“A Practical Guide to Generative AI” serves as an essential resource for understanding and navigating the rapidly evolving field of generative artificial intelligence. This guide delves into the foundational concepts, techniques, and applications of generative AI, offering readers a comprehensive overview of how these systems create new content, from text and images to music and beyond. It explores the underlying algorithms, such as generative adversarial networks (GANs) and transformers, and provides practical insights into their implementation and potential impact across various industries. Designed for both newcomers and seasoned professionals, this guide equips readers with the knowledge and tools needed to harness the power of generative AI effectively and ethically.
Understanding The Basics Of Generative AI
Generative AI, a subset of artificial intelligence, has rapidly emerged as a transformative force across various industries. At its core, generative AI refers to algorithms that can create new content, whether it be text, images, music, or even complex data structures, by learning patterns from existing data. Understanding the basics of generative AI is crucial for anyone looking to harness its potential or simply comprehend its impact on modern technology.
To begin with, generative AI models are primarily based on neural networks, particularly deep learning architectures. These models are trained on vast datasets, allowing them to learn intricate patterns and relationships within the data. One of the most popular types of generative models is the Generative Adversarial Network (GAN), which consists of two neural networks: a generator and a discriminator. The generator creates new data instances, while the discriminator evaluates them for authenticity. Through this adversarial process, the generator improves its ability to produce data that closely resembles the real thing.
Another significant model in the realm of generative AI is the Variational Autoencoder (VAE). Unlike GANs, VAEs focus on learning a compressed representation of the data, which can then be used to generate new instances. This approach is particularly useful for tasks that require a more structured understanding of the data, such as generating variations of an object or filling in missing information.
Transitioning from the technical underpinnings to practical applications, generative AI has found a home in numerous fields. In the creative arts, for instance, artists and musicians are leveraging these models to push the boundaries of their work, creating pieces that blend human creativity with machine-generated innovation. In the realm of design, architects and product designers use generative AI to explore novel forms and structures that might not have been conceived through traditional methods.
Moreover, the impact of generative AI extends beyond the creative industries. In healthcare, for example, researchers are utilizing these models to generate synthetic medical data, which can be used to train other AI systems without compromising patient privacy. This capability is particularly valuable in scenarios where real-world data is scarce or sensitive. Additionally, in the field of drug discovery, generative AI is being employed to design new molecules, potentially accelerating the development of new treatments.
Despite its promising applications, generative AI is not without challenges. One of the primary concerns is the ethical implications of its use. The ability to generate highly realistic content raises questions about authenticity and the potential for misuse, such as in the creation of deepfakes. Furthermore, the quality of the generated content is heavily dependent on the quality and diversity of the training data. Biases present in the data can be inadvertently learned and perpetuated by the models, leading to skewed or unfair outcomes.
In conclusion, understanding the basics of generative AI involves not only grasping the technical aspects of how these models function but also appreciating their wide-ranging applications and the ethical considerations they entail. As generative AI continues to evolve, it holds the promise of revolutionizing industries and reshaping the way we interact with technology. However, it is imperative that we approach its development and deployment with a balanced perspective, ensuring that its benefits are maximized while its risks are carefully managed.
Key Applications Of Generative AI In Various Industries
Generative AI, a subset of artificial intelligence, has rapidly evolved to become a transformative force across various industries. By leveraging algorithms that can create new content, such as text, images, and even music, generative AI is reshaping how businesses operate and innovate. One of the most prominent applications of generative AI is in the creative industries, where it is used to generate art, design, and multimedia content. Artists and designers are increasingly collaborating with AI to push the boundaries of creativity, producing works that blend human intuition with machine precision. This collaboration not only enhances the creative process but also democratizes art by making sophisticated tools accessible to a broader audience.
Transitioning from the arts to the realm of entertainment, generative AI is making significant strides in the film and gaming industries. In filmmaking, AI algorithms are employed to generate realistic visual effects and even entire scenes, reducing production costs and time. Similarly, in the gaming industry, generative AI is used to create expansive, immersive worlds that adapt to player interactions, offering a personalized gaming experience. These applications highlight the potential of generative AI to revolutionize content creation by automating complex tasks while maintaining high levels of creativity and engagement.
In addition to creative fields, generative AI is making a substantial impact in healthcare. By analyzing vast amounts of medical data, AI models can generate insights that aid in diagnosis and treatment planning. For instance, generative AI can simulate molecular structures to accelerate drug discovery, potentially reducing the time and cost associated with bringing new medications to market. Furthermore, AI-generated models can assist in personalized medicine by predicting patient responses to different treatments, thereby improving outcomes and reducing adverse effects. This application underscores the potential of generative AI to enhance healthcare delivery and patient care.
Moreover, the financial sector is witnessing a paradigm shift with the integration of generative AI. Financial institutions are utilizing AI to generate predictive models that assess market trends and risks, enabling more informed decision-making. Additionally, generative AI is employed in fraud detection, where it analyzes transaction patterns to identify anomalies and potential threats. By automating these processes, financial organizations can enhance their operational efficiency and security, ultimately providing better services to their clients.
Transitioning to the field of manufacturing, generative AI is optimizing production processes and product design. By simulating various design scenarios, AI can suggest innovative solutions that improve product performance and reduce material waste. In manufacturing operations, AI-driven automation streamlines workflows, increases precision, and minimizes human error. These advancements not only boost productivity but also contribute to sustainable practices by reducing resource consumption.
Finally, the education sector is beginning to harness the power of generative AI to personalize learning experiences. AI-driven platforms can generate customized educational content tailored to individual learning styles and paces, thereby enhancing student engagement and comprehension. Additionally, AI can assist educators by automating administrative tasks, allowing them to focus more on teaching and student interaction.
In conclusion, generative AI is proving to be a versatile tool with applications spanning numerous industries. Its ability to generate new content and insights is driving innovation, improving efficiency, and opening new possibilities for growth and development. As generative AI continues to evolve, its integration into various sectors will likely deepen, further transforming the way we live and work.
Ethical Considerations In The Use Of Generative AI
Generative AI, a rapidly advancing field within artificial intelligence, has the potential to revolutionize various industries by creating content that ranges from text and images to music and beyond. However, as with any powerful technology, the deployment of generative AI comes with a set of ethical considerations that must be carefully navigated to ensure its responsible use. Understanding these ethical dimensions is crucial for developers, policymakers, and users alike, as they collectively shape the future of AI applications.
One of the primary ethical concerns surrounding generative AI is the potential for misuse in creating misleading or harmful content. Deepfakes, which are hyper-realistic digital forgeries, exemplify this risk. They can be used to fabricate videos or audio recordings that convincingly depict individuals saying or doing things they never did. This capability poses significant threats to privacy, reputation, and even national security. To mitigate these risks, it is essential to develop robust detection tools and establish clear legal frameworks that penalize malicious use while protecting individuals’ rights.
Moreover, generative AI systems often rely on vast datasets to learn and generate content. These datasets can inadvertently perpetuate biases present in the data, leading to outputs that reinforce stereotypes or discriminate against certain groups. For instance, a generative AI trained on biased text data might produce content that reflects gender or racial prejudices. Addressing this issue requires a concerted effort to curate diverse and representative datasets, as well as implementing algorithms that can identify and correct biases in real-time.
In addition to bias, the question of intellectual property rights presents another ethical challenge. Generative AI can create works that closely resemble existing copyrighted material, raising questions about originality and ownership. Determining who holds the rights to AI-generated content—whether it be the developer, the user, or the AI itself—remains a complex legal issue. Establishing clear guidelines and policies will be crucial in navigating these intellectual property concerns, ensuring that creators are fairly compensated while fostering innovation.
Furthermore, the environmental impact of generative AI cannot be overlooked. Training large AI models requires substantial computational resources, which in turn consume significant amounts of energy. This energy consumption contributes to carbon emissions, raising concerns about the sustainability of AI technologies. To address this, researchers and developers must prioritize the development of more energy-efficient algorithms and explore alternative energy sources to power AI systems.
Another ethical consideration involves the transparency and accountability of generative AI systems. Users often interact with AI-generated content without understanding how it was created or the underlying decision-making processes. This opacity can lead to a lack of trust and accountability, particularly when AI systems produce controversial or harmful outputs. To enhance transparency, developers should strive to create explainable AI models that allow users to understand the rationale behind AI-generated content. Additionally, establishing accountability mechanisms will ensure that developers and organizations are held responsible for the outputs of their AI systems.
In conclusion, while generative AI holds immense promise for innovation and creativity, its ethical implications must be carefully considered to ensure its responsible use. By addressing issues such as misuse, bias, intellectual property, environmental impact, and transparency, stakeholders can harness the potential of generative AI while safeguarding against its risks. As this technology continues to evolve, ongoing dialogue and collaboration among developers, policymakers, and society at large will be essential in navigating the ethical landscape of generative AI.
Tools And Technologies For Developing Generative AI Models
Generative AI has emerged as a transformative force in the realm of artificial intelligence, offering the ability to create content that ranges from text and images to music and beyond. As the demand for generative AI models continues to grow, understanding the tools and technologies that facilitate their development becomes increasingly important. This article aims to provide a comprehensive overview of the essential tools and technologies that are instrumental in developing generative AI models, thereby equipping developers and researchers with the knowledge needed to navigate this dynamic field.
To begin with, one of the foundational technologies in the development of generative AI models is deep learning frameworks. These frameworks, such as TensorFlow and PyTorch, provide the necessary infrastructure for building and training complex neural networks. TensorFlow, developed by Google, is renowned for its flexibility and scalability, making it a popular choice for both research and production environments. PyTorch, on the other hand, is favored for its dynamic computation graph, which allows for more intuitive model building and debugging. Both frameworks offer extensive libraries and tools that simplify the process of developing generative models, thereby enabling researchers to focus on innovation rather than implementation details.
In addition to deep learning frameworks, the choice of hardware plays a crucial role in the development of generative AI models. Graphics Processing Units (GPUs) have become the standard for training deep learning models due to their ability to perform parallel computations efficiently. Companies like NVIDIA have developed specialized GPUs that are optimized for AI workloads, significantly reducing training times and improving model performance. Furthermore, the advent of cloud computing platforms such as Amazon Web Services (AWS), Google Cloud Platform (GCP), and Microsoft Azure has democratized access to powerful computational resources. These platforms offer scalable infrastructure that can be tailored to the specific needs of generative AI projects, allowing developers to experiment with larger models and datasets without the constraints of local hardware limitations.
Transitioning from hardware to software, another critical component in the development of generative AI models is the availability of pre-trained models and transfer learning techniques. Pre-trained models, such as OpenAI’s GPT series and Google’s BERT, provide a robust starting point for developing generative applications. These models have been trained on vast amounts of data and can be fine-tuned for specific tasks, significantly reducing the time and resources required for training from scratch. Transfer learning, in this context, allows developers to leverage the knowledge embedded in these pre-trained models, adapting them to new domains with relatively small datasets.
Moreover, the integration of generative AI models into real-world applications necessitates the use of robust deployment tools. Frameworks like TensorFlow Serving and TorchServe facilitate the deployment of models in production environments, ensuring that they can handle real-time data and scale according to demand. These tools provide APIs and interfaces that simplify the process of integrating generative models into existing systems, thereby bridging the gap between development and deployment.
In conclusion, the development of generative AI models is underpinned by a suite of sophisticated tools and technologies. From deep learning frameworks and specialized hardware to pre-trained models and deployment solutions, each component plays a vital role in the creation and implementation of generative AI applications. As the field continues to evolve, staying abreast of these tools and technologies will be essential for developers and researchers aiming to harness the full potential of generative AI. By understanding and utilizing these resources, one can contribute to the advancement of AI and its myriad applications across diverse industries.
Challenges And Limitations Of Generative AI
Generative AI, a subset of artificial intelligence focused on creating data that mimics real-world inputs, has garnered significant attention for its potential to revolutionize various industries. However, despite its promising capabilities, generative AI is not without its challenges and limitations. Understanding these constraints is crucial for effectively harnessing the technology while mitigating potential risks.
One of the primary challenges of generative AI lies in the quality and bias of the data it is trained on. Generative models, such as Generative Adversarial Networks (GANs) and Variational Autoencoders (VAEs), rely heavily on large datasets to learn patterns and generate new content. If the training data is biased or unrepresentative, the AI can inadvertently perpetuate or even amplify these biases in its outputs. This issue is particularly concerning in applications like content creation and decision-making, where biased outputs can lead to misinformation or unfair treatment of certain groups. Therefore, ensuring the diversity and quality of training data is paramount to minimizing bias in generative AI systems.
Moreover, the interpretability of generative AI models poses another significant limitation. These models often function as “black boxes,” making it difficult for developers and users to understand how specific outputs are generated. This lack of transparency can be problematic, especially in fields where accountability and explainability are critical, such as healthcare and finance. Consequently, there is a growing demand for research into methods that can enhance the interpretability of generative models, allowing stakeholders to better trust and validate the AI’s outputs.
In addition to interpretability, the computational resources required for training and deploying generative AI models present a substantial challenge. These models typically demand significant processing power and memory, which can be costly and environmentally taxing. As a result, organizations with limited resources may find it difficult to implement generative AI solutions effectively. To address this, researchers are exploring more efficient algorithms and hardware optimizations that can reduce the computational burden without compromising performance.
Furthermore, the potential for misuse of generative AI technology cannot be overlooked. The ability to create realistic synthetic content, such as deepfakes, raises ethical and security concerns. Malicious actors could exploit these capabilities to produce deceptive media, manipulate public opinion, or commit fraud. To combat these threats, it is essential to develop robust detection mechanisms and establish regulatory frameworks that govern the ethical use of generative AI.
Despite these challenges, the limitations of generative AI also present opportunities for innovation and improvement. By addressing issues related to data quality, model interpretability, computational efficiency, and ethical use, researchers and practitioners can enhance the reliability and applicability of generative AI systems. Collaborative efforts between academia, industry, and policymakers will be crucial in navigating these challenges and unlocking the full potential of generative AI.
In conclusion, while generative AI holds immense promise, it is imperative to recognize and address its inherent challenges and limitations. By doing so, we can ensure that this transformative technology is developed and deployed responsibly, ultimately benefiting society as a whole. As the field continues to evolve, ongoing research and dialogue will be essential in overcoming these obstacles and paving the way for a future where generative AI can be harnessed safely and effectively.
Future Trends And Innovations In Generative AI
Generative AI, a subset of artificial intelligence focused on creating new content, has rapidly evolved, offering transformative potential across various industries. As we look to the future, several trends and innovations are poised to redefine the landscape of generative AI, promising to enhance its capabilities and broaden its applications. Understanding these trends is crucial for businesses and individuals aiming to leverage this technology effectively.
One of the most significant trends in generative AI is the increasing sophistication of models. Recent advancements in deep learning have led to the development of more complex algorithms capable of producing highly realistic content. For instance, models like GPT-3 and its successors have demonstrated an impressive ability to generate human-like text, opening new possibilities for content creation, customer service, and even education. As these models continue to evolve, we can expect even greater accuracy and creativity, enabling more nuanced and context-aware interactions.
In addition to text generation, generative AI is making strides in the realm of visual content. Innovations in generative adversarial networks (GANs) have facilitated the creation of hyper-realistic images and videos. This capability is being harnessed in fields such as entertainment, advertising, and design, where the demand for high-quality visual content is ever-growing. Moreover, the ability to generate synthetic data is proving invaluable for training AI models, particularly in scenarios where real-world data is scarce or sensitive. This trend is likely to accelerate, with generative AI playing a pivotal role in data augmentation and simulation.
Another promising area of development is the integration of generative AI with other emerging technologies. For example, the convergence of AI and blockchain is creating new opportunities for secure and transparent content generation and distribution. By leveraging blockchain’s decentralized nature, generative AI can ensure the authenticity and provenance of digital assets, addressing concerns around deepfakes and intellectual property rights. Furthermore, the combination of AI and the Internet of Things (IoT) is enabling the creation of intelligent environments that can autonomously generate and adapt content based on real-time data inputs.
As generative AI becomes more prevalent, ethical considerations are gaining prominence. The potential for misuse, particularly in the creation of misleading or harmful content, necessitates the development of robust ethical frameworks and regulatory guidelines. Researchers and policymakers are increasingly focused on ensuring that generative AI is used responsibly, with an emphasis on transparency, accountability, and fairness. This includes implementing mechanisms to detect and mitigate biases in AI-generated content, as well as establishing clear guidelines for its use in sensitive areas such as journalism and public communication.
Looking ahead, the democratization of generative AI tools is set to empower a broader range of users. As these technologies become more accessible, individuals and small businesses will be able to harness the power of AI to innovate and compete on a level playing field with larger organizations. This democratization is likely to spur a wave of creativity and entrepreneurship, as users experiment with new applications and business models.
In conclusion, the future of generative AI is marked by rapid advancements and a growing array of applications. As models become more sophisticated and integrated with other technologies, the potential for innovation is vast. However, with these opportunities come challenges, particularly in terms of ethical use and regulation. By staying informed about these trends and developments, stakeholders can navigate the evolving landscape of generative AI and harness its potential for positive impact.
Q&A
1. **What is the primary focus of “A Practical Guide to Generative AI”?**
– The guide primarily focuses on providing practical insights and methodologies for implementing and utilizing generative AI technologies in various applications.
2. **What are some key applications of generative AI discussed in the guide?**
– Key applications include content creation, data augmentation, design and art generation, and enhancing machine learning models.
3. **Does the guide cover ethical considerations in generative AI?**
– Yes, the guide addresses ethical considerations, including issues related to bias, intellectual property, and the potential misuse of generative AI technologies.
4. **What technical prerequisites are recommended for understanding the guide?**
– A basic understanding of machine learning concepts, familiarity with neural networks, and some programming experience are recommended for readers.
5. **Are there any case studies or real-world examples included in the guide?**
– Yes, the guide includes several case studies and real-world examples to illustrate the practical applications and impact of generative AI.
6. **Does the guide provide any tools or frameworks for working with generative AI?**
– The guide discusses various tools and frameworks, such as TensorFlow, PyTorch, and GANs (Generative Adversarial Networks), to help readers implement generative AI solutions.”A Practical Guide to Generative AI” provides a comprehensive overview of the rapidly evolving field of generative artificial intelligence, offering insights into its foundational concepts, applications, and ethical considerations. The guide effectively demystifies complex topics, making them accessible to a broad audience, from beginners to seasoned professionals. It emphasizes the transformative potential of generative AI across various industries, while also highlighting the importance of responsible use and the challenges associated with bias and data privacy. Overall, the guide serves as a valuable resource for anyone looking to understand and leverage the capabilities of generative AI in practical, innovative ways.
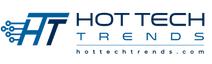