underlying principles that govern factual accuracy. This involves a multi-faceted approach that includes the identification of credible sources, the establishment of verification protocols, and the incorporation of feedback mechanisms. By prioritizing these elements, developers can create models that are not only capable of generating coherent and contextually relevant responses but also grounded in verifiable facts. This shift towards a more rigorous assessment framework is essential, as it addresses the growing concerns regarding misinformation and the potential consequences of deploying models that lack factual integrity.
Moreover, the implementation of FACTS Grounding requires collaboration among various stakeholders, including researchers, developers, and domain experts. This collaborative effort is crucial for developing a shared understanding of what constitutes factual accuracy in different contexts. By engaging with experts from diverse fields, AI developers can ensure that their models are equipped with the necessary knowledge to navigate complex subject matter. This interdisciplinary approach not only enhances the factual grounding of the models but also fosters a culture of accountability within the AI development community.
In addition to collaboration, the use of advanced technologies plays a pivotal role in the successful implementation of FACTS Grounding. Leveraging techniques such as natural language processing, machine learning, and data mining can significantly enhance the ability of models to discern factual information from a vast array of sources. By employing these technologies, developers can create systems that are not only adept at generating human-like text but also proficient in cross-referencing information against established databases and repositories. This capability is particularly important in an era where the volume of information available online is overwhelming, making it challenging to ascertain the accuracy of any given statement.
Furthermore, the establishment of clear metrics for evaluating factual accuracy is a critical component of FACTS Grounding. By defining specific criteria for assessment, developers can systematically measure the performance of their models in terms of factual correctness. This quantitative approach allows for continuous improvement, as developers can identify areas where models may fall short and implement targeted interventions to enhance their accuracy. Additionally, the establishment of benchmarks can facilitate comparisons across different models, promoting a competitive environment that encourages innovation and excellence in factual grounding.
Ultimately, the successful implementation of FACTS Grounding in AI development has the potential to transform the landscape of large language models. By prioritizing factual accuracy and establishing a rigorous framework for assessment, developers can create models that not only meet the demands of users but also contribute positively to the broader discourse surrounding information integrity. As the field of artificial intelligence continues to evolve, the commitment to grounding models in verifiable facts will be essential in building trust and ensuring that these powerful tools serve as reliable sources of information in an increasingly complex world. In this way, FACTS Grounding not only sets a new standard for AI development but also paves the way for a future where technology and truth coexist harmoniously.
Future Implications of FACTS Grounding for AI Ethics and Accountability
The emergence of large language models (LLMs) has revolutionized the landscape of artificial intelligence, offering unprecedented capabilities in natural language processing and generation. However, with these advancements come significant ethical considerations, particularly concerning the accuracy and reliability of the information produced by these models. In this context, FACTS Grounding represents a pivotal development aimed at establishing a new standard for assessing the factual accuracy of LLMs. The implications of this framework extend far beyond technical enhancements; they touch upon the core principles of AI ethics and accountability.
To begin with, the implementation of FACTS Grounding is likely to enhance the transparency of LLMs. By providing a structured approach to evaluate the factual accuracy of generated content, stakeholders can better understand how these models arrive at their conclusions. This transparency is crucial, as it allows users to discern the reliability of the information presented, thereby fostering a more informed public discourse. As society increasingly relies on AI-generated content for decision-making, the ability to trace the factual basis of such information becomes paramount. Consequently, FACTS Grounding could serve as a benchmark for developers and researchers, encouraging them to prioritize accuracy in their models.
Moreover, the ethical implications of FACTS Grounding extend to the accountability of AI developers and organizations. As LLMs become more integrated into various sectors, including education, healthcare, and journalism, the potential for misinformation grows. By adopting a rigorous framework for assessing factual accuracy, organizations can be held accountable for the outputs of their models. This accountability is essential in mitigating the risks associated with the dissemination of false or misleading information. In this regard, FACTS Grounding not only promotes ethical practices among developers but also empowers users to demand higher standards from AI systems.
In addition to enhancing transparency and accountability, FACTS Grounding has the potential to influence regulatory frameworks surrounding AI. As governments and regulatory bodies grapple with the implications of AI technologies, the establishment of clear standards for factual accuracy could inform policy decisions. By integrating FACTS Grounding into regulatory guidelines, policymakers can create a more robust framework that addresses the ethical challenges posed by LLMs. This alignment between technical standards and regulatory measures could lead to a more responsible deployment of AI technologies, ultimately benefiting society as a whole.
Furthermore, the adoption of FACTS Grounding may catalyze a cultural shift within the AI community. As the demand for ethical AI practices grows, developers may increasingly prioritize the integration of factual accuracy assessments in their work. This shift could foster a collaborative environment where researchers share best practices and methodologies for grounding LLM outputs in verifiable facts. Such collaboration would not only enhance the quality of AI-generated content but also contribute to a collective understanding of the ethical responsibilities inherent in AI development.
In conclusion, the future implications of FACTS Grounding for AI ethics and accountability are profound. By establishing a new standard for assessing the factual accuracy of large language models, this framework promotes transparency, accountability, and responsible AI practices. As society continues to navigate the complexities of AI technologies, the principles embedded in FACTS Grounding will be instrumental in shaping a more ethical and accountable landscape. Ultimately, the successful integration of these standards could lead to a future where AI serves as a reliable partner in human decision-making, fostering trust and integrity in the information age.
Q&A
1. **What is FACTS Grounding?**
FACTS Grounding is a framework designed to assess the factual accuracy of large language models by establishing a standardized method for evaluating their outputs against reliable sources.
2. **Why is FACTS Grounding important?**
It is important because it helps ensure that language models provide accurate and trustworthy information, reducing the risk of misinformation and enhancing their reliability in various applications.
3. **How does FACTS Grounding evaluate factual accuracy?**
It evaluates factual accuracy by comparing the model’s outputs to verified data from credible sources, using metrics that quantify the degree of alignment between the two.
4. **What are the key components of the FACTS Grounding framework?**
The key components include a set of criteria for assessing factual claims, a methodology for sourcing reliable information, and metrics for measuring accuracy and consistency.
5. **What challenges does FACTS Grounding address?**
It addresses challenges such as the prevalence of misinformation, the difficulty in verifying claims made by language models, and the need for a systematic approach to evaluate their outputs.
6. **How can FACTS Grounding impact the development of future language models?**
It can guide the development of more accurate and reliable language models by providing benchmarks for factual accuracy, encouraging improvements in training data quality, and fostering accountability in AI-generated content.FACTS Grounding establishes a new benchmark for evaluating the factual accuracy of large language models by providing a structured framework that emphasizes the importance of verifiable information. This approach enhances the reliability of AI-generated content, ensuring that outputs are not only coherent but also factually correct. By prioritizing factual grounding, FACTS Grounding aims to mitigate misinformation and improve the overall trustworthiness of language models in various applications.
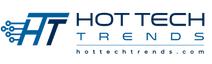