In the rapidly evolving landscape of technology, AI-driven data centers are emerging as pivotal components in the quest for enhanced efficiency and innovation. These advanced facilities leverage artificial intelligence to optimize operations, reduce energy consumption, and improve overall performance. However, despite their potential, many data centers remain shackled by operational inertia, a resistance to change that hinders the full realization of AI’s transformative capabilities. Accelerating the adoption of AI-driven solutions in data centers requires a strategic approach to overcome these barriers, fostering an environment where agility and adaptability are prioritized. By breaking free from this inertia, organizations can unlock unprecedented levels of operational excellence, driving forward the next wave of technological advancement.
Understanding Operational Inertia in AI-Driven Data Centers
In the rapidly evolving landscape of technology, AI-driven data centers have emerged as pivotal components in the digital infrastructure, offering unprecedented capabilities in data processing and management. However, despite their potential, these data centers often face a significant challenge: operational inertia. This phenomenon, characterized by the resistance to change and adaptation within organizational processes, can impede the full realization of AI’s transformative power. Understanding operational inertia in AI-driven data centers is crucial for organizations aiming to harness the full potential of artificial intelligence.
Operational inertia in AI-driven data centers can be attributed to several factors, including legacy systems, entrenched workflows, and cultural resistance to change. Legacy systems, often deeply embedded within an organization’s infrastructure, can be particularly challenging to overhaul. These systems, while reliable, may not be optimized for the dynamic and scalable demands of AI technologies. Consequently, they can act as a bottleneck, limiting the efficiency and responsiveness of data centers. Transitioning from these legacy systems to more agile and AI-compatible solutions requires not only technological upgrades but also a strategic vision that aligns with the organization’s long-term goals.
Moreover, entrenched workflows within data centers contribute significantly to operational inertia. These workflows, developed over years of practice, are often resistant to change due to their perceived reliability and the comfort they provide to employees. However, in the context of AI-driven data centers, such rigidity can stifle innovation and adaptability. To overcome this, organizations must foster a culture of continuous improvement and flexibility, encouraging employees to embrace new methodologies and technologies. This cultural shift is essential for breaking free from the constraints of operational inertia and enabling data centers to evolve in tandem with technological advancements.
Cultural resistance to change is another critical aspect of operational inertia. Employees accustomed to traditional processes may be hesitant to adopt AI-driven solutions, fearing job displacement or the complexity of new systems. Addressing these concerns requires transparent communication and comprehensive training programs that emphasize the benefits of AI integration. By demonstrating how AI can augment human capabilities rather than replace them, organizations can alleviate fears and build a workforce that is both skilled and confident in utilizing AI technologies.
Furthermore, the integration of AI into data centers necessitates a reevaluation of existing security protocols. As AI systems process vast amounts of sensitive data, ensuring robust cybersecurity measures becomes paramount. Operational inertia can hinder the implementation of these necessary security upgrades, leaving data centers vulnerable to potential threats. Therefore, organizations must prioritize cybersecurity as an integral component of their AI strategy, ensuring that data protection measures evolve in parallel with technological advancements.
In conclusion, overcoming operational inertia in AI-driven data centers is essential for organizations seeking to leverage the full potential of artificial intelligence. By addressing the challenges posed by legacy systems, entrenched workflows, and cultural resistance, organizations can create a more agile and responsive data center environment. This transformation requires a strategic approach that encompasses technological upgrades, cultural shifts, and enhanced security measures. As organizations navigate this complex landscape, the ability to adapt and innovate will be key to breaking free from operational inertia and unlocking the transformative power of AI in data centers.
Strategies to Overcome Operational Inertia in Data Centers
In the rapidly evolving landscape of technology, data centers have become the backbone of digital infrastructure, supporting everything from cloud computing to artificial intelligence (AI) applications. However, as these facilities grow in complexity and scale, they often encounter a significant challenge: operational inertia. This phenomenon, characterized by resistance to change and slow adaptation to new technologies, can hinder the efficiency and effectiveness of data centers. To address this issue, it is crucial to implement strategies that not only overcome operational inertia but also accelerate the integration of AI-driven solutions.
One of the primary strategies to combat operational inertia is fostering a culture of innovation within the organization. Encouraging a mindset that embraces change and values continuous improvement can significantly reduce resistance to new technologies. This involves providing ongoing training and development opportunities for staff, ensuring they are well-versed in the latest AI advancements and data center management techniques. By equipping employees with the necessary skills and knowledge, organizations can create an environment where innovation thrives, and operational inertia is minimized.
In addition to cultivating a culture of innovation, it is essential to implement robust change management processes. These processes should be designed to facilitate smooth transitions when integrating AI-driven solutions into existing data center operations. This can be achieved by establishing clear communication channels, setting realistic timelines, and involving key stakeholders in the decision-making process. By doing so, organizations can ensure that changes are implemented efficiently and with minimal disruption to ongoing operations.
Moreover, leveraging data analytics can play a pivotal role in overcoming operational inertia. By harnessing the power of AI and machine learning, data centers can gain valuable insights into their operations, identifying areas where improvements can be made. Predictive analytics, for instance, can help anticipate potential issues before they arise, allowing for proactive maintenance and reducing downtime. This data-driven approach not only enhances operational efficiency but also supports informed decision-making, enabling organizations to adapt more readily to technological advancements.
Furthermore, adopting a modular approach to data center design can facilitate the integration of AI-driven solutions. Modular data centers offer flexibility and scalability, allowing organizations to incrementally incorporate new technologies without the need for extensive overhauls. This approach not only reduces the risk associated with large-scale changes but also enables data centers to remain agile in the face of evolving technological demands. By breaking down complex systems into manageable components, organizations can more easily implement AI-driven solutions, thereby overcoming operational inertia.
Collaboration with technology partners and industry experts is another effective strategy for addressing operational inertia. By engaging with external stakeholders, organizations can gain access to a wealth of knowledge and experience, helping them navigate the complexities of AI integration. These partnerships can provide valuable insights into best practices, emerging trends, and potential pitfalls, enabling data centers to make informed decisions and accelerate the adoption of AI-driven solutions.
In conclusion, overcoming operational inertia in data centers requires a multifaceted approach that combines cultural, procedural, and technological strategies. By fostering a culture of innovation, implementing robust change management processes, leveraging data analytics, adopting modular designs, and collaborating with industry experts, organizations can effectively break free from operational inertia. As a result, they can accelerate the integration of AI-driven solutions, enhancing the efficiency and effectiveness of their data center operations and positioning themselves for success in the digital age.
The Role of Automation in Accelerating AI-Driven Data Centers
In the rapidly evolving landscape of technology, AI-driven data centers are emerging as pivotal components in the digital infrastructure, offering unprecedented capabilities in data processing and management. As organizations increasingly rely on these advanced systems, the role of automation becomes crucial in accelerating their efficiency and effectiveness. Automation, in this context, serves as a catalyst, breaking free from the operational inertia that often hampers the full potential of AI-driven data centers.
To begin with, automation in AI-driven data centers facilitates the seamless integration of various processes, thereby enhancing operational efficiency. By automating routine tasks such as data backup, system updates, and network monitoring, data centers can significantly reduce the time and resources required for manual interventions. This not only minimizes human error but also allows IT personnel to focus on more strategic initiatives, thereby driving innovation and growth. Moreover, automation enables real-time data processing and analysis, which is essential for organizations that need to make swift, data-driven decisions.
Furthermore, automation plays a critical role in optimizing resource allocation within AI-driven data centers. Through intelligent algorithms and machine learning models, automated systems can predict workload patterns and adjust resource distribution accordingly. This dynamic allocation ensures that computational power is utilized efficiently, reducing energy consumption and operational costs. As a result, data centers can achieve a higher level of sustainability, aligning with global efforts to reduce carbon footprints and promote environmental responsibility.
In addition to resource optimization, automation enhances the scalability of AI-driven data centers. As data volumes continue to grow exponentially, the ability to scale operations without compromising performance becomes a key competitive advantage. Automated systems can quickly adapt to changing demands, provisioning additional resources as needed and decommissioning them when they are no longer required. This flexibility not only supports business continuity but also allows organizations to respond agilely to market fluctuations and emerging opportunities.
Security is another domain where automation significantly impacts AI-driven data centers. With the increasing sophistication of cyber threats, maintaining robust security measures is paramount. Automated security protocols can continuously monitor network activity, detect anomalies, and respond to potential threats in real-time. This proactive approach to cybersecurity ensures that data integrity and confidentiality are maintained, safeguarding sensitive information from unauthorized access and breaches.
Moreover, automation fosters innovation by enabling AI-driven data centers to experiment with new technologies and methodologies. By automating the deployment and testing of new applications, data centers can rapidly iterate and refine their offerings, accelerating the development cycle. This agility is crucial in a competitive landscape where the ability to innovate quickly can determine an organization’s success.
In conclusion, the role of automation in accelerating AI-driven data centers is multifaceted, encompassing operational efficiency, resource optimization, scalability, security, and innovation. By breaking free from operational inertia, automation empowers data centers to harness the full potential of AI technologies, driving transformative change across industries. As organizations continue to navigate the complexities of the digital age, embracing automation will be essential in unlocking new opportunities and achieving sustainable growth. Through strategic implementation of automated systems, AI-driven data centers can not only meet the demands of today but also anticipate and adapt to the challenges of tomorrow.
Leveraging Machine Learning for Enhanced Data Center Efficiency
In the rapidly evolving landscape of technology, data centers have become the backbone of digital infrastructure, supporting everything from cloud computing to artificial intelligence applications. As the demand for data processing and storage continues to surge, the need for more efficient and intelligent data center operations has never been more critical. Leveraging machine learning to enhance data center efficiency is emerging as a pivotal strategy to address these challenges, offering a pathway to break free from operational inertia and drive significant improvements in performance and sustainability.
Machine learning, a subset of artificial intelligence, involves the use of algorithms that enable systems to learn from data and make decisions with minimal human intervention. In the context of data centers, machine learning can be harnessed to optimize a wide array of operational aspects, from energy consumption to predictive maintenance. By analyzing vast amounts of data generated by data center operations, machine learning models can identify patterns and anomalies that would be impossible for human operators to detect. This capability allows for more precise control over resources, leading to enhanced efficiency and reduced operational costs.
One of the most significant areas where machine learning can make an impact is in energy management. Data centers are notorious for their high energy consumption, which not only drives up operational costs but also contributes to environmental concerns. Machine learning algorithms can analyze historical and real-time data to predict energy usage patterns and optimize cooling systems, which are among the largest consumers of energy in data centers. By dynamically adjusting cooling based on current and predicted workloads, data centers can achieve substantial energy savings without compromising performance.
Moreover, machine learning can play a crucial role in predictive maintenance, a proactive approach to equipment management that can prevent costly downtime. By continuously monitoring the health of data center equipment, machine learning models can predict potential failures before they occur, allowing operators to perform maintenance at the most opportune times. This not only extends the lifespan of critical infrastructure but also ensures that data centers remain operational and efficient.
Transitioning to a more machine learning-driven approach also facilitates better resource allocation. Data centers often face the challenge of balancing workloads across servers to maximize efficiency. Machine learning algorithms can analyze workload patterns and predict future demands, enabling more effective distribution of tasks across available resources. This leads to improved utilization rates and can significantly reduce the need for additional hardware, further driving down costs and energy consumption.
Furthermore, the integration of machine learning into data center operations can enhance security measures. By analyzing network traffic and user behavior, machine learning models can detect unusual activities that may indicate security threats. This allows for quicker response times and more robust protection against cyberattacks, safeguarding sensitive data and maintaining the integrity of data center operations.
In conclusion, the application of machine learning in data centers represents a transformative shift towards more intelligent and efficient operations. By breaking free from traditional operational inertia, data centers can achieve significant improvements in energy management, predictive maintenance, resource allocation, and security. As the digital landscape continues to expand, the adoption of machine learning technologies will be essential for data centers to meet the growing demands of the future while maintaining sustainability and cost-effectiveness. Embracing these advancements not only enhances operational efficiency but also positions data centers as pivotal players in the ongoing evolution of technology.
Case Studies: Successful AI Implementations in Data Centers
In recent years, the integration of artificial intelligence (AI) into data center operations has emerged as a transformative force, enabling organizations to break free from the constraints of traditional operational models. By examining successful case studies, we can gain valuable insights into how AI-driven solutions are revolutionizing data center management, enhancing efficiency, and reducing costs. One notable example is Google’s implementation of AI to optimize energy consumption in its data centers. By leveraging machine learning algorithms, Google has been able to predict and manage energy usage with remarkable precision. This approach not only minimizes energy waste but also significantly reduces the carbon footprint of its operations. The AI system continuously analyzes data from thousands of sensors, adjusting cooling systems and other variables in real-time to maintain optimal conditions. As a result, Google has reported a 40% reduction in energy used for cooling, demonstrating the substantial impact of AI on operational efficiency.
Transitioning to another compelling case, Microsoft’s Project Natick explores the potential of underwater data centers, utilizing AI to monitor and manage these unique environments. By deploying data centers on the ocean floor, Microsoft aims to address challenges related to cooling and energy efficiency. AI plays a crucial role in this endeavor by analyzing environmental data and optimizing operations to ensure reliability and sustainability. The project has shown promising results, with AI systems effectively managing power consumption and maintaining stable conditions, thus proving the viability of underwater data centers as a sustainable solution for future needs.
Furthermore, Equinix, a global leader in data center services, has successfully implemented AI to enhance predictive maintenance and improve equipment reliability. By employing AI-driven analytics, Equinix can anticipate equipment failures before they occur, allowing for proactive maintenance and reducing downtime. This predictive capability is achieved through the continuous monitoring of equipment performance data, which AI algorithms analyze to identify patterns and anomalies. Consequently, Equinix has reported a significant decrease in unexpected outages, leading to improved service reliability and customer satisfaction.
In addition to these examples, Alibaba’s use of AI in its data centers highlights the potential for AI to optimize resource allocation and workload management. By employing AI algorithms to analyze data traffic patterns and predict demand, Alibaba can dynamically allocate resources to meet fluctuating workloads. This approach not only maximizes resource utilization but also ensures that customer demands are met efficiently. The implementation of AI has enabled Alibaba to achieve a higher level of operational agility, allowing the company to scale its services seamlessly in response to changing market conditions.
Moreover, IBM’s application of AI in its data centers underscores the importance of AI in enhancing cybersecurity measures. By utilizing AI to monitor network traffic and detect anomalies, IBM can identify potential security threats in real-time. This proactive approach to cybersecurity is crucial in an era where data breaches and cyberattacks are increasingly sophisticated. AI-driven security systems can quickly adapt to new threats, providing a robust defense mechanism that traditional methods cannot match.
In conclusion, these case studies illustrate the profound impact of AI on data center operations, showcasing its potential to drive efficiency, sustainability, and security. As organizations continue to embrace AI-driven solutions, the data center landscape is poised for a significant transformation, breaking free from operational inertia and paving the way for a more innovative and resilient future. Through the strategic implementation of AI, data centers can not only meet the demands of today but also anticipate and adapt to the challenges of tomorrow.
Future Trends in AI-Driven Data Center Operations
In the rapidly evolving landscape of technology, AI-driven data centers are emerging as pivotal components in the digital infrastructure, promising to revolutionize how data is managed, processed, and utilized. As organizations increasingly rely on data to drive decision-making and innovation, the demand for more efficient, intelligent, and scalable data center operations has never been more critical. The integration of artificial intelligence into data center management is not merely an enhancement but a transformative shift that addresses the limitations of traditional systems, which often suffer from operational inertia.
One of the primary advantages of AI-driven data centers is their ability to optimize energy consumption, a significant concern given the growing environmental impact of data centers worldwide. By leveraging machine learning algorithms, these centers can predict and adjust energy usage in real-time, ensuring that resources are allocated efficiently without compromising performance. This capability not only reduces operational costs but also aligns with global sustainability goals, making AI-driven data centers an attractive option for environmentally conscious organizations.
Moreover, AI enhances predictive maintenance capabilities, a crucial aspect of data center operations. Traditional maintenance strategies often rely on scheduled checks and reactive measures, which can lead to unexpected downtimes and increased operational costs. In contrast, AI systems can analyze vast amounts of data from various sensors and logs to predict potential failures before they occur. This proactive approach minimizes downtime, enhances reliability, and extends the lifespan of critical infrastructure components, thereby providing a significant competitive advantage.
In addition to energy optimization and predictive maintenance, AI-driven data centers offer improved security measures. As cyber threats become more sophisticated, the need for robust security protocols is paramount. AI can detect anomalies and potential security breaches in real-time, allowing for swift responses to mitigate risks. By continuously learning from new data, AI systems can adapt to emerging threats, providing a dynamic and resilient defense mechanism that traditional security measures may lack.
Furthermore, the scalability of AI-driven data centers is another compelling benefit. As businesses grow and their data needs expand, the ability to scale operations efficiently is crucial. AI facilitates this by automating routine tasks and optimizing resource allocation, enabling data centers to handle increased workloads without a proportional increase in operational complexity or cost. This scalability ensures that organizations can continue to innovate and expand without being hindered by their data infrastructure.
Despite these advantages, the transition to AI-driven data centers is not without challenges. The integration of AI technologies requires significant investment in both hardware and software, as well as a skilled workforce capable of managing and maintaining these advanced systems. Additionally, concerns about data privacy and the ethical use of AI must be addressed to ensure that these technologies are deployed responsibly.
Nevertheless, the potential benefits of AI-driven data centers far outweigh the challenges, making them a critical component of future data infrastructure. As technology continues to advance, the role of AI in data center operations will likely expand, driving further innovation and efficiency. By breaking free from operational inertia, organizations can harness the full potential of their data, paving the way for a more intelligent and sustainable digital future. In conclusion, the acceleration of AI-driven data centers represents a significant step forward in the evolution of data management, offering a glimpse into a future where data centers are not just passive repositories of information but active participants in the digital ecosystem.
Q&A
1. **What is operational inertia in data centers?**
Operational inertia refers to the resistance to change and the slow adaptation of new technologies and processes within data centers, often due to legacy systems and established workflows.
2. **How can AI accelerate data center operations?**
AI can optimize resource allocation, predict maintenance needs, enhance energy efficiency, and automate routine tasks, leading to faster and more efficient data center operations.
3. **What role does machine learning play in AI-driven data centers?**
Machine learning algorithms analyze vast amounts of data to identify patterns and anomalies, enabling predictive maintenance, dynamic resource management, and improved decision-making.
4. **What are the benefits of breaking free from operational inertia?**
Overcoming operational inertia can lead to increased agility, reduced costs, improved performance, and the ability to quickly adapt to technological advancements and market demands.
5. **What challenges do data centers face in implementing AI solutions?**
Challenges include integrating AI with existing systems, ensuring data security and privacy, managing the complexity of AI models, and addressing the skills gap in AI expertise.
6. **How can data centers overcome these challenges?**
Data centers can overcome these challenges by investing in AI training for staff, collaborating with AI solution providers, adopting scalable AI platforms, and prioritizing security and compliance measures.Accelerating AI-driven data centers involves leveraging advanced technologies to enhance efficiency, scalability, and adaptability, thereby overcoming operational inertia. By integrating AI, data centers can optimize resource management, reduce energy consumption, and improve predictive maintenance, leading to cost savings and increased performance. The transition requires a strategic approach to address challenges such as data security, infrastructure upgrades, and workforce training. Ultimately, embracing AI-driven solutions enables data centers to meet the growing demands of digital transformation, ensuring they remain competitive and capable of supporting future technological advancements.
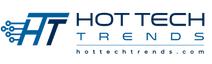