Exploring the Climate Effects of Generative AI: A Q&A Session delves into the environmental implications of generative artificial intelligence technologies. As these systems become increasingly integrated into various sectors, understanding their energy consumption, carbon footprint, and potential for sustainable innovation is crucial. This session aims to address key questions surrounding the climate impact of generative AI, offering insights from experts in the field and fostering a dialogue on how to balance technological advancement with environmental responsibility.
Understanding Generative AI’s Carbon Footprint
As the world increasingly embraces technological advancements, the environmental implications of these innovations have come under scrutiny. Generative AI, a subset of artificial intelligence that focuses on creating new content, has gained significant attention for its potential applications across various sectors. However, understanding its carbon footprint is crucial in evaluating its overall impact on the environment. The energy consumption associated with training and deploying generative AI models is a primary concern, as these processes often require substantial computational resources.
To grasp the carbon footprint of generative AI, it is essential to consider the energy sources that power the data centers housing these models. Many data centers rely on fossil fuels, which contribute to greenhouse gas emissions. Consequently, the carbon footprint of generative AI can vary significantly depending on the energy mix of the region in which the data center operates. For instance, data centers powered by renewable energy sources, such as wind or solar, tend to have a lower carbon footprint compared to those reliant on coal or natural gas. This disparity highlights the importance of transitioning to sustainable energy solutions to mitigate the environmental impact of AI technologies.
Moreover, the scale of generative AI models plays a pivotal role in determining their carbon emissions. Larger models, which often yield more sophisticated outputs, require more extensive training and, consequently, consume more energy. Research indicates that the training of a single large AI model can emit as much carbon as five cars over their lifetimes. This alarming statistic underscores the need for researchers and developers to consider the environmental costs associated with their innovations. As the demand for more advanced generative AI systems continues to grow, it becomes increasingly vital to balance performance with sustainability.
In addition to the direct energy consumption associated with training models, the operational phase of generative AI also contributes to its carbon footprint. Once deployed, these models require ongoing computational resources for inference, which can further exacerbate their environmental impact. Therefore, it is essential to adopt energy-efficient practices throughout the lifecycle of generative AI systems. Techniques such as model distillation, which involves creating smaller, more efficient versions of large models, can help reduce energy consumption while maintaining performance levels.
Furthermore, the role of software optimization cannot be overlooked in the quest to minimize the carbon footprint of generative AI. By improving algorithms and enhancing the efficiency of code, developers can significantly reduce the computational resources required for training and inference. This optimization not only leads to lower energy consumption but also promotes faster processing times, ultimately benefiting users and the environment alike.
As stakeholders in the AI community become more aware of the environmental implications of their work, there is a growing emphasis on developing sustainable practices. Initiatives aimed at promoting transparency in energy usage and carbon emissions are gaining traction, encouraging organizations to disclose their environmental impact. This shift towards accountability is crucial in fostering a culture of sustainability within the AI sector.
In conclusion, understanding the carbon footprint of generative AI is a multifaceted endeavor that requires careful consideration of energy sources, model size, operational practices, and software optimization. As the technology continues to evolve, it is imperative for researchers, developers, and organizations to prioritize sustainability in their efforts. By doing so, they can harness the transformative potential of generative AI while minimizing its environmental impact, ultimately contributing to a more sustainable future.
The Role of Data Centers in AI’s Environmental Impact
As the adoption of generative AI technologies continues to rise, it is essential to examine the environmental implications associated with their deployment, particularly the role of data centers. These facilities serve as the backbone of AI operations, housing the servers and infrastructure necessary for processing vast amounts of data. Consequently, understanding their environmental impact is crucial for assessing the overall sustainability of AI technologies.
Data centers consume significant amounts of energy, primarily due to the need for continuous operation and cooling systems that maintain optimal temperatures for servers. This energy consumption is a critical factor in evaluating the carbon footprint of generative AI. As AI models grow in complexity and size, the demand for computational power increases, leading to higher energy requirements. In fact, studies have shown that training large AI models can consume as much energy as several households would use in a year. This raises important questions about the sources of energy powering these data centers. If the energy comes from fossil fuels, the environmental impact is exacerbated, contributing to greenhouse gas emissions and climate change.
Moreover, the geographical distribution of data centers plays a significant role in their environmental impact. Many data centers are located in regions where electricity is generated from non-renewable sources, further intensifying their carbon footprint. However, there is a growing trend toward establishing data centers in areas with abundant renewable energy resources, such as wind or solar power. This shift not only mitigates the environmental impact but also aligns with global efforts to transition to sustainable energy sources. By leveraging renewable energy, data centers can significantly reduce their carbon emissions, making the operation of generative AI technologies more environmentally friendly.
In addition to energy consumption, the physical infrastructure of data centers also poses environmental challenges. The construction and maintenance of these facilities require substantial resources, including water for cooling systems and materials for building. As the demand for AI capabilities increases, so does the need for more data centers, which can lead to habitat disruption and increased resource extraction. Therefore, it is imperative to consider the lifecycle of data centers, from construction to decommissioning, in order to fully understand their environmental impact.
To address these challenges, many companies are adopting strategies aimed at improving the sustainability of their data centers. These strategies include optimizing energy efficiency through advanced cooling technologies, utilizing artificial intelligence for resource management, and implementing rigorous recycling programs for electronic waste. Furthermore, some organizations are committing to carbon neutrality by investing in carbon offset projects or purchasing renewable energy credits. Such initiatives not only help mitigate the environmental impact of data centers but also demonstrate a commitment to corporate social responsibility.
In conclusion, the role of data centers in the environmental impact of generative AI is multifaceted and requires careful consideration. As the demand for AI technologies continues to grow, it is essential to prioritize sustainable practices in the design and operation of data centers. By focusing on energy efficiency, renewable energy sources, and responsible resource management, the AI industry can work towards minimizing its ecological footprint. Ultimately, fostering a balance between technological advancement and environmental stewardship will be crucial in ensuring that generative AI contributes positively to society while safeguarding the planet for future generations.
Mitigating Climate Change Through AI Innovations
As the world grapples with the pressing challenges of climate change, innovative technologies are increasingly being recognized for their potential to mitigate environmental impacts. Among these technologies, generative artificial intelligence (AI) stands out as a powerful tool that can contribute to climate change solutions. By harnessing the capabilities of AI, researchers and organizations are exploring new avenues to reduce carbon footprints, optimize resource use, and enhance sustainability practices across various sectors.
One of the most significant ways generative AI can aid in climate change mitigation is through its ability to analyze vast amounts of data. By processing complex datasets related to energy consumption, emissions, and environmental conditions, AI can identify patterns and trends that may not be immediately apparent to human analysts. This data-driven approach enables more informed decision-making, allowing businesses and governments to implement strategies that effectively reduce greenhouse gas emissions. For instance, AI algorithms can optimize energy usage in buildings by predicting occupancy patterns and adjusting heating, ventilation, and air conditioning systems accordingly, thereby minimizing energy waste.
Moreover, generative AI can play a crucial role in advancing renewable energy technologies. By simulating various scenarios and outcomes, AI can assist in the design and optimization of solar panels, wind turbines, and other renewable energy systems. This capability not only enhances the efficiency of these technologies but also accelerates their deployment, making clean energy sources more accessible and cost-effective. As a result, the transition from fossil fuels to renewable energy can be expedited, significantly contributing to global efforts to combat climate change.
In addition to optimizing energy systems, generative AI can also facilitate sustainable agricultural practices. By analyzing data related to soil health, weather patterns, and crop yields, AI can provide farmers with actionable insights that promote efficient resource use and reduce environmental impacts. For example, AI-driven precision agriculture techniques can help farmers determine the optimal amount of water, fertilizers, and pesticides needed for their crops, thereby minimizing waste and preventing runoff that can harm local ecosystems. This not only enhances food security but also supports the broader goal of sustainable land management.
Furthermore, generative AI can assist in climate modeling and forecasting, providing valuable insights into future climate scenarios. By simulating the effects of various climate policies and interventions, AI can help policymakers understand the potential outcomes of their decisions. This predictive capability is essential for developing effective strategies to mitigate climate change and adapt to its impacts. As governments and organizations strive to meet ambitious climate targets, the ability to model and anticipate the consequences of different actions becomes increasingly critical.
However, while the potential of generative AI in mitigating climate change is significant, it is essential to approach its implementation thoughtfully. The energy consumption associated with training and deploying AI models must be considered, as it can contribute to the very problem it seeks to solve. Therefore, researchers and practitioners are exploring ways to make AI systems more energy-efficient and sustainable. This includes developing algorithms that require less computational power and utilizing renewable energy sources to power data centers.
In conclusion, generative AI holds immense promise for mitigating climate change through its ability to analyze data, optimize systems, and inform decision-making across various sectors. By leveraging these innovations, society can make significant strides toward a more sustainable future. However, it is crucial to balance the benefits of AI with its environmental impact, ensuring that the technology serves as a genuine ally in the fight against climate change. As we continue to explore the intersection of AI and environmental sustainability, the potential for transformative change remains within reach.
Comparing Traditional Computing and Generative AI Emissions
As the world increasingly turns to advanced technologies, the environmental implications of these innovations have come under scrutiny. In particular, the rise of generative artificial intelligence (AI) has prompted discussions about its energy consumption and carbon emissions compared to traditional computing methods. To understand the climate effects of generative AI, it is essential to first examine the fundamental differences in how these systems operate and the resources they require.
Traditional computing, which encompasses a wide range of applications from basic data processing to complex simulations, typically relies on established algorithms that have been optimized over decades. These systems often operate on fixed datasets and perform specific tasks with relatively predictable energy consumption patterns. In contrast, generative AI models, such as those used for creating text, images, or music, require extensive training on vast datasets. This training process involves running complex algorithms on powerful hardware, often utilizing graphics processing units (GPUs) or tensor processing units (TPUs) that consume significant amounts of electricity.
The energy demands of generative AI are particularly pronounced during the training phase, where models learn from millions of examples. This phase can last for days or even weeks, depending on the complexity of the model and the size of the dataset. Consequently, the carbon footprint associated with this training can be substantial, especially if the energy sources powering the data centers are not renewable. In contrast, once a generative AI model is trained, its operational phase—where it generates outputs based on the learned patterns—tends to be less energy-intensive. However, the initial emissions from training can overshadow the operational emissions, raising concerns about the overall sustainability of these technologies.
Moreover, the comparison between traditional computing and generative AI emissions is not straightforward. Traditional computing systems can also be energy-intensive, particularly when they are scaled up for large-scale data processing or cloud computing services. However, the efficiency of these systems has improved over time, with advancements in hardware and software leading to reduced energy consumption per task. In contrast, generative AI is still in a relatively nascent stage, and while there are ongoing efforts to enhance its efficiency, the rapid pace of development often outstrips these improvements.
Transitioning to a more sustainable future requires a nuanced understanding of these technologies’ environmental impacts. Researchers and developers are increasingly aware of the need to mitigate the carbon footprint of generative AI. This awareness has led to initiatives aimed at optimizing algorithms, improving hardware efficiency, and utilizing renewable energy sources for data centers. For instance, some organizations are exploring techniques such as model distillation, which reduces the size of AI models without significantly compromising their performance, thereby lowering energy requirements.
In conclusion, while generative AI presents exciting opportunities for innovation, it is crucial to consider its environmental implications in comparison to traditional computing. The energy consumption and emissions associated with training generative AI models can be significant, particularly if powered by non-renewable energy sources. However, as the field evolves, there is potential for advancements that could enhance efficiency and sustainability. By fostering a dialogue around these issues, stakeholders can work towards solutions that balance technological progress with environmental responsibility, ensuring that the benefits of generative AI do not come at the expense of our planet’s health.
Policy Implications for Sustainable AI Development
As the capabilities of generative artificial intelligence (AI) continue to expand, so too do the implications for environmental sustainability. The intersection of AI technology and climate change presents a complex landscape that necessitates careful consideration of policy frameworks aimed at promoting sustainable development. In this context, it is essential to explore the policy implications that arise from the increasing deployment of generative AI systems, particularly in relation to their energy consumption and carbon footprint.
To begin with, it is crucial to recognize that the training and operation of generative AI models often require substantial computational resources, which in turn demand significant energy inputs. This energy consumption can lead to increased greenhouse gas emissions, particularly if the energy sources are not renewable. Therefore, policymakers must prioritize the establishment of guidelines that encourage the use of renewable energy in data centers and AI operations. By incentivizing the transition to green energy sources, governments can help mitigate the environmental impact of AI technologies while simultaneously fostering innovation in the renewable energy sector.
Moreover, the development of generative AI should be accompanied by robust regulatory frameworks that promote transparency and accountability. Policymakers can implement measures that require organizations to disclose the energy consumption and carbon emissions associated with their AI systems. Such transparency not only empowers consumers to make informed choices but also encourages companies to adopt more sustainable practices. By holding organizations accountable for their environmental impact, policymakers can drive a culture of sustainability within the tech industry.
In addition to transparency, there is a pressing need for research and development initiatives focused on improving the energy efficiency of AI models. Policymakers can play a pivotal role by allocating funding and resources to support academic and industry research aimed at creating more efficient algorithms and hardware. By fostering collaboration between researchers, technologists, and environmental scientists, governments can facilitate the development of innovative solutions that reduce the energy demands of generative AI systems.
Furthermore, it is essential to consider the broader implications of generative AI on various sectors, including manufacturing, transportation, and agriculture. As these industries increasingly adopt AI technologies, policymakers must ensure that the integration of AI aligns with sustainability goals. This alignment can be achieved through the establishment of industry-specific guidelines that promote the responsible use of AI while minimizing environmental harm. For instance, in agriculture, AI can optimize resource use and reduce waste, but it is vital that such applications are developed with sustainability in mind.
Additionally, international cooperation is paramount in addressing the global challenges posed by generative AI and climate change. Policymakers should engage in dialogue with international counterparts to share best practices and develop harmonized standards for sustainable AI development. By fostering a collaborative approach, countries can collectively address the environmental impacts of AI technologies and work towards a more sustainable future.
In conclusion, the policy implications for sustainable AI development are multifaceted and require a comprehensive approach. By prioritizing renewable energy, promoting transparency, investing in research, and fostering international cooperation, policymakers can guide the development of generative AI in a manner that aligns with environmental sustainability goals. As the technology continues to evolve, it is imperative that the policies governing its use evolve as well, ensuring that the benefits of generative AI do not come at the expense of our planet’s health. Through thoughtful and proactive policymaking, we can harness the potential of AI while safeguarding the environment for future generations.
Future Trends: Greener Generative AI Solutions
As the world increasingly turns to generative artificial intelligence (AI) for various applications, from content creation to complex problem-solving, it is essential to consider the environmental implications of these technologies. The energy consumption associated with training and deploying generative AI models has raised concerns about their carbon footprint. However, as the field evolves, there is a growing focus on developing greener generative AI solutions that aim to mitigate these environmental impacts. This shift is not only necessary for sustainability but also presents opportunities for innovation and efficiency.
One of the most promising trends in the pursuit of greener generative AI is the optimization of algorithms. Researchers are actively exploring ways to enhance the efficiency of AI models, which can significantly reduce the computational resources required for training. By refining algorithms to require less data and fewer processing cycles, developers can decrease energy consumption without sacrificing performance. This optimization process often involves leveraging techniques such as model pruning, quantization, and knowledge distillation, which streamline models while maintaining their effectiveness.
In addition to algorithmic improvements, the use of more efficient hardware is another avenue being explored. The development of specialized chips designed for AI tasks, such as tensor processing units (TPUs) and field-programmable gate arrays (FPGAs), can lead to substantial energy savings. These hardware solutions are tailored to perform specific computations more efficiently than general-purpose processors, thereby reducing the overall energy footprint of generative AI applications. As manufacturers continue to innovate in this space, the potential for greener AI solutions becomes increasingly viable.
Moreover, the integration of renewable energy sources into data centers that host AI models is a critical factor in reducing the carbon emissions associated with generative AI. Many tech companies are committing to powering their operations with renewable energy, such as solar and wind power. By transitioning to these sustainable energy sources, organizations can significantly lower the environmental impact of their AI initiatives. This commitment not only aligns with global sustainability goals but also enhances the public perception of companies that prioritize eco-friendly practices.
Another trend gaining traction is the development of AI models that are inherently more efficient in their design. Researchers are investigating architectures that require fewer parameters and less training data, which can lead to lower energy consumption. For instance, advancements in few-shot and zero-shot learning allow models to generalize from limited examples, reducing the need for extensive datasets and the associated computational costs. This approach not only conserves resources but also accelerates the deployment of AI solutions across various sectors.
Furthermore, collaboration among stakeholders in the AI ecosystem is essential for fostering greener practices. By sharing best practices, research findings, and technological advancements, organizations can collectively work towards minimizing the environmental impact of generative AI. Initiatives that promote transparency in energy usage and carbon emissions can also encourage accountability and drive progress in the development of sustainable AI solutions.
In conclusion, the future of generative AI holds significant promise for greener solutions that address the pressing environmental challenges associated with these technologies. Through algorithmic optimization, efficient hardware utilization, renewable energy integration, and innovative model design, the AI community is poised to make substantial strides in reducing its carbon footprint. As these trends continue to evolve, they will not only contribute to a more sustainable future but also enhance the overall effectiveness and accessibility of generative AI across various industries. The commitment to greener practices will ultimately shape the trajectory of AI development, ensuring that technological advancements align with the imperative of environmental stewardship.
Q&A
1. **Q: What are the primary climate effects associated with generative AI?**
A: The primary climate effects include increased energy consumption, carbon emissions from data centers, and resource use for hardware production.
2. **Q: How does the energy consumption of generative AI compare to traditional computing methods?**
A: Generative AI typically requires significantly more computational power, leading to higher energy consumption compared to traditional computing methods.
3. **Q: What measures can be taken to mitigate the climate impact of generative AI?**
A: Measures include optimizing algorithms for efficiency, using renewable energy sources for data centers, and improving hardware efficiency.
4. **Q: Are there any specific studies that quantify the climate impact of generative AI?**
A: Yes, several studies have analyzed the carbon footprint of training large AI models, highlighting their substantial energy demands and associated emissions.
5. **Q: How does the lifecycle of AI hardware contribute to climate effects?**
A: The lifecycle of AI hardware involves resource extraction, manufacturing, and disposal, all of which contribute to environmental degradation and carbon emissions.
6. **Q: What role can policymakers play in addressing the climate effects of generative AI?**
A: Policymakers can implement regulations promoting energy efficiency, incentivize the use of renewable energy, and support research into sustainable AI technologies.The exploration of the climate effects of generative AI reveals a complex interplay between technological advancement and environmental impact. While generative AI offers significant benefits in various sectors, its energy consumption and carbon footprint raise important concerns. Addressing these challenges requires a collaborative effort among researchers, policymakers, and industry leaders to develop sustainable practices and technologies that mitigate environmental harm while harnessing the potential of AI. Ultimately, a balanced approach is essential to ensure that the growth of generative AI contributes positively to both innovation and climate goals.
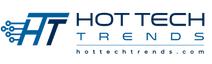