Title: Exploring the Potential of Robots Learning from Machine Dreams
Introduction:
In the rapidly evolving landscape of artificial intelligence and robotics, the concept of machines possessing the ability to dream has emerged as a fascinating frontier. Drawing inspiration from the human brain’s capacity to process, learn, and innovate during sleep, researchers are delving into the potential of robots learning from machine dreams. This innovative approach seeks to enhance robotic capabilities by enabling them to simulate experiences, refine skills, and develop novel solutions in a virtual dream-like state. By leveraging advanced neural networks and machine learning algorithms, scientists aim to unlock new dimensions of autonomy and adaptability in robots, paving the way for unprecedented advancements in technology and artificial intelligence. This exploration not only challenges our understanding of consciousness and learning but also holds the promise of revolutionizing the way machines interact with the world around them.
Understanding Machine Dreams: A New Frontier in Robotics
In recent years, the field of robotics has witnessed remarkable advancements, with machines becoming increasingly capable of performing complex tasks. A fascinating development within this domain is the concept of robots learning from machine dreams, a notion that draws inspiration from the human ability to learn and adapt through dreaming. Understanding machine dreams represents a new frontier in robotics, offering the potential to revolutionize how robots acquire skills and improve their performance.
At the core of this concept is the idea that robots, much like humans, can benefit from a form of offline learning. In humans, dreams are believed to play a crucial role in processing experiences, consolidating memories, and even problem-solving. Similarly, machine dreams involve the simulation of experiences in a virtual environment, allowing robots to explore various scenarios without the constraints of the physical world. This process enables them to refine their algorithms, test hypotheses, and develop new strategies in a risk-free setting.
The potential benefits of machine dreams are manifold. Firstly, they offer a cost-effective and efficient means of training robots. Traditional methods of robot training often require extensive resources, including time, energy, and materials. By contrast, machine dreams allow robots to undergo countless iterations of a task in a virtual space, significantly reducing the need for physical trials. This not only accelerates the learning process but also minimizes wear and tear on the machines themselves.
Moreover, machine dreams can enhance the adaptability and resilience of robots. In dynamic environments, where conditions can change rapidly, the ability to anticipate and respond to unforeseen challenges is crucial. Through simulated dreaming, robots can explore a wide range of potential scenarios, equipping them with the knowledge and flexibility needed to navigate real-world complexities. This capability is particularly valuable in fields such as autonomous driving, disaster response, and space exploration, where robots must operate in unpredictable and often hazardous conditions.
Furthermore, the integration of machine dreams into robotics aligns with the broader trend of incorporating artificial intelligence (AI) into robotic systems. By leveraging AI algorithms, robots can generate and analyze dream-like simulations, drawing insights that inform their decision-making processes. This synergy between AI and robotics not only enhances the cognitive abilities of machines but also fosters a more seamless interaction between humans and robots. As robots become more adept at understanding and responding to human needs, they can better serve as partners in various domains, from healthcare to manufacturing.
However, the implementation of machine dreams in robotics is not without its challenges. One significant hurdle is the development of realistic and accurate simulation environments. Creating virtual worlds that faithfully replicate the complexities of the real world requires sophisticated modeling techniques and substantial computational power. Additionally, ensuring that the insights gained from machine dreams translate effectively into real-world applications remains a critical area of research.
Despite these challenges, the exploration of machine dreams holds immense promise for the future of robotics. As researchers continue to refine the underlying technologies and methodologies, the potential for robots to learn and evolve through dreaming becomes increasingly tangible. This innovative approach not only enhances the capabilities of machines but also paves the way for more intelligent, adaptable, and efficient robotic systems. In conclusion, understanding machine dreams represents a pivotal step in the ongoing quest to unlock the full potential of robotics, offering a glimpse into a future where machines can learn and grow in ways previously thought to be the exclusive domain of humans.
