The Advanced Alert System for Emerging AI Threats is a cutting-edge framework designed to proactively identify, assess, and mitigate potential risks associated with the rapid development and deployment of artificial intelligence technologies. As AI systems become increasingly integrated into critical sectors such as finance, healthcare, and national security, the need for robust monitoring and response mechanisms has never been more urgent. This system leverages state-of-the-art machine learning algorithms and real-time data analytics to detect anomalies and potential threats, ensuring that stakeholders are equipped with timely and actionable intelligence. By fostering collaboration between industry leaders, policymakers, and cybersecurity experts, the Advanced Alert System aims to safeguard technological advancements while promoting responsible AI innovation.
Understanding The Need For Advanced Alert Systems In AI Security
In the rapidly evolving landscape of artificial intelligence, the need for robust security measures has become increasingly apparent. As AI systems become more sophisticated, they also become more susceptible to a range of threats that can compromise their integrity and functionality. This growing concern underscores the necessity for advanced alert systems specifically designed to identify and mitigate emerging AI threats. Such systems are crucial in maintaining the reliability and trustworthiness of AI technologies, which are now integral to various sectors, including healthcare, finance, and national security.
To understand the importance of advanced alert systems in AI security, it is essential to first recognize the nature of the threats these systems face. AI technologies are vulnerable to a variety of attacks, such as data poisoning, adversarial attacks, and model inversion. Data poisoning involves the manipulation of training data to corrupt the learning process, leading to inaccurate or biased outcomes. Adversarial attacks, on the other hand, involve the introduction of subtle perturbations to input data, causing AI models to make incorrect predictions. Model inversion attacks aim to extract sensitive information from AI models, posing significant privacy risks. These threats highlight the complexity and sophistication of potential attacks, necessitating equally sophisticated defense mechanisms.
Advanced alert systems serve as a critical line of defense by providing real-time monitoring and detection of unusual activities that may indicate a security breach. By leveraging machine learning algorithms and anomaly detection techniques, these systems can identify patterns and behaviors that deviate from the norm, thereby flagging potential threats before they can cause significant harm. Moreover, the integration of advanced alert systems with existing cybersecurity frameworks enhances the overall resilience of AI systems, ensuring that they can withstand and recover from attacks more effectively.
Furthermore, the implementation of advanced alert systems is not only about detecting threats but also about understanding and responding to them in a timely manner. These systems are designed to provide actionable insights that enable organizations to take proactive measures in mitigating risks. For instance, upon detecting an anomaly, an alert system can trigger automated responses, such as isolating affected components or initiating a comprehensive security audit. This capability is particularly valuable in high-stakes environments where rapid response is crucial to minimizing damage and maintaining operational continuity.
In addition to their technical capabilities, advanced alert systems also play a pivotal role in fostering a culture of security awareness within organizations. By continuously monitoring AI systems and providing regular updates on potential threats, these systems help to keep security at the forefront of organizational priorities. This heightened awareness encourages stakeholders to remain vigilant and proactive in addressing security challenges, ultimately contributing to a more secure AI ecosystem.
As AI technologies continue to advance and permeate various aspects of society, the development and deployment of advanced alert systems will become increasingly vital. These systems not only protect AI systems from emerging threats but also ensure that the benefits of AI can be realized without compromising security and privacy. In conclusion, the integration of advanced alert systems into AI security frameworks represents a critical step forward in safeguarding the future of artificial intelligence, enabling it to thrive in a secure and trustworthy manner.
Key Features Of An Effective AI Threat Alert System
An effective AI threat alert system is crucial in today’s rapidly evolving technological landscape, where artificial intelligence continues to advance at an unprecedented pace. As AI systems become more sophisticated, the potential for misuse and unintended consequences grows, necessitating robust mechanisms to detect and mitigate emerging threats. One of the key features of an effective AI threat alert system is its ability to provide real-time monitoring and analysis. This involves continuously scanning for anomalies and deviations from expected behavior in AI systems, which can indicate potential threats. By leveraging advanced algorithms and machine learning techniques, the system can identify patterns and trends that may signify malicious activities or vulnerabilities, allowing for timely intervention.
In addition to real-time monitoring, an effective alert system must incorporate comprehensive threat intelligence. This involves gathering and analyzing data from a wide range of sources, including cybersecurity reports, academic research, and industry insights. By synthesizing this information, the system can develop a nuanced understanding of the threat landscape, enabling it to anticipate and respond to emerging risks more effectively. Furthermore, integrating threat intelligence with real-time monitoring enhances the system’s ability to differentiate between benign anomalies and genuine threats, reducing the likelihood of false positives and ensuring that resources are allocated efficiently.
Another critical feature of an advanced AI threat alert system is its adaptability. As AI technologies evolve, so too do the tactics and techniques employed by malicious actors. Therefore, the alert system must be capable of evolving in tandem, updating its algorithms and threat models to address new challenges. This adaptability can be achieved through continuous learning mechanisms, where the system refines its understanding of threats based on new data and experiences. By maintaining a dynamic and flexible approach, the alert system can remain effective in the face of an ever-changing threat landscape.
Moreover, an effective AI threat alert system should prioritize transparency and explainability. As AI systems become more complex, understanding the rationale behind their decisions becomes increasingly challenging. However, for an alert system to be trusted and relied upon, it must provide clear and comprehensible explanations for its alerts and recommendations. This transparency not only facilitates informed decision-making by human operators but also aids in the identification and rectification of any biases or errors within the system itself. By fostering trust and accountability, transparency ensures that the alert system can be effectively integrated into broader security frameworks.
Furthermore, collaboration and communication are essential components of an effective AI threat alert system. Given the global and interconnected nature of AI technologies, no single entity can address all potential threats in isolation. Therefore, the alert system should facilitate information sharing and collaboration among stakeholders, including governments, industry leaders, and academic institutions. By fostering a collaborative environment, the system can leverage diverse perspectives and expertise, enhancing its ability to identify and mitigate threats. Additionally, effective communication ensures that alerts and recommendations are conveyed clearly and promptly, enabling swift and coordinated responses to emerging risks.
In conclusion, an effective AI threat alert system is characterized by its real-time monitoring capabilities, comprehensive threat intelligence, adaptability, transparency, and collaborative approach. By integrating these key features, the system can provide a robust defense against the myriad threats posed by advanced AI technologies. As AI continues to shape the future, investing in such alert systems will be essential to safeguarding both technological advancements and societal well-being.
Integrating Machine Learning In AI Threat Detection
In the rapidly evolving landscape of artificial intelligence, the integration of machine learning into AI threat detection systems has become increasingly crucial. As AI technologies advance, so do the potential threats they pose, necessitating sophisticated mechanisms to identify and mitigate these risks. Machine learning, with its ability to analyze vast amounts of data and recognize patterns, offers a promising solution for enhancing the capabilities of AI threat detection systems.
To begin with, the integration of machine learning into AI threat detection involves the development of algorithms that can process and analyze data in real-time. These algorithms are designed to identify anomalies and potential threats by learning from historical data and adapting to new information. By continuously updating their knowledge base, machine learning models can detect emerging threats that may not have been previously identified. This dynamic learning process is essential in a field where new threats can arise rapidly and unpredictably.
Moreover, machine learning enhances the precision of threat detection systems by reducing false positives and negatives. Traditional systems often struggle with accurately distinguishing between benign and malicious activities, leading to either unnecessary alerts or missed threats. Machine learning models, however, can be trained to recognize subtle patterns and correlations that may indicate a genuine threat. This improved accuracy not only enhances security but also reduces the burden on human analysts, allowing them to focus on more complex tasks.
In addition to improving accuracy, machine learning facilitates the automation of threat detection processes. Automation is particularly valuable in managing the sheer volume of data generated by AI systems. By automating routine tasks, machine learning allows for faster response times and more efficient resource allocation. This is especially important in environments where time is of the essence, such as in cybersecurity or critical infrastructure protection.
Furthermore, the integration of machine learning into AI threat detection systems supports the development of predictive models. These models can anticipate potential threats before they materialize, providing organizations with the opportunity to implement proactive measures. Predictive analytics, powered by machine learning, enables organizations to stay one step ahead of potential adversaries, thereby enhancing their overall security posture.
However, the integration of machine learning into AI threat detection is not without its challenges. One significant concern is the quality and diversity of the data used to train machine learning models. Inadequate or biased data can lead to inaccurate predictions and potentially overlook certain types of threats. Therefore, it is imperative to ensure that the data used is comprehensive and representative of the diverse range of potential threats.
Additionally, there is the challenge of maintaining transparency and interpretability in machine learning models. As these models become more complex, understanding how they arrive at specific conclusions can become difficult. This lack of transparency can hinder trust and accountability, particularly in high-stakes environments. Consequently, ongoing research is focused on developing methods to enhance the interpretability of machine learning models without compromising their effectiveness.
In conclusion, the integration of machine learning into AI threat detection systems represents a significant advancement in the field of security. By enhancing accuracy, enabling automation, and supporting predictive analytics, machine learning offers a robust framework for identifying and mitigating emerging AI threats. Despite the challenges associated with data quality and model transparency, the potential benefits of this integration are substantial, paving the way for more secure and resilient AI systems. As AI technologies continue to evolve, the role of machine learning in threat detection will undoubtedly become even more critical, underscoring the need for continued innovation and collaboration in this domain.
Case Studies: Successful Implementation Of AI Alert Systems
In recent years, the rapid advancement of artificial intelligence has brought about significant benefits across various sectors, from healthcare to finance. However, alongside these benefits, there has been a growing concern about the potential threats posed by AI systems, particularly those that are autonomous and capable of making decisions without human intervention. To address these concerns, several organizations have successfully implemented advanced alert systems designed to detect and mitigate emerging AI threats. These case studies provide valuable insights into the effectiveness of such systems and highlight the importance of proactive measures in safeguarding against potential risks.
One notable example of a successful implementation is the AI alert system developed by a leading financial institution. This system was designed to monitor and analyze vast amounts of transaction data in real-time, identifying patterns that could indicate fraudulent activities or other financial threats. By leveraging machine learning algorithms, the system was able to detect anomalies that would have been difficult for human analysts to identify. As a result, the institution was able to respond swiftly to potential threats, minimizing financial losses and enhancing the security of its operations. This case underscores the critical role that AI alert systems can play in the financial sector, where the speed and accuracy of threat detection are paramount.
Transitioning to another sector, the healthcare industry has also seen the successful deployment of AI alert systems, particularly in the realm of patient safety. A prominent hospital network implemented an AI-driven alert system to monitor patient data and predict potential adverse events, such as medication errors or sudden health deteriorations. By integrating this system with electronic health records, healthcare providers were able to receive timely alerts, allowing them to intervene before minor issues escalated into serious complications. This proactive approach not only improved patient outcomes but also reduced the burden on healthcare staff, demonstrating the transformative potential of AI in enhancing patient care.
Moreover, the implementation of AI alert systems has extended to the realm of cybersecurity, where the stakes are particularly high. A major technology company developed an advanced alert system to protect its infrastructure from cyber threats. This system utilized AI to continuously analyze network traffic and identify suspicious activities that could indicate a breach. By employing sophisticated algorithms, the system was able to adapt to new threats and provide real-time alerts to the security team. Consequently, the company was able to fortify its defenses and maintain the integrity of its data, highlighting the indispensable role of AI in modern cybersecurity strategies.
In addition to these sector-specific implementations, there are also cross-industry applications of AI alert systems that have proven successful. For instance, a multinational corporation adopted an AI-driven alert system to monitor compliance with regulatory standards across its global operations. This system was capable of analyzing vast amounts of data from various sources, ensuring that the company adhered to complex and ever-changing regulations. By providing timely alerts about potential compliance issues, the system enabled the corporation to address concerns proactively, thereby avoiding costly penalties and reputational damage.
In conclusion, these case studies illustrate the successful implementation of advanced alert systems for emerging AI threats across diverse sectors. By leveraging the power of AI, organizations can enhance their ability to detect and respond to potential risks, thereby safeguarding their operations and stakeholders. As AI continues to evolve, the development and deployment of such alert systems will become increasingly vital in ensuring that the benefits of AI are realized while minimizing its associated risks.
Future Trends In AI Threat Alert Technologies
As artificial intelligence continues to evolve at an unprecedented pace, the potential for emerging threats has become a significant concern for researchers, policymakers, and industry leaders alike. The development of an advanced alert system for AI threats is not only a proactive measure but also a necessary one to ensure the safe and ethical deployment of AI technologies. This system aims to identify, assess, and mitigate risks associated with AI, thereby safeguarding both technological advancements and societal well-being.
To begin with, the complexity of AI systems has increased the difficulty of predicting and managing potential threats. As AI becomes more integrated into critical infrastructure, from healthcare to finance, the stakes are higher than ever. An advanced alert system would serve as an early warning mechanism, capable of detecting anomalies and potential threats before they manifest into significant issues. This proactive approach is essential in a landscape where the speed of AI development often outpaces regulatory and ethical considerations.
Moreover, the integration of machine learning and data analytics into the alert system enhances its ability to process vast amounts of data in real-time. By leveraging these technologies, the system can identify patterns and trends that may indicate emerging threats. For instance, unusual data access patterns or deviations in AI behavior could trigger alerts, prompting further investigation. This capability is crucial in a world where cyber threats are becoming increasingly sophisticated and difficult to detect using traditional methods.
In addition to technological advancements, collaboration between various stakeholders is vital for the success of an advanced alert system. Governments, private sector companies, and academic institutions must work together to share information and resources. This collaborative effort ensures that the alert system is comprehensive and up-to-date, reflecting the latest developments in AI technology and threat landscapes. Furthermore, international cooperation is essential, as AI threats often transcend national borders, requiring a coordinated global response.
Transitioning from the technical aspects, it is also important to consider the ethical implications of an advanced alert system. Ensuring that the system respects privacy and civil liberties is paramount. This involves implementing robust data protection measures and establishing clear guidelines on how data is collected, stored, and used. Transparency in the system’s operations can help build public trust, which is crucial for its acceptance and effectiveness.
Furthermore, the development of an advanced alert system should be accompanied by comprehensive training and education programs. These initiatives would equip stakeholders with the necessary skills and knowledge to effectively utilize the system and respond to alerts. By fostering a culture of awareness and preparedness, organizations can better manage AI threats and minimize potential impacts.
Looking ahead, the continuous evolution of AI technologies will undoubtedly present new challenges and opportunities. An advanced alert system must be adaptable, capable of evolving alongside AI advancements to remain effective. This requires ongoing research and development, as well as a commitment to innovation and improvement.
In conclusion, the establishment of an advanced alert system for emerging AI threats is a critical step towards ensuring the safe and responsible use of AI technologies. By combining technological innovation with collaboration and ethical considerations, such a system can provide a robust defense against potential threats. As we navigate the complexities of the AI-driven future, proactive measures like these will be essential in safeguarding both technological progress and societal interests.
Challenges And Solutions In Developing AI Threat Alert Systems
The development of advanced alert systems for emerging AI threats presents a complex array of challenges and solutions, reflecting the intricate nature of artificial intelligence itself. As AI technologies continue to evolve at a rapid pace, the potential for misuse or unintended consequences grows, necessitating robust mechanisms to detect and mitigate these threats. One of the primary challenges in developing such systems is the sheer diversity of AI applications, each with its own set of vulnerabilities and potential risks. From autonomous vehicles to financial algorithms, the scope of AI’s influence is vast, requiring alert systems to be highly adaptable and capable of addressing a wide range of scenarios.
Moreover, the dynamic nature of AI technologies means that threat landscapes are constantly shifting. This necessitates alert systems that are not only reactive but also proactive, capable of anticipating potential threats before they fully materialize. To achieve this, developers must integrate advanced machine learning techniques that can analyze patterns and predict future risks. However, this introduces another layer of complexity, as these systems must be trained on vast datasets to ensure accuracy, raising concerns about data privacy and security.
In addition to technical challenges, there are significant ethical considerations in the development of AI threat alert systems. Ensuring that these systems operate transparently and without bias is crucial, as any perceived unfairness could undermine public trust. This requires a careful balance between automation and human oversight, where AI systems are designed to assist human decision-makers rather than replace them entirely. By maintaining this balance, developers can ensure that alert systems are both effective and accountable.
Collaboration across various sectors is also essential in addressing the challenges associated with AI threat alert systems. Governments, private companies, and academic institutions must work together to establish standards and best practices that guide the development and deployment of these systems. Such collaboration can facilitate the sharing of information and resources, enabling more comprehensive and coordinated responses to emerging threats. Furthermore, international cooperation is vital, as AI threats often transcend national borders, requiring a unified global approach.
Despite these challenges, there are promising solutions on the horizon. Advances in AI explainability are helping to demystify complex algorithms, making it easier for developers to identify potential vulnerabilities and address them proactively. Additionally, the integration of blockchain technology offers a potential solution for enhancing the security and integrity of AI systems, providing a tamper-proof record of data and transactions that can be used to verify the authenticity of alerts.
Furthermore, the development of AI threat alert systems can benefit from the incorporation of feedback loops, where the system continuously learns from past incidents to improve its accuracy and responsiveness. By analyzing the outcomes of previous alerts, these systems can refine their algorithms and better anticipate future threats, creating a cycle of continuous improvement.
In conclusion, while the development of advanced alert systems for emerging AI threats presents numerous challenges, it also offers opportunities for innovation and collaboration. By leveraging cutting-edge technologies and fostering cooperation across sectors, developers can create robust systems that effectively safeguard against the risks associated with AI. As these systems continue to evolve, they will play a crucial role in ensuring the safe and responsible deployment of artificial intelligence, ultimately contributing to a more secure and resilient digital landscape.
Q&A
1. **What is the purpose of an Advanced Alert System for Emerging AI Threats?**
The purpose is to detect, monitor, and alert stakeholders about potential threats and risks associated with emerging AI technologies, ensuring timely responses to mitigate harm.
2. **How does the system identify emerging AI threats?**
It uses a combination of machine learning algorithms, threat intelligence feeds, and expert analysis to identify patterns and anomalies that may indicate emerging AI threats.
3. **What types of threats does the system monitor?**
The system monitors threats such as AI-driven cyberattacks, misuse of AI technologies, ethical violations, and unintended consequences of AI deployment.
4. **Who are the primary users of this alert system?**
Primary users include cybersecurity professionals, AI researchers, policymakers, and organizations deploying AI technologies.
5. **What are the key components of the alert system?**
Key components include data collection modules, threat analysis engines, alert dissemination channels, and a user interface for monitoring and response.
6. **How does the system ensure timely alerts?**
The system employs real-time data processing and automated alert mechanisms to ensure that stakeholders receive timely notifications about potential threats.The Advanced Alert System for Emerging AI Threats is a critical framework designed to proactively identify, assess, and mitigate potential risks associated with the rapid development and deployment of artificial intelligence technologies. By integrating real-time monitoring, threat intelligence, and adaptive response strategies, this system aims to safeguard against malicious AI activities, unintended consequences, and ethical violations. It emphasizes collaboration among stakeholders, including governments, industry leaders, and researchers, to ensure a comprehensive and coordinated approach to AI threat management. Ultimately, the system seeks to enhance the resilience and security of AI ecosystems, fostering trust and enabling the responsible advancement of AI technologies.
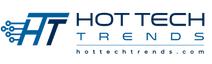