Decentralizing AI involves distributing the development, deployment, and governance of artificial intelligence systems across multiple entities and locations, rather than centralizing control within a single organization or authority. This approach aims to mitigate various risks associated with AI, such as data privacy concerns, monopolistic control, and systemic vulnerabilities. By leveraging decentralized networks and technologies like blockchain, stakeholders can enhance transparency, accountability, and resilience in AI systems. Decentralization also fosters innovation by enabling diverse contributions from a broader range of participants, reducing the potential for bias and enhancing the robustness of AI applications. As AI continues to permeate various aspects of society, adopting decentralized strategies becomes crucial to ensuring ethical standards, safeguarding individual rights, and promoting equitable access to AI’s benefits.
Understanding Decentralized AI: Key Concepts and Benefits
Decentralizing artificial intelligence (AI) represents a paradigm shift in how AI systems are developed, deployed, and managed. This approach seeks to distribute the control and decision-making processes across a network rather than centralizing them within a single entity. Understanding decentralized AI requires a grasp of its foundational concepts and the benefits it offers, particularly in mitigating risks associated with traditional, centralized AI systems.
At its core, decentralized AI leverages distributed ledger technologies, such as blockchain, to ensure transparency, security, and accountability. By decentralizing the control of AI models, stakeholders can collectively oversee the development and deployment processes, thereby reducing the potential for misuse or bias. This collaborative approach not only democratizes AI but also enhances trust among users and developers. Furthermore, decentralized AI systems can operate on peer-to-peer networks, allowing for more robust and resilient infrastructures that are less susceptible to single points of failure.
One of the primary benefits of decentralized AI is its potential to enhance data privacy. In centralized systems, data is often stored and processed in large, centralized databases, making it vulnerable to breaches and unauthorized access. Decentralized AI, on the other hand, can employ techniques such as federated learning, where data remains on local devices and only model updates are shared across the network. This ensures that sensitive information is not exposed, thereby safeguarding user privacy while still enabling the development of powerful AI models.
Moreover, decentralizing AI can lead to more equitable access to AI technologies. In a centralized model, access to AI capabilities is often limited to large corporations or entities with significant resources. Decentralized AI, however, can empower smaller organizations and individuals by providing them with the tools and platforms needed to develop and deploy AI solutions. This democratization of AI can foster innovation and competition, leading to a more diverse range of applications and solutions that cater to a broader spectrum of needs.
In addition to privacy and accessibility, decentralized AI can also enhance the robustness and reliability of AI systems. By distributing the computational processes across multiple nodes, decentralized AI can reduce the risk of system failures and improve the overall resilience of the network. This is particularly important in critical applications where reliability is paramount, such as in healthcare or autonomous vehicles. Furthermore, decentralized systems can facilitate more efficient resource utilization, as computational tasks can be distributed to underutilized nodes, optimizing performance and reducing costs.
Transitioning to decentralized AI, however, is not without its challenges. It requires a rethinking of existing infrastructures and the development of new protocols and standards to ensure interoperability and security. Additionally, there is a need for governance frameworks that can effectively manage decentralized networks and address issues such as accountability and ethical considerations. Despite these challenges, the potential benefits of decentralized AI make it a compelling strategy for mitigating the risks associated with traditional AI systems.
In conclusion, understanding decentralized AI involves recognizing its key concepts and the myriad benefits it offers. By decentralizing control and decision-making, AI systems can become more transparent, secure, and equitable. As the field of AI continues to evolve, embracing decentralization could be a crucial step in ensuring that AI technologies are developed and deployed in a manner that is both responsible and beneficial to society as a whole.
Implementing Blockchain for Secure AI Decentralization
In recent years, the rapid advancement of artificial intelligence (AI) has brought about significant benefits across various sectors, from healthcare to finance. However, the centralization of AI systems has raised concerns regarding data privacy, security, and the potential for misuse. To address these challenges, the integration of blockchain technology presents a promising strategy for decentralizing AI, thereby mitigating associated risks. By leveraging the inherent characteristics of blockchain, such as transparency, immutability, and decentralization, AI systems can be made more secure and trustworthy.
