Manipulated images, often crafted through techniques like Photoshop or advanced AI-driven tools, have a profound impact on both machine vision systems and human perception. In the realm of machine vision, these altered images can deceive algorithms designed for tasks such as facial recognition, object detection, and scene understanding, leading to errors and biases in automated decision-making processes. For humans, manipulated images can distort reality, influence opinions, and shape beliefs by presenting false or misleading visual information. This dual impact underscores the critical need for robust detection methods and ethical guidelines to address the challenges posed by image manipulation in an increasingly digital world.
Impact Of Manipulated Images On Machine Learning Algorithms
In recent years, the proliferation of manipulated images has posed significant challenges not only to human perception but also to the integrity of machine learning algorithms. As digital technology advances, the ease with which images can be altered has increased, leading to widespread implications across various domains. The impact of these manipulated images is profound, affecting both the accuracy of machine vision systems and the way humans interpret visual information.
To begin with, machine learning algorithms, particularly those used in image recognition and computer vision, rely heavily on large datasets to learn and make accurate predictions. These datasets are expected to contain authentic and representative samples of the real world. However, when manipulated images infiltrate these datasets, they introduce noise and bias, which can significantly degrade the performance of these algorithms. For instance, an algorithm trained on a dataset containing doctored images may struggle to distinguish between genuine and altered content, leading to erroneous outputs. This is particularly concerning in critical applications such as autonomous vehicles, medical imaging, and security systems, where the cost of errors can be substantial.
Moreover, the presence of manipulated images in training datasets can lead to the development of biased models. These models may inadvertently learn to recognize and prioritize features that are not representative of real-world scenarios, thus perpetuating inaccuracies. As a result, the reliability of machine vision systems is compromised, necessitating the development of more robust algorithms capable of detecting and mitigating the effects of image manipulation. Researchers are actively exploring techniques such as adversarial training and anomaly detection to enhance the resilience of machine learning models against such perturbations.
In parallel, the impact of manipulated images on human perception cannot be overlooked. Humans are naturally inclined to trust visual information, often perceiving images as accurate representations of reality. However, with the increasing sophistication of image editing tools, it has become more challenging for individuals to discern between authentic and altered images. This has significant implications for areas such as journalism, social media, and public discourse, where manipulated images can be used to mislead and manipulate public opinion. The spread of misinformation through doctored images can exacerbate societal issues, leading to polarization and mistrust.
Furthermore, the psychological effects of manipulated images on human perception are profound. Studies have shown that repeated exposure to altered images can alter an individual’s memory and perception of events, leading to the formation of false memories. This phenomenon underscores the need for greater awareness and education regarding the potential for image manipulation and its effects on perception.
In response to these challenges, there is a growing emphasis on developing tools and techniques to detect and counteract image manipulation. Advances in forensic analysis and machine learning are being leveraged to create algorithms capable of identifying inconsistencies and anomalies in images, thereby aiding in the verification of their authenticity. Additionally, initiatives aimed at educating the public about the prevalence and impact of manipulated images are crucial in fostering a more discerning and informed society.
In conclusion, the impact of manipulated images on both machine learning algorithms and human perception is a multifaceted issue that requires concerted efforts from researchers, technologists, and educators. As technology continues to evolve, it is imperative to develop robust solutions that can safeguard the integrity of visual information and ensure that both machines and humans can navigate the digital landscape with confidence and accuracy.
How Altered Visuals Influence Human Cognitive Biases
In an era where digital imagery is omnipresent, the manipulation of images has become a significant concern, affecting both machine vision systems and human perception. The alteration of visuals, whether through subtle retouching or more overt modifications, can profoundly influence cognitive biases, shaping the way individuals interpret and respond to visual information. As technology advances, the line between authentic and manipulated images becomes increasingly blurred, raising questions about the reliability of visual media and its impact on human cognition.
To begin with, it is essential to understand how manipulated images can influence human perception. Visuals are a powerful medium for communication, often evoking emotional responses and shaping opinions. When images are altered, they can create misleading narratives, leading viewers to form biased interpretations. For instance, the enhancement of certain features in a photograph can exaggerate reality, prompting viewers to perceive the subject in a way that aligns with the manipulator’s intent. This can reinforce existing stereotypes or create new ones, as individuals tend to rely on visual cues to make quick judgments.
Moreover, the impact of manipulated images extends beyond individual perception to influence societal attitudes and beliefs. In the context of social media, where images are rapidly disseminated and consumed, altered visuals can contribute to the spread of misinformation. This phenomenon is particularly concerning in the realm of news and politics, where doctored images can sway public opinion and affect democratic processes. The ease with which images can be shared and reshaped online amplifies their potential to mislead, making it crucial for viewers to critically assess the authenticity of the visuals they encounter.
In addition to affecting human perception, manipulated images pose challenges for machine vision systems. These systems, which rely on algorithms to interpret visual data, can be easily deceived by altered images. For example, adversarial attacks, where subtle changes are made to an image to confuse machine learning models, can lead to incorrect classifications or identifications. This vulnerability highlights the need for robust algorithms capable of detecting and mitigating the effects of image manipulation. As machine vision becomes increasingly integrated into various sectors, from autonomous vehicles to security systems, ensuring the accuracy and reliability of these technologies is paramount.
Transitioning to the cognitive biases influenced by manipulated images, it is important to consider how these biases affect decision-making processes. Cognitive biases are systematic patterns of deviation from norm or rationality in judgment, often resulting from the brain’s attempt to simplify information processing. When individuals are exposed to manipulated images, their cognitive biases can be exacerbated, leading to skewed perceptions and decisions. For instance, the confirmation bias, where individuals favor information that confirms their preexisting beliefs, can be intensified by images that have been altered to align with those beliefs. This can hinder critical thinking and perpetuate misinformation.
In conclusion, the manipulation of images has far-reaching implications for both human perception and machine vision. As digital imagery continues to play a central role in communication and information dissemination, it is imperative to develop strategies to identify and counteract the effects of altered visuals. Educating individuals about the potential biases introduced by manipulated images and enhancing the capabilities of machine vision systems to detect such alterations are crucial steps in addressing this challenge. By fostering a more discerning approach to visual media, society can mitigate the impact of manipulated images on cognitive biases and ensure a more informed and objective interpretation of the world.
The Role Of Deepfakes In Shaping Public Opinion
In recent years, the proliferation of deepfake technology has raised significant concerns about its impact on both machine vision systems and human perception. Deepfakes, which utilize artificial intelligence to create hyper-realistic manipulated images and videos, have the potential to significantly influence public opinion. As these technologies become more sophisticated, the line between reality and fabrication blurs, posing challenges for both automated systems and human observers in discerning authenticity.
To begin with, deepfakes present a formidable challenge to machine vision systems, which are increasingly relied upon for tasks ranging from security surveillance to content moderation on social media platforms. These systems, designed to recognize patterns and identify objects, can be easily deceived by manipulated images that mimic real-world scenarios. For instance, a deepfake video of a public figure making controversial statements can be indistinguishable from genuine footage to an algorithm trained on traditional datasets. This vulnerability not only undermines the reliability of machine vision but also raises the stakes for developers to enhance detection capabilities.
Moreover, the implications of deepfakes extend beyond machine vision, affecting human perception in profound ways. Humans, despite their cognitive abilities, are not immune to the persuasive power of manipulated media. Deepfakes can exploit cognitive biases, such as the tendency to believe information that confirms pre-existing beliefs, thereby shaping public opinion in subtle yet impactful ways. For example, a deepfake video that aligns with a viewer’s political stance can reinforce their beliefs, even if the content is entirely fabricated. This phenomenon underscores the potential of deepfakes to polarize societies by amplifying misinformation and eroding trust in legitimate sources.
Furthermore, the role of deepfakes in shaping public opinion is exacerbated by the rapid dissemination of content through social media platforms. In an era where information spreads at an unprecedented pace, deepfakes can quickly reach a wide audience, making it challenging to contain their influence once they go viral. The virality of such content can lead to real-world consequences, including reputational damage, political unrest, and even threats to national security. Consequently, the need for effective countermeasures becomes increasingly urgent.
In response to these challenges, researchers and technologists are actively developing tools to detect and mitigate the impact of deepfakes. Advances in machine learning and computer vision are being leveraged to create algorithms capable of identifying subtle inconsistencies in manipulated media. However, the arms race between deepfake creators and detection technologies continues, as each advancement in detection is met with more sophisticated methods of evasion. This ongoing battle highlights the necessity for a multi-faceted approach that combines technological solutions with public awareness and education.
In conclusion, the rise of deepfakes represents a significant threat to both machine vision systems and human perception, with far-reaching implications for public opinion. As these technologies continue to evolve, it is imperative to address the challenges they pose through a combination of technological innovation and societal engagement. By fostering a more informed public and developing robust detection mechanisms, it is possible to mitigate the impact of deepfakes and preserve the integrity of information in the digital age. The responsibility lies not only with technologists and policymakers but also with individuals to critically evaluate the media they consume, ensuring that truth prevails in an increasingly complex information landscape.
Challenges In Detecting Manipulated Images In AI Systems
The proliferation of manipulated images in the digital age presents significant challenges for both machine vision systems and human perception. As technology advances, the ability to alter images with precision and subtlety has become increasingly accessible, leading to a surge in the creation and dissemination of manipulated content. This phenomenon poses a dual challenge: it complicates the task of developing robust AI systems capable of detecting such alterations, and it also affects human perception, often leading to misinformation and distorted realities.
Machine vision systems, which rely on algorithms to interpret visual data, face considerable hurdles in identifying manipulated images. These systems are typically trained on large datasets to recognize patterns and features within images. However, the sophistication of modern image manipulation techniques, such as deepfakes and generative adversarial networks (GANs), can deceive even the most advanced algorithms. These techniques can produce images that are nearly indistinguishable from authentic ones, making it difficult for AI systems to detect anomalies. Consequently, researchers are continually striving to enhance the capabilities of machine vision systems by developing more sophisticated algorithms and incorporating techniques such as anomaly detection and adversarial training.
In addition to the technical challenges, the issue of manipulated images also has profound implications for human perception. The human brain is wired to trust visual information, often accepting images as truthful representations of reality. This inherent trust can be exploited by manipulated images, leading to the spread of misinformation and the reinforcement of false narratives. The impact of such images is particularly pronounced in the context of social media, where they can be rapidly disseminated to a wide audience, influencing public opinion and even affecting political outcomes. As a result, there is a growing need for public awareness and education on the potential for image manipulation and the importance of critical evaluation of visual content.
Moreover, the interplay between machine vision and human perception in the context of manipulated images is complex and multifaceted. On one hand, advancements in AI technology hold the promise of developing tools that can assist humans in identifying manipulated content, thereby enhancing our ability to discern truth from falsehood. On the other hand, the very same technologies that are used to detect manipulations can also be employed to create more convincing fakes, perpetuating a cycle of deception and detection. This dynamic underscores the importance of ongoing research and collaboration between technologists, ethicists, and policymakers to address the ethical and societal implications of image manipulation.
In conclusion, the challenges posed by manipulated images are significant and multifaceted, affecting both machine vision systems and human perception. As technology continues to evolve, it is imperative that we develop more sophisticated AI systems capable of detecting image manipulations while also fostering a more informed and discerning public. By addressing these challenges through a combination of technological innovation and public education, we can mitigate the impact of manipulated images and preserve the integrity of visual information in the digital age. The path forward requires a concerted effort from all stakeholders to ensure that the benefits of technological advancements are harnessed responsibly and ethically, safeguarding both machine and human perception from the distortions of manipulated imagery.
Psychological Effects Of Image Manipulation On Human Perception
In an era where digital imagery is omnipresent, the manipulation of images has become a common practice, influencing both machine vision systems and human perception. The psychological effects of image manipulation on human perception are profound, as they shape our understanding of reality and influence our decision-making processes. As technology advances, the line between authentic and altered images becomes increasingly blurred, raising concerns about the implications for both individuals and society at large.
To begin with, image manipulation can significantly alter human perception by distorting reality. When individuals are exposed to manipulated images, their ability to discern truth from fiction is compromised. This is particularly concerning in the context of news media and social platforms, where images are often used to convey information quickly and effectively. Manipulated images can lead to the spread of misinformation, as viewers may accept altered visuals as factual representations. This can result in skewed perceptions of events, people, and places, ultimately affecting public opinion and behavior.
Moreover, the psychological impact of manipulated images extends to self-perception and body image. In the realm of advertising and social media, images are frequently edited to present idealized versions of beauty and success. This can lead to unrealistic standards and expectations, causing individuals to feel inadequate or dissatisfied with their own appearances. The constant exposure to such images can contribute to issues like low self-esteem, body dysmorphia, and eating disorders, particularly among impressionable demographics such as teenagers and young adults.
In addition to affecting human perception, manipulated images pose challenges for machine vision systems. These systems, which rely on algorithms to interpret visual data, can be easily deceived by altered images. For instance, adversarial attacks, which involve making subtle changes to images that are imperceptible to the human eye, can cause machine vision systems to misclassify objects or fail to recognize them altogether. This vulnerability has significant implications for applications such as autonomous vehicles, facial recognition, and security systems, where accuracy is paramount.
Furthermore, the interplay between human perception and machine vision is complex, as each influences the other. As machine vision systems become more integrated into daily life, they shape the way humans perceive and interact with the world. For example, the use of filters and editing tools on social media platforms not only affects how users present themselves but also how they perceive others. This creates a feedback loop where manipulated images influence human perception, which in turn affects the development and deployment of machine vision technologies.
To address the psychological effects of image manipulation on human perception, it is crucial to promote media literacy and critical thinking skills. Educating individuals about the prevalence and impact of manipulated images can empower them to question and analyze the visuals they encounter. Additionally, advancements in technology can aid in the detection of altered images, providing tools for both humans and machines to discern authenticity.
In conclusion, the manipulation of images has far-reaching effects on both human perception and machine vision. As digital imagery continues to evolve, it is essential to remain vigilant about the potential consequences of altered visuals. By fostering awareness and developing robust detection methods, society can mitigate the psychological impact of image manipulation and preserve the integrity of visual information.
Ethical Implications Of Image Manipulation In Media And Technology
The advent of sophisticated image manipulation technologies has significantly transformed the landscape of media and technology, raising profound ethical implications. As digital tools become increasingly advanced, the line between reality and fabrication blurs, affecting both machine vision systems and human perception. This dual impact necessitates a closer examination of the ethical considerations surrounding manipulated images.
To begin with, the influence of manipulated images on machine vision systems cannot be overstated. Machine vision, which relies on algorithms to interpret visual data, is susceptible to errors when fed altered images. These systems, employed in various applications such as facial recognition, autonomous vehicles, and medical diagnostics, depend on the integrity of visual inputs to function accurately. When images are manipulated, the algorithms may produce flawed outputs, leading to potentially dangerous consequences. For instance, in the realm of autonomous vehicles, altered images could mislead the system into misinterpreting road signs or obstacles, posing a risk to public safety. Therefore, ensuring the authenticity of images used in machine vision is paramount to maintaining the reliability of these technologies.
Simultaneously, the manipulation of images has a profound effect on human perception, shaping public opinion and influencing societal norms. In the media, altered images can be used to misrepresent facts, sway public sentiment, or perpetuate stereotypes. This manipulation can lead to misinformation, eroding trust in media sources and contributing to the spread of fake news. Moreover, the prevalence of digitally altered images in advertising and social media can distort perceptions of reality, fostering unrealistic standards of beauty and success. This can have detrimental effects on mental health, particularly among impressionable audiences who may strive to emulate these unattainable ideals.
The ethical implications of image manipulation extend beyond the immediate effects on machine vision and human perception. They also raise questions about accountability and transparency. As images become easier to alter, determining the authenticity of visual content becomes increasingly challenging. This necessitates the development of robust verification methods to distinguish between genuine and manipulated images. Furthermore, there is a pressing need for ethical guidelines and regulations to govern the use of image manipulation technologies. These guidelines should address issues such as consent, disclosure, and the potential harm caused by altered images.
In addition, the responsibility of media outlets and technology companies in mitigating the negative impacts of image manipulation cannot be overlooked. These entities play a crucial role in shaping public discourse and have a duty to uphold ethical standards. By implementing policies that promote transparency and accuracy, they can help restore public trust and ensure that manipulated images do not undermine the integrity of information.
In conclusion, the ethical implications of image manipulation in media and technology are multifaceted, affecting both machine vision systems and human perception. As these technologies continue to evolve, it is imperative to address the challenges they pose through the development of ethical guidelines, robust verification methods, and responsible practices by media and technology companies. By doing so, we can harness the benefits of image manipulation technologies while minimizing their potential harms, ultimately fostering a more informed and discerning society.
Q&A
1. **What are manipulated images?**
Manipulated images are photographs or graphics that have been digitally altered or edited to change their content, appearance, or context, often using software like Photoshop.
2. **How do manipulated images affect machine vision?**
Manipulated images can deceive machine vision systems by altering features that these systems rely on for recognition and classification, leading to incorrect outputs or decisions.
3. **In what ways do manipulated images impact human perception?**
Manipulated images can mislead human perception by presenting false or distorted visual information, which can influence beliefs, opinions, and decision-making processes.
4. **What are some common techniques used in image manipulation?**
Common techniques include cropping, color adjustment, retouching, compositing, and the use of filters or effects to alter the image’s original content.
5. **Why is it important to detect manipulated images?**
Detecting manipulated images is crucial to prevent misinformation, protect privacy, maintain trust in media, and ensure the integrity of visual data used in various applications.
6. **What tools or methods are used to identify manipulated images?**
Tools and methods include digital forensics techniques, machine learning algorithms, and software designed to analyze inconsistencies in lighting, shadows, metadata, and pixel-level anomalies.Manipulated images significantly impact both machine vision and human perception by distorting reality and influencing decision-making processes. For machine vision, altered images can lead to misclassification, errors in object detection, and compromised algorithmic integrity, undermining the reliability of automated systems. For human perception, these images can shape beliefs, alter memories, and affect judgments, often leading to misinformation and cognitive biases. The dual impact on both domains underscores the need for advanced detection technologies and critical media literacy to mitigate the effects of image manipulation.
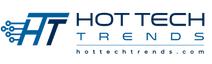