Creating trustworthy AI solutions for healthcare is a critical endeavor that combines cutting-edge technology with ethical responsibility to enhance patient care and improve health outcomes. As AI continues to revolutionize the healthcare industry, it is essential to ensure that these solutions are reliable, transparent, and aligned with the highest standards of patient safety and privacy. Trustworthy AI in healthcare involves rigorous validation and testing, adherence to regulatory standards, and the incorporation of ethical considerations to prevent biases and ensure equitable access to care. By fostering collaboration among technologists, healthcare professionals, and policymakers, we can develop AI systems that not only advance medical research and diagnostics but also earn the trust of patients and practitioners alike. This approach promises to unlock the full potential of AI in transforming healthcare delivery while safeguarding the fundamental principles of medical ethics and patient rights.
Ensuring Data Privacy and Security in AI Healthcare Solutions
In the rapidly evolving landscape of healthcare, artificial intelligence (AI) has emerged as a transformative force, offering unprecedented opportunities to enhance patient care, streamline operations, and improve diagnostic accuracy. However, as AI solutions become increasingly integrated into healthcare systems, ensuring data privacy and security has become a paramount concern. The sensitive nature of healthcare data, coupled with the potential risks associated with data breaches, necessitates robust measures to protect patient information while leveraging AI’s capabilities.
To begin with, the foundation of trustworthy AI solutions in healthcare lies in the implementation of stringent data privacy protocols. Healthcare organizations must adhere to established regulations such as the Health Insurance Portability and Accountability Act (HIPAA) in the United States or the General Data Protection Regulation (GDPR) in Europe. These regulations provide a framework for safeguarding patient data, ensuring that AI systems are designed with privacy by default. By embedding privacy considerations into the development process, organizations can mitigate risks and build trust with patients and stakeholders.
Moreover, the adoption of advanced encryption techniques is crucial in protecting healthcare data from unauthorized access. Encryption ensures that data is transformed into a secure format that can only be accessed by authorized individuals. This is particularly important when data is transmitted between different systems or stored in cloud environments. By employing robust encryption methods, healthcare providers can prevent data breaches and maintain the confidentiality of patient information.
In addition to encryption, implementing access controls is essential for maintaining data security. Access controls determine who can view or modify data within an AI system, ensuring that only authorized personnel have the necessary permissions. Role-based access control (RBAC) is a widely used approach that assigns access rights based on an individual’s role within the organization. This minimizes the risk of data exposure by limiting access to sensitive information to those who require it for their specific duties.
Furthermore, the use of anonymization and de-identification techniques can enhance data privacy in AI healthcare solutions. Anonymization involves removing personally identifiable information (PII) from datasets, rendering them untraceable to individual patients. De-identification, on the other hand, involves modifying data to prevent the identification of individuals while retaining its utility for analysis. These techniques enable healthcare organizations to utilize large datasets for AI training and research without compromising patient privacy.
Another critical aspect of ensuring data privacy and security in AI healthcare solutions is conducting regular audits and assessments. By continuously monitoring AI systems for vulnerabilities and compliance with privacy regulations, organizations can identify potential risks and implement corrective measures promptly. Regular audits also demonstrate a commitment to transparency and accountability, fostering trust among patients and stakeholders.
Moreover, fostering a culture of privacy and security awareness within healthcare organizations is vital. Training programs and workshops can educate staff about the importance of data protection and the role they play in maintaining it. By promoting a culture of vigilance and responsibility, organizations can reduce the likelihood of human errors that may lead to data breaches.
In conclusion, as AI continues to revolutionize healthcare, ensuring data privacy and security remains a critical challenge. By adhering to regulatory frameworks, employing advanced encryption and access controls, utilizing anonymization techniques, conducting regular audits, and fostering a culture of awareness, healthcare organizations can create trustworthy AI solutions. These measures not only protect patient information but also build confidence in AI technologies, ultimately paving the way for a more secure and efficient healthcare system.
Implementing Ethical Guidelines for AI in Healthcare
Implementing ethical guidelines for AI in healthcare is a critical endeavor that requires a multifaceted approach, ensuring that these advanced technologies are both effective and trustworthy. As artificial intelligence continues to revolutionize the healthcare industry, it is imperative to address the ethical considerations that accompany its integration. This involves not only understanding the potential benefits but also recognizing the challenges and risks associated with AI applications in healthcare settings.
To begin with, the development of AI solutions in healthcare must prioritize patient safety and privacy. Ensuring that AI systems are designed with robust security measures is essential to protect sensitive patient data from breaches and unauthorized access. This involves implementing encryption protocols and access controls, as well as regularly updating systems to address potential vulnerabilities. Moreover, transparency in data usage and AI decision-making processes is crucial. Patients and healthcare providers should be informed about how data is collected, stored, and utilized, fostering trust and confidence in AI technologies.
In addition to data security, fairness and equity are fundamental ethical principles that must be upheld in AI healthcare solutions. AI systems should be designed to avoid biases that could lead to unequal treatment of patients based on race, gender, socioeconomic status, or other factors. This requires a comprehensive approach to data collection and analysis, ensuring that datasets are representative and inclusive. By doing so, AI can help reduce disparities in healthcare access and outcomes, promoting a more equitable healthcare system.
Furthermore, accountability is a key component of ethical AI implementation in healthcare. Developers and healthcare providers must establish clear lines of responsibility for AI systems’ actions and decisions. This includes creating mechanisms for monitoring and evaluating AI performance, as well as establishing protocols for addressing errors or adverse outcomes. By holding AI systems accountable, stakeholders can ensure that these technologies are used responsibly and effectively.
Another important aspect of implementing ethical guidelines for AI in healthcare is fostering collaboration among various stakeholders. This includes engaging with healthcare professionals, patients, policymakers, and technology developers to create a shared understanding of ethical considerations and best practices. Collaborative efforts can lead to the development of comprehensive guidelines and standards that reflect the diverse perspectives and needs of all parties involved. Such collaboration can also facilitate the continuous improvement of AI systems, as feedback from different stakeholders can inform future iterations and enhancements.
Moreover, education and training play a vital role in the ethical implementation of AI in healthcare. Healthcare professionals must be equipped with the knowledge and skills to effectively use AI technologies, understanding both their capabilities and limitations. This involves providing training programs that cover the ethical implications of AI, as well as practical guidance on integrating these tools into clinical practice. By empowering healthcare providers with the necessary expertise, the healthcare industry can maximize the benefits of AI while minimizing potential risks.
In conclusion, implementing ethical guidelines for AI in healthcare is a complex but essential task that requires a comprehensive and collaborative approach. By prioritizing patient safety, fairness, accountability, and education, stakeholders can create trustworthy AI solutions that enhance healthcare delivery and improve patient outcomes. As AI continues to evolve, ongoing efforts to address ethical considerations will be crucial in ensuring that these technologies are used responsibly and effectively, ultimately benefiting patients and healthcare systems worldwide.
Building Transparent AI Models for Medical Applications
In the rapidly evolving landscape of healthcare, the integration of artificial intelligence (AI) presents both unprecedented opportunities and significant challenges. As AI technologies become increasingly embedded in medical applications, the need for transparency in AI models has become paramount. Transparency is not merely a technical requirement; it is a foundational element that fosters trust among healthcare professionals, patients, and regulatory bodies. To build trustworthy AI solutions, it is essential to focus on creating transparent AI models that can be easily understood and evaluated by all stakeholders involved.
One of the primary reasons transparency is crucial in AI models for healthcare is the need for accountability. In medical applications, decisions made by AI systems can have profound implications on patient outcomes. Therefore, it is imperative that these systems operate in a manner that is both explainable and justifiable. By ensuring that AI models are transparent, healthcare providers can better understand the decision-making processes of these systems, thereby facilitating more informed and responsible use. This understanding is particularly important in scenarios where AI models are used to assist in diagnosis or treatment planning, as it allows clinicians to verify and validate the recommendations provided by the AI.
Moreover, transparency in AI models enhances the ability to identify and mitigate biases that may exist within the system. Bias in AI can arise from various sources, including the data used to train the models and the algorithms themselves. In the context of healthcare, biased AI systems can lead to disparities in treatment and care, disproportionately affecting certain patient groups. By building transparent AI models, developers and healthcare professionals can scrutinize the data and algorithms, ensuring that biases are identified and addressed. This proactive approach not only improves the fairness of AI systems but also strengthens the trust of patients and healthcare providers in these technologies.
In addition to accountability and bias mitigation, transparency in AI models also facilitates compliance with regulatory standards. Healthcare is a highly regulated industry, with stringent requirements for the safety and efficacy of medical technologies. Transparent AI models allow for easier auditing and assessment by regulatory bodies, ensuring that these systems meet the necessary standards for deployment in clinical settings. This compliance is essential for the widespread adoption of AI in healthcare, as it provides assurance to both providers and patients that the technology is safe and reliable.
Furthermore, transparency fosters collaboration and innovation within the healthcare ecosystem. When AI models are transparent, they can be more easily shared and improved upon by researchers and developers. This openness encourages collaboration across institutions and disciplines, leading to the development of more robust and effective AI solutions. By promoting a culture of transparency, the healthcare industry can harness the collective expertise of its stakeholders to drive innovation and improve patient care.
In conclusion, building transparent AI models for medical applications is a critical step towards creating trustworthy AI solutions in healthcare. Transparency ensures accountability, mitigates bias, facilitates regulatory compliance, and fosters collaboration and innovation. As AI continues to transform the healthcare landscape, it is essential that these technologies are developed and implemented with a focus on transparency, ensuring that they serve the best interests of patients and healthcare providers alike. By prioritizing transparency, the healthcare industry can fully realize the potential of AI to enhance patient outcomes and revolutionize medical practice.
Enhancing Patient-Provider Trust Through AI Technologies
In the rapidly evolving landscape of healthcare, the integration of artificial intelligence (AI) technologies has emerged as a transformative force, promising to enhance patient-provider trust. As healthcare systems worldwide grapple with increasing demands and complexities, AI offers innovative solutions that can streamline processes, improve diagnostic accuracy, and personalize patient care. However, the successful implementation of AI in healthcare hinges on the establishment of trust between patients and providers, a critical component that ensures the ethical and effective use of these technologies.
To begin with, AI technologies have the potential to significantly improve diagnostic accuracy, thereby fostering trust. By analyzing vast amounts of data with precision and speed, AI can assist healthcare providers in making more informed decisions. For instance, AI algorithms can detect patterns in medical imaging that may be imperceptible to the human eye, leading to earlier and more accurate diagnoses. This capability not only enhances the confidence of healthcare providers in their diagnostic processes but also reassures patients that they are receiving the most accurate assessments possible. Consequently, as patients witness the tangible benefits of AI in improving health outcomes, their trust in both the technology and their healthcare providers is likely to grow.
Moreover, AI can play a pivotal role in personalizing patient care, which is another crucial factor in building trust. By leveraging machine learning algorithms, AI can analyze individual patient data to tailor treatment plans that align with each patient’s unique needs and preferences. This personalized approach not only improves the efficacy of treatments but also empowers patients by involving them more directly in their healthcare journey. When patients feel that their specific circumstances are being considered and that they are active participants in their care, their trust in the healthcare system is strengthened.
In addition to enhancing diagnostic accuracy and personalizing care, AI technologies can also improve communication between patients and providers, further solidifying trust. AI-powered chatbots and virtual assistants can provide patients with timely information and support, answering questions and addressing concerns outside of traditional office hours. This increased accessibility ensures that patients feel supported and informed, reducing anxiety and fostering a sense of trust in their healthcare providers. Furthermore, AI can facilitate more efficient and transparent communication by organizing and presenting patient data in a clear and comprehensible manner, enabling providers to explain complex medical information more effectively.
However, while AI holds immense potential to enhance patient-provider trust, it is imperative to address the ethical considerations associated with its use. Ensuring data privacy and security is paramount, as patients must have confidence that their sensitive information is protected. Additionally, transparency in AI algorithms and decision-making processes is essential to avoid biases and ensure fairness. By prioritizing these ethical considerations, healthcare providers can build a foundation of trust that supports the responsible and equitable use of AI technologies.
In conclusion, the integration of AI technologies in healthcare presents a unique opportunity to enhance patient-provider trust. By improving diagnostic accuracy, personalizing care, and facilitating effective communication, AI can transform the healthcare experience for both patients and providers. However, the ethical implementation of these technologies is crucial to maintaining trust and ensuring that AI serves as a beneficial tool in the healthcare landscape. As we continue to navigate the complexities of AI in healthcare, fostering trust will remain a central focus, guiding the development of trustworthy AI solutions that prioritize patient well-being and provider integrity.
Validating AI Algorithms with Clinical Trials and Real-World Data
In the rapidly evolving landscape of healthcare, artificial intelligence (AI) has emerged as a transformative force, promising to enhance diagnostic accuracy, streamline administrative processes, and personalize patient care. However, the integration of AI into healthcare systems necessitates a rigorous validation process to ensure these technologies are both effective and trustworthy. Central to this validation process is the use of clinical trials and real-world data, which together provide a comprehensive framework for assessing the performance and safety of AI algorithms.
Clinical trials have long been the gold standard for evaluating new medical interventions, and their application to AI solutions is no exception. By systematically testing AI algorithms in controlled environments, clinical trials offer a structured approach to determine their efficacy and potential risks. These trials typically involve a series of phases, each designed to answer specific questions about the AI’s functionality and impact. Initially, small-scale pilot studies may be conducted to assess feasibility and refine the algorithm. Subsequently, larger trials are implemented to evaluate the AI’s performance against established benchmarks, often comparing it to existing diagnostic or treatment methods.
The use of clinical trials in validating AI algorithms is crucial for several reasons. Firstly, it provides empirical evidence of the algorithm’s capabilities, which is essential for gaining regulatory approval and clinician acceptance. Secondly, clinical trials help identify any unintended consequences or biases that may arise from the AI’s application, thereby ensuring patient safety. Moreover, these trials facilitate the collection of standardized data, which can be used to further refine and improve the algorithm.
While clinical trials are indispensable, they are not without limitations. They often involve a select group of participants and controlled conditions that may not fully represent the diversity and complexity of real-world healthcare settings. This is where real-world data becomes invaluable. By analyzing data from electronic health records, insurance claims, and patient registries, researchers can gain insights into how AI algorithms perform across different populations and clinical environments. Real-world data provides a broader perspective, capturing the variability and nuances that are often absent in clinical trials.
The integration of real-world data into the validation process complements the findings from clinical trials, offering a more holistic view of the AI’s effectiveness. It allows for the continuous monitoring of the algorithm’s performance post-deployment, enabling timely updates and adjustments. Furthermore, real-world data can uncover patterns and trends that may not be apparent in controlled trials, such as long-term outcomes and rare adverse events.
To create trustworthy AI solutions for healthcare, it is imperative to establish a robust validation framework that leverages both clinical trials and real-world data. This dual approach not only enhances the reliability of AI algorithms but also fosters confidence among healthcare providers and patients. As AI continues to advance, ongoing collaboration between technologists, clinicians, and regulatory bodies will be essential to ensure that these innovations are safe, effective, and equitable.
In conclusion, the validation of AI algorithms through clinical trials and real-world data is a critical step in their integration into healthcare. By combining the rigor of clinical trials with the breadth of real-world data, we can create AI solutions that are not only innovative but also trustworthy, ultimately improving patient outcomes and transforming the future of healthcare.
Addressing Bias and Fairness in AI-Driven Healthcare Systems
In the rapidly evolving landscape of healthcare, artificial intelligence (AI) has emerged as a transformative force, offering unprecedented opportunities to enhance patient care, streamline operations, and improve diagnostic accuracy. However, as AI-driven healthcare systems become more prevalent, addressing bias and ensuring fairness within these technologies is paramount. The integration of AI into healthcare must be approached with a commitment to creating trustworthy solutions that prioritize patient safety and equity.
Bias in AI systems often stems from the data used to train these models. In healthcare, this can lead to significant disparities in treatment outcomes, particularly for marginalized groups. For instance, if an AI system is trained predominantly on data from a specific demographic, it may not perform as effectively for individuals outside that group. This can result in misdiagnoses or inappropriate treatment recommendations, exacerbating existing health inequities. Therefore, it is crucial to ensure that the datasets used in developing AI healthcare solutions are diverse and representative of the entire population.
To address these challenges, developers and healthcare providers must collaborate to implement rigorous data collection and validation processes. By incorporating data from a wide range of sources, including different geographic regions, ethnicities, and socioeconomic backgrounds, AI systems can be trained to recognize and accommodate the diverse needs of all patients. Moreover, ongoing monitoring and evaluation of AI systems are essential to identify and rectify any biases that may emerge over time. This continuous feedback loop allows for the refinement of algorithms, ensuring they remain fair and effective.
In addition to data diversity, transparency in AI decision-making processes is vital for building trust in these technologies. Healthcare professionals and patients alike must understand how AI systems arrive at their conclusions. This can be achieved through the development of explainable AI models that provide clear, understandable insights into their decision-making processes. By demystifying AI operations, stakeholders can better assess the reliability and fairness of these systems, fostering greater confidence in their use.
Furthermore, ethical considerations must be at the forefront of AI development in healthcare. Establishing ethical guidelines and frameworks can help ensure that AI technologies are designed and implemented with a focus on patient welfare and equity. Engaging with ethicists, patient advocacy groups, and other stakeholders can provide valuable perspectives on the potential impacts of AI systems, guiding the creation of solutions that are both effective and just.
Collaboration between technology developers, healthcare providers, and regulatory bodies is also essential in addressing bias and fairness in AI-driven healthcare systems. Regulatory frameworks must evolve to keep pace with technological advancements, providing clear guidelines for the development and deployment of AI solutions. By working together, these entities can establish standards that promote fairness, accountability, and transparency, ultimately leading to more trustworthy AI systems.
In conclusion, as AI continues to revolutionize healthcare, addressing bias and ensuring fairness in these systems is critical. By prioritizing diverse data, transparency, ethical considerations, and collaborative efforts, stakeholders can create AI solutions that are not only innovative but also equitable and trustworthy. As we move forward, it is imperative to remain vigilant in our efforts to mitigate bias and promote fairness, ensuring that AI-driven healthcare systems benefit all individuals, regardless of their background or circumstances.
Q&A
1. **What is a key consideration in developing trustworthy AI for healthcare?**
Ensuring data privacy and security is a key consideration, as healthcare data is highly sensitive and must be protected against unauthorized access and breaches.
2. **How can bias be minimized in AI healthcare solutions?**
Bias can be minimized by using diverse and representative datasets during the training phase, and by continuously monitoring and adjusting algorithms to ensure fair and equitable outcomes.
3. **Why is transparency important in AI healthcare solutions?**
Transparency is crucial because it allows healthcare professionals to understand and trust the AI’s decision-making process, which is essential for clinical adoption and patient safety.
4. **What role does regulatory compliance play in trustworthy AI for healthcare?**
Regulatory compliance ensures that AI solutions meet established standards and guidelines, which helps in maintaining safety, efficacy, and ethical standards in healthcare applications.
5. **How can AI solutions be validated for accuracy in healthcare?**
AI solutions can be validated through rigorous testing and validation processes, including clinical trials and peer-reviewed studies, to ensure they provide accurate and reliable results.
6. **What is the importance of involving healthcare professionals in AI development?**
Involving healthcare professionals is important to ensure that AI solutions are practical, relevant, and aligned with clinical needs, ultimately leading to better integration and acceptance in healthcare settings.Creating trustworthy AI solutions for healthcare is essential to harness the full potential of technology while ensuring patient safety, privacy, and ethical standards. Trustworthy AI in healthcare must be built on a foundation of transparency, accountability, and fairness. This involves rigorous validation and testing of AI models, ensuring they are free from biases and can generalize across diverse patient populations. Data privacy and security must be prioritized, with robust measures in place to protect sensitive patient information. Collaboration between AI developers, healthcare professionals, and regulatory bodies is crucial to establish clear guidelines and standards. Continuous monitoring and updating of AI systems are necessary to maintain their reliability and effectiveness. By addressing these challenges, we can create AI solutions that not only enhance healthcare delivery but also earn the trust of patients and providers, ultimately leading to improved health outcomes and a more efficient healthcare system.
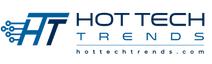