Blockchain technology, at its core, is a distributed ledger that records transactions across multiple nodes in a network. This decentralized nature ensures that no single entity has control over the entire system, thereby reducing the risk of data manipulation or unauthorized access. When applied to AI, blockchain can facilitate the secure sharing of data and models across different stakeholders without compromising privacy. For instance, data used to train AI models can be stored on a blockchain, allowing multiple parties to contribute and verify the data’s integrity without exposing sensitive information.
Moreover, smart contracts, which are self-executing contracts with the terms of the agreement directly written into code, can be employed to automate and enforce agreements between parties in an AI ecosystem. These contracts can ensure that AI models are used as intended and that any changes to the models are transparent and agreed upon by all parties involved. This level of transparency and automation not only enhances trust but also reduces the potential for disputes and errors.
In addition to data security and transparency, blockchain can also play a crucial role in enhancing the accountability of AI systems. By recording every interaction and decision made by an AI model on a blockchain, stakeholders can trace the decision-making process and identify any biases or errors. This audit trail is invaluable for ensuring compliance with regulatory standards and for building public trust in AI technologies.
Furthermore, the decentralized nature of blockchain can foster innovation by enabling a more collaborative approach to AI development. Researchers and developers from around the world can contribute to and access a shared pool of data and models, accelerating the pace of innovation while maintaining security and privacy. This open and collaborative environment can lead to the development of more robust and diverse AI systems that are better equipped to handle complex real-world challenges.
However, implementing blockchain for AI decentralization is not without its challenges. The scalability of blockchain networks remains a significant concern, as the technology must be able to handle the vast amounts of data generated by AI systems efficiently. Additionally, the integration of blockchain with existing AI infrastructures requires careful planning and consideration to ensure compatibility and performance.
Despite these challenges, the potential benefits of using blockchain to decentralize AI are substantial. By addressing key issues related to security, transparency, and accountability, blockchain can help mitigate the risks associated with centralized AI systems. As the technology continues to evolve, it is crucial for stakeholders to collaborate and explore innovative solutions that leverage the strengths of both AI and blockchain. In doing so, they can pave the way for a more secure, transparent, and equitable future in which AI technologies are developed and deployed responsibly.
Privacy-Preserving Techniques in Decentralized AI Systems
In the rapidly evolving landscape of artificial intelligence, the decentralization of AI systems has emerged as a promising approach to mitigate various risks associated with centralized models. As AI technologies become increasingly integrated into our daily lives, concerns about privacy, data security, and ethical use have intensified. Decentralized AI systems, which distribute data processing across multiple nodes rather than relying on a single centralized server, offer a compelling solution to these challenges. One of the most critical aspects of implementing decentralized AI is the incorporation of privacy-preserving techniques, which ensure that sensitive information remains secure while still enabling the robust functionality of AI applications.
To begin with, privacy-preserving techniques in decentralized AI systems are essential for protecting user data from unauthorized access and potential misuse. One such technique is federated learning, which allows machine learning models to be trained across multiple decentralized devices or servers while keeping the data localized. In this approach, only the model updates, rather than the raw data, are shared with a central server. This ensures that personal data remains on the user’s device, significantly reducing the risk of data breaches and enhancing privacy.
Moreover, differential privacy is another technique that plays a crucial role in safeguarding individual data within decentralized AI systems. By adding a controlled amount of noise to the data, differential privacy ensures that the output of a computation does not reveal specific information about any single individual. This method provides a mathematical guarantee of privacy, making it a valuable tool for organizations that handle sensitive data. When combined with federated learning, differential privacy can further enhance the security and privacy of decentralized AI systems, creating a robust framework for data protection.
In addition to these techniques, homomorphic encryption offers a powerful solution for privacy preservation in decentralized AI. This form of encryption allows computations to be performed on encrypted data without requiring decryption, ensuring that the data remains secure throughout the processing phase. By enabling encrypted data to be used in AI model training and inference, homomorphic encryption eliminates the need to expose raw data, thus maintaining privacy and confidentiality. Although computationally intensive, advancements in this field are making it increasingly feasible for practical applications.
