Deep learning models have increasingly drawn inspiration from various domains to enhance their capabilities, and one intriguing intersection is with developmental psychology, particularly in the realm of intuitive physics. Intuitive physics refers to the innate human ability to understand and predict the physical properties and behaviors of objects in the world, a skill that emerges early in human development. By emulating this cognitive process, deep learning models aim to replicate the human-like understanding of physical interactions and dynamics. This approach leverages insights from developmental psychology to inform the design and training of neural networks, enabling them to grasp concepts such as object permanence, causality, and motion prediction. The integration of these psychological insights into deep learning frameworks not only advances the field of artificial intelligence but also provides a deeper understanding of human cognition and its potential applications in creating more sophisticated and intuitive AI systems.
Understanding Intuitive Physics: How Deep Learning Models Mimic Human Cognition
In recent years, the intersection of artificial intelligence and cognitive science has led to groundbreaking advancements in understanding how machines can emulate human-like reasoning. One of the most intriguing areas of this research is the development of deep learning models that mimic intuitive physics, a fundamental aspect of human cognition. Intuitive physics refers to the innate ability of humans to understand and predict the physical properties and behaviors of objects in the world around them. This capability is evident from a young age, as even infants can anticipate the trajectory of a moving object or recognize when an object is unsupported and likely to fall. By drawing insights from developmental psychology, researchers are now able to design deep learning models that replicate these intuitive processes, thereby enhancing the cognitive abilities of artificial systems.
The foundation of this research lies in the understanding that human cognition is not solely based on explicit learning but also on implicit, intuitive processes that develop over time. Developmental psychology provides a framework for understanding how these intuitive abilities emerge in humans, offering valuable insights into how they can be replicated in machines. By studying the stages of cognitive development in children, researchers can identify key milestones in the acquisition of intuitive physics, such as object permanence and causality. These insights inform the design of deep learning models, enabling them to simulate similar developmental trajectories.
One of the primary challenges in this endeavor is the creation of models that can generalize from limited data, much like human infants who learn about the world through sparse and often ambiguous experiences. To address this, researchers employ techniques such as transfer learning and meta-learning, which allow models to leverage prior knowledge and adapt to new situations with minimal data. These approaches are inspired by the human ability to apply learned concepts to novel contexts, a hallmark of intuitive reasoning.
Moreover, the integration of neural networks with probabilistic reasoning frameworks has proven to be a powerful tool in emulating intuitive physics. By combining the pattern recognition capabilities of neural networks with the uncertainty handling of probabilistic models, researchers can create systems that not only predict physical outcomes but also quantify the confidence of their predictions. This mirrors the human tendency to assess the likelihood of various outcomes based on past experiences and current observations.
The implications of these advancements are far-reaching, with potential applications spanning robotics, autonomous vehicles, and virtual reality. In robotics, for instance, machines equipped with an understanding of intuitive physics can navigate complex environments more effectively, avoiding obstacles and interacting with objects in a human-like manner. Similarly, autonomous vehicles can benefit from these models by predicting the behavior of other vehicles and pedestrians, thereby enhancing safety and efficiency.
In conclusion, the emulation of intuitive physics through deep learning models represents a significant step forward in the quest to create machines that think and reason like humans. By leveraging insights from developmental psychology, researchers are able to design systems that not only replicate human cognitive abilities but also extend them in novel and innovative ways. As this field continues to evolve, it holds the promise of transforming how machines interact with the world, ultimately leading to more intelligent and adaptable artificial systems.
Developmental Psychology Meets AI: Insights into Intuitive Physics
In recent years, the intersection of developmental psychology and artificial intelligence has opened new avenues for understanding how machines can emulate human-like cognitive processes. One of the most intriguing areas of exploration is the emulation of intuitive physics by deep learning models, inspired by insights from developmental psychology. Intuitive physics refers to the innate ability of humans, even from a very young age, to understand and predict the behavior of physical objects in the world. This capability, which includes grasping concepts such as gravity, object permanence, and causality, is fundamental to human cognition and interaction with the environment.
Developmental psychology has long studied how infants and young children develop an understanding of these physical principles. Research suggests that even infants possess a rudimentary grasp of intuitive physics, which becomes more sophisticated as they grow. This developmental trajectory provides a rich framework for designing AI systems that can mimic similar learning processes. By examining how children learn about the physical world, researchers can draw parallels to how machines might be trained to develop a comparable understanding.
Deep learning models, particularly those employing neural networks, have shown promise in replicating aspects of intuitive physics. These models can be trained on vast datasets to recognize patterns and make predictions about physical interactions. However, unlike traditional programming approaches that rely on explicit rules, deep learning models learn from examples, much like children do. This learning process involves adjusting the model’s parameters to minimize errors in prediction, thereby gradually improving its understanding of physical phenomena.
One of the key insights from developmental psychology that has informed the design of these models is the importance of embodied learning. Children learn about the world not just by observing it but by interacting with it. This active engagement allows them to test hypotheses and refine their understanding through trial and error. Similarly, AI researchers have found that models trained in environments where they can simulate interactions with objects tend to develop a more robust understanding of intuitive physics. By allowing models to “play” in virtual environments, they can explore a wide range of scenarios and learn from the outcomes, much like a child playing with toys.
Moreover, the concept of transfer learning, which is prevalent in both human learning and AI, plays a crucial role in this context. Children often apply knowledge gained in one context to new, unfamiliar situations. Deep learning models can be designed to exhibit similar capabilities, enabling them to generalize their understanding of physics across different scenarios. This ability to transfer knowledge is essential for creating AI systems that can adapt to new environments and tasks without requiring extensive retraining.
In addition to these parallels, the collaboration between developmental psychology and AI research has highlighted the importance of curiosity-driven learning. Children are naturally curious, and this intrinsic motivation drives them to explore and learn about their surroundings. Incorporating mechanisms that mimic curiosity into AI models can enhance their ability to discover new patterns and improve their predictive accuracy.
In conclusion, the integration of insights from developmental psychology into the design of deep learning models represents a promising frontier in AI research. By emulating the ways in which humans, particularly children, learn about the physical world, these models can achieve a more nuanced understanding of intuitive physics. This interdisciplinary approach not only advances the field of artificial intelligence but also provides valuable insights into the fundamental nature of human cognition. As research continues to evolve, the collaboration between these two fields holds the potential to unlock new levels of machine intelligence that are both sophisticated and human-like.
Bridging the Gap: Deep Learning and Human Intuition in Physics
In recent years, the field of artificial intelligence has made significant strides, particularly in the realm of deep learning. One of the most intriguing developments is the ability of deep learning models to emulate human-like intuition in understanding physical phenomena. This advancement is largely attributed to insights drawn from developmental psychology, which studies how humans, especially infants, develop an understanding of the physical world. By bridging the gap between deep learning and human intuition, researchers are uncovering new ways to enhance machine learning models, making them more adept at predicting and interpreting complex physical interactions.
The concept of intuitive physics refers to the innate ability of humans to predict and understand the behavior of objects in the physical world. From a young age, humans can anticipate the trajectory of a thrown ball or understand that unsupported objects will fall. This intuitive grasp of physics is not explicitly taught but rather developed through interaction with the environment. Developmental psychologists have long studied these phenomena, seeking to understand the underlying cognitive processes that enable such intuitive understanding. By examining how infants and young children perceive and interact with their surroundings, researchers have gained valuable insights into the fundamental principles of intuitive physics.
In parallel, the field of deep learning has been exploring ways to replicate this intuitive understanding in artificial systems. Traditional machine learning models often rely on large datasets and explicit programming to perform specific tasks. However, these models typically lack the flexibility and adaptability that characterize human intuition. By incorporating principles from developmental psychology, researchers are developing deep learning models that can learn and generalize from limited data, much like humans do. This approach involves training models on a variety of physical scenarios, allowing them to develop an implicit understanding of the underlying principles governing these interactions.
One of the key challenges in this endeavor is designing models that can learn from experience in a manner similar to human learning. To address this, researchers have turned to techniques such as reinforcement learning, which allows models to learn through trial and error. By simulating environments where models can experiment with different actions and observe the outcomes, researchers enable these systems to develop a more nuanced understanding of physical laws. This process mirrors the way infants learn about the world by interacting with it, gradually building an intuitive sense of how objects behave.
Moreover, the integration of developmental psychology insights into deep learning models has led to the development of more robust and versatile systems. These models are not only capable of predicting physical interactions with greater accuracy but also exhibit a degree of adaptability that was previously unattainable. For instance, they can generalize learned principles to novel situations, demonstrating a level of flexibility akin to human intuition. This capability is particularly valuable in applications such as robotics, where machines must navigate and interact with dynamic environments.
In conclusion, the fusion of deep learning and developmental psychology represents a promising frontier in artificial intelligence research. By emulating human-like intuition in understanding physics, researchers are creating models that are more efficient, adaptable, and capable of tackling complex tasks. As this interdisciplinary approach continues to evolve, it holds the potential to revolutionize the way machines interact with the physical world, ultimately bridging the gap between artificial and human intelligence.
The Role of Developmental Psychology in Enhancing AI’s Understanding of Physics
In recent years, the intersection of artificial intelligence and developmental psychology has opened new avenues for enhancing AI’s understanding of intuitive physics. This interdisciplinary approach leverages insights from how humans, particularly infants, develop an understanding of the physical world to inform the design of deep learning models. By examining the cognitive processes that underpin human learning, researchers aim to create AI systems that can emulate similar intuitive reasoning about physical phenomena.
Developmental psychology provides a rich framework for understanding how humans acquire knowledge about the world. Infants, for instance, demonstrate an innate ability to grasp basic physical principles, such as object permanence and causality, even before they can articulate these concepts. This early understanding is not the result of explicit teaching but rather an intuitive grasp of how objects interact in space and time. By studying these developmental milestones, AI researchers can identify key cognitive processes that can be translated into computational models.
One of the primary challenges in developing AI systems with an understanding of intuitive physics is the complexity of real-world environments. Traditional AI models often rely on large datasets and predefined rules to make predictions, which can limit their ability to generalize across different scenarios. However, by incorporating principles from developmental psychology, researchers can design models that learn from experience in a manner akin to human infants. This approach allows AI systems to develop a more flexible and robust understanding of physical interactions.
To achieve this, researchers have begun to integrate concepts such as curiosity-driven learning and exploration into AI models. These concepts, rooted in developmental psychology, emphasize the importance of self-directed exploration in acquiring knowledge. By encouraging AI systems to actively explore their environments, researchers can facilitate the development of intuitive physics models that are more adaptable and capable of handling novel situations. This method not only enhances the AI’s ability to predict physical outcomes but also improves its capacity for problem-solving in dynamic environments.
Moreover, the use of developmental psychology insights in AI research has led to the creation of models that can simulate human-like reasoning processes. For instance, some deep learning models are now capable of performing tasks that require an understanding of cause-and-effect relationships, a fundamental aspect of intuitive physics. By mimicking the way humans infer causality from observed events, these models can make more accurate predictions about future states of the world. This capability is particularly valuable in applications such as robotics, where understanding the consequences of actions is crucial for effective decision-making.
In addition to improving AI’s understanding of physics, the integration of developmental psychology insights also contributes to the broader goal of creating more human-like AI systems. By emulating the cognitive processes that underlie human learning, researchers can develop AI models that are not only more efficient but also more relatable and intuitive for human users. This alignment with human cognitive processes enhances the potential for AI systems to collaborate with humans in various domains, from education to healthcare.
In conclusion, the role of developmental psychology in enhancing AI’s understanding of physics is a promising area of research that bridges the gap between human cognition and artificial intelligence. By drawing on insights from how humans naturally learn about the world, researchers can design AI systems that are more adept at navigating complex environments and making informed decisions. As this interdisciplinary collaboration continues to evolve, it holds the potential to significantly advance the capabilities of AI, bringing us closer to the development of truly intelligent systems.
Emulating Human Intuition: Deep Learning Models and Physics
In recent years, the intersection of artificial intelligence and cognitive science has led to groundbreaking advancements in the development of deep learning models that emulate human intuition, particularly in understanding intuitive physics. This fascinating convergence draws inspiration from developmental psychology, which provides insights into how humans, even from infancy, develop an innate understanding of the physical world. By leveraging these insights, researchers are crafting models that not only mimic human-like reasoning but also enhance the capabilities of machines in processing and interpreting complex physical interactions.
The concept of intuitive physics refers to the human ability to predict and understand the behavior of objects in the physical world without formal training. This includes anticipating how objects will move, interact, or change over time based on their properties and the forces acting upon them. Developmental psychology has long studied how infants and young children acquire this understanding, revealing that even at a very young age, humans possess a rudimentary grasp of concepts such as gravity, object permanence, and causality. These insights have become invaluable in informing the design of deep learning models that aim to replicate similar intuitive processes.
Deep learning, a subset of machine learning, involves the use of neural networks with multiple layers to analyze and interpret data. These models have shown remarkable success in various domains, from image and speech recognition to natural language processing. However, emulating human intuition, particularly in the realm of physics, presents unique challenges. Traditional models often rely on vast amounts of data and explicit programming to make predictions, which contrasts with the more fluid and adaptive nature of human intuition. To bridge this gap, researchers are turning to developmental psychology to understand the underlying mechanisms of intuitive physics and incorporate these principles into model design.
One approach involves training deep learning models using environments that simulate physical interactions, allowing them to learn through observation and experimentation, much like a child exploring their surroundings. By exposing models to a wide range of scenarios, they can begin to develop an understanding of physical laws and principles, such as momentum and energy conservation, through pattern recognition and generalization. This method not only enhances the model’s predictive capabilities but also enables it to adapt to new and unfamiliar situations, mirroring the flexibility of human intuition.
Moreover, incorporating insights from developmental psychology into model architecture can lead to more efficient learning processes. For instance, researchers are exploring the use of attention mechanisms, which mimic the human ability to focus on relevant aspects of a scene while ignoring extraneous information. This allows models to prioritize critical features and relationships within a given context, thereby improving their ability to make accurate predictions about physical interactions.
The implications of these advancements are far-reaching, with potential applications spanning robotics, autonomous vehicles, and virtual reality, among others. By endowing machines with a more human-like understanding of physics, we can create systems that interact more naturally and effectively with the world around them. This not only enhances their functionality but also paves the way for more intuitive human-machine collaboration.
In conclusion, the integration of developmental psychology insights into deep learning models represents a significant step forward in emulating human intuition, particularly in the realm of intuitive physics. By drawing on the innate abilities that humans develop from infancy, researchers are crafting models that not only replicate but also expand upon these capabilities, leading to more adaptive and intelligent systems. As this field continues to evolve, it holds the promise of unlocking new possibilities in artificial intelligence, ultimately bringing us closer to machines that think and reason like humans.
Insights from Developmental Psychology: Transforming AI’s Approach to Physics
In recent years, the field of artificial intelligence has witnessed remarkable advancements, particularly in the realm of deep learning. One of the most intriguing developments is the application of insights from developmental psychology to enhance AI’s understanding of intuitive physics. This interdisciplinary approach seeks to emulate the way humans, especially infants, perceive and interact with the physical world. By integrating principles from developmental psychology, researchers aim to create deep learning models that can grasp the fundamental concepts of physics in a manner akin to human intuition.
To begin with, it is essential to understand the concept of intuitive physics. This term refers to the innate ability of humans to predict and understand the behavior of objects in their environment. From a young age, humans develop an understanding of basic physical principles such as gravity, object permanence, and causality. These intuitive insights allow individuals to navigate the world effectively, making predictions about how objects will move or interact. Developmental psychology has long studied these phenomena, providing valuable insights into how humans acquire and refine their understanding of physics.
Incorporating these insights into AI models involves mimicking the developmental stages through which humans acquire intuitive physics. Researchers have observed that infants learn about the physical world through exploration and interaction. They experiment with objects, observe outcomes, and gradually build a mental model of how the world operates. By simulating this learning process, AI models can be trained to develop a similar understanding of physics. This approach contrasts with traditional methods that rely heavily on pre-programmed rules and equations, offering a more flexible and adaptive framework.
Moreover, the integration of developmental psychology into AI models has the potential to address some of the limitations of current deep learning systems. Traditional models often struggle with tasks that require an understanding of physical interactions, such as predicting the trajectory of a bouncing ball or understanding the stability of a stack of blocks. By emulating the human learning process, AI systems can develop a more nuanced understanding of these tasks, leading to improved performance and generalization across a wider range of scenarios.
Furthermore, this interdisciplinary approach fosters a deeper understanding of the cognitive processes underlying human intuition. By studying how AI models learn and apply intuitive physics, researchers can gain insights into the mechanisms that drive human cognition. This reciprocal relationship between AI and psychology not only enhances the capabilities of artificial systems but also contributes to our understanding of human intelligence.
In addition to improving AI’s performance, the application of developmental psychology insights has broader implications for the field of robotics. Robots equipped with an intuitive understanding of physics can interact more naturally with their environment, leading to advancements in areas such as autonomous navigation, object manipulation, and human-robot interaction. This progress could pave the way for more sophisticated and versatile robotic systems capable of performing complex tasks in dynamic and unpredictable settings.
In conclusion, the integration of developmental psychology insights into deep learning models represents a significant step forward in the quest to emulate human-like understanding of intuitive physics. By drawing inspiration from the way humans learn and interact with the physical world, researchers are developing AI systems that are more adaptable, efficient, and capable of tackling complex tasks. This interdisciplinary approach not only enhances the capabilities of artificial intelligence but also deepens our understanding of the cognitive processes that underpin human intuition, ultimately bridging the gap between human and machine intelligence.
Q&A
1. **What is the main focus of the research on deep learning models emulating intuitive physics?**
The research focuses on developing deep learning models that can mimic human-like intuitive physics by incorporating insights from developmental psychology, aiming to understand and predict physical interactions in the environment as humans do.
2. **How do developmental psychology insights contribute to these models?**
Developmental psychology provides insights into how humans, especially infants, develop an understanding of physical properties and interactions, which can be used to inform the design and training of deep learning models to improve their intuitive physics capabilities.
3. **What are some key features of intuitive physics that these models aim to replicate?**
Key features include the ability to predict object trajectories, understand object permanence, and recognize causal relationships between physical events, similar to how humans naturally perceive and interact with the physical world.
4. **What are the potential applications of deep learning models with intuitive physics capabilities?**
Potential applications include robotics, where robots can better interact with their environment, virtual reality systems that simulate realistic physical interactions, and enhanced AI systems for scientific research and education.
5. **What challenges do researchers face in developing these models?**
Challenges include creating models that generalize well across different physical scenarios, ensuring computational efficiency, and integrating complex psychological insights into the model architecture and training processes.
6. **How do these models differ from traditional physics engines?**
Unlike traditional physics engines that rely on predefined rules and equations, these deep learning models aim to learn and infer physical principles from data, allowing for more flexible and adaptive understanding of novel physical situations.The integration of developmental psychology insights into deep learning models to emulate intuitive physics represents a significant advancement in artificial intelligence. By mimicking the cognitive processes observed in human infants, these models can better understand and predict physical interactions in the environment. This approach not only enhances the model’s ability to generalize from limited data but also aligns its learning trajectory with human-like reasoning patterns. Consequently, this fusion of psychology and AI fosters the development of more robust and adaptable systems, capable of performing complex tasks with a level of intuition previously unattainable by traditional machine learning methods.
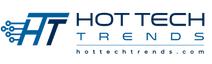