Deprecated: Implicit conversion from float 18.9 to int loses precision in /home/hottech/public_html/wp-content/plugins/internal-links/core/links/text-to-link-converter-factory.php on line 35
Deprecated: Implicit conversion from float 18.9 to int loses precision in /home/hottech/public_html/wp-content/plugins/internal-links/core/links/text-to-link-converter-factory.php on line 35
Exploring the future of AlphaFold’s next generation delves into the transformative potential of advanced protein structure prediction technologies. AlphaFold, developed by DeepMind, has already revolutionized the field of computational biology by accurately predicting protein structures, a task that was once considered one of the grand challenges in science. As we look to the future, the next generation of AlphaFold promises to further accelerate scientific discovery and innovation. This evolution is expected to enhance our understanding of complex biological processes, facilitate drug discovery, and enable the design of novel proteins with unprecedented precision. By integrating cutting-edge machine learning techniques, expanding its database, and improving its predictive accuracy, the future iterations of AlphaFold could unlock new frontiers in biotechnology and medicine, offering solutions to some of the most pressing health and environmental challenges of our time.
Advancements In Protein Structure Prediction
The field of protein structure prediction has witnessed a remarkable transformation with the advent of AlphaFold, a groundbreaking artificial intelligence system developed by DeepMind. This innovative tool has revolutionized our understanding of protein folding, a complex process that has long posed a significant challenge to scientists. As we explore the future of AlphaFold’s next generation, it is essential to consider the advancements that have been made and the potential implications for various scientific disciplines.
AlphaFold’s initial success was marked by its ability to predict protein structures with unprecedented accuracy, a feat that was previously thought to be decades away. This achievement was particularly significant given the complexity of proteins, which are composed of long chains of amino acids that fold into intricate three-dimensional shapes. The precise folding of these chains is crucial for the protein’s function, and any errors in this process can lead to diseases such as Alzheimer’s and cystic fibrosis. By accurately predicting protein structures, AlphaFold has opened new avenues for understanding these diseases at a molecular level, potentially leading to more effective treatments.
Building on this success, the next generation of AlphaFold promises to further enhance our capabilities in protein structure prediction. One of the key advancements is the integration of more sophisticated machine learning algorithms, which can process vast amounts of data more efficiently. This improvement allows for the analysis of more complex proteins and the prediction of their structures with even greater precision. Moreover, the incorporation of additional data sources, such as cryo-electron microscopy and nuclear magnetic resonance spectroscopy, provides a more comprehensive understanding of protein dynamics and interactions.
In addition to these technical advancements, the future of AlphaFold’s next generation is likely to be characterized by increased collaboration across scientific disciplines. The ability to predict protein structures accurately has implications beyond molecular biology, extending into fields such as drug discovery, bioengineering, and synthetic biology. For instance, in drug discovery, understanding the precise structure of target proteins can significantly accelerate the development of new therapeutics by identifying potential binding sites for drug molecules. Similarly, in bioengineering, the ability to design proteins with specific functions could lead to the creation of novel biomaterials with applications in medicine and industry.
Furthermore, the open-access nature of AlphaFold’s predictions has democratized the field of protein structure prediction, enabling researchers worldwide to benefit from its capabilities. This accessibility fosters a collaborative environment where scientists can share insights and build upon each other’s work, driving innovation and accelerating progress. As a result, the next generation of AlphaFold is poised to play a pivotal role in advancing our understanding of biology and addressing some of the most pressing challenges in health and disease.
In conclusion, the future of AlphaFold’s next generation holds immense promise for the field of protein structure prediction. With continued advancements in machine learning algorithms, integration of diverse data sources, and increased interdisciplinary collaboration, AlphaFold is set to further transform our understanding of proteins and their functions. As we look ahead, the potential applications of this technology are vast, offering new opportunities for scientific discovery and innovation. The journey of AlphaFold is a testament to the power of artificial intelligence in unraveling the complexities of the natural world, paving the way for a new era of research and discovery.
AlphaFold’s Impact On Drug Discovery
The advent of AlphaFold, a groundbreaking artificial intelligence system developed by DeepMind, has significantly transformed the landscape of drug discovery. By accurately predicting protein structures, AlphaFold has opened new avenues for understanding biological processes at a molecular level. This advancement is particularly crucial in drug discovery, where the precise knowledge of protein structures can lead to the development of more effective and targeted therapeutics. As we explore the future of AlphaFold’s next generation, it is essential to consider its profound impact on the pharmaceutical industry and the potential it holds for revolutionizing drug development.