Furthermore, secure multi-party computation (SMPC) is another innovative approach that facilitates privacy-preserving operations in decentralized AI systems. SMPC allows multiple parties to jointly compute a function over their inputs while keeping those inputs private. This technique is particularly useful in scenarios where data from different sources needs to be combined for AI model training without compromising the privacy of each source. By ensuring that no single party has access to the complete dataset, SMPC enhances the security and privacy of decentralized AI systems.
As we continue to explore the potential of decentralized AI, it is imperative to address the challenges associated with privacy and data security. The integration of privacy-preserving techniques such as federated learning, differential privacy, homomorphic encryption, and secure multi-party computation is essential for building trust and ensuring the ethical use of AI technologies. By prioritizing privacy in the design and implementation of decentralized AI systems, we can harness the full potential of AI while safeguarding individual rights and maintaining public confidence. As these techniques evolve and mature, they will undoubtedly play a pivotal role in shaping the future of AI, enabling a more secure and privacy-conscious digital ecosystem.
Collaborative AI Models: Enhancing Decentralization and Reducing Risks
In recent years, the rapid advancement of artificial intelligence (AI) has brought about significant transformations across various sectors. However, with these advancements come inherent risks, particularly concerning data privacy, security, and the concentration of power. To address these challenges, the concept of decentralizing AI has gained traction, with collaborative AI models emerging as a promising strategy to enhance decentralization and mitigate associated risks. By distributing AI processes and decision-making across multiple nodes, collaborative AI models aim to reduce the vulnerabilities linked to centralized systems.
One of the primary benefits of decentralizing AI through collaborative models is the enhancement of data privacy. In traditional centralized AI systems, vast amounts of data are often stored and processed in a single location, making them susceptible to breaches and unauthorized access. In contrast, decentralized AI models distribute data across various nodes, ensuring that no single entity has complete access to the entire dataset. This distribution not only enhances privacy but also aligns with data protection regulations, such as the General Data Protection Regulation (GDPR), which emphasize the importance of safeguarding personal information.
Moreover, collaborative AI models can significantly improve the robustness and resilience of AI systems. Centralized systems are often vulnerable to single points of failure, where a malfunction or attack on the central node can disrupt the entire system. By decentralizing AI processes, collaborative models distribute the computational load and decision-making across multiple nodes, thereby reducing the risk of system-wide failures. This distributed approach ensures that even if one node is compromised, the overall system remains functional and secure.
In addition to enhancing privacy and resilience, decentralizing AI through collaborative models can also democratize access to AI technologies. Centralized AI systems are typically controlled by a few large organizations, leading to a concentration of power and resources. This concentration can stifle innovation and limit opportunities for smaller entities to participate in the AI ecosystem. By adopting decentralized models, a wider range of stakeholders, including startups, academic institutions, and individual developers, can contribute to and benefit from AI advancements. This democratization fosters a more inclusive and competitive environment, driving innovation and ensuring that AI technologies are developed in a manner that serves the broader public interest.
Furthermore, collaborative AI models can facilitate more transparent and accountable AI systems. In centralized models, decision-making processes are often opaque, making it difficult to understand how AI systems arrive at specific conclusions. Decentralized models, on the other hand, promote transparency by allowing multiple stakeholders to participate in the development and oversight of AI systems. This collaborative approach encourages the sharing of knowledge and expertise, leading to more robust and explainable AI models. Additionally, it enables the establishment of governance frameworks that ensure AI systems operate ethically and responsibly.
In conclusion, the decentralization of AI through collaborative models presents a viable strategy to mitigate the risks associated with centralized AI systems. By enhancing data privacy, improving system resilience, democratizing access, and promoting transparency, these models address key concerns related to the concentration of power and the potential misuse of AI technologies. As the field of AI continues to evolve, embracing decentralization and collaboration will be crucial in ensuring that AI systems are developed and deployed in a manner that is secure, equitable, and aligned with societal values.
Governance Frameworks for Decentralized AI Networks
In the rapidly evolving landscape of artificial intelligence, the decentralization of AI networks presents both opportunities and challenges. As AI systems become more integrated into various aspects of society, the need for robust governance frameworks to manage decentralized AI networks becomes increasingly critical. These frameworks are essential to mitigate risks associated with decentralized AI, ensuring that the benefits of AI are maximized while minimizing potential harms.
To begin with, decentralization in AI refers to the distribution of AI processing and decision-making across multiple nodes or entities, rather than relying on a single centralized authority. This approach can enhance the resilience and scalability of AI systems, as well as promote innovation by allowing diverse stakeholders to contribute to AI development. However, decentralization also introduces complexities in governance, as it requires coordination among various actors with potentially conflicting interests.
One strategy to address these complexities is the establishment of clear and transparent protocols for data sharing and usage. In decentralized AI networks, data is often distributed across different nodes, making it crucial to have standardized protocols that ensure data integrity, privacy, and security. By implementing such protocols, stakeholders can build trust in the system, knowing that their data is being handled responsibly and ethically.
Moreover, decentralized AI networks necessitate the development of consensus mechanisms to facilitate decision-making. These mechanisms can take various forms, such as voting systems or algorithmic consensus, and are designed to ensure that decisions are made in a fair and democratic manner. By incorporating consensus mechanisms, decentralized AI networks can prevent the concentration of power in the hands of a few entities, thereby reducing the risk of biased or unethical outcomes.
In addition to technical solutions, governance frameworks for decentralized AI networks must also consider legal and regulatory aspects. Policymakers play a crucial role in establishing the legal parameters within which decentralized AI networks operate. This includes setting standards for accountability and liability, as well as ensuring compliance with existing laws and regulations. By providing a clear legal framework, policymakers can help mitigate risks associated with decentralized AI, such as data breaches or misuse of AI technologies.
Furthermore, fostering collaboration among stakeholders is essential for effective governance of decentralized AI networks. This involves engaging a diverse range of actors, including governments, private sector companies, academia, and civil society, in the development and implementation of governance frameworks. By encouraging collaboration, stakeholders can share knowledge and resources, as well as align their efforts towards common goals, thereby enhancing the overall effectiveness of governance strategies.
Finally, continuous monitoring and evaluation are vital components of governance frameworks for decentralized AI networks. As AI technologies and their applications continue to evolve, governance frameworks must be adaptable to changing circumstances. Regular assessments of the effectiveness of governance strategies can help identify areas for improvement and ensure that the frameworks remain relevant and effective in mitigating risks.
In conclusion, the decentralization of AI networks presents unique challenges that require comprehensive governance frameworks to address. By implementing clear data protocols, consensus mechanisms, legal standards, and fostering collaboration among stakeholders, these frameworks can effectively mitigate risks associated with decentralized AI. As the field of AI continues to advance, it is imperative that governance frameworks evolve in tandem, ensuring that the benefits of AI are realized while safeguarding against potential harms.
Case Studies: Successful Decentralization of AI Applications
In recent years, the decentralization of artificial intelligence (AI) has emerged as a pivotal strategy to mitigate the risks associated with centralized AI systems. By distributing AI capabilities across various nodes rather than relying on a single, centralized entity, organizations can enhance security, improve resilience, and foster innovation. Several case studies illustrate the successful implementation of decentralized AI applications, offering valuable insights into the potential benefits and challenges of this approach.
One notable example is the Ocean Protocol, a decentralized data exchange protocol that enables secure and privacy-preserving data sharing. By leveraging blockchain technology, Ocean Protocol allows data providers to maintain control over their data while making it accessible to AI developers. This decentralized approach not only mitigates the risk of data breaches but also democratizes access to data, enabling a wider range of participants to contribute to AI development. Consequently, this fosters a more diverse and inclusive AI ecosystem, reducing the concentration of power in the hands of a few large tech companies.
