Recent advancements in artificial intelligence have led to the development of a groundbreaking AI model capable of predicting the stages of breast tumors and assessing their risk of progressing to invasive cancer. This innovative model leverages vast datasets and sophisticated algorithms to analyze tumor characteristics with unprecedented accuracy, offering a powerful tool for early detection and personalized treatment planning. By identifying high-risk cases at an early stage, this AI-driven approach holds the potential to significantly improve patient outcomes, reduce the burden of invasive procedures, and enhance the overall effectiveness of breast cancer management strategies.
Understanding AI’s Role in Predicting Breast Tumor Stages
In recent years, the integration of artificial intelligence (AI) into the medical field has opened new avenues for enhancing diagnostic accuracy and patient care. One of the most promising applications of AI is in the prediction of breast tumor stages, particularly in assessing the risk of tumors becoming invasive cancer. This advancement is crucial, as early detection and accurate staging of breast tumors can significantly influence treatment decisions and patient outcomes. By leveraging sophisticated algorithms and vast datasets, AI models are now capable of analyzing complex patterns in medical imaging and histopathological data, offering insights that were previously unattainable through traditional methods.
The process begins with the collection of extensive data from mammograms, biopsies, and other diagnostic tools. AI models are trained on this data to recognize subtle differences between benign, pre-invasive, and invasive breast tumors. Through machine learning techniques, these models learn to identify specific markers and patterns that may indicate a higher likelihood of a tumor progressing to an invasive stage. This capability is particularly beneficial in cases where human interpretation might be challenging due to the nuanced nature of the data.
Moreover, AI’s ability to process and analyze large volumes of data quickly and accurately provides a significant advantage over conventional diagnostic methods. Traditional approaches often rely on the expertise of pathologists, who may face limitations in terms of time and the sheer volume of cases. In contrast, AI models can rapidly evaluate numerous cases, providing consistent and objective assessments. This not only aids in reducing the workload of healthcare professionals but also minimizes the risk of human error, thereby enhancing the overall reliability of diagnoses.
Furthermore, the predictive power of AI models extends beyond mere identification. By analyzing historical data and outcomes, these models can offer prognostic insights, helping clinicians to stratify patients based on their risk levels. This stratification is essential for tailoring treatment plans to individual patients, ensuring that those at higher risk receive more aggressive interventions, while those at lower risk can avoid unnecessary procedures. Consequently, AI-driven predictions can lead to more personalized and effective treatment strategies, ultimately improving patient survival rates and quality of life.
Despite these advancements, it is important to acknowledge the challenges and limitations associated with AI in medical diagnostics. One significant concern is the need for high-quality, diverse datasets to train AI models effectively. Biases in data can lead to skewed predictions, which may adversely affect patient care. Therefore, ongoing efforts to ensure the inclusivity and representativeness of training datasets are crucial. Additionally, the integration of AI into clinical practice requires careful consideration of ethical and regulatory issues, including patient privacy and the transparency of AI decision-making processes.
In conclusion, the role of AI in predicting breast tumor stages represents a transformative development in oncology. By enhancing the accuracy and efficiency of diagnoses, AI models hold the potential to revolutionize the way breast cancer is detected and treated. As research and technology continue to evolve, the collaboration between AI and healthcare professionals will be pivotal in realizing the full potential of these innovations. Ultimately, the goal is to provide patients with the best possible care, leveraging the power of AI to improve outcomes and save lives.
How AI Models Enhance Early Detection of Invasive Cancer
In recent years, the integration of artificial intelligence (AI) into the medical field has revolutionized the way healthcare professionals approach diagnosis and treatment. One of the most promising applications of AI is in the early detection of invasive cancer, particularly breast cancer. Breast cancer remains one of the most common cancers affecting women worldwide, and early detection is crucial for improving survival rates. AI models have shown significant potential in predicting the stages of breast tumors that are at risk of becoming invasive, thereby enhancing early detection and intervention strategies.
The development of AI models for this purpose involves training algorithms on vast datasets of medical images and patient records. These datasets include mammograms, histopathological images, and clinical data, which provide a comprehensive view of the tumor’s characteristics. By analyzing these data, AI models can identify patterns and features that may not be immediately apparent to human observers. This capability allows AI to predict with remarkable accuracy which tumors are likely to progress to invasive cancer.
One of the key advantages of using AI in this context is its ability to process and analyze large volumes of data quickly and efficiently. Traditional methods of tumor analysis can be time-consuming and subject to human error. In contrast, AI models can rapidly assess numerous variables and provide a risk assessment that can guide clinical decision-making. This speed and accuracy are particularly beneficial in busy clinical settings, where timely decisions are critical.
Moreover, AI models can continuously learn and improve over time. As more data become available, these models can refine their predictions, leading to even greater accuracy in identifying high-risk tumors. This adaptability ensures that AI remains a valuable tool in the ever-evolving landscape of cancer diagnosis and treatment. Furthermore, AI’s ability to integrate new information means that it can stay up-to-date with the latest research findings and clinical guidelines, providing healthcare professionals with the most current insights.
In addition to improving diagnostic accuracy, AI models also have the potential to reduce the burden on healthcare systems. By accurately identifying tumors at risk of becoming invasive, AI can help prioritize patients who need immediate attention, thereby optimizing resource allocation. This prioritization can lead to more efficient use of medical resources and potentially reduce healthcare costs associated with late-stage cancer treatments.
Despite these promising developments, it is important to acknowledge the challenges and limitations associated with AI in cancer detection. Ensuring the quality and diversity of training data is crucial, as biased or incomplete datasets can lead to inaccurate predictions. Additionally, the integration of AI into clinical practice requires careful consideration of ethical and legal implications, particularly concerning patient privacy and data security.
In conclusion, AI models represent a significant advancement in the early detection of invasive breast cancer. By accurately predicting which tumors are at risk of becoming invasive, these models enhance the ability of healthcare professionals to make informed decisions and provide timely interventions. As AI technology continues to evolve, it holds the promise of further improving cancer diagnosis and treatment, ultimately leading to better patient outcomes. The ongoing collaboration between AI researchers, clinicians, and policymakers will be essential in realizing the full potential of AI in the fight against cancer.
The Impact of AI on Breast Cancer Prognosis
The advent of artificial intelligence (AI) in the medical field has ushered in a new era of diagnostic and prognostic capabilities, particularly in the realm of oncology. Among the most promising developments is the use of AI models to predict the stages of breast tumors that are at risk of progressing to invasive cancer. This innovation holds significant potential for improving patient outcomes by enabling more precise and timely interventions. As breast cancer remains one of the most prevalent cancers worldwide, the ability to accurately predict its progression is crucial for effective treatment planning and management.
AI models, through their ability to analyze vast datasets with remarkable speed and accuracy, have demonstrated a capacity to identify patterns and correlations that may elude human experts. In the context of breast cancer, these models are trained on extensive datasets comprising medical images, histopathological data, and patient records. By processing this information, AI can discern subtle indicators of tumor behavior that might predict a transition from a non-invasive to an invasive state. This capability is particularly valuable in cases of ductal carcinoma in situ (DCIS), a non-invasive form of breast cancer that can sometimes progress to invasive ductal carcinoma (IDC).
The integration of AI into breast cancer prognosis is not merely about enhancing diagnostic accuracy; it also involves refining the stratification of patients based on their risk profiles. By identifying which tumors are more likely to become invasive, clinicians can tailor treatment plans to the individual needs of patients. This personalized approach not only optimizes therapeutic outcomes but also minimizes unnecessary interventions for those with a lower risk of progression. Consequently, patients can avoid the physical and emotional burdens associated with overtreatment, while healthcare systems can allocate resources more efficiently.
Moreover, the predictive power of AI models extends beyond the immediate clinical benefits. By providing insights into the biological mechanisms underlying tumor progression, these models contribute to the broader understanding of cancer biology. This knowledge can inform the development of novel therapeutic strategies and preventive measures, ultimately advancing the fight against breast cancer. Furthermore, the continuous refinement of AI algorithms, driven by ongoing research and technological advancements, promises to enhance their predictive accuracy and expand their applicability to other cancer types.
Despite the promising potential of AI in breast cancer prognosis, it is essential to acknowledge the challenges and limitations that accompany its implementation. The quality and diversity of the data used to train AI models are critical factors that influence their performance. Ensuring that datasets are representative of diverse populations is crucial to avoid biases that could compromise the accuracy and fairness of predictions. Additionally, the integration of AI into clinical practice necessitates collaboration between technologists and healthcare professionals to ensure that these tools are user-friendly and seamlessly incorporated into existing workflows.
In conclusion, the use of AI models to predict the stages of breast tumors at risk of becoming invasive cancer represents a significant advancement in the field of oncology. By enhancing diagnostic precision and enabling personalized treatment strategies, AI has the potential to transform breast cancer prognosis and improve patient outcomes. As research and technology continue to evolve, the role of AI in healthcare is poised to expand, offering new opportunities to combat cancer and other complex diseases. However, careful consideration of ethical and practical challenges is essential to fully realize the benefits of this transformative technology.
AI Innovations in Identifying High-Risk Breast Tumors
In recent years, the field of artificial intelligence has made significant strides in healthcare, particularly in the early detection and treatment of cancer. One of the most promising developments is the use of AI models to predict the stages of breast tumors that are at risk of becoming invasive cancer. This innovation holds the potential to revolutionize the way clinicians approach breast cancer diagnosis and treatment, offering a more precise and personalized strategy for managing the disease.
Breast cancer remains one of the most common cancers affecting women worldwide, and early detection is crucial for improving survival rates. Traditionally, the assessment of breast tumors has relied heavily on histopathological examination, where pathologists analyze tissue samples under a microscope to determine the stage and aggressiveness of the cancer. However, this method can be subjective and time-consuming, often leading to variability in diagnosis. In contrast, AI models can process vast amounts of data quickly and consistently, providing a more objective analysis.
The development of AI models for predicting breast tumor stages involves training algorithms on large datasets of medical images and patient records. These models learn to recognize patterns and features that are indicative of different stages of tumor development. By analyzing factors such as tumor size, shape, and cellular characteristics, AI can predict which tumors are likely to progress to invasive cancer. This capability is particularly valuable for identifying high-risk tumors that may not yet exhibit overt signs of invasiveness but have the potential to become more aggressive.
One of the key advantages of using AI in this context is its ability to integrate and analyze diverse data sources. For instance, AI models can combine imaging data with genomic information, providing a more comprehensive view of the tumor’s biology. This holistic approach enables the identification of molecular markers that are associated with tumor progression, offering insights into the underlying mechanisms driving cancer development. Consequently, clinicians can make more informed decisions about treatment options, tailoring interventions to the specific characteristics of each patient’s tumor.
Moreover, AI models can enhance the efficiency of clinical workflows by automating routine tasks and reducing the burden on healthcare professionals. For example, AI can pre-screen mammograms and flag suspicious areas for further review, allowing radiologists to focus their attention on cases that require more detailed analysis. This not only speeds up the diagnostic process but also helps to minimize the risk of human error, ultimately improving the accuracy of breast cancer diagnoses.
Despite these promising developments, the integration of AI into clinical practice is not without challenges. Ensuring the reliability and generalizability of AI models requires rigorous validation and testing across diverse patient populations. Additionally, ethical considerations, such as data privacy and the potential for algorithmic bias, must be addressed to build trust in AI-driven healthcare solutions. Nevertheless, ongoing research and collaboration between AI developers, clinicians, and regulatory bodies are paving the way for the safe and effective implementation of these technologies.
In conclusion, AI models represent a significant advancement in the identification of high-risk breast tumors, offering a powerful tool for predicting the stages at which tumors may become invasive. By providing a more accurate and personalized approach to breast cancer diagnosis and treatment, AI has the potential to improve patient outcomes and transform the landscape of cancer care. As research continues to evolve, the integration of AI into clinical practice promises to enhance our ability to combat this pervasive disease.
The Future of Cancer Treatment: AI-Driven Predictions
In recent years, the integration of artificial intelligence (AI) into the medical field has opened new avenues for enhancing diagnostic accuracy and treatment efficacy. One of the most promising developments in this domain is the use of AI models to predict the stages of breast tumors that are at risk of becoming invasive cancer. This advancement holds significant potential for transforming cancer treatment, offering a more personalized and proactive approach to patient care.
Breast cancer remains one of the most prevalent forms of cancer worldwide, and early detection is crucial for improving patient outcomes. Traditionally, the assessment of breast tumors involves a combination of imaging techniques, biopsies, and histopathological evaluations. However, these methods can sometimes be subjective and may not always accurately predict the progression of the disease. This is where AI-driven predictions come into play, providing a more objective and data-driven approach to understanding tumor behavior.
AI models, particularly those based on machine learning algorithms, have demonstrated remarkable capabilities in analyzing vast amounts of medical data. By training these models on large datasets of breast cancer cases, researchers can identify patterns and correlations that may not be immediately apparent to human clinicians. These models can then predict which tumors are likely to progress to invasive cancer, allowing for earlier intervention and tailored treatment plans.
One of the key advantages of AI in this context is its ability to process and analyze complex datasets with high precision. For instance, AI models can evaluate imaging data, such as mammograms and MRIs, alongside genetic and molecular information to provide a comprehensive assessment of a tumor’s characteristics. This multi-faceted analysis enables the identification of subtle indicators of malignancy that might be overlooked in traditional evaluations.
Moreover, AI-driven predictions can enhance the decision-making process for oncologists. By providing a probabilistic assessment of a tumor’s potential to become invasive, AI models can inform treatment strategies, such as the need for surgery, chemotherapy, or radiation therapy. This not only helps in optimizing treatment plans but also reduces the risk of overtreatment, which can have significant side effects and impact a patient’s quality of life.
The implementation of AI in predicting breast tumor stages also underscores the importance of interdisciplinary collaboration. The development and refinement of these models require the expertise of data scientists, oncologists, radiologists, and pathologists working together to ensure that the AI systems are both accurate and clinically relevant. This collaborative approach is essential for translating AI innovations from research settings to real-world clinical applications.
Despite the promising potential of AI in cancer treatment, there are challenges that need to be addressed. Ensuring the ethical use of AI, maintaining patient privacy, and overcoming biases in training data are critical considerations. Additionally, the integration of AI into existing healthcare systems requires careful planning and resource allocation to ensure that these technologies are accessible and beneficial to all patients.
In conclusion, AI-driven predictions represent a significant leap forward in the future of cancer treatment. By accurately predicting the stages of breast tumors at risk of becoming invasive, AI models offer the potential for more personalized and effective treatment strategies. As research and technology continue to advance, the role of AI in oncology is poised to expand, ultimately improving patient outcomes and transforming the landscape of cancer care.
Challenges and Opportunities in AI-Based Cancer Diagnosis
The advent of artificial intelligence (AI) in the medical field has ushered in a new era of diagnostic capabilities, particularly in the realm of oncology. One of the most promising developments is the use of AI models to predict the stages of breast tumors that are at risk of becoming invasive cancer. This innovation presents both significant challenges and remarkable opportunities in AI-based cancer diagnosis, offering a glimpse into the future of personalized medicine.
AI models, trained on vast datasets of medical images and patient histories, have demonstrated an impressive ability to identify patterns that may elude even the most experienced clinicians. By analyzing mammograms and other diagnostic images, these models can assess the likelihood of a breast tumor progressing to an invasive stage. This capability is crucial, as early detection and intervention are key to improving patient outcomes. The ability to predict tumor behavior with high accuracy could lead to more tailored treatment plans, potentially sparing patients from aggressive therapies that may not be necessary.
However, the integration of AI into cancer diagnosis is not without its challenges. One of the primary concerns is the quality and diversity of the data used to train these models. AI systems rely heavily on the data they are fed, and any biases present in the training data can lead to skewed results. For instance, if the dataset lacks diversity in terms of patient demographics, the AI model may not perform as well across different populations. This issue underscores the importance of using comprehensive and representative datasets to ensure that AI tools are equitable and effective for all patients.
Moreover, the interpretability of AI models remains a significant hurdle. While these systems can provide predictions with remarkable accuracy, understanding the rationale behind their decisions is often challenging. This “black box” nature of AI can be problematic in a clinical setting, where transparency and trust are paramount. Clinicians need to understand how an AI model arrived at a particular conclusion to make informed decisions about patient care. Therefore, developing AI models that offer not only accurate predictions but also clear explanations is essential for their successful adoption in healthcare.
Despite these challenges, the opportunities presented by AI in cancer diagnosis are immense. The potential for AI to enhance early detection and improve prognostic assessments could revolutionize the way breast cancer is managed. By identifying tumors at risk of becoming invasive, AI models can help prioritize patients for more intensive monitoring or intervention, ultimately leading to better outcomes. Furthermore, the integration of AI into diagnostic workflows can alleviate some of the burdens on healthcare professionals, allowing them to focus on more complex cases and patient interactions.
In addition to improving diagnostic accuracy, AI has the potential to democratize access to high-quality cancer care. In regions where access to experienced oncologists is limited, AI tools can serve as valuable decision-support systems, providing insights that might otherwise be unavailable. This democratization could lead to more equitable healthcare outcomes, reducing disparities in cancer diagnosis and treatment.
In conclusion, while the use of AI models to predict breast tumor stages at risk of becoming invasive cancer presents several challenges, the opportunities it offers are transformative. By addressing issues related to data quality, model interpretability, and equitable access, the medical community can harness the full potential of AI to improve cancer diagnosis and patient care. As research and development in this field continue to advance, the integration of AI into oncology promises to be a pivotal step toward more personalized and effective cancer treatment strategies.
Q&A
1. **What is the primary goal of the AI model in predicting breast tumor stages?**
The primary goal of the AI model is to accurately predict which breast tumors are at risk of becoming invasive cancer, thereby aiding in early intervention and treatment planning.
2. **How does the AI model improve the diagnosis process for breast cancer?**
The AI model enhances the diagnosis process by providing more precise risk assessments, reducing the likelihood of over-treatment or under-treatment, and assisting pathologists in identifying high-risk cases more efficiently.
3. **What type of data is used to train the AI model for predicting breast tumor stages?**
The AI model is typically trained using a combination of histopathological images, patient medical records, and genomic data to ensure comprehensive analysis and accurate predictions.
4. **What are the potential benefits of using AI in predicting breast tumor invasiveness?**
Potential benefits include improved patient outcomes through personalized treatment plans, reduced healthcare costs by avoiding unnecessary procedures, and increased accuracy in identifying tumors that require aggressive treatment.
5. **What challenges might arise in implementing AI models for breast cancer prediction in clinical settings?**
Challenges include ensuring data privacy and security, integrating AI tools with existing medical systems, obtaining regulatory approvals, and addressing potential biases in the AI model’s predictions.
6. **How does the AI model’s prediction accuracy compare to traditional methods?**
The AI model generally offers higher prediction accuracy compared to traditional methods, as it can analyze complex patterns in large datasets that may not be easily discernible by human experts alone.The development of an AI model capable of predicting the stages of breast tumors at risk of becoming invasive cancer represents a significant advancement in oncology and personalized medicine. By accurately identifying which tumors are likely to progress to invasive cancer, this technology can enhance early intervention strategies, improve patient outcomes, and optimize treatment plans. The model’s predictive capabilities could lead to more targeted surveillance and therapeutic approaches, reducing unnecessary treatments for low-risk cases while focusing resources on high-risk patients. Overall, this innovation holds promise for transforming breast cancer management and improving survival rates through precise and timely medical interventions.
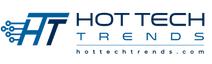