Initially, drug discovery was a labor-intensive and time-consuming process, often involving trial and error to determine the structure of proteins. Traditional methods such as X-ray crystallography and nuclear magnetic resonance spectroscopy, while effective, are resource-intensive and not always feasible for all proteins. AlphaFold’s ability to predict protein structures with remarkable accuracy has alleviated these challenges, providing researchers with a powerful tool to accelerate the drug discovery process. Consequently, pharmaceutical companies can now identify potential drug targets more efficiently, reducing the time and cost associated with bringing new drugs to market.
Moreover, AlphaFold’s impact extends beyond mere efficiency. By providing detailed insights into protein structures, it enables researchers to understand the mechanisms of diseases at a molecular level. This understanding is crucial for designing drugs that can precisely target disease-causing proteins, thereby increasing the efficacy of treatments and minimizing side effects. For instance, in the case of neurodegenerative diseases such as Alzheimer’s, where protein misfolding plays a critical role, AlphaFold’s predictions can aid in the development of drugs that specifically target and correct these misfolded proteins.
As we look to the future, the next generation of AlphaFold promises even greater advancements. With continuous improvements in AI algorithms and computational power, future iterations of AlphaFold are expected to predict protein structures with even higher accuracy and speed. This progress will likely expand its applicability to more complex proteins and larger biological systems, further enhancing its utility in drug discovery. Additionally, the integration of AlphaFold with other AI-driven technologies, such as machine learning models for drug screening, could create a synergistic effect, streamlining the entire drug development pipeline.
Furthermore, the democratization of AlphaFold’s technology is poised to have a significant impact on global health. By making its predictions accessible to researchers worldwide, AlphaFold can empower scientists in resource-limited settings to contribute to drug discovery efforts. This democratization could lead to a more equitable distribution of medical advancements, addressing health disparities and improving outcomes for underserved populations.
In conclusion, AlphaFold’s impact on drug discovery is both profound and far-reaching. By transforming the way researchers understand and interact with protein structures, it has set the stage for a new era of precision medicine. As we anticipate the next generation of AlphaFold, the potential for further innovation and collaboration in the pharmaceutical industry is immense. With continued advancements, AlphaFold is poised to not only accelerate drug discovery but also to fundamentally change the way we approach the treatment of diseases, ultimately improving health outcomes on a global scale.
Integrating AlphaFold With Genomic Data
The integration of AlphaFold with genomic data represents a significant leap forward in the field of bioinformatics, promising to revolutionize our understanding of protein structures and their functions. AlphaFold, developed by DeepMind, has already made headlines by predicting protein structures with remarkable accuracy, a task that has traditionally been both time-consuming and resource-intensive. As we look to the future, the potential of combining AlphaFold’s capabilities with genomic data could unlock new insights into the complex mechanisms of life, offering unprecedented opportunities for scientific discovery and medical advancement.
To begin with, the synergy between AlphaFold and genomic data lies in the ability to predict protein structures directly from genetic sequences. Genomic data provides a comprehensive blueprint of an organism’s DNA, encoding the instructions for protein synthesis. By leveraging this information, AlphaFold can predict the three-dimensional structures of proteins, which are crucial for understanding their functions and interactions within biological systems. This integration could streamline the process of annotating genomes, allowing researchers to infer the functions of newly discovered genes more efficiently.
Moreover, the integration of AlphaFold with genomic data could enhance our understanding of genetic variations and their implications for health and disease. Genetic mutations can lead to alterations in protein structures, potentially disrupting their normal functions and leading to various diseases. By predicting how these mutations affect protein structures, researchers can gain insights into the molecular basis of diseases, paving the way for the development of targeted therapies and personalized medicine. This approach could be particularly beneficial in the field of oncology, where understanding the structural impact of mutations in cancer-related genes could inform the design of more effective treatments.
In addition to its applications in medicine, the integration of AlphaFold with genomic data holds promise for advancing our knowledge of evolutionary biology. By comparing protein structures across different species, scientists can trace the evolutionary history of proteins, shedding light on how they have adapted to perform specific functions over time. This could provide valuable insights into the mechanisms of evolution and the origins of complex biological systems. Furthermore, understanding the evolutionary relationships between proteins could aid in the identification of conserved structural motifs, which are often critical for protein function.
Despite these promising prospects, the integration of AlphaFold with genomic data also presents several challenges. One of the primary concerns is the sheer volume of genomic data available, which requires sophisticated computational tools and infrastructure to manage and analyze effectively. Additionally, while AlphaFold has demonstrated impressive accuracy in predicting protein structures, there are still limitations, particularly when it comes to modeling protein complexes and dynamic conformational changes. Addressing these challenges will require continued advancements in computational methods and collaboration across disciplines.
In conclusion, the integration of AlphaFold with genomic data represents a transformative opportunity for the life sciences. By combining the predictive power of AlphaFold with the wealth of information contained in genomic sequences, researchers can gain deeper insights into the structure and function of proteins, with far-reaching implications for medicine, evolutionary biology, and beyond. As we continue to refine these technologies and overcome existing challenges, the future holds great promise for unlocking the mysteries of the molecular world and improving human health.
AlphaFold And The Future Of Personalized Medicine
The advent of AlphaFold, a groundbreaking artificial intelligence system developed by DeepMind, has revolutionized the field of structural biology by accurately predicting protein structures. This innovation has not only accelerated scientific research but also opened new avenues in personalized medicine. As we explore the future of AlphaFold’s next generation, it is essential to consider how this technology could transform healthcare by tailoring medical treatments to individual patients.
AlphaFold’s ability to predict protein structures with remarkable accuracy has profound implications for understanding diseases at a molecular level. Proteins are fundamental to virtually all biological processes, and their structures determine their functions. Misfolded proteins are often implicated in diseases such as Alzheimer’s, Parkinson’s, and cystic fibrosis. By providing detailed insights into protein structures, AlphaFold enables researchers to identify potential therapeutic targets more efficiently. Consequently, this paves the way for the development of drugs that can specifically interact with these targets, offering more effective treatments with fewer side effects.
Transitioning from traditional approaches to personalized medicine involves leveraging AlphaFold’s capabilities to design therapies tailored to an individual’s unique genetic makeup. Personalized medicine aims to move away from the one-size-fits-all model, recognizing that genetic variations can significantly influence how patients respond to treatments. By integrating AlphaFold’s protein structure predictions with genomic data, healthcare providers can develop customized treatment plans that optimize efficacy and minimize adverse reactions. This approach holds promise for conditions such as cancer, where genetic mutations can drive disease progression and impact treatment outcomes.
Moreover, AlphaFold’s next generation could enhance the precision of diagnostic tools. Accurate protein structure predictions can aid in the identification of biomarkers—molecules that indicate the presence or progression of a disease. These biomarkers can be used to develop diagnostic tests that detect diseases at an earlier stage, improving the chances of successful intervention. For instance, in oncology, early detection of cancer-specific proteins could lead to timely and targeted treatments, significantly improving patient prognosis.
In addition to diagnostics and therapeutics, AlphaFold’s advancements could facilitate the development of novel vaccines. Understanding the structure of viral proteins is crucial for designing vaccines that elicit a robust immune response. AlphaFold’s ability to predict these structures can accelerate vaccine development, as demonstrated during the COVID-19 pandemic. By rapidly providing insights into the structure of the virus’s spike protein, AlphaFold contributed to the swift development of effective vaccines. As new pathogens emerge, this capability will be invaluable in responding to future public health threats.
While the potential of AlphaFold in personalized medicine is immense, several challenges must be addressed to fully realize its benefits. Integrating AlphaFold’s predictions with clinical data requires robust computational infrastructure and interdisciplinary collaboration among biologists, clinicians, and data scientists. Additionally, ethical considerations surrounding data privacy and the equitable distribution of personalized therapies must be carefully navigated to ensure that advancements in personalized medicine are accessible to all.
In conclusion, AlphaFold’s next generation holds the promise of transforming personalized medicine by providing unprecedented insights into protein structures. By enabling the development of targeted therapies, enhancing diagnostic precision, and accelerating vaccine design, AlphaFold has the potential to revolutionize healthcare. As we continue to explore its capabilities, it is crucial to address the associated challenges and ensure that the benefits of this technology are realized in a manner that is both ethical and equitable. The future of personalized medicine, powered by AlphaFold, is indeed a promising frontier in the quest for improved health outcomes.