Similarly, the SingularityNET platform exemplifies the potential of decentralized AI networks. SingularityNET is a decentralized marketplace for AI services, where developers can create, share, and monetize AI algorithms. By decentralizing the development and distribution of AI services, SingularityNET reduces dependency on centralized platforms and encourages collaboration among developers worldwide. This open and collaborative environment accelerates innovation and ensures that AI advancements are not confined to a select group of entities. Moreover, the platform’s use of blockchain technology ensures transparency and trust, addressing concerns about the ethical use of AI.
Transitioning to another successful case, the Fetch.ai project demonstrates how decentralized AI can optimize complex systems. Fetch.ai is a decentralized network that uses autonomous economic agents to perform tasks such as optimizing energy grids, managing supply chains, and coordinating transportation systems. By decentralizing decision-making processes, Fetch.ai enhances the efficiency and resilience of these systems, reducing the risk of single points of failure. Furthermore, the use of decentralized AI agents allows for real-time adaptation to changing conditions, improving the overall performance of the systems they manage.
In addition to these examples, the healthcare sector has also seen promising developments in decentralized AI applications. For instance, the Alethea AI project focuses on creating decentralized AI-generated media, which can be used to develop personalized healthcare solutions. By decentralizing the creation and distribution of AI-generated content, Alethea AI empowers patients and healthcare providers to access tailored information and services, enhancing patient care and outcomes. This approach not only mitigates the risks associated with centralized control over sensitive healthcare data but also promotes innovation in personalized medicine.
While these case studies highlight the potential benefits of decentralizing AI, it is important to acknowledge the challenges that accompany this approach. Decentralized systems often require robust infrastructure and governance mechanisms to ensure their effective operation. Additionally, issues related to interoperability, scalability, and regulatory compliance must be addressed to fully realize the potential of decentralized AI applications. Nevertheless, the successful implementation of decentralized AI in various domains underscores its viability as a strategy to mitigate risks and drive innovation.
In conclusion, the decentralization of AI presents a promising avenue for addressing the risks associated with centralized AI systems. Through case studies such as Ocean Protocol, SingularityNET, Fetch.ai, and Alethea AI, it is evident that decentralized AI can enhance security, foster collaboration, and improve system resilience. As the field continues to evolve, these examples serve as valuable models for organizations seeking to harness the benefits of decentralized AI while navigating its inherent challenges.
Q&A
1. **What is decentralizing AI?**
Decentralizing AI involves distributing AI development and deployment across multiple nodes or entities rather than relying on a centralized authority, enhancing transparency, security, and resilience.
2. **Why is decentralizing AI important?**
It mitigates risks such as data privacy breaches, single points of failure, and monopolistic control, promoting fairness and inclusivity in AI technologies.
3. **What are some strategies for decentralizing AI?**
Strategies include using blockchain technology for secure data sharing, federated learning for distributed model training, and open-source platforms to encourage collaborative development.
4. **How does federated learning contribute to decentralizing AI?**
Federated learning allows AI models to be trained across decentralized devices or servers without sharing raw data, preserving privacy and reducing the need for centralized data storage.
5. **What role does blockchain play in decentralizing AI?**
Blockchain provides a secure, transparent ledger for recording AI transactions and data exchanges, ensuring trust and accountability in decentralized AI systems.
6. **What are the potential challenges of decentralizing AI?**
Challenges include ensuring interoperability between decentralized systems, managing the complexity of distributed networks, and addressing potential security vulnerabilities.Decentralizing AI involves distributing the development, deployment, and governance of artificial intelligence systems across multiple entities to reduce the concentration of power and mitigate associated risks. This approach can enhance transparency, accountability, and resilience by preventing monopolistic control and fostering diverse perspectives in AI development. Strategies for decentralization include open-source collaboration, federated learning, and blockchain integration, which collectively promote data privacy, security, and equitable access to AI technologies. By decentralizing AI, stakeholders can address ethical concerns, reduce systemic biases, and ensure that AI advancements benefit a broader spectrum of society, ultimately leading to more robust and trustworthy AI systems.
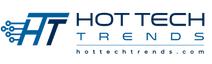