In an era where artificial intelligence (AI) is increasingly integrated into various aspects of decision-making, understanding when to trust an AI model becomes crucial. The deployment of AI systems spans diverse fields such as healthcare, finance, autonomous vehicles, and more, where the stakes can be incredibly high. Trust in AI models is not merely about their technical performance but also involves considerations of transparency, reliability, ethical implications, and alignment with human values. As AI models grow in complexity, the challenge lies in evaluating their outputs critically and ensuring they operate within the intended parameters. This necessitates a comprehensive framework for assessing AI trustworthiness, encompassing factors like data quality, model interpretability, bias mitigation, and robust testing. By establishing clear criteria for trust, stakeholders can make informed decisions about when and how to rely on AI, ultimately fostering a symbiotic relationship between humans and machines.
Understanding AI Model Transparency
In the rapidly evolving landscape of artificial intelligence, understanding when to trust an AI model is paramount. As AI systems become increasingly integrated into various aspects of our lives, from healthcare to finance, the need for transparency in these models has never been more critical. Transparency in AI refers to the clarity and openness with which an AI system’s processes and decisions can be understood by humans. This concept is essential for building trust, as it allows users to comprehend how decisions are made and to ensure that these decisions are fair, unbiased, and reliable.
To begin with, transparency in AI models can be achieved through several means, one of which is explainability. Explainability involves providing clear and understandable explanations of how an AI model arrives at a particular decision or prediction. This is particularly important in high-stakes domains such as medicine, where understanding the rationale behind a diagnosis can significantly impact patient outcomes. By offering insights into the decision-making process, explainability helps users assess the reliability of the model and fosters trust.
Moreover, transparency is closely linked to the concept of interpretability, which refers to the degree to which a human can understand the cause of a decision. While some AI models, like decision trees, are inherently interpretable due to their straightforward structure, others, such as deep neural networks, are often seen as “black boxes” due to their complexity. To address this, researchers are developing techniques to make these complex models more interpretable, such as feature importance scores and visualization tools. These methods aim to shed light on the inner workings of AI systems, thereby enhancing transparency and trust.
In addition to explainability and interpretability, accountability plays a crucial role in AI model transparency. Accountability involves ensuring that AI systems are designed and deployed in a manner that allows for responsibility to be assigned for their actions. This is vital in scenarios where AI models may cause harm or make erroneous decisions. By establishing clear lines of accountability, organizations can ensure that there are mechanisms in place to address any issues that arise, further bolstering trust in AI systems.
Furthermore, transparency is not solely a technical challenge but also an ethical one. Ethical considerations must be taken into account when developing and deploying AI models. This includes ensuring that models are free from bias and that they respect user privacy. Bias in AI can lead to unfair treatment of individuals or groups, undermining trust in the system. Therefore, it is essential to implement rigorous testing and validation processes to identify and mitigate any biases present in AI models.
In conclusion, deciding when to trust an AI model hinges on understanding its transparency. By focusing on explainability, interpretability, accountability, and ethical considerations, we can build AI systems that are not only powerful but also trustworthy. As AI continues to permeate various sectors, fostering transparency will be key to ensuring that these technologies are used responsibly and effectively. Ultimately, transparent AI models will empower users to make informed decisions, paving the way for a future where AI is a trusted partner in our daily lives.
Evaluating AI Model Accuracy
In the rapidly evolving landscape of artificial intelligence, determining when to trust an AI model is a critical consideration for researchers, developers, and end-users alike. Evaluating the accuracy of an AI model is a multifaceted process that requires a comprehensive understanding of various metrics and methodologies. As AI systems become increasingly integrated into decision-making processes across diverse sectors, the importance of accurately assessing their reliability cannot be overstated.
To begin with, accuracy is often the most straightforward metric used to evaluate an AI model. It measures the proportion of correct predictions made by the model out of the total number of predictions. While accuracy provides a general sense of a model’s performance, it is not always sufficient on its own, especially in cases where the data is imbalanced. For instance, in a dataset where 95% of the instances belong to one class, a model that predicts the majority class every time would achieve 95% accuracy, yet it would fail to capture the nuances of the minority class. Therefore, additional metrics such as precision, recall, and F1-score are essential to gain a more nuanced understanding of a model’s performance.
Precision, which measures the proportion of true positive predictions out of all positive predictions made, is particularly important in scenarios where false positives carry significant consequences. Conversely, recall, which assesses the proportion of true positive predictions out of all actual positive instances, is crucial in situations where missing a positive instance is costly. The F1-score, a harmonic mean of precision and recall, provides a balanced measure that is especially useful when dealing with imbalanced datasets.
Beyond these metrics, it is also vital to consider the context in which the AI model will be deployed. The operational environment can significantly impact the model’s performance, and thus, its accuracy in a controlled testing environment may not directly translate to real-world applications. Consequently, it is imperative to conduct thorough testing in conditions that closely mimic the intended deployment setting. This includes accounting for potential variations in data distribution, noise, and other environmental factors that could affect the model’s predictions.
Moreover, transparency and interpretability are increasingly recognized as crucial components in evaluating AI model accuracy. Understanding how a model arrives at its predictions can help identify potential biases and areas where the model may be prone to errors. Techniques such as feature importance analysis and model-agnostic interpretability methods can provide insights into the decision-making process of complex models, thereby enhancing trust in their predictions.
In addition to technical evaluations, ethical considerations play a pivotal role in deciding when to trust an AI model. Ensuring that the model’s predictions do not perpetuate or exacerbate existing biases is essential for maintaining fairness and equity. This requires a careful examination of the training data and the implementation of bias mitigation strategies throughout the model development lifecycle.
In conclusion, evaluating the accuracy of an AI model is a comprehensive process that extends beyond simple metrics. It involves a careful consideration of precision, recall, and F1-score, as well as an understanding of the operational context and the model’s interpretability. By integrating these elements with ethical considerations, stakeholders can make informed decisions about when to trust an AI model, ultimately leading to more reliable and responsible AI deployments.
Assessing AI Model Bias
In the rapidly evolving landscape of artificial intelligence, the question of when to trust an AI model has become increasingly pertinent. As AI systems are integrated into various sectors, from healthcare to finance, the potential for bias within these models poses significant ethical and practical challenges. Understanding and assessing AI model bias is crucial for ensuring that these systems operate fairly and effectively.
To begin with, it is essential to recognize that AI models are only as unbiased as the data they are trained on. Data, inherently, can reflect the prejudices and inequalities present in society. Consequently, if an AI model is trained on biased data, it is likely to perpetuate or even exacerbate these biases. For instance, if a facial recognition system is trained predominantly on images of individuals from a particular ethnic group, it may perform poorly when identifying individuals from other groups. This highlights the importance of using diverse and representative datasets to train AI models, thereby minimizing the risk of bias.
Moreover, the algorithms themselves can introduce bias. The design and implementation of an algorithm can inadvertently favor certain outcomes over others. This is often due to the assumptions and simplifications made during the development process. Therefore, it is crucial for developers to be aware of these potential pitfalls and to rigorously test their models across a wide range of scenarios. By doing so, they can identify and mitigate any biases that may arise.
In addition to data and algorithmic considerations, the context in which an AI model is deployed plays a significant role in determining its trustworthiness. An AI model that performs well in one setting may not necessarily do so in another. For example, a model designed to predict loan defaults in one country may not be applicable in another due to differences in economic conditions and cultural factors. Thus, it is vital to assess the model’s performance in the specific context in which it will be used, ensuring that it remains reliable and unbiased.
Furthermore, transparency is a key factor in assessing AI model bias. Stakeholders, including developers, users, and regulators, must have access to information about how the model was trained, the data used, and the decision-making processes involved. This transparency allows for greater scrutiny and accountability, enabling stakeholders to identify and address any biases that may be present. In this regard, explainability tools can be invaluable, providing insights into how and why a model makes certain decisions.
Another important aspect to consider is the continuous monitoring and updating of AI models. As societal norms and data evolve, so too should the models that rely on them. Regularly updating models with new data and re-evaluating their performance can help ensure that they remain unbiased and relevant. This ongoing process of assessment and refinement is crucial for maintaining trust in AI systems.
In conclusion, deciding when to trust an AI model involves a comprehensive assessment of potential biases. By considering the data, algorithms, context, transparency, and continuous monitoring, stakeholders can make informed decisions about the reliability and fairness of AI systems. As AI continues to permeate various aspects of our lives, addressing these biases is not only a technical challenge but also a moral imperative, ensuring that AI serves as a tool for progress rather than a perpetuator of inequality.
Analyzing AI Model Interpretability
In the rapidly evolving landscape of artificial intelligence, the question of when to trust an AI model has become increasingly pertinent. As AI systems are integrated into critical decision-making processes across various sectors, understanding the interpretability of these models is essential. Interpretability refers to the degree to which a human can understand the cause of a decision made by an AI model. This understanding is crucial for ensuring that AI systems are reliable, transparent, and aligned with human values.
To begin with, the interpretability of an AI model is often determined by its complexity. Simpler models, such as linear regressions or decision trees, are inherently more interpretable because their decision-making processes can be easily traced and understood. These models allow users to see how input variables are weighted and how they contribute to the final output. However, as the complexity of a model increases, as seen in deep learning models with numerous layers and parameters, interpretability tends to decrease. This complexity can obscure the decision-making process, making it challenging for users to discern how specific inputs influence outputs.
Despite this challenge, several techniques have been developed to enhance the interpretability of complex models. One such approach is the use of surrogate models, which are simpler models that approximate the behavior of more complex ones. By analyzing the surrogate model, users can gain insights into the decision-making process of the original model. Additionally, feature importance techniques, such as SHAP (Shapley Additive Explanations) values, provide a way to quantify the contribution of each input feature to the model’s predictions. These techniques help demystify the inner workings of AI models, thereby increasing trust in their outputs.
Moreover, the context in which an AI model is deployed plays a significant role in determining the level of interpretability required. In high-stakes environments, such as healthcare or autonomous driving, the need for interpretability is paramount. In these scenarios, understanding the rationale behind a model’s decision can be the difference between life and death. Consequently, stakeholders in these fields often demand models that are not only accurate but also transparent and explainable. Conversely, in low-stakes applications, such as movie recommendations, the need for interpretability may be less critical, allowing for the use of more complex models without extensive scrutiny.
Furthermore, the audience’s expertise also influences the interpretability requirements. For instance, data scientists and AI researchers may be comfortable with less interpretable models, as they possess the technical skills to understand and validate the model’s behavior. In contrast, end-users or decision-makers without a technical background may require more interpretable models to build trust and confidence in the AI system’s outputs.
In conclusion, deciding when to trust an AI model hinges on a careful analysis of its interpretability. By considering the model’s complexity, employing techniques to enhance transparency, and evaluating the context and audience, stakeholders can make informed decisions about the trustworthiness of AI systems. As AI continues to permeate various aspects of society, fostering trust through interpretability will remain a critical component of responsible AI deployment. This approach not only ensures that AI systems are aligned with human values but also paves the way for their ethical and effective integration into our daily lives.
Reviewing AI Model Reliability
In the rapidly evolving landscape of artificial intelligence, determining when to trust an AI model is a critical consideration for developers, businesses, and end-users alike. As AI systems become increasingly integrated into various aspects of daily life, from healthcare diagnostics to financial forecasting, the reliability of these models is paramount. Understanding the factors that contribute to an AI model’s reliability can help stakeholders make informed decisions about their deployment and use.
To begin with, the data used to train an AI model plays a crucial role in its reliability. High-quality, diverse, and representative datasets are essential for developing models that perform well across different scenarios. If a model is trained on biased or incomplete data, it may produce skewed results, leading to unreliable outcomes. Therefore, it is imperative to scrutinize the data sources and ensure that they encompass a wide range of variables and scenarios. This not only enhances the model’s accuracy but also its generalizability to real-world applications.
Moreover, the choice of algorithm and model architecture significantly impacts an AI model’s reliability. Different algorithms have varying strengths and weaknesses, and selecting the appropriate one depends on the specific task at hand. For instance, deep learning models are highly effective for image and speech recognition tasks, while simpler linear models might suffice for straightforward predictive tasks. Evaluating the model’s performance through rigorous testing and validation processes is essential to ascertain its reliability. Cross-validation techniques, such as k-fold validation, can provide insights into how well the model generalizes to unseen data, thereby offering a measure of its robustness.
In addition to technical considerations, transparency and interpretability are vital components of AI model reliability. Users are more likely to trust a model if they can understand how it arrives at its decisions. Explainable AI (XAI) techniques aim to make AI systems more transparent by providing insights into their decision-making processes. By elucidating the factors that influence a model’s predictions, XAI can help build trust among users and facilitate the identification of potential biases or errors in the model.
Furthermore, continuous monitoring and updating of AI models are necessary to maintain their reliability over time. As new data becomes available and environments change, models may need to be retrained or fine-tuned to ensure they remain accurate and relevant. Implementing a robust feedback loop that incorporates user input and real-world performance data can help identify areas for improvement and prevent model drift.
Ethical considerations also play a significant role in assessing AI model reliability. Ensuring that models are developed and deployed in a manner that respects privacy, fairness, and accountability is crucial for building trust. Adhering to ethical guidelines and industry standards can help mitigate potential risks associated with AI systems and foster public confidence in their use.
In conclusion, deciding when to trust an AI model involves a multifaceted evaluation of its data quality, algorithmic choices, transparency, adaptability, and ethical considerations. By carefully examining these factors, stakeholders can make informed decisions about the reliability of AI models and their suitability for specific applications. As AI technology continues to advance, ongoing research and collaboration among developers, regulators, and users will be essential to ensure that AI systems are both reliable and trustworthy.
Considering Ethical Implications of AI Models
In the rapidly evolving landscape of artificial intelligence, the question of when to trust an AI model is becoming increasingly pertinent. As these models are integrated into various aspects of society, from healthcare to finance, the ethical implications of their deployment must be carefully considered. Trust in AI models is not merely a technical issue but a multifaceted challenge that encompasses ethical, social, and legal dimensions. Therefore, understanding when to trust an AI model requires a comprehensive evaluation of these factors.
To begin with, transparency is a crucial element in establishing trust in AI models. Transparency involves the ability to understand how an AI model makes decisions, which is essential for assessing its reliability and fairness. When AI models operate as black boxes, their decision-making processes remain opaque, making it difficult to identify potential biases or errors. Consequently, ensuring that AI models are transparent can help stakeholders, including developers, users, and regulators, to scrutinize their operations and make informed decisions about their deployment.
Moreover, accountability is another critical factor in determining the trustworthiness of AI models. Accountability refers to the mechanisms in place to hold developers and users responsible for the outcomes of AI systems. This includes establishing clear lines of responsibility and ensuring that there are consequences for misuse or harm caused by AI models. By fostering a culture of accountability, organizations can mitigate risks and build public confidence in AI technologies.
In addition to transparency and accountability, the ethical implications of AI models also hinge on their fairness and inclusivity. AI models are often trained on large datasets that may contain biases reflecting historical and societal inequalities. If these biases are not addressed, AI models can perpetuate or even exacerbate discrimination. Therefore, it is imperative to implement strategies that ensure AI models are fair and inclusive, such as diverse training datasets and bias detection algorithms. By doing so, developers can create AI systems that are more equitable and just.
Furthermore, the ethical use of AI models necessitates a consideration of privacy concerns. As AI systems often rely on vast amounts of personal data, safeguarding this information is paramount. Ensuring that AI models comply with data protection regulations and employ robust security measures can help protect individuals’ privacy and foster trust in these technologies. Additionally, obtaining informed consent from users and providing them with control over their data are essential practices in respecting privacy rights.
As we navigate the ethical implications of AI models, it is also important to consider the broader societal impact of these technologies. AI models have the potential to transform industries and reshape the workforce, leading to significant economic and social changes. Therefore, it is crucial to engage in ongoing dialogue with diverse stakeholders, including policymakers, industry leaders, and the public, to address the societal implications of AI deployment. By fostering collaboration and inclusivity, we can ensure that AI technologies are developed and used in ways that benefit society as a whole.
In conclusion, deciding when to trust an AI model involves a careful consideration of ethical implications, including transparency, accountability, fairness, privacy, and societal impact. By addressing these factors, we can build trust in AI technologies and harness their potential for positive change. As AI continues to advance, it is imperative that we remain vigilant in our ethical considerations, ensuring that these powerful tools are used responsibly and for the greater good.
Q&A
1. **Question:** What factors should be considered when deciding to trust an AI model?
**Answer:** Factors include the model’s accuracy, transparency, explainability, robustness, bias, and alignment with ethical standards.
2. **Question:** How does transparency affect trust in an AI model?
**Answer:** Transparency allows users to understand how the model makes decisions, which can increase trust by making the process more understandable and accountable.
3. **Question:** Why is explainability important in trusting an AI model?
**Answer:** Explainability helps users comprehend the reasoning behind the model’s outputs, making it easier to trust and verify its decisions.
4. **Question:** What role does bias play in trusting an AI model?
**Answer:** Bias can lead to unfair or inaccurate outcomes, so identifying and mitigating bias is crucial for ensuring the model’s trustworthiness.
5. **Question:** How can robustness influence the trustworthiness of an AI model?
**Answer:** A robust model performs reliably under various conditions and inputs, which enhances trust by demonstrating consistent and dependable behavior.
6. **Question:** What ethical considerations are important when trusting an AI model?
**Answer:** Ethical considerations include ensuring the model respects privacy, fairness, and does not cause harm, aligning with societal values and norms.Deciding when to trust an AI model involves evaluating several key factors: the model’s accuracy and performance metrics, the quality and representativeness of the training data, the transparency and interpretability of the model, and the context in which it is applied. Trust should be based on rigorous testing and validation, ensuring the model performs reliably across diverse scenarios. Additionally, understanding the model’s limitations and potential biases is crucial. Trust is also contingent on the ethical considerations and alignment with human values. Ultimately, trust in an AI model is a balance between technical robustness and ethical responsibility, requiring continuous monitoring and adaptation to new data and contexts.
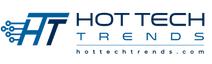