Ethical Considerations In AI-Driven Biology
The advent of AlphaFold, an artificial intelligence system developed by DeepMind, has revolutionized the field of structural biology by predicting protein structures with remarkable accuracy. As we look toward the future of AlphaFold’s next generation, it is imperative to consider the ethical implications that accompany such groundbreaking advancements. The potential of AI-driven biology to transform scientific research and healthcare is immense, yet it also raises significant ethical questions that must be addressed to ensure responsible development and deployment.
To begin with, the accessibility of AlphaFold’s technology presents both opportunities and challenges. On one hand, making this technology widely available can democratize scientific research, enabling researchers from diverse backgrounds and institutions to access cutting-edge tools. This could accelerate discoveries in drug development, disease understanding, and synthetic biology. However, the widespread availability of such powerful technology also raises concerns about misuse. There is a risk that individuals or organizations with malicious intent could exploit AI-driven biology for harmful purposes, such as creating biological weapons or engaging in unethical genetic modifications. Therefore, it is crucial to establish robust regulatory frameworks and guidelines to govern the use of AI in biology, ensuring that its benefits are maximized while minimizing potential risks.
Moreover, the integration of AI systems like AlphaFold into biological research necessitates a reevaluation of data privacy and security. The vast amounts of biological data required to train and refine AI models pose significant privacy concerns. As these models become more sophisticated, they may inadvertently reveal sensitive information about individuals or populations. Consequently, it is essential to implement stringent data protection measures and ethical guidelines to safeguard personal and genetic information. Researchers and policymakers must collaborate to develop standards that balance the need for data sharing with the imperative to protect individual privacy.
In addition to privacy concerns, the reliance on AI-driven systems in biology raises questions about accountability and transparency. As AI models become more complex, understanding their decision-making processes becomes increasingly challenging. This opacity can lead to difficulties in attributing responsibility when errors occur or when AI-generated predictions are used in critical applications, such as clinical decision-making. To address this, it is vital to prioritize the development of explainable AI systems that provide insights into their reasoning processes. This transparency will not only enhance trust in AI-driven biology but also facilitate informed decision-making by researchers and clinicians.
Furthermore, the rapid advancement of AI in biology necessitates a reevaluation of the ethical frameworks that guide scientific research. Traditional ethical principles, such as beneficence, non-maleficence, autonomy, and justice, must be adapted to address the unique challenges posed by AI technologies. Engaging ethicists, scientists, and policymakers in ongoing dialogue is essential to ensure that ethical considerations keep pace with technological advancements. This collaborative approach will help identify potential ethical dilemmas early on and develop strategies to address them effectively.
In conclusion, while the future of AlphaFold’s next generation holds tremendous promise for advancing our understanding of biology and improving human health, it also presents significant ethical challenges that must be carefully navigated. By proactively addressing issues related to accessibility, data privacy, accountability, and ethical frameworks, we can harness the power of AI-driven biology responsibly and ethically. As we continue to explore the frontiers of this transformative technology, it is our collective responsibility to ensure that its development and application align with the values and principles that underpin ethical scientific research.
AlphaFold’s Role In Understanding Complex Diseases
AlphaFold, a groundbreaking artificial intelligence system developed by DeepMind, has revolutionized the field of structural biology by predicting protein structures with remarkable accuracy. This innovation has opened new avenues for understanding complex diseases, which often involve intricate interactions between numerous proteins. As we explore the future of AlphaFold’s next generation, it is essential to consider its potential role in unraveling the mysteries of these diseases and advancing medical research.
Proteins are fundamental to virtually all biological processes, and their functions are determined by their three-dimensional structures. Misfolded proteins or aberrant protein interactions can lead to a variety of complex diseases, including neurodegenerative disorders, cancers, and autoimmune conditions. Traditionally, determining protein structures has been a labor-intensive and time-consuming process, relying on techniques such as X-ray crystallography and nuclear magnetic resonance spectroscopy. However, AlphaFold’s ability to predict protein structures with high precision has significantly accelerated this process, providing researchers with a powerful tool to investigate the molecular underpinnings of disease.
As we look to the future, the next generation of AlphaFold promises to enhance our understanding of complex diseases even further. One potential area of impact is in the study of protein-protein interactions, which are critical in cellular signaling pathways and disease mechanisms. By accurately modeling these interactions, AlphaFold could help identify novel therapeutic targets and facilitate the design of drugs that can modulate these interactions effectively. This capability is particularly relevant in the context of diseases like cancer, where dysregulated signaling pathways often drive tumor growth and progression.
Moreover, AlphaFold’s advancements could play a pivotal role in personalized medicine. Complex diseases often exhibit significant variability among patients, influenced by genetic, environmental, and lifestyle factors. By integrating AlphaFold’s structural predictions with genomic and clinical data, researchers could gain insights into the specific protein alterations present in individual patients. This information could guide the development of tailored treatment strategies, improving patient outcomes and minimizing adverse effects.
In addition to its applications in drug discovery and personalized medicine, AlphaFold’s next generation could contribute to a deeper understanding of disease mechanisms at the systems level. Complex diseases are characterized by their multifactorial nature, involving networks of interacting proteins and pathways. By providing detailed structural information, AlphaFold could help elucidate these networks, revealing how perturbations in one part of the system can lead to cascading effects and disease manifestation. This systems-level understanding could inform the development of more comprehensive therapeutic approaches that address multiple aspects of disease pathology.
Furthermore, as AlphaFold continues to evolve, its integration with other emerging technologies, such as cryo-electron microscopy and machine learning algorithms, could enhance its predictive capabilities. These synergies could enable the exploration of dynamic protein conformations and transient interactions, which are often challenging to capture but crucial for understanding disease processes.
In conclusion, the future of AlphaFold’s next generation holds immense promise for advancing our understanding of complex diseases. By providing accurate protein structure predictions, it has the potential to transform drug discovery, personalize medical treatments, and unravel the intricate networks underlying disease mechanisms. As researchers continue to harness the power of AlphaFold, we can anticipate significant strides in the fight against complex diseases, ultimately leading to improved health outcomes and a deeper comprehension of the biological world.
Q&A
1. **Question:** What advancements are expected in the next generation of AlphaFold?
**Answer:** The next generation of AlphaFold is expected to improve in accuracy, speed, and the ability to predict protein structures in more complex environments, such as within cellular contexts or in interaction with other biomolecules.
2. **Question:** How might AlphaFold’s next generation impact drug discovery?
**Answer:** It could significantly accelerate drug discovery by providing more precise protein structure predictions, enabling researchers to identify potential drug targets and design effective molecules more efficiently.
3. **Question:** What challenges does AlphaFold face in predicting protein structures?
**Answer:** Challenges include predicting structures of proteins with few known homologs, modeling protein dynamics and flexibility, and accurately predicting protein-protein and protein-ligand interactions.
4. **Question:** How could AlphaFold’s next generation contribute to personalized medicine?
**Answer:** By providing detailed insights into individual protein variants, it could help tailor medical treatments to the specific molecular profiles of patients, improving the efficacy and safety of therapies.
5. **Question:** What role might machine learning play in the future development of AlphaFold?
**Answer:** Machine learning will continue to be crucial, with advancements in algorithms and computational power enhancing AlphaFold’s ability to learn from vast datasets and improve prediction accuracy.
6. **Question:** How could AlphaFold’s next generation influence our understanding of diseases?
**Answer:** It could provide deeper insights into the molecular mechanisms of diseases by revealing the structures of disease-related proteins, potentially leading to new therapeutic strategies and interventions.AlphaFold’s next generation holds immense potential for revolutionizing the field of structural biology and beyond. By advancing its capabilities in protein structure prediction, it could significantly accelerate drug discovery, enhance our understanding of complex biological processes, and facilitate the development of novel therapeutics. The integration of AlphaFold with other computational and experimental techniques may lead to more comprehensive models of cellular mechanisms and disease pathways. Furthermore, as the technology evolves, it could democratize access to high-quality structural data, fostering innovation across diverse scientific disciplines. However, challenges such as computational resource demands, data privacy concerns, and the need for continuous validation against experimental data must be addressed. Overall, the future of AlphaFold’s next generation promises to be transformative, with the potential to unlock new frontiers in science and medicine.
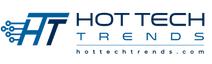