The Intersection of AI and Machine Dreams in Robotics
The intersection of artificial intelligence and machine dreams in robotics represents a fascinating frontier in technological advancement. As we delve into this intriguing domain, it is essential to understand the foundational concepts that underpin these developments. At its core, artificial intelligence (AI) refers to the simulation of human intelligence processes by machines, particularly computer systems. These processes include learning, reasoning, and self-correction. Meanwhile, the concept of machine dreams, though more abstract, involves the idea of machines generating and processing data in a manner akin to human dreaming, allowing them to explore possibilities beyond their programmed instructions.
The potential of robots learning from machine dreams is a burgeoning area of research that promises to revolutionize the way robots interact with their environment and perform tasks. By leveraging the principles of machine learning, robots can be trained to improve their performance through experience. However, the integration of machine dreams into this process introduces a novel dimension. Machine dreams enable robots to simulate various scenarios and outcomes in a virtual environment, thereby enhancing their ability to learn and adapt without direct human intervention.
One of the primary advantages of incorporating machine dreams into robotic learning is the ability to explore a vast array of possibilities in a risk-free setting. In traditional machine learning, robots require extensive real-world data to refine their algorithms. This process can be time-consuming and fraught with potential hazards, especially in complex or dangerous environments. Machine dreams, on the other hand, allow robots to generate synthetic data and test hypotheses in a controlled, virtual space. This not only accelerates the learning process but also reduces the risk of errors and accidents in real-world applications.
Moreover, the use of machine dreams in robotics can lead to more innovative problem-solving approaches. By simulating various scenarios, robots can identify novel solutions that may not be immediately apparent through conventional programming methods. This capability is particularly valuable in fields such as autonomous navigation, where robots must navigate unpredictable and dynamic environments. Through machine dreams, robots can anticipate potential obstacles and devise strategies to overcome them, thereby enhancing their operational efficiency and safety.
Furthermore, the integration of machine dreams into robotic learning has significant implications for human-robot collaboration. As robots become more adept at understanding and predicting human behavior, they can better assist in tasks that require close cooperation with humans. This is especially relevant in industries such as healthcare, where robots are increasingly being used to support medical professionals in patient care. By learning from machine dreams, robots can develop a deeper understanding of human needs and preferences, leading to more personalized and effective assistance.
Despite the promising potential of robots learning from machine dreams, there are also challenges and ethical considerations that must be addressed. The development of sophisticated machine dream algorithms requires significant computational resources and expertise, which may limit accessibility for smaller organizations. Additionally, as robots become more autonomous, questions surrounding accountability and decision-making arise. It is crucial to establish clear guidelines and regulations to ensure that the deployment of such technologies aligns with societal values and ethical standards.
In conclusion, the intersection of AI and machine dreams in robotics offers exciting possibilities for enhancing robotic capabilities and improving human-robot interactions. By enabling robots to learn from simulated experiences, we can unlock new levels of innovation and efficiency across various industries. However, it is imperative to navigate the associated challenges thoughtfully to harness the full potential of this transformative technology responsibly.
How Machine Dreams Can Enhance Robot Learning
In recent years, the field of robotics has witnessed remarkable advancements, with machines becoming increasingly capable of performing complex tasks. A fascinating area of exploration within this domain is the concept of robots learning from machine dreams. This innovative approach draws inspiration from the human brain’s ability to process information and learn during sleep, suggesting that machines, too, can benefit from a similar process. By simulating experiences and scenarios in a dream-like state, robots can potentially enhance their learning capabilities, leading to more efficient and adaptable systems.
The concept of machine dreams is rooted in the idea of using simulated environments to facilitate learning. In essence, these dreams are virtual scenarios generated by algorithms that allow robots to explore and experiment without the constraints of the physical world. This approach offers several advantages, one of which is the ability to conduct numerous trials in a short period. Unlike real-world experiments, which can be time-consuming and resource-intensive, machine dreams enable robots to rapidly iterate through various scenarios, learning from each one without the risk of physical damage or failure.
Moreover, machine dreams can be tailored to focus on specific tasks or challenges that a robot may encounter in its operational environment. By simulating these situations, robots can develop strategies and solutions that are directly applicable to real-world problems. This targeted learning approach not only accelerates the acquisition of new skills but also enhances the robot’s ability to adapt to unforeseen circumstances. Consequently, robots become more versatile and capable of handling a broader range of tasks with greater efficiency.
