“Enhancing Language Models: A Dialogue with AI” explores the transformative advancements in artificial intelligence, focusing on the development and refinement of language models. This work delves into the intricate processes that enable machines to understand, generate, and interact using human language with increasing sophistication. By examining the dialogue between AI researchers and the technology itself, the text highlights the collaborative efforts to push the boundaries of what language models can achieve. It addresses the challenges and breakthroughs in natural language processing, offering insights into how these models are trained, fine-tuned, and applied across various domains. Through this exploration, the narrative underscores the potential of AI to revolutionize communication, enhance human-computer interaction, and contribute to a wide array of fields, from customer service to creative writing.
Understanding the Basics of Language Models
Language models have become a cornerstone of modern artificial intelligence, playing a pivotal role in various applications ranging from chatbots to translation services. At their core, these models are designed to understand and generate human language, making them indispensable tools in the digital age. To comprehend the intricacies of language models, it is essential to explore their foundational principles and the mechanisms that enable them to process and produce language with remarkable accuracy.
Initially, language models were relatively simple, relying on basic statistical methods to predict the likelihood of a word following a given sequence. These early models, known as n-grams, were limited by their inability to capture long-range dependencies in text. However, the advent of deep learning revolutionized the field, introducing neural networks capable of processing vast amounts of data and learning complex patterns. This shift marked a significant leap forward, allowing language models to understand context and nuance in ways previously unattainable.
Central to the development of advanced language models is the concept of representation learning. By transforming words into vectors through techniques such as word embeddings, models can capture semantic relationships between words. This transformation enables the model to understand that “king” and “queen” are related in a similar way to “man” and “woman.” Such insights are crucial for tasks like sentiment analysis, where understanding the sentiment behind words is more important than the words themselves.
Moreover, the introduction of transformer architectures has further enhanced the capabilities of language models. Transformers, with their attention mechanisms, allow models to weigh the importance of different words in a sentence, thereby improving their ability to understand context. This innovation has led to the creation of models like BERT and GPT, which have set new benchmarks in natural language processing tasks. These models are pre-trained on vast corpora of text, enabling them to generate coherent and contextually relevant responses.
Despite these advancements, challenges remain in the quest to perfect language models. One significant issue is the tendency of models to produce biased or inappropriate content, reflecting the biases present in the training data. Addressing this requires ongoing efforts to curate diverse and representative datasets, as well as developing techniques to mitigate bias during the training process. Additionally, the computational resources required to train large models pose environmental and economic concerns, prompting researchers to explore more efficient training methods.
As we continue to refine language models, the dialogue between humans and AI becomes increasingly sophisticated. These models are not only tools for communication but also partners in creativity and problem-solving. They assist in drafting articles, composing music, and even generating code, showcasing their versatility and potential. However, it is crucial to maintain a balance between leveraging their capabilities and ensuring ethical and responsible use.
In conclusion, understanding the basics of language models involves appreciating the evolution from simple statistical methods to complex neural networks. The advancements in representation learning and transformer architectures have significantly enhanced their performance, yet challenges such as bias and resource consumption persist. As we navigate these complexities, the dialogue with AI continues to evolve, promising a future where language models are integral to our interaction with technology. Through ongoing research and innovation, we can harness their potential while addressing the ethical considerations that accompany their use.
The Role of Data in Enhancing AI Dialogue
In the rapidly evolving field of artificial intelligence, the enhancement of language models has become a focal point for researchers and developers alike. Central to this enhancement is the role of data, which serves as the foundational element in training and refining these models. As we delve into the intricacies of AI dialogue, it becomes evident that the quality, diversity, and volume of data significantly influence the performance and capabilities of language models.
To begin with, the quality of data is paramount in shaping the effectiveness of AI dialogue systems. High-quality data ensures that language models can generate coherent, contextually relevant, and accurate responses. This is achieved by curating datasets that are free from errors, biases, and inconsistencies. By doing so, developers can mitigate the risk of propagating misinformation or perpetuating stereotypes through AI-generated content. Furthermore, high-quality data allows language models to better understand nuances in human language, such as idiomatic expressions, cultural references, and emotional undertones, thereby enhancing their ability to engage in meaningful and human-like conversations.
In addition to quality, the diversity of data plays a crucial role in enhancing AI dialogue. Language models trained on diverse datasets are more adept at handling a wide range of topics, dialects, and linguistic styles. This diversity enables AI systems to cater to a global audience, accommodating variations in language use across different regions and cultures. Moreover, diverse data exposure helps language models to generalize better, allowing them to respond appropriately to novel or unexpected inputs. This adaptability is essential for creating AI systems that can seamlessly integrate into various applications, from customer service chatbots to virtual personal assistants.
The volume of data is another critical factor in the development of robust language models. Large datasets provide the extensive exposure needed for AI systems to learn complex language patterns and relationships. With more data, language models can improve their predictive accuracy and fluency, resulting in more natural and engaging dialogues. However, it is important to balance the volume of data with computational resources and ethical considerations. While larger datasets can enhance model performance, they also require significant processing power and storage capacity. Additionally, the collection and use of vast amounts of data raise privacy and security concerns that must be addressed to maintain public trust in AI technologies.
Transitioning from the role of data to the practical implications of enhanced AI dialogue, it is clear that advancements in language models have far-reaching effects across various sectors. In customer service, for instance, improved AI dialogue systems can lead to more efficient and satisfactory interactions, reducing wait times and enhancing user experience. In education, AI-driven tutoring systems can provide personalized learning experiences, adapting to individual student needs and fostering better educational outcomes. Moreover, in healthcare, AI dialogue systems can assist in patient triage and support, offering timely and accurate information to both patients and healthcare providers.
In conclusion, the role of data in enhancing AI dialogue is multifaceted, encompassing quality, diversity, and volume. As language models continue to evolve, the careful curation and utilization of data will remain a cornerstone of their development. By prioritizing these aspects, we can ensure that AI dialogue systems become more effective, inclusive, and beneficial to society as a whole. As we move forward, ongoing research and collaboration will be essential in addressing the challenges and opportunities presented by this dynamic field.
Techniques for Improving AI Conversational Skills
In recent years, the development of artificial intelligence has seen remarkable advancements, particularly in the realm of language models. These models, which are designed to understand and generate human-like text, have become increasingly sophisticated, enabling them to engage in more natural and meaningful conversations. However, the quest to enhance AI conversational skills is ongoing, as researchers and developers strive to create systems that can seamlessly interact with humans. To achieve this, several techniques have been employed, each contributing to the refinement of AI dialogue capabilities.
One of the primary techniques used to improve AI conversational skills is the incorporation of large-scale datasets. By training language models on vast amounts of text data, these systems can learn the nuances of human language, including grammar, syntax, and context. This extensive training allows AI to generate responses that are not only coherent but also contextually relevant. Moreover, the diversity of the datasets ensures that the AI can handle a wide range of topics, making it more versatile in conversation.
In addition to large-scale datasets, fine-tuning is another critical technique in enhancing AI dialogue. Fine-tuning involves taking a pre-trained language model and further training it on a specific dataset that is relevant to the desired application. This process allows the model to adapt to particular conversational contexts, such as customer service or technical support, thereby improving its ability to provide accurate and helpful responses. Fine-tuning ensures that the AI can cater to specific needs while maintaining the general language understanding acquired during initial training.
Furthermore, reinforcement learning has emerged as a powerful tool in refining AI conversational skills. This technique involves training the model through a system of rewards and penalties, encouraging it to generate responses that are more aligned with human preferences. By simulating interactions and providing feedback, reinforcement learning helps the AI to learn from its mistakes and improve over time. This iterative process is crucial in developing models that can engage in more natural and satisfying dialogues with users.
Another innovative approach to enhancing AI conversational skills is the integration of emotional intelligence. By incorporating sentiment analysis and emotion recognition, AI systems can better understand the emotional tone of a conversation and respond appropriately. This capability is particularly important in applications where empathy and understanding are essential, such as mental health support or customer service. By recognizing and adapting to the emotional state of the user, AI can provide more personalized and effective interactions.
Moreover, the use of multi-modal inputs is gaining traction as a means to improve AI dialogue. By combining text with other forms of data, such as images or audio, AI systems can gain a more comprehensive understanding of the context. This multi-modal approach allows the AI to interpret and respond to cues that are not solely text-based, thereby enhancing its conversational abilities. For instance, in a video call, the AI could analyze facial expressions and tone of voice to better gauge the user’s intent and emotions.
In conclusion, the enhancement of AI conversational skills is a multifaceted endeavor that involves a combination of techniques. From leveraging large-scale datasets and fine-tuning to employing reinforcement learning and integrating emotional intelligence, each method plays a vital role in advancing the capabilities of language models. As these techniques continue to evolve, the potential for AI to engage in more meaningful and human-like conversations becomes increasingly attainable, paving the way for more sophisticated and effective interactions between humans and machines.
Ethical Considerations in AI Language Development
In recent years, the development of artificial intelligence, particularly in the realm of language models, has seen remarkable advancements. These models, capable of generating human-like text, have found applications in various fields, from customer service to creative writing. However, as these technologies become more sophisticated, ethical considerations in their development and deployment have come to the forefront. It is crucial to address these concerns to ensure that AI language models are used responsibly and do not inadvertently cause harm.
One of the primary ethical concerns is the potential for bias in AI language models. These models are trained on vast datasets that often contain biases present in human language. Consequently, if not carefully managed, AI can perpetuate or even amplify these biases, leading to unfair or discriminatory outcomes. For instance, biased language models might generate content that reflects gender, racial, or cultural stereotypes. To mitigate this risk, developers must implement strategies to identify and reduce bias in training data and model outputs. This involves not only refining algorithms but also fostering diverse teams that can provide a broader perspective on potential biases.
Moreover, the issue of misinformation is another significant ethical challenge. AI language models can generate text that appears credible but is factually incorrect or misleading. This capability raises concerns about the spread of misinformation, particularly in sensitive areas such as health, politics, and finance. To address this, developers are exploring ways to incorporate fact-checking mechanisms and ensure that AI-generated content is aligned with verified information. Additionally, transparency in AI operations is essential, allowing users to understand the limitations and potential inaccuracies of these models.
Privacy is another critical consideration in the development of AI language models. These models often require large amounts of data to function effectively, raising concerns about the collection and use of personal information. Ensuring that data is anonymized and used ethically is paramount to maintaining user trust. Furthermore, developers must comply with data protection regulations, such as the General Data Protection Regulation (GDPR), to safeguard user privacy. By prioritizing privacy, developers can create AI systems that respect individual rights while still delivering powerful language capabilities.
The potential for misuse of AI language models also warrants attention. These models can be used to generate harmful content, such as hate speech or propaganda, which can have real-world consequences. To prevent such misuse, developers are implementing safeguards and monitoring systems to detect and mitigate harmful outputs. Additionally, fostering a culture of responsibility among users and developers is crucial, emphasizing the importance of ethical AI use.
Finally, the ethical development of AI language models requires ongoing dialogue between stakeholders, including developers, ethicists, policymakers, and the public. This collaborative approach ensures that diverse perspectives are considered and that AI technologies are developed in a manner that aligns with societal values. By engaging in open discussions, stakeholders can address emerging ethical challenges and work towards solutions that balance innovation with responsibility.
In conclusion, as AI language models continue to evolve, addressing ethical considerations is essential to their responsible development and deployment. By tackling issues such as bias, misinformation, privacy, and misuse, developers can create AI systems that not only enhance human capabilities but also uphold ethical standards. Through collaboration and transparency, the AI community can ensure that these powerful tools are used for the greater good, fostering trust and confidence in the technology.
The Future of AI-Driven Communication
In recent years, the rapid advancement of artificial intelligence has significantly transformed the landscape of communication. Among the most notable developments is the evolution of language models, which have become increasingly sophisticated in their ability to understand and generate human-like text. As we look to the future of AI-driven communication, it is essential to explore how these models can be further enhanced to improve their efficacy and reliability. This dialogue with AI not only promises to revolutionize the way we interact with technology but also poses intriguing questions about the nature of communication itself.
To begin with, the current generation of language models, such as OpenAI’s GPT series, has demonstrated remarkable capabilities in generating coherent and contextually relevant text. These models are trained on vast datasets, enabling them to capture the nuances of human language and produce responses that are often indistinguishable from those written by humans. However, despite these impressive achievements, there are still areas where language models can be improved. For instance, they sometimes struggle with understanding context over extended conversations, leading to responses that may be accurate in isolation but lack coherence when viewed as part of a larger dialogue.
Addressing these challenges requires a multifaceted approach. One potential avenue for enhancement is the integration of more sophisticated context-awareness mechanisms. By incorporating memory networks or similar architectures, language models could better retain and utilize information from previous interactions, thereby improving their ability to maintain context over longer exchanges. Additionally, advancements in natural language understanding could enable these models to grasp subtler aspects of human communication, such as tone, intent, and emotion, further enhancing their ability to engage in meaningful dialogue.
Moreover, the ethical implications of AI-driven communication cannot be overlooked. As language models become more adept at mimicking human conversation, the potential for misuse increases. Ensuring that these technologies are developed and deployed responsibly is paramount. This involves implementing robust safeguards to prevent the generation of harmful or misleading content and establishing clear guidelines for their ethical use. Furthermore, transparency in how these models operate and make decisions is crucial to building trust with users and ensuring accountability.
In addition to ethical considerations, the future of AI-driven communication also hinges on the ability to personalize interactions. As language models become more integrated into our daily lives, the demand for personalized experiences will grow. By leveraging user data, AI systems can tailor their responses to better align with individual preferences and needs. However, this personalization must be balanced with privacy concerns, necessitating the development of secure methods for data handling and storage.
Looking ahead, the potential applications of enhanced language models are vast and varied. From customer service and education to healthcare and entertainment, AI-driven communication has the power to transform numerous industries. By continuing to refine these models and address the challenges they present, we can unlock new possibilities for human-AI interaction, ultimately leading to more efficient, effective, and engaging communication.
In conclusion, the future of AI-driven communication is both promising and complex. As we engage in this ongoing dialogue with AI, it is crucial to focus on enhancing language models to better understand and respond to human needs while addressing ethical and privacy concerns. By doing so, we can harness the full potential of these technologies, paving the way for a new era of communication that is both innovative and responsible.
Case Studies: Successful Enhancements in AI Language Models
In recent years, the field of artificial intelligence has witnessed remarkable advancements, particularly in the development and enhancement of language models. These models, which are designed to understand and generate human language, have become increasingly sophisticated, enabling a wide range of applications from chatbots to content creation. A closer examination of successful enhancements in AI language models reveals the intricate processes and innovative strategies that have contributed to their evolution.
One of the most significant case studies in this domain is the development of OpenAI’s GPT series. The progression from GPT-2 to GPT-3 marked a substantial leap in the model’s capabilities, primarily due to the increase in parameters and the volume of data used for training. This expansion allowed the model to generate more coherent and contextually relevant text, thereby improving its utility across various applications. The transition from GPT-2 to GPT-3 exemplifies how scaling up model size and training data can lead to significant performance improvements, a strategy that has been emulated by other organizations seeking to enhance their language models.
In addition to scaling, another successful enhancement strategy involves fine-tuning models for specific tasks. Fine-tuning allows a general-purpose language model to be adapted to particular domains or applications, thereby increasing its effectiveness and accuracy. For instance, models fine-tuned for medical or legal contexts can provide more precise and relevant information, as they are trained on specialized datasets that reflect the nuances of these fields. This approach not only enhances the model’s performance but also broadens its applicability, making it a valuable tool for industry-specific tasks.
Moreover, the integration of reinforcement learning techniques has also played a pivotal role in enhancing language models. By incorporating feedback mechanisms, models can learn from their interactions and improve over time. This iterative process enables the model to refine its responses based on user input, leading to more natural and engaging dialogues. Reinforcement learning thus represents a dynamic enhancement strategy that aligns with the evolving nature of human communication, allowing AI to better mimic conversational patterns.
Furthermore, the development of multilingual models has been a noteworthy advancement in the field. By training models on diverse linguistic datasets, researchers have created systems capable of understanding and generating text in multiple languages. This capability not only enhances the accessibility of AI technologies but also facilitates cross-cultural communication and collaboration. The success of multilingual models underscores the importance of inclusivity and diversity in AI development, as it broadens the scope of potential applications and user engagement.
In addition to these technical enhancements, ethical considerations have also been at the forefront of language model development. Ensuring that models are free from biases and can generate content responsibly is crucial for their successful deployment. Researchers have implemented various strategies to mitigate biases, such as curating balanced training datasets and employing fairness algorithms. These efforts highlight the importance of ethical AI practices in the enhancement process, as they ensure that language models are not only effective but also equitable and trustworthy.
In conclusion, the successful enhancements in AI language models are the result of a combination of scaling, fine-tuning, reinforcement learning, multilingual capabilities, and ethical considerations. These strategies have collectively contributed to the development of more sophisticated and versatile language models, paving the way for their widespread adoption across diverse sectors. As AI continues to evolve, ongoing dialogue and collaboration between researchers, developers, and users will be essential in driving further advancements and ensuring that language models remain a powerful tool for innovation and communication.
Q&A
1. **What is the primary goal of enhancing language models?**
The primary goal is to improve their ability to understand and generate human-like text, making interactions more natural and contextually relevant.
2. **How can language models be improved for better dialogue?**
They can be improved through techniques like fine-tuning on specific datasets, incorporating user feedback, and using advanced architectures like transformers.
3. **What role does user feedback play in enhancing language models?**
User feedback helps identify areas where the model may be lacking, allowing developers to make targeted improvements and refine the model’s responses.
4. **What are some challenges in enhancing language models for dialogue?**
Challenges include handling ambiguous queries, maintaining context over long conversations, and ensuring responses are both accurate and appropriate.
5. **How does context retention improve dialogue in language models?**
Context retention allows the model to remember previous interactions, leading to more coherent and relevant responses in ongoing conversations.
6. **What ethical considerations are involved in enhancing language models?**
Ethical considerations include ensuring privacy, avoiding bias, and preventing the generation of harmful or misleading content.Enhancing language models involves improving their ability to understand and generate human-like text, which is crucial for effective AI-human interaction. This process includes refining algorithms, increasing data diversity, and incorporating ethical considerations to ensure responsible AI deployment. By advancing these models, we can achieve more accurate, context-aware, and empathetic dialogues, ultimately leading to more meaningful and productive interactions between humans and AI systems.
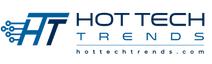