At NeurIPS 2022, DeepMind unveiled groundbreaking findings that further cement its position at the forefront of artificial intelligence research. The conference, renowned for showcasing cutting-edge advancements in machine learning and computational neuroscience, provided the perfect platform for DeepMind to present its latest innovations. These findings highlight significant strides in areas such as reinforcement learning, neural network architectures, and AI interpretability. By pushing the boundaries of what is possible with AI, DeepMind’s research not only advances theoretical understanding but also paves the way for practical applications that could transform industries and improve everyday life.
Advancements In Reinforcement Learning Techniques
At the NeurIPS 2022 conference, DeepMind unveiled groundbreaking advancements in reinforcement learning techniques, marking a significant milestone in the field of artificial intelligence. These developments promise to enhance the efficiency and applicability of AI systems across various domains. Reinforcement learning, a subset of machine learning, involves training algorithms to make decisions by rewarding desired behaviors and penalizing undesired ones. DeepMind’s latest findings focus on improving the adaptability and generalization of these algorithms, which are crucial for their deployment in real-world scenarios.
One of the key innovations presented by DeepMind is the introduction of a novel algorithm that significantly reduces the amount of data required for training. Traditionally, reinforcement learning algorithms demand vast amounts of data to achieve optimal performance, which can be a limiting factor in environments where data collection is costly or impractical. DeepMind’s new approach leverages advanced techniques in transfer learning, allowing the algorithm to apply knowledge gained from previous tasks to new, related tasks. This not only accelerates the learning process but also enhances the algorithm’s ability to generalize across different environments.
Moreover, DeepMind has made strides in improving the stability and robustness of reinforcement learning models. A common challenge in the field is the tendency of models to overfit to specific training environments, leading to suboptimal performance when faced with novel situations. To address this, DeepMind has developed a method that incorporates elements of meta-learning, enabling models to adapt more effectively to changes and uncertainties in their environment. This advancement is particularly relevant for applications such as autonomous driving and robotics, where adaptability is paramount.
In addition to these technical improvements, DeepMind’s research also explores the ethical implications of deploying reinforcement learning systems. As AI becomes increasingly integrated into society, ensuring that these systems operate fairly and transparently is of utmost importance. DeepMind has proposed a framework for evaluating the ethical considerations of reinforcement learning applications, emphasizing the need for accountability and bias mitigation. This framework aims to guide researchers and practitioners in developing AI systems that align with societal values and ethical standards.
Furthermore, DeepMind’s findings highlight the potential for reinforcement learning to revolutionize industries beyond traditional tech sectors. For instance, in healthcare, reinforcement learning algorithms can optimize treatment plans by continuously learning from patient data, leading to more personalized and effective care. Similarly, in finance, these algorithms can enhance decision-making processes by analyzing complex market dynamics and adapting strategies in real-time. The versatility of reinforcement learning techniques underscores their potential to drive innovation across a wide range of fields.
In conclusion, DeepMind’s newest findings presented at NeurIPS 2022 represent a significant leap forward in reinforcement learning techniques. By addressing key challenges such as data efficiency, model robustness, and ethical considerations, DeepMind is paving the way for more adaptable and responsible AI systems. As these advancements continue to evolve, they hold the promise of transforming industries and improving the quality of life across the globe. The ongoing research and development in this area will undoubtedly shape the future of artificial intelligence, offering exciting possibilities for both researchers and practitioners alike.
Breakthroughs In Natural Language Processing
At the NeurIPS 2022 conference, DeepMind unveiled groundbreaking advancements in the field of natural language processing (NLP), marking a significant leap forward in the capabilities of artificial intelligence. These findings have the potential to revolutionize how machines understand and generate human language, offering profound implications for various applications ranging from automated customer service to advanced research tools. DeepMind’s latest research focuses on enhancing the comprehension and contextual understanding of language models, addressing some of the most persistent challenges in NLP.
One of the key breakthroughs presented by DeepMind is the development of a novel architecture that significantly improves the ability of language models to grasp context and nuance. Traditional models often struggle with understanding the subtleties of human language, such as idiomatic expressions or context-dependent meanings. However, DeepMind’s new approach incorporates advanced techniques that allow models to better capture these complexities. By leveraging a more sophisticated understanding of context, these models can generate responses that are not only more accurate but also more aligned with human-like reasoning.
Moreover, DeepMind’s research introduces innovative methods for training language models more efficiently. The team has developed a new training paradigm that reduces the computational resources required while maintaining, or even enhancing, the performance of the models. This advancement is particularly significant given the growing concerns about the environmental impact of large-scale AI training processes. By optimizing the training efficiency, DeepMind’s approach not only makes NLP technologies more sustainable but also more accessible to a broader range of researchers and developers.
In addition to these technical advancements, DeepMind’s findings also explore the ethical dimensions of NLP. The team has placed a strong emphasis on ensuring that their models are designed with fairness and inclusivity in mind. This involves implementing mechanisms to mitigate biases that can arise in language models, which often reflect the prejudices present in the data they are trained on. By addressing these ethical considerations, DeepMind aims to create NLP systems that are not only powerful but also equitable and trustworthy.
Furthermore, the implications of DeepMind’s research extend beyond the realm of technology into practical applications that can benefit society at large. For instance, improved language models can enhance the quality of machine translation services, making cross-cultural communication more seamless and accessible. Additionally, these advancements can be applied to educational tools, providing personalized learning experiences that adapt to the unique needs of each student. In the healthcare sector, more sophisticated NLP systems can assist in analyzing medical literature, aiding professionals in staying abreast of the latest research and improving patient outcomes.
As DeepMind continues to push the boundaries of what is possible in natural language processing, the potential for transformative change becomes increasingly apparent. The findings presented at NeurIPS 2022 not only highlight the remarkable progress made in understanding and generating human language but also underscore the importance of responsible AI development. By prioritizing efficiency, ethical considerations, and practical applications, DeepMind is setting a new standard for the future of NLP. As these technologies continue to evolve, they promise to unlock new possibilities for innovation and collaboration across diverse fields, ultimately enhancing the way we interact with and understand the world around us.
Innovations In AI Safety And Ethics
At the NeurIPS 2022 conference, DeepMind unveiled groundbreaking findings that have significant implications for the fields of artificial intelligence safety and ethics. As AI systems become increasingly integrated into various aspects of society, ensuring their safe and ethical deployment has become a paramount concern. DeepMind’s latest research addresses these challenges by proposing innovative frameworks and methodologies that aim to enhance the reliability and accountability of AI technologies.
One of the key highlights of DeepMind’s presentation was the introduction of a novel approach to AI alignment, which seeks to ensure that AI systems act in accordance with human values and intentions. This approach involves the development of advanced algorithms that can interpret and align with complex human preferences, thereby reducing the risk of unintended consequences. By leveraging techniques from reinforcement learning and inverse reward design, DeepMind’s researchers have made significant strides in creating AI systems that can better understand and adhere to ethical guidelines.
In addition to alignment, DeepMind’s findings also emphasize the importance of transparency in AI systems. Transparency is crucial for building trust between AI technologies and their users, as it allows for greater scrutiny and understanding of how these systems operate. DeepMind has proposed a set of transparency tools that enable users to visualize and interpret the decision-making processes of AI models. These tools are designed to provide insights into the inner workings of AI systems, thereby facilitating more informed decision-making and fostering a culture of accountability.
Moreover, DeepMind’s research addresses the challenge of robustness in AI systems. Robustness refers to the ability of AI models to perform reliably under a wide range of conditions, including those that were not anticipated during the training phase. To enhance robustness, DeepMind has developed techniques that enable AI systems to adapt to novel situations and recover from errors. This is achieved through the integration of uncertainty estimation methods, which allow AI models to assess the reliability of their predictions and adjust their behavior accordingly.
Furthermore, DeepMind’s findings highlight the significance of fairness in AI systems. As AI technologies are increasingly used in decision-making processes that impact individuals and communities, ensuring fairness is essential to prevent discrimination and bias. DeepMind has introduced a framework for evaluating and mitigating bias in AI models, which involves the use of fairness metrics and bias detection algorithms. This framework is designed to identify and address potential sources of bias, thereby promoting equitable outcomes and fostering social justice.
In conclusion, DeepMind’s newest findings presented at NeurIPS 2022 represent a significant advancement in the pursuit of safe and ethical AI. By focusing on alignment, transparency, robustness, and fairness, DeepMind is paving the way for the development of AI systems that are not only technologically advanced but also socially responsible. These innovations have the potential to transform the way AI is integrated into society, ensuring that it serves as a force for good. As the field of AI continues to evolve, the insights provided by DeepMind’s research will undoubtedly play a crucial role in shaping the future of AI safety and ethics.
Novel Approaches To Neural Network Optimization
At the NeurIPS 2022 conference, DeepMind unveiled groundbreaking findings that promise to reshape the landscape of neural network optimization. These novel approaches, developed by some of the brightest minds in artificial intelligence, offer fresh insights into enhancing the efficiency and effectiveness of neural networks, which are the backbone of modern AI systems. As the demand for more sophisticated AI applications grows, the need for optimized neural networks becomes increasingly critical. DeepMind’s latest research addresses this need by introducing innovative techniques that could significantly improve the performance of AI models.
One of the key highlights of DeepMind’s presentation was the introduction of a new optimization algorithm that leverages advanced mathematical frameworks to streamline the training process of neural networks. This algorithm, which builds upon existing gradient descent methods, incorporates elements of stochastic optimization to better navigate the complex loss landscapes typical of deep learning models. By doing so, it reduces the time and computational resources required to train large-scale networks, making it a valuable tool for researchers and practitioners alike.
Moreover, DeepMind’s researchers have also explored the potential of meta-learning in neural network optimization. Meta-learning, often referred to as “learning to learn,” involves training models to adapt quickly to new tasks with minimal data. By integrating meta-learning strategies into the optimization process, DeepMind has demonstrated that neural networks can achieve higher levels of generalization, thereby improving their ability to perform well on unseen data. This approach not only enhances the robustness of AI models but also opens up new possibilities for their application in dynamic and unpredictable environments.
In addition to these advancements, DeepMind has made significant strides in understanding the role of regularization techniques in neural network optimization. Regularization, a method used to prevent overfitting, is crucial for ensuring that models do not simply memorize training data but instead learn underlying patterns. DeepMind’s research has introduced novel regularization methods that are more effective in maintaining a balance between model complexity and performance. These methods have shown promise in reducing the risk of overfitting, thereby enhancing the reliability of AI systems in real-world applications.
Furthermore, DeepMind’s findings emphasize the importance of collaboration between different optimization strategies. By combining various approaches, such as gradient-based methods, meta-learning, and regularization, researchers can create more robust optimization frameworks that leverage the strengths of each technique. This holistic approach not only improves the efficiency of neural network training but also fosters innovation by encouraging the exploration of new optimization paradigms.
As the field of artificial intelligence continues to evolve, the significance of DeepMind’s contributions cannot be overstated. Their novel approaches to neural network optimization have the potential to drive significant advancements in AI research and development. By addressing key challenges in the optimization process, DeepMind is paving the way for more efficient, adaptable, and reliable AI systems. These findings, presented at NeurIPS 2022, underscore the importance of continued research and collaboration in the quest to unlock the full potential of artificial intelligence. As researchers and practitioners digest these insights, the future of neural network optimization looks promising, with DeepMind’s innovations serving as a catalyst for further exploration and discovery in this rapidly advancing field.
Enhancements In AI Interpretability And Transparency
At the NeurIPS 2022 conference, DeepMind unveiled groundbreaking advancements in the realm of artificial intelligence, particularly focusing on interpretability and transparency. These developments mark a significant stride towards demystifying the often opaque decision-making processes of AI systems. As AI continues to permeate various aspects of society, the need for systems that are not only powerful but also understandable and trustworthy has become increasingly paramount. DeepMind’s latest findings address this necessity by enhancing the interpretability of AI models, thereby fostering greater transparency and trust.
One of the key highlights of DeepMind’s presentation was the introduction of novel techniques that allow for a more granular understanding of how AI models arrive at specific decisions. Traditionally, AI systems, especially those based on deep learning, have been criticized for their “black box” nature, where the internal workings are not easily interpretable by humans. DeepMind’s research aims to illuminate these inner workings by developing methods that can trace and explain the decision pathways within neural networks. This is achieved through innovative visualization tools and algorithms that map out the decision-making process, providing insights into which features or data points are most influential in the model’s predictions.
Moreover, DeepMind’s advancements are not limited to post-hoc interpretability, which involves analyzing models after they have been trained. The research also emphasizes the importance of building interpretability into the model design from the outset. By incorporating interpretability as a core component of the model architecture, DeepMind ensures that AI systems are inherently more transparent. This proactive approach not only aids in understanding but also enhances the reliability and robustness of AI models, as it allows for the identification and mitigation of biases and errors during the development phase.
In addition to interpretability, DeepMind’s findings also address the broader issue of transparency in AI systems. Transparency is crucial for fostering trust between AI systems and their users, particularly in high-stakes applications such as healthcare, finance, and autonomous vehicles. DeepMind’s research proposes frameworks for documenting and communicating the capabilities and limitations of AI models. These frameworks serve as a guide for users to better understand the scope and constraints of AI systems, thereby enabling more informed decision-making.
Furthermore, DeepMind’s commitment to transparency extends to the ethical implications of AI deployment. The research underscores the importance of aligning AI systems with human values and societal norms. By ensuring that AI models are interpretable and transparent, DeepMind aims to facilitate ethical oversight and accountability, which are essential for the responsible deployment of AI technologies.
In conclusion, DeepMind’s newest findings presented at NeurIPS 2022 represent a significant leap forward in enhancing the interpretability and transparency of AI systems. By shedding light on the decision-making processes of AI models and embedding transparency into their design, DeepMind is paving the way for more trustworthy and reliable AI applications. These advancements not only address current challenges but also set a precedent for future research and development in the field of artificial intelligence. As AI continues to evolve, the principles of interpretability and transparency will undoubtedly play a crucial role in shaping the future of this transformative technology.
Cutting-edge Developments In Quantum Computing Applications
At the NeurIPS 2022 conference, DeepMind unveiled groundbreaking findings that have the potential to revolutionize the field of quantum computing applications. As researchers and industry leaders gathered to explore the latest advancements in artificial intelligence and machine learning, DeepMind’s presentation stood out for its innovative approach to harnessing the power of quantum mechanics. The company’s research delves into the intricate relationship between quantum computing and artificial intelligence, offering new insights that could significantly enhance computational capabilities.
Quantum computing, a field that leverages the principles of quantum mechanics, promises to solve complex problems far beyond the reach of classical computers. DeepMind’s latest findings focus on optimizing quantum algorithms, which are essential for realizing the full potential of quantum computers. By improving these algorithms, DeepMind aims to address some of the most pressing challenges in quantum computing, such as error correction and qubit coherence. These improvements are crucial for the development of more reliable and efficient quantum systems.
One of the key aspects of DeepMind’s research is the application of machine learning techniques to quantum computing. By integrating machine learning models with quantum algorithms, DeepMind has demonstrated the ability to enhance the performance of quantum systems. This integration allows for more accurate predictions and optimizations, which are vital for tackling complex computational tasks. Moreover, the use of machine learning in quantum computing opens up new possibilities for automating the design and discovery of novel quantum algorithms, potentially accelerating the pace of innovation in this rapidly evolving field.
In addition to algorithmic advancements, DeepMind’s findings also highlight the potential for quantum computing to transform various industries. For instance, in the realm of pharmaceuticals, quantum computing could enable the simulation of molecular interactions at an unprecedented scale, leading to the discovery of new drugs and therapies. Similarly, in the field of materials science, quantum computers could facilitate the design of new materials with unique properties, paving the way for advancements in technology and manufacturing.
Furthermore, DeepMind’s research underscores the importance of collaboration between academia and industry in advancing quantum computing applications. By fostering partnerships with leading research institutions and technology companies, DeepMind is contributing to a broader ecosystem that supports the development and deployment of quantum technologies. This collaborative approach not only accelerates progress but also ensures that the benefits of quantum computing are widely accessible.
As the field of quantum computing continues to evolve, the implications of DeepMind’s findings are far-reaching. The integration of machine learning with quantum algorithms represents a significant step forward in overcoming the limitations of current quantum systems. By addressing key challenges such as error correction and qubit coherence, DeepMind is paving the way for more robust and scalable quantum computers. These advancements hold the promise of transforming industries and solving complex problems that were previously deemed intractable.
In conclusion, DeepMind’s presentation at NeurIPS 2022 marks a pivotal moment in the development of quantum computing applications. Through innovative research and strategic collaborations, DeepMind is at the forefront of a technological revolution that has the potential to reshape the future of computing. As these cutting-edge developments continue to unfold, the world eagerly anticipates the transformative impact of quantum computing on science, industry, and society at large.
Q&A
1. **Question:** What was one of the key focuses of DeepMind’s research presented at NeurIPS 2022?
**Answer:** One of the key focuses was on advancements in reinforcement learning, particularly in improving sample efficiency and generalization.
2. **Question:** What novel approach did DeepMind introduce for improving AI model training?
**Answer:** DeepMind introduced a novel approach called “DreamerV3,” which enhances model-based reinforcement learning by using world models to predict future states and rewards.
3. **Question:** How did DeepMind address the challenge of AI interpretability in their NeurIPS 2022 findings?
**Answer:** DeepMind presented new techniques for AI interpretability, including methods for visualizing and understanding the decision-making processes of complex neural networks.
4. **Question:** What breakthrough did DeepMind achieve in the field of protein folding, as discussed at NeurIPS 2022?
**Answer:** DeepMind showcased improvements in their AlphaFold model, which now predicts protein structures with even greater accuracy and speed, aiding in biological research.
5. **Question:** What was a significant application of DeepMind’s research in real-world scenarios highlighted at the conference?
**Answer:** A significant application was in healthcare, where DeepMind’s AI models are being used to predict patient deterioration and optimize treatment plans in hospitals.
6. **Question:** How did DeepMind’s findings contribute to the field of AI ethics and safety?
**Answer:** DeepMind emphasized the importance of AI ethics and safety by developing frameworks for ensuring that AI systems are aligned with human values and can be deployed responsibly.DeepMind’s newest findings unveiled at NeurIPS 2022 highlighted significant advancements in artificial intelligence, particularly in the areas of reinforcement learning, neural network architectures, and AI safety. The research demonstrated improved efficiency and performance in AI models, showcasing novel techniques for training and optimization. These findings contribute to the broader understanding of AI capabilities and limitations, offering potential applications across various domains while emphasizing the importance of ethical considerations and safety in AI development. Overall, DeepMind’s work continues to push the boundaries of what is possible in AI research, paving the way for future innovations.
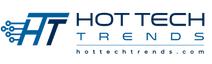