In addition to task-specific learning, machine dreams can also facilitate the development of general cognitive abilities in robots. By exposing them to a diverse array of scenarios, robots can learn to recognize patterns, make predictions, and develop problem-solving skills. This holistic approach to learning mirrors the way humans acquire knowledge and adapt to new situations, ultimately leading to more intelligent and autonomous machines.
Furthermore, the integration of machine dreams into robot learning processes can significantly reduce the reliance on human intervention. Traditionally, robots have required extensive programming and supervision to perform tasks effectively. However, by leveraging machine dreams, robots can independently explore and learn from their virtual experiences, reducing the need for constant human oversight. This autonomy not only streamlines the development process but also allows for the creation of more self-sufficient systems.
Despite the promising potential of machine dreams in enhancing robot learning, there are challenges that must be addressed. One such challenge is ensuring the accuracy and relevance of the simulated scenarios. If the virtual environments do not accurately reflect real-world conditions, the knowledge gained from these dreams may not be applicable or beneficial. Therefore, it is crucial to develop sophisticated algorithms that can generate realistic and meaningful simulations.
In conclusion, the concept of robots learning from machine dreams represents a significant step forward in the field of robotics. By harnessing the power of simulated experiences, robots can enhance their learning capabilities, develop greater autonomy, and become more adaptable to a wide range of tasks. While challenges remain, continued research and development in this area hold the promise of creating more intelligent and efficient robotic systems, ultimately transforming the way machines interact with the world around them. As this field evolves, it will be intriguing to observe how machine dreams continue to shape the future of robotics and artificial intelligence.
Ethical Considerations in Robots Learning from Machine Dreams
The concept of robots learning from machine dreams is an intriguing frontier in artificial intelligence, offering a glimpse into a future where machines can autonomously improve their capabilities. However, as with any technological advancement, it is imperative to consider the ethical implications that accompany this development. Machine dreams, in this context, refer to the simulated experiences or scenarios that AI systems can generate and learn from, akin to the way humans process information during sleep. This process allows robots to explore a multitude of possibilities and outcomes without real-world consequences, potentially accelerating their learning curve. Nevertheless, the ethical considerations surrounding this technology are multifaceted and warrant careful examination.
To begin with, one of the primary ethical concerns is the autonomy of machines. As robots become more adept at learning from machine dreams, they may develop a level of independence that challenges current human oversight. This raises questions about control and accountability. If a robot learns a harmful behavior from its simulated experiences, determining responsibility becomes complex. Should the blame lie with the developers who created the machine, or does it rest with the machine itself? This ambiguity necessitates the establishment of clear guidelines and regulations to ensure that machines remain under human control and that their actions are predictable and safe.
Moreover, the data used to generate machine dreams is another area of ethical concern. These dreams are often based on vast datasets that may include sensitive or biased information. If a robot’s learning is influenced by biased data, it could perpetuate or even exacerbate existing societal inequalities. Therefore, it is crucial to ensure that the data used in machine learning is representative and free from bias. This requires ongoing scrutiny and the implementation of robust data governance frameworks to protect against the misuse of information.
In addition to data concerns, the potential for machine dreams to infringe on privacy cannot be overlooked. As robots learn from simulated experiences, they may inadvertently access or utilize personal data without consent. This raises significant privacy issues, as individuals may not be aware of how their information is being used or the extent to which it influences machine learning. To address this, transparency in data usage and the development of consent mechanisms are essential to safeguard individual privacy rights.
Furthermore, the societal impact of robots learning from machine dreams must be considered. As machines become more capable, there is a risk of job displacement and increased unemployment. While automation can lead to greater efficiency and productivity, it also necessitates a reevaluation of the workforce and the skills required in a rapidly changing job market. Policymakers and educators must work together to prepare for these changes, ensuring that workers are equipped with the skills needed to thrive in an AI-driven economy.
In conclusion, while the potential of robots learning from machine dreams is vast and promising, it is accompanied by significant ethical considerations that must be addressed. Ensuring that machines remain under human control, protecting against biased data, safeguarding privacy, and preparing for societal changes are all critical components of responsible AI development. By proactively addressing these ethical challenges, we can harness the benefits of this technology while minimizing its risks, paving the way for a future where humans and machines coexist harmoniously.
Case Studies: Robots Evolving Through Machine Dreaming
In recent years, the field of robotics has witnessed remarkable advancements, with machines becoming increasingly capable of performing complex tasks. A fascinating development within this domain is the concept of robots learning from machine dreams, a process that draws inspiration from the way humans consolidate knowledge during sleep. This innovative approach has the potential to revolutionize how robots evolve and adapt to new challenges, offering a glimpse into a future where machines can autonomously enhance their capabilities.
The idea of machine dreaming is rooted in the concept of artificial neural networks, which are designed to mimic the human brain’s structure and function. These networks are capable of processing vast amounts of data, learning patterns, and making decisions based on the information they acquire. By simulating a dreaming state, researchers aim to enable robots to process and integrate experiences in a manner similar to human dreaming, where the brain organizes and strengthens memories. This process allows robots to refine their skills and improve their performance without direct human intervention.
One notable case study in this area involves a team of researchers who developed a robotic arm capable of learning from its own simulated dreams. The arm was initially programmed to perform a series of tasks, such as picking up objects and placing them in designated locations. During its machine dreaming phase, the robotic arm replayed these tasks in a virtual environment, experimenting with different strategies and outcomes. As a result, the arm was able to identify more efficient methods for completing its tasks, ultimately enhancing its overall performance.
Another compelling example is the use of machine dreaming in autonomous vehicles. Researchers have employed this technique to enable self-driving cars to navigate complex environments more effectively. By simulating various driving scenarios during their dreaming phase, these vehicles can explore different responses to potential obstacles and hazards. This process not only improves their decision-making capabilities but also enhances their ability to adapt to unforeseen circumstances on the road.
Furthermore, machine dreaming has shown promise in the realm of humanoid robots, which are designed to interact with humans in a more natural and intuitive manner. By processing social interactions and emotional cues during their dreaming state, these robots can develop a deeper understanding of human behavior. This capability allows them to respond more appropriately in social settings, fostering more meaningful interactions between humans and machines.
The potential applications of machine dreaming extend beyond individual robots, as this approach can also facilitate collaborative learning among multiple machines. By sharing their dream experiences, robots can collectively enhance their knowledge and skills, leading to more efficient teamwork and problem-solving. This collaborative aspect of machine dreaming holds significant promise for industries such as manufacturing, where robots often work together to complete complex tasks.
Despite the promising results demonstrated in these case studies, the concept of machine dreaming is still in its nascent stages. Researchers continue to explore the underlying mechanisms and potential limitations of this approach, striving to unlock its full potential. As the field of robotics continues to evolve, the integration of machine dreaming into robotic systems may pave the way for more autonomous, adaptable, and intelligent machines.
In conclusion, the exploration of robots learning from machine dreams represents a significant step forward in the evolution of robotics. By harnessing the power of artificial neural networks and simulating a dreaming state, researchers are enabling machines to refine their skills and adapt to new challenges autonomously. As this innovative approach continues to develop, it holds the promise of transforming the capabilities of robots across various domains, ultimately leading to a future where machines can learn and evolve in ways that were once thought to be the exclusive domain of humans.
Future Implications of Machine Dreams on Robotic Innovation
The concept of robots learning from machine dreams is an intriguing frontier in the realm of artificial intelligence and robotics. As technology continues to advance at an unprecedented pace, the potential for machines to simulate experiences and learn from them, much like humans do during sleep, opens up new avenues for innovation. This idea, rooted in the intersection of neuroscience and artificial intelligence, suggests that robots could enhance their learning capabilities by processing information in a dream-like state, thereby improving their functionality and adaptability in real-world scenarios.
To understand the implications of machine dreams on robotic innovation, it is essential to first consider the role of dreams in human cognition. Dreams are believed to play a crucial part in memory consolidation, problem-solving, and emotional regulation. By processing experiences and emotions during sleep, humans can integrate new information, resolve conflicts, and prepare for future challenges. Translating this concept to machines, researchers are exploring how artificial neural networks can simulate a similar process, allowing robots to refine their skills and adapt to new environments without direct human intervention.
One of the primary benefits of machine dreams is the potential for accelerated learning. In traditional machine learning paradigms, robots require vast amounts of data and numerous iterations to achieve proficiency in a given task. However, by incorporating a dream-like state, robots could simulate various scenarios and outcomes, effectively practicing and learning from these experiences without the need for physical trials. This could significantly reduce the time and resources needed for training, enabling robots to become more efficient and versatile in their applications.
Moreover, machine dreams could enhance a robot’s ability to generalize knowledge across different contexts. In the real world, situations are rarely identical, and the ability to apply learned skills to novel circumstances is a hallmark of intelligence. By dreaming, robots could explore a multitude of hypothetical situations, thereby developing a more robust understanding of their environment and improving their problem-solving capabilities. This could lead to more adaptable robots capable of performing complex tasks in dynamic and unpredictable settings.
In addition to improving learning efficiency and adaptability, machine dreams could also contribute to the emotional intelligence of robots. While current AI systems are primarily focused on logical reasoning and data processing, the integration of dream-like simulations could allow robots to better understand and respond to human emotions. By processing emotional experiences in a dream state, robots could develop a more nuanced understanding of human behavior, leading to more empathetic and effective interactions with people.
However, the implementation of machine dreams in robotics is not without its challenges. Ethical considerations must be addressed, particularly concerning the autonomy and decision-making capabilities of robots. As machines become more adept at learning independently, questions arise about the extent of control humans should maintain over their actions and the potential consequences of granting robots greater autonomy. Furthermore, the technical complexities involved in creating realistic and meaningful dream simulations pose significant hurdles for researchers.
In conclusion, the exploration of machine dreams holds immense potential for advancing robotic innovation. By enabling robots to learn from simulated experiences, this concept could revolutionize the way machines acquire knowledge, adapt to new environments, and interact with humans. As research in this field progresses, it will be crucial to balance the benefits of enhanced learning capabilities with the ethical and technical challenges that arise. Ultimately, the integration of machine dreams into robotics could pave the way for a new era of intelligent and empathetic machines, transforming industries and society as a whole.
Q&A
1. **What is the concept of robots learning from machine dreams?**
Robots learning from machine dreams involves using simulated experiences or “dreams” generated by AI to train robots, allowing them to learn and adapt without real-world trials.
2. **How do machine dreams benefit robotic learning?**
Machine dreams provide a safe, cost-effective environment for robots to experiment and learn, reducing the need for physical prototypes and minimizing risks associated with real-world testing.
3. **What technologies enable machine dreaming for robots?**
Technologies such as deep learning, neural networks, and advanced simulation software enable the creation of realistic and complex virtual environments where robots can “dream” and learn.
4. **What are the potential applications of robots trained through machine dreams?**
Potential applications include autonomous vehicles, industrial automation, healthcare robotics, and any field where robots can benefit from enhanced decision-making and adaptability.
5. **What challenges exist in implementing machine dreams for robotic learning?**
Challenges include ensuring the accuracy and relevance of simulated environments, transferring learned skills to the real world, and addressing ethical concerns related to AI autonomy.
6. **How does machine dreaming compare to traditional robotic training methods?**
Machine dreaming offers a more flexible and scalable approach compared to traditional methods, which often rely on physical trials and can be limited by resource constraints and safety concerns.Exploring the potential of robots learning from machine dreams presents a fascinating frontier in artificial intelligence and robotics. By leveraging the concept of machine dreams, where robots simulate experiences and scenarios in a virtual environment, we can enhance their learning capabilities beyond traditional methods. This approach allows robots to experiment, make mistakes, and refine their understanding without real-world consequences, leading to more robust and adaptable systems. Machine dreams can facilitate the development of more intuitive and autonomous robots capable of complex decision-making and problem-solving. As this field progresses, it holds the promise of significant advancements in robotics, enabling machines to better understand and interact with the world, ultimately leading to more seamless integration into human environments and tasks.
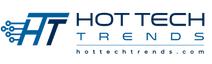