AlphaFold, an artificial intelligence system developed by DeepMind, has made a groundbreaking advancement in the field of biology by solving one of the most enduring challenges: the protein folding problem. Proteins, essential molecules that perform a vast array of functions within living organisms, must fold into specific three-dimensional shapes to function correctly. Predicting these shapes from amino acid sequences has been a complex puzzle for scientists for decades. AlphaFold’s innovative approach utilizes deep learning techniques to accurately predict protein structures, offering unprecedented insights into biological processes and accelerating research in drug discovery, disease understanding, and bioengineering. This breakthrough represents a significant leap forward in computational biology, promising to transform our understanding of life at the molecular level.
Understanding Protein Folding: How AlphaFold Revolutionizes Biology
The field of biology has long been captivated by the intricate process of protein folding, a fundamental mechanism that dictates the structure and function of proteins within living organisms. Proteins, composed of long chains of amino acids, must fold into specific three-dimensional shapes to perform their biological functions effectively. Misfolded proteins can lead to a myriad of diseases, including Alzheimer’s and cystic fibrosis, making the understanding of protein folding a critical area of research. For decades, scientists have grappled with the challenge of predicting protein structures based solely on their amino acid sequences, a problem often referred to as the “protein folding problem.” The complexity of this task arises from the astronomical number of possible configurations a protein chain can adopt, making experimental determination both time-consuming and costly.
In recent years, a groundbreaking development has emerged from the realm of artificial intelligence, offering a promising solution to this longstanding conundrum. AlphaFold, an AI system developed by DeepMind, has revolutionized the way scientists approach protein structure prediction. By leveraging deep learning techniques, AlphaFold has demonstrated an unprecedented ability to predict protein structures with remarkable accuracy. This achievement marks a significant milestone in computational biology, as it provides researchers with a powerful tool to explore the vast landscape of protein structures more efficiently than ever before.
The success of AlphaFold can be attributed to its innovative approach, which combines vast amounts of biological data with sophisticated algorithms. By training on known protein structures, AlphaFold has learned to recognize patterns and relationships within the data, enabling it to predict the folding of new proteins with remarkable precision. This capability was showcased in the Critical Assessment of protein Structure Prediction (CASP) competition, where AlphaFold outperformed other methods, achieving accuracy levels comparable to experimental techniques.
The implications of AlphaFold’s success are profound, extending across various domains of biological research. For instance, in drug discovery, understanding the precise structure of target proteins is crucial for designing effective therapeutics. AlphaFold’s predictions can accelerate this process by providing detailed structural insights, potentially leading to the development of new drugs for previously intractable diseases. Moreover, in the field of synthetic biology, researchers can now design novel proteins with specific functions, opening up possibilities for innovative applications in medicine, agriculture, and environmental science.
Furthermore, AlphaFold’s impact is not limited to practical applications; it also enriches our fundamental understanding of biology. By elucidating the structures of proteins that were previously difficult to study, AlphaFold contributes to a deeper comprehension of cellular processes and molecular interactions. This knowledge can inform future research directions and inspire new hypotheses about the mechanisms underlying life itself.
As we reflect on the achievements of AlphaFold, it is essential to acknowledge the collaborative efforts that have driven this advancement. The integration of computational power, biological expertise, and interdisciplinary collaboration has been instrumental in overcoming the challenges of protein folding prediction. Looking ahead, the continued refinement of AI models like AlphaFold holds the promise of further breakthroughs, potentially unraveling even more complex biological mysteries.
In conclusion, AlphaFold represents a transformative leap in our ability to understand and predict protein structures, offering a powerful tool that bridges the gap between computational predictions and experimental validation. Its impact on biology is profound, with far-reaching implications for research, medicine, and our fundamental understanding of life. As we continue to explore the potential of AI in biology, AlphaFold stands as a testament to the remarkable possibilities that arise when cutting-edge technology meets scientific inquiry.
The Impact of AlphaFold on Drug Discovery and Development
The advent of AlphaFold, a groundbreaking artificial intelligence system developed by DeepMind, has marked a significant milestone in the field of structural biology, particularly in drug discovery and development. By accurately predicting protein structures, AlphaFold addresses one of the most intricate challenges in biology, thereby opening new avenues for scientific exploration and pharmaceutical innovation. Understanding the three-dimensional structure of proteins is crucial, as it provides insights into their functions and interactions, which are essential for designing effective drugs. Traditionally, determining protein structures has been a labor-intensive and costly process, often taking years of meticulous experimentation. However, AlphaFold’s ability to predict these structures with remarkable accuracy has the potential to revolutionize this aspect of drug discovery.
The implications of AlphaFold’s success are profound, as it accelerates the initial stages of drug development. By providing researchers with detailed protein models, AlphaFold enables a more efficient identification of potential drug targets. This capability is particularly beneficial in the context of diseases where protein misfolding or malfunction plays a critical role, such as Alzheimer’s, Parkinson’s, and various cancers. With a clearer understanding of the structural underpinnings of these diseases, scientists can design molecules that specifically interact with the target proteins, thereby enhancing the precision and efficacy of therapeutic interventions.
Moreover, AlphaFold’s impact extends beyond the identification of drug targets to the optimization of drug candidates. In the drug development pipeline, once a potential drug target is identified, the next step involves designing molecules that can effectively bind to the target protein. AlphaFold’s accurate predictions facilitate the rational design of these molecules by providing insights into the binding sites and conformational changes of proteins. This knowledge allows researchers to predict how a drug candidate will interact with its target, thus enabling the optimization of its binding affinity and specificity. Consequently, this reduces the reliance on trial-and-error methods, which are often time-consuming and resource-intensive.
Furthermore, AlphaFold’s contributions are not limited to the pharmaceutical industry alone. Its ability to predict protein structures has significant implications for biotechnology and synthetic biology. For instance, in enzyme engineering, understanding the structure of enzymes is crucial for modifying their activity or stability for industrial applications. AlphaFold’s predictions can guide the design of enzymes with enhanced properties, thereby improving the efficiency of processes such as biofuel production and waste degradation.
In addition to its practical applications, AlphaFold’s success underscores the transformative potential of artificial intelligence in scientific research. By solving a problem that has eluded scientists for decades, AlphaFold exemplifies how AI can complement human expertise and drive innovation. This achievement also highlights the importance of interdisciplinary collaboration, as it brings together insights from biology, computer science, and mathematics to address complex challenges.
In conclusion, AlphaFold represents a paradigm shift in the field of structural biology, with far-reaching implications for drug discovery and development. Its ability to predict protein structures with unprecedented accuracy not only accelerates the identification and optimization of drug candidates but also enhances our understanding of fundamental biological processes. As researchers continue to explore the potential of AlphaFold and similar AI technologies, the future of drug discovery promises to be more efficient, precise, and innovative, ultimately leading to improved therapeutic outcomes for patients worldwide.
AlphaFold and Its Role in Advancing Genomic Research
AlphaFold, a groundbreaking artificial intelligence system developed by DeepMind, has made significant strides in the field of genomic research by addressing one of biology’s most enduring challenges: protein structure prediction. Proteins, the workhorses of the cell, are composed of long chains of amino acids that fold into intricate three-dimensional shapes. These shapes are crucial because they determine the protein’s function within biological processes. Understanding protein structures is essential for numerous applications, including drug discovery, disease understanding, and synthetic biology. However, predicting how a protein will fold based solely on its amino acid sequence has been a formidable task for scientists for decades.
The introduction of AlphaFold has revolutionized this area of research. By leveraging deep learning techniques, AlphaFold can predict protein structures with remarkable accuracy. This achievement was highlighted in the 2020 Critical Assessment of protein Structure Prediction (CASP) competition, where AlphaFold outperformed other methods, achieving a median Global Distance Test (GDT) score of 92.4 out of 100. This level of precision is comparable to experimental methods such as X-ray crystallography and cryo-electron microscopy, which are time-consuming and costly. Consequently, AlphaFold’s ability to predict protein structures quickly and accurately has the potential to accelerate research across various domains.
Moreover, the implications of AlphaFold’s success extend beyond mere structural predictions. By providing insights into protein folding, AlphaFold aids researchers in understanding the fundamental principles of molecular biology. This understanding is crucial for elucidating the mechanisms of diseases caused by misfolded proteins, such as Alzheimer’s and Parkinson’s. Furthermore, AlphaFold’s predictions can assist in the design of novel proteins with specific functions, opening new avenues in synthetic biology and biotechnology. For instance, researchers can engineer enzymes with enhanced capabilities for industrial applications or develop proteins that can neutralize environmental toxins.
In addition to its scientific contributions, AlphaFold represents a significant advancement in the application of artificial intelligence to complex biological problems. The system’s success underscores the potential of AI to transform scientific research by providing tools that can handle vast amounts of data and uncover patterns that are not immediately apparent to human researchers. This capability is particularly valuable in genomics, where the sheer volume of data generated by sequencing technologies can be overwhelming. By integrating AI with traditional research methods, scientists can gain deeper insights and make more informed decisions.
Furthermore, the open-access nature of AlphaFold’s predictions has democratized access to protein structure data. DeepMind’s decision to release the AlphaFold Protein Structure Database, which contains predictions for nearly all known proteins, has empowered researchers worldwide to explore new hypotheses and conduct experiments that were previously out of reach. This open-access model fosters collaboration and accelerates scientific discovery by removing barriers to information sharing.
In conclusion, AlphaFold has solved a major biological mystery by accurately predicting protein structures, thereby advancing genomic research in unprecedented ways. Its impact is felt across various scientific disciplines, from understanding disease mechanisms to designing novel proteins. As researchers continue to explore the potential applications of AlphaFold, it is clear that this AI system has not only addressed a longstanding challenge but also paved the way for future innovations in biology and beyond. The integration of artificial intelligence into scientific research promises to unlock new possibilities, ultimately enhancing our understanding of life at the molecular level.
How AlphaFold Enhances Our Knowledge of Human Diseases
AlphaFold, a groundbreaking artificial intelligence system developed by DeepMind, has revolutionized the field of structural biology by accurately predicting protein structures. This advancement has profound implications for our understanding of human diseases, as proteins play a crucial role in virtually all biological processes. The ability to predict protein structures with high accuracy allows researchers to gain insights into the molecular mechanisms underlying various diseases, thereby enhancing our knowledge and opening new avenues for therapeutic interventions.
Proteins are complex molecules composed of amino acids, and their functions are determined by their three-dimensional structures. Misfolded proteins or those with aberrant structures can lead to a range of diseases, including Alzheimer’s, Parkinson’s, and cystic fibrosis. Traditionally, determining protein structures has been a labor-intensive and time-consuming process, often requiring years of experimental work using techniques such as X-ray crystallography or cryo-electron microscopy. However, AlphaFold’s ability to predict protein structures with remarkable precision has significantly accelerated this process, providing researchers with a powerful tool to study proteins associated with human diseases.
One of the most significant contributions of AlphaFold is its potential to elucidate the structural basis of genetic mutations that cause diseases. Many genetic disorders result from single amino acid changes that alter protein structure and function. By accurately predicting how these mutations affect protein folding, AlphaFold enables scientists to better understand the molecular consequences of genetic variations. This knowledge is crucial for developing targeted therapies that can correct or compensate for the effects of these mutations, thereby offering hope for patients with rare genetic diseases.
Moreover, AlphaFold’s predictions can aid in the identification of novel drug targets. Understanding the structure of disease-related proteins allows researchers to identify potential binding sites for small molecules or biologics, which can modulate protein function. This structural information is invaluable in the drug discovery process, as it guides the design of molecules that can specifically interact with target proteins, increasing the likelihood of developing effective and safe therapeutics. Consequently, AlphaFold’s contributions extend beyond basic research, impacting the pharmaceutical industry by streamlining the drug development pipeline.
In addition to its applications in drug discovery, AlphaFold enhances our understanding of complex biological systems and pathways. Proteins often interact with each other and with other biomolecules to form intricate networks that regulate cellular functions. By providing detailed structural information, AlphaFold facilitates the study of these interactions, enabling researchers to map out signaling pathways and identify key nodes that may be disrupted in disease states. This systems-level understanding is essential for developing comprehensive treatment strategies that address the multifaceted nature of many diseases.
Furthermore, AlphaFold’s impact is not limited to human health; it also extends to other areas of biology, such as agriculture and environmental science. For instance, understanding the structure of plant proteins can lead to the development of crops with improved resistance to pests and diseases, while insights into microbial proteins can inform bioremediation efforts to address environmental pollution. Thus, AlphaFold’s ability to predict protein structures has far-reaching implications across various scientific disciplines.
In conclusion, AlphaFold represents a monumental leap forward in our ability to understand the molecular underpinnings of human diseases. By providing accurate protein structure predictions, it enhances our knowledge of disease mechanisms, facilitates drug discovery, and contributes to a broader understanding of biological systems. As researchers continue to harness the power of AlphaFold, we can anticipate significant advancements in the diagnosis, treatment, and prevention of diseases, ultimately improving human health and well-being.
The Future of Structural Biology in the Age of AlphaFold
The advent of AlphaFold, an artificial intelligence system developed by DeepMind, marks a transformative moment in the field of structural biology. For decades, scientists have grappled with the challenge of predicting protein structures, a task that is crucial for understanding biological processes and developing new therapeutics. Proteins, composed of long chains of amino acids, fold into intricate three-dimensional shapes that determine their function. However, predicting these shapes from amino acid sequences has been a formidable challenge, often requiring years of laborious experimental work. AlphaFold has revolutionized this process by accurately predicting protein structures in a matter of days, thus solving a major biological mystery that has long eluded researchers.
The implications of AlphaFold’s success are profound, as it opens new avenues for scientific exploration and innovation. By providing detailed insights into protein structures, AlphaFold enables researchers to better understand the molecular mechanisms underlying various diseases. This understanding is crucial for the development of targeted therapies, as it allows scientists to design drugs that can precisely interact with specific proteins. Moreover, AlphaFold’s ability to predict protein structures with high accuracy accelerates the drug discovery process, potentially leading to faster development of new treatments for a wide range of conditions.
In addition to its impact on drug discovery, AlphaFold is poised to advance our understanding of fundamental biological processes. Proteins are involved in virtually every aspect of cellular function, from catalyzing metabolic reactions to providing structural support. By elucidating the structures of proteins that were previously difficult to study, AlphaFold enhances our knowledge of how these molecules work and interact within the cell. This deeper understanding could lead to breakthroughs in areas such as synthetic biology, where researchers aim to engineer new biological systems for applications in medicine, agriculture, and industry.
Furthermore, AlphaFold’s success underscores the potential of artificial intelligence to address complex scientific challenges. The system’s ability to learn from vast amounts of data and make accurate predictions demonstrates the power of machine learning in advancing scientific research. As AI technologies continue to evolve, they are likely to play an increasingly important role in other areas of biology and beyond. This integration of AI into scientific research not only accelerates the pace of discovery but also democratizes access to cutting-edge tools, enabling researchers around the world to tackle pressing scientific questions.
Despite its remarkable achievements, AlphaFold is not without limitations. While it excels at predicting the structures of individual proteins, it is less effective at modeling protein complexes and dynamic conformational changes. These aspects are crucial for understanding how proteins function in their native environments, where they often interact with other molecules and undergo structural changes. Addressing these challenges will require further advancements in AI and computational methods, as well as continued collaboration between computational scientists and experimental biologists.
In conclusion, AlphaFold represents a significant leap forward in the field of structural biology, offering unprecedented insights into the architecture of proteins. Its ability to solve complex biological problems highlights the transformative potential of artificial intelligence in scientific research. As we continue to explore the capabilities of AI-driven technologies, the future of structural biology looks promising, with the potential for groundbreaking discoveries that could reshape our understanding of life at the molecular level.
AlphaFold’s Contribution to Personalized Medicine and Healthcare
AlphaFold, an artificial intelligence system developed by DeepMind, has made significant strides in the field of biology by accurately predicting protein structures. This breakthrough has profound implications for personalized medicine and healthcare, as it addresses a long-standing challenge in understanding the complex mechanisms of life. Proteins, the building blocks of life, perform a vast array of functions within organisms, and their specific functions are determined by their three-dimensional structures. Traditionally, determining these structures has been a laborious and time-consuming process, often taking years of experimental work. However, AlphaFold’s ability to predict protein structures with remarkable accuracy has revolutionized this aspect of biological research.
The implications of AlphaFold’s success extend far beyond the realm of academic research. In the context of personalized medicine, understanding protein structures is crucial for the development of targeted therapies. Personalized medicine aims to tailor medical treatment to the individual characteristics of each patient, and a deep understanding of protein structures can facilitate this by enabling the design of drugs that precisely interact with specific proteins. For instance, many diseases, including cancer and genetic disorders, are linked to malfunctioning proteins. By accurately predicting the structure of these proteins, AlphaFold can aid in the identification of potential drug targets, thereby accelerating the development of new treatments.
Moreover, AlphaFold’s contributions to healthcare are not limited to drug development. The system’s ability to predict protein structures can also enhance diagnostic processes. For example, certain diseases are characterized by the presence of specific protein markers. By understanding the structure of these markers, healthcare professionals can develop more accurate diagnostic tests, leading to earlier detection and improved patient outcomes. Furthermore, AlphaFold’s predictions can assist in understanding the mechanisms of diseases at a molecular level, providing insights that could lead to novel therapeutic approaches.
In addition to its direct applications in medicine, AlphaFold’s impact on healthcare is amplified by its potential to democratize access to protein structure information. Traditionally, the resources required to determine protein structures have been concentrated in well-funded laboratories, limiting access for researchers in less affluent regions. However, AlphaFold’s predictions are accessible to researchers worldwide, enabling a more equitable distribution of scientific knowledge. This democratization can foster collaboration and innovation, as researchers from diverse backgrounds contribute to the understanding of diseases and the development of treatments.
Furthermore, the integration of AlphaFold into healthcare systems could lead to more efficient use of resources. By reducing the time and cost associated with experimental determination of protein structures, AlphaFold allows researchers and healthcare providers to allocate resources more effectively. This efficiency can accelerate the pace of scientific discovery and the translation of research findings into clinical practice, ultimately benefiting patients.
In conclusion, AlphaFold’s ability to solve the complex problem of protein structure prediction has far-reaching implications for personalized medicine and healthcare. By facilitating the development of targeted therapies, enhancing diagnostic processes, and democratizing access to scientific knowledge, AlphaFold is poised to transform the way we understand and treat diseases. As the system continues to evolve and improve, its contributions to healthcare are likely to expand, offering new opportunities for innovation and collaboration in the pursuit of improved patient outcomes.
Q&A
1. **What is AlphaFold?**
AlphaFold is an artificial intelligence system developed by DeepMind that predicts protein structures with high accuracy.
2. **What major biological mystery did AlphaFold solve?**
AlphaFold solved the protein folding problem, which involves predicting a protein’s 3D structure from its amino acid sequence.
3. **Why is protein folding important?**
Protein folding is crucial because the 3D structure of a protein determines its function in biological processes, impacting areas like drug discovery and disease understanding.
4. **How accurate is AlphaFold’s prediction?**
AlphaFold’s predictions are highly accurate, often comparable to experimental methods, achieving a median Global Distance Test (GDT) score of around 92.4 in the CASP14 competition.
5. **What impact does AlphaFold have on scientific research?**
AlphaFold accelerates research by providing rapid and accurate protein structure predictions, aiding in understanding diseases, developing new treatments, and advancing biotechnology.
6. **What are the limitations of AlphaFold?**
While highly accurate, AlphaFold may struggle with predicting structures of proteins with complex interactions or those that require understanding of dynamic conformational changes.AlphaFold, developed by DeepMind, represents a groundbreaking advancement in computational biology by accurately predicting protein structures from amino acid sequences. This achievement addresses a long-standing challenge in biology, often referred to as the “protein folding problem,” which has puzzled scientists for decades. By leveraging deep learning techniques, AlphaFold can predict the three-dimensional shapes of proteins with remarkable accuracy, significantly accelerating research in fields such as drug discovery, disease understanding, and bioengineering. The implications of AlphaFold’s success are profound, offering the potential to revolutionize our understanding of biological processes and enabling new innovations in medicine and biotechnology.
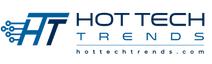