The rapid advancement of generative artificial intelligence (AI) technologies has ushered in a new era of innovation, creativity, and efficiency across various sectors. However, alongside these promising developments, there arises a critical need to assess the social and ethical implications associated with their deployment. Generative AI, characterized by its ability to produce content such as text, images, music, and even complex simulations, poses unique challenges and opportunities that demand careful consideration. As these systems become increasingly integrated into daily life, they raise profound questions about privacy, security, bias, accountability, and the broader impact on human creativity and employment. This introduction explores the multifaceted social and ethical dimensions of generative AI, emphasizing the importance of responsible development and deployment to ensure that these technologies benefit society while minimizing potential harms.
Understanding Bias in Generative AI Models
Generative AI models have rapidly advanced in recent years, offering unprecedented capabilities in creating text, images, and other forms of media. However, as these models become more integrated into various aspects of society, it is crucial to understand the social and ethical implications they carry, particularly concerning bias. Bias in generative AI models can manifest in numerous ways, often reflecting and amplifying existing societal prejudices. This issue arises primarily from the data used to train these models, which can inadvertently encode biases present in the real world. Consequently, when these models generate content, they may perpetuate stereotypes or unfairly represent certain groups, leading to significant ethical concerns.
To comprehend the roots of bias in generative AI, it is essential to examine the data sources and training processes involved. Generative AI models learn from vast datasets, which are often scraped from the internet or other large-scale repositories. These datasets, while extensive, are not immune to the biases inherent in human language and culture. For instance, if a dataset contains more content from a particular demographic or cultural perspective, the model may develop a skewed understanding of the world, favoring that perspective over others. This imbalance can result in outputs that reinforce existing power dynamics and marginalize underrepresented groups.
Moreover, the complexity of generative AI models adds another layer of difficulty in identifying and mitigating bias. These models, often described as “black boxes,” operate through intricate neural networks that make it challenging to trace how specific inputs lead to particular outputs. This opacity complicates efforts to diagnose and correct biased behavior, as developers may struggle to pinpoint the exact source of the problem. Consequently, addressing bias in generative AI requires a multifaceted approach, combining technical solutions with a broader understanding of societal context.
One potential strategy for mitigating bias involves curating more balanced and representative training datasets. By ensuring that the data used to train generative AI models encompasses a diverse range of perspectives and experiences, developers can help reduce the risk of biased outputs. However, this approach is not without its challenges, as it requires careful consideration of what constitutes a “balanced” dataset and how to achieve it without introducing new forms of bias. Additionally, ongoing monitoring and evaluation of model outputs are necessary to identify and address any emerging biases that may arise as the model interacts with new data.
Another promising avenue for addressing bias in generative AI is the development of fairness-aware algorithms. These algorithms are designed to detect and mitigate bias during the training process, ensuring that the model’s outputs are more equitable and representative. While still an emerging field, fairness-aware algorithms hold significant potential for reducing bias in generative AI, provided they are implemented thoughtfully and in conjunction with other strategies.
Ultimately, understanding and addressing bias in generative AI models is a complex and ongoing challenge that requires collaboration between technologists, ethicists, and policymakers. By fostering a multidisciplinary dialogue, stakeholders can work together to develop solutions that not only enhance the technical robustness of generative AI but also ensure its ethical deployment in society. As generative AI continues to evolve, it is imperative that we remain vigilant in assessing its social and ethical implications, striving to create models that reflect the diversity and complexity of the world they seek to emulate.
The Impact of Generative AI on Privacy and Data Security
The advent of generative AI has ushered in a new era of technological innovation, promising to revolutionize industries ranging from entertainment to healthcare. However, as with any transformative technology, it brings with it a host of social and ethical implications that warrant careful consideration. Among these, the impact of generative AI on privacy and data security stands out as a particularly pressing concern. As generative AI systems become increasingly sophisticated, they require vast amounts of data to function effectively. This data often includes personal information, raising significant privacy issues. The collection, storage, and processing of such data can lead to potential breaches of privacy if not managed with stringent safeguards. Consequently, individuals may find their personal information exposed or misused, leading to a loss of trust in AI technologies and the organizations that deploy them.
Moreover, the ability of generative AI to create highly realistic content, such as deepfakes, further complicates the privacy landscape. These AI-generated artifacts can be used to impersonate individuals, potentially leading to identity theft or reputational damage. The ease with which such content can be produced and disseminated poses a challenge to existing legal and regulatory frameworks, which may struggle to keep pace with the rapid evolution of AI capabilities. In this context, it becomes imperative for policymakers to develop robust regulations that address the unique challenges posed by generative AI, ensuring that privacy rights are upheld while fostering innovation.
In addition to privacy concerns, generative AI also raises significant data security issues. The reliance on large datasets makes AI systems attractive targets for cyberattacks, as malicious actors seek to exploit vulnerabilities in data storage and processing infrastructures. A successful breach could result in the unauthorized access to sensitive information, with potentially devastating consequences for individuals and organizations alike. To mitigate these risks, it is essential for developers and users of generative AI to implement comprehensive security measures, including encryption, access controls, and regular audits of data handling practices.
Furthermore, the ethical implications of generative AI extend beyond privacy and data security. The technology’s potential to automate decision-making processes raises questions about accountability and transparency. As AI systems are increasingly used to make decisions that affect people’s lives, such as in hiring or lending, it is crucial to ensure that these systems operate fairly and without bias. This requires a commitment to transparency in AI development, allowing stakeholders to understand how decisions are made and to challenge them if necessary.
The intersection of generative AI with privacy and data security also highlights the need for interdisciplinary collaboration. Addressing the complex challenges posed by this technology requires input from a diverse range of experts, including technologists, ethicists, legal scholars, and policymakers. By working together, these stakeholders can develop comprehensive strategies that balance the benefits of generative AI with the need to protect individual rights and societal values.
In conclusion, while generative AI holds immense potential to drive progress and innovation, it also presents significant social and ethical challenges, particularly in the realms of privacy and data security. As we continue to integrate AI into various aspects of our lives, it is crucial to remain vigilant and proactive in addressing these issues. By fostering a culture of responsibility and collaboration, we can harness the power of generative AI while safeguarding the fundamental rights and values that underpin our society.
Ethical Considerations in AI-Generated Content
The rapid advancement of generative artificial intelligence (AI) has ushered in a new era of content creation, offering unprecedented opportunities and challenges. As AI systems become increasingly capable of producing text, images, music, and even video, it is crucial to assess the social and ethical implications of these technologies. The ethical considerations surrounding AI-generated content are multifaceted, encompassing issues of authorship, authenticity, bias, and the potential for misuse.
To begin with, the question of authorship in AI-generated content raises significant ethical concerns. Traditionally, authorship has been associated with human creativity and intellectual labor. However, when AI systems generate content, the lines between human and machine authorship become blurred. This ambiguity poses challenges in attributing credit and responsibility. For instance, if an AI-generated article contains misinformation, determining accountability becomes complex. Should the blame lie with the developers of the AI, the users who deployed it, or the AI itself? This conundrum necessitates a reevaluation of existing intellectual property laws and ethical guidelines to accommodate the unique nature of AI-generated works.
Moreover, the authenticity of AI-generated content is another pressing ethical issue. As AI systems become more sophisticated, they can produce content that is indistinguishable from that created by humans. This capability raises concerns about the potential for deception and manipulation. For example, AI-generated deepfake videos can convincingly portray individuals saying or doing things they never did, posing significant risks to personal reputations and public trust. Consequently, there is a growing need for mechanisms to verify the authenticity of content and to educate the public about the potential for AI-driven misinformation.
In addition to authorship and authenticity, bias in AI-generated content is a critical ethical consideration. AI systems learn from vast datasets, which often contain historical biases and prejudices. If these biases are not addressed, AI-generated content can perpetuate and even amplify existing inequalities. For instance, language models trained on biased data may produce content that reflects gender, racial, or cultural stereotypes. This not only undermines the credibility of AI-generated content but also exacerbates social divisions. Therefore, it is imperative for developers to implement strategies to identify and mitigate bias in AI systems, ensuring that the content they produce is fair and inclusive.
Furthermore, the potential for misuse of AI-generated content cannot be overlooked. While these technologies offer creative possibilities, they also present opportunities for malicious actors to exploit them. The ease with which AI can generate convincing fake news, propaganda, or harmful content poses significant risks to societal stability and democratic processes. As such, there is an urgent need for regulatory frameworks and ethical guidelines to govern the use of generative AI, balancing innovation with the protection of public interests.
In conclusion, the ethical considerations surrounding AI-generated content are complex and multifaceted, requiring careful examination and proactive measures. As generative AI continues to evolve, it is essential for stakeholders, including developers, policymakers, and society at large, to engage in ongoing dialogue and collaboration. By addressing issues of authorship, authenticity, bias, and misuse, we can harness the potential of generative AI while safeguarding ethical standards and promoting a more equitable and trustworthy digital landscape.
Generative AI and Its Role in Misinformation
Generative AI, a rapidly advancing field within artificial intelligence, has garnered significant attention for its ability to create content that closely mimics human output. This technology, which includes models capable of generating text, images, and even music, holds immense potential for innovation across various sectors. However, as with any powerful tool, it also presents challenges, particularly in the realm of misinformation. The proliferation of generative AI raises critical social and ethical questions that must be addressed to mitigate its potential negative impacts.
One of the primary concerns surrounding generative AI is its capacity to produce convincing yet false information. These AI systems can generate text that appears authentic, making it increasingly difficult for individuals to discern fact from fiction. This capability poses a significant threat to the integrity of information, as it can be exploited to create misleading news articles, fake reviews, or even fabricated social media posts. Consequently, the spread of misinformation can be accelerated, leading to a misinformed public and potentially influencing public opinion and behavior in harmful ways.
Moreover, the ability of generative AI to create deepfakes—highly realistic but fake audio and video content—further complicates the landscape of misinformation. Deepfakes can be used to impersonate individuals, including public figures, thereby eroding trust in media and institutions. The potential for such technology to be used in political campaigns or to incite social unrest is a pressing concern. As these AI-generated contents become more sophisticated, the challenge of detecting and countering them grows, necessitating the development of advanced tools and strategies to identify and mitigate their impact.
In addition to the technical challenges, the ethical implications of generative AI in misinformation are profound. The creators and deployers of these technologies must grapple with questions of responsibility and accountability. Who should be held accountable when AI-generated content causes harm? How can developers ensure that their creations are not misused? These questions highlight the need for robust ethical guidelines and regulatory frameworks to govern the use of generative AI. Such measures could include transparency requirements, where AI-generated content is clearly labeled, and the implementation of ethical standards in the development and deployment of AI systems.
Furthermore, the societal impact of generative AI extends beyond misinformation. It also raises concerns about privacy and consent, as individuals may find their likeness or personal data used without permission in AI-generated content. This underscores the importance of establishing clear consent protocols and privacy protections to safeguard individuals’ rights in the digital age.
In response to these challenges, collaboration between technologists, policymakers, and ethicists is crucial. By working together, these stakeholders can develop comprehensive strategies to address the social and ethical implications of generative AI. This includes investing in research to improve the detection of AI-generated misinformation, as well as fostering public awareness and education to enhance media literacy and critical thinking skills.
In conclusion, while generative AI offers exciting possibilities for innovation, its role in misinformation presents significant social and ethical challenges. Addressing these issues requires a multifaceted approach that combines technological solutions with ethical considerations and regulatory oversight. By proactively engaging with these challenges, society can harness the benefits of generative AI while minimizing its potential harms, ensuring that this powerful technology serves the greater good.
The Societal Effects of AI-Driven Automation
The advent of generative AI has ushered in a new era of technological advancement, promising to revolutionize various sectors through automation. As these AI-driven systems become increasingly integrated into the fabric of society, it is crucial to assess their social and ethical implications. The societal effects of AI-driven automation are multifaceted, encompassing economic, cultural, and ethical dimensions that warrant careful consideration.
To begin with, the economic impact of generative AI is profound. Automation has the potential to significantly enhance productivity by performing tasks more efficiently than human labor. This can lead to cost savings for businesses and potentially lower prices for consumers. However, the flip side of this economic boon is the displacement of jobs. As AI systems become capable of performing complex tasks, there is a growing concern about the future of work. Many fear that widespread automation could lead to significant job losses, particularly in industries that rely heavily on routine and repetitive tasks. This raises important questions about how society will adapt to these changes and what measures can be implemented to support displaced workers.
Moreover, the cultural implications of AI-driven automation are equally significant. As AI systems become more prevalent, they have the potential to influence cultural norms and values. For instance, the way we interact with technology and each other may change as AI becomes more integrated into our daily lives. This could lead to shifts in social dynamics, as people may rely more on AI for decision-making and problem-solving. Additionally, the content generated by AI, such as art, music, and literature, challenges traditional notions of creativity and authorship. This prompts a reevaluation of what it means to be creative and how society values human versus machine-generated content.
Transitioning to the ethical considerations, the deployment of generative AI raises several moral dilemmas. One of the primary concerns is the potential for bias in AI systems. Since these systems are trained on large datasets, they can inadvertently learn and perpetuate existing biases present in the data. This can lead to unfair outcomes, particularly in areas such as hiring, law enforcement, and lending, where biased decisions can have significant consequences. Ensuring fairness and transparency in AI systems is therefore a critical ethical challenge that must be addressed.
Furthermore, the issue of accountability in AI-driven automation is a pressing ethical concern. As AI systems become more autonomous, determining responsibility for their actions becomes increasingly complex. This raises questions about who should be held accountable when an AI system makes a mistake or causes harm. Establishing clear guidelines and regulations for accountability is essential to ensure that AI systems are used responsibly and ethically.
In conclusion, while generative AI offers numerous benefits, its societal effects are complex and multifaceted. The economic, cultural, and ethical implications of AI-driven automation must be carefully considered to ensure that the technology is harnessed for the greater good. As we continue to integrate AI into various aspects of society, it is imperative to engage in ongoing dialogue and collaboration among stakeholders, including policymakers, technologists, and the public, to navigate the challenges and opportunities presented by this transformative technology. By doing so, we can work towards a future where AI-driven automation enhances human well-being while upholding social and ethical values.
Addressing Accountability in AI-Generated Decisions
As the capabilities of generative artificial intelligence (AI) continue to expand, the social and ethical implications of its deployment have become a focal point of discussion among technologists, ethicists, and policymakers. One of the most pressing concerns is the issue of accountability in AI-generated decisions. This concern arises from the fact that AI systems, particularly those employing machine learning and neural networks, often operate as “black boxes,” making it difficult to trace how specific decisions are made. Consequently, when these systems are used in critical areas such as healthcare, finance, or criminal justice, the lack of transparency can lead to significant ethical dilemmas.
To address these challenges, it is essential to first understand the nature of decision-making in generative AI systems. Unlike traditional software, which follows explicit instructions provided by human programmers, generative AI models learn from vast datasets to identify patterns and make predictions. This learning process, while powerful, introduces a level of complexity that can obscure the rationale behind specific outputs. As a result, when an AI system generates a decision that impacts human lives, determining who is responsible for that decision becomes a complex issue. Is it the developers who created the algorithm, the organization that deployed it, or the AI itself?
In response to these questions, there is a growing consensus that a multi-faceted approach is necessary to ensure accountability. One potential solution is the implementation of robust auditing mechanisms. By regularly examining AI systems for biases and errors, organizations can identify and rectify issues before they lead to harmful outcomes. Moreover, these audits can provide a clearer understanding of how AI systems arrive at their decisions, thereby enhancing transparency and trust.
Another critical aspect of addressing accountability is the establishment of clear regulatory frameworks. Governments and international bodies are increasingly recognizing the need for regulations that define the responsibilities of AI developers and users. Such regulations could mandate the inclusion of explainability features in AI systems, ensuring that decisions can be traced back to specific data inputs and algorithmic processes. Furthermore, these frameworks could impose penalties for non-compliance, thereby incentivizing organizations to prioritize ethical considerations in their AI deployments.
In addition to regulatory measures, fostering a culture of ethical AI development within organizations is crucial. This involves training developers and engineers to consider the social implications of their work and encouraging them to prioritize fairness and inclusivity in their designs. By embedding ethical considerations into the development process, organizations can proactively address potential issues before they arise.
Moreover, public engagement and education play a vital role in ensuring accountability. By raising awareness about the capabilities and limitations of generative AI, society can better understand the potential risks and benefits associated with its use. This understanding can empower individuals to advocate for responsible AI practices and hold organizations accountable for their actions.
In conclusion, addressing accountability in AI-generated decisions requires a comprehensive approach that combines technical, regulatory, and cultural strategies. By implementing robust auditing mechanisms, establishing clear regulatory frameworks, fostering ethical development practices, and engaging the public, society can navigate the complex landscape of generative AI responsibly. As these technologies continue to evolve, it is imperative that we remain vigilant in our efforts to ensure that AI serves the greater good, rather than exacerbating existing inequalities or creating new ethical dilemmas.
Q&A
1. **What are the potential social impacts of generative AI?**
Generative AI can influence job markets by automating creative tasks, potentially leading to job displacement in fields like design, writing, and art. It can also affect social interactions by creating realistic deepfakes, which may contribute to misinformation and erosion of trust.
2. **How does generative AI pose ethical challenges in content creation?**
Generative AI can produce content that infringes on intellectual property rights, as it may generate works similar to existing copyrighted material. Additionally, it can create harmful or biased content if the training data contains such biases.
3. **What are the privacy concerns associated with generative AI?**
Generative AI can be used to synthesize personal data, such as creating fake identities or mimicking individuals’ voices and images, leading to privacy violations and identity theft.
4. **How can generative AI affect decision-making processes?**
Generative AI can influence decision-making by generating persuasive content or recommendations that may not be transparent or explainable, potentially leading to biased or unethical outcomes.
5. **What are the implications of generative AI on misinformation?**
Generative AI can produce highly convincing fake news, images, and videos, making it challenging to distinguish between real and fake information, thus exacerbating the spread of misinformation.
6. **How can society address the ethical concerns of generative AI?**
Addressing these concerns requires developing robust ethical guidelines, implementing regulatory frameworks, promoting transparency in AI systems, and fostering public awareness and education about the capabilities and limitations of generative AI.Generative AI, with its ability to create content ranging from text and images to music and video, presents significant social and ethical implications that require careful assessment. Socially, it has the potential to democratize content creation, enhance creativity, and drive innovation across various industries. However, it also poses risks such as the proliferation of misinformation, deepfakes, and the erosion of trust in digital content. Ethically, generative AI raises concerns about authorship, intellectual property rights, and the potential for bias and discrimination embedded within AI-generated outputs. Additionally, there are implications for privacy, as AI systems often require vast amounts of data, which can lead to surveillance and data misuse. To address these challenges, it is crucial to establish robust ethical guidelines, promote transparency in AI development, and ensure accountability for AI-generated content. Engaging diverse stakeholders, including technologists, ethicists, policymakers, and the public, is essential to navigate the complex landscape of generative AI responsibly. Ultimately, balancing innovation with ethical considerations will be key to harnessing the benefits of generative AI while mitigating its risks.
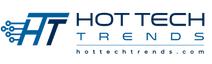