The rapid advancement of large language models (LLMs) has sparked significant interest and optimism regarding their potential to revolutionize various fields, from natural language processing to artificial intelligence. These models, with their impressive ability to generate human-like text, have been hailed as groundbreaking tools capable of understanding and reasoning about complex topics. However, this enthusiasm has also led to a tendency to overestimate their reasoning abilities. While LLMs excel at pattern recognition and can produce coherent and contextually relevant text, they often lack true comprehension and the ability to engage in genuine reasoning. This overestimation can lead to unrealistic expectations and misapplications of these technologies, highlighting the need for a more nuanced understanding of their capabilities and limitations. As we continue to integrate LLMs into critical applications, it is crucial to recognize that their apparent reasoning skills are largely a reflection of their training data and algorithms, rather than an indication of true cognitive understanding.
Misconceptions About AI: Understanding the Limits of Large Language Models
The rapid advancement of artificial intelligence, particularly in the realm of large language models (LLMs), has sparked both excitement and misconceptions about their capabilities. These models, such as OpenAI’s GPT series, have demonstrated remarkable proficiency in generating human-like text, leading many to overestimate their reasoning abilities. However, it is crucial to understand the limitations of these models to avoid unrealistic expectations and potential misapplications.
To begin with, large language models are fundamentally statistical tools that predict the next word in a sequence based on patterns learned from vast amounts of text data. This process allows them to produce coherent and contextually relevant responses, often giving the impression of understanding and reasoning. However, this impression is largely an artifact of their design rather than an indication of genuine cognitive abilities. Unlike humans, LLMs do not possess consciousness, self-awareness, or the ability to comprehend the meaning behind the text they generate. They operate without an understanding of the world, relying solely on the correlations present in their training data.
Moreover, the reasoning capabilities of LLMs are limited by their lack of true comprehension. While they can mimic logical reasoning by recognizing patterns in data, they do not engage in abstract thinking or possess the ability to apply knowledge in novel situations. For instance, when faced with tasks that require common sense reasoning or understanding of complex cause-and-effect relationships, LLMs often falter. This limitation is evident in their tendency to produce plausible-sounding but factually incorrect or nonsensical responses when confronted with questions outside their training data’s scope.
Furthermore, the overestimation of LLMs’ reasoning abilities can lead to significant risks, particularly when these models are deployed in critical applications. In fields such as healthcare, law, and finance, reliance on LLMs without human oversight can result in erroneous decisions with serious consequences. It is essential to recognize that while LLMs can assist in processing and generating information, they should not be viewed as replacements for human judgment and expertise. Instead, they should be integrated as tools that augment human capabilities, with their outputs subject to careful scrutiny and validation.
In addition to these practical concerns, the ethical implications of overestimating LLMs’ reasoning abilities must be considered. The anthropomorphization of these models can lead to misplaced trust and accountability issues. If users believe that LLMs possess human-like understanding, they may attribute responsibility for errors or biases to the models themselves rather than the developers and organizations that deploy them. This misattribution can hinder efforts to address the underlying biases present in the training data and the models’ design, perpetuating systemic issues.
In conclusion, while large language models represent a significant technological achievement, it is imperative to maintain a realistic perspective on their capabilities. By acknowledging their limitations in reasoning and understanding, we can better harness their potential while mitigating the risks associated with their use. As we continue to explore the possibilities of AI, a balanced approach that combines technological innovation with ethical considerations and human oversight will be essential in ensuring that these powerful tools are used responsibly and effectively.
The Hype vs. Reality: Debunking Myths About AI Reasoning
In recent years, large language models (LLMs) have captured the imagination of both the public and the tech industry, promising a future where artificial intelligence can understand and reason like humans. These models, powered by vast amounts of data and sophisticated algorithms, have demonstrated impressive capabilities in generating human-like text, translating languages, and even composing poetry. However, amidst the excitement, there is a growing concern that the reasoning abilities of these models are being overestimated. This overestimation stems from a misunderstanding of what these models are truly capable of and the limitations inherent in their design.
To begin with, it is essential to understand that large language models are fundamentally statistical tools. They are trained on extensive datasets to predict the next word in a sequence, based on the context provided by preceding words. This process allows them to generate coherent and contextually relevant text. However, this does not equate to genuine understanding or reasoning. The models do not possess an awareness of the content they produce; rather, they rely on patterns and correlations learned from the data. Consequently, while they can mimic reasoning by producing text that appears logical, they do not engage in the cognitive processes that underpin human reasoning.
Moreover, the training data used for these models plays a crucial role in shaping their outputs. Since LLMs are trained on vast corpora of text from the internet, they inherit the biases, inaccuracies, and inconsistencies present in these sources. This can lead to outputs that are not only factually incorrect but also ethically problematic. For instance, when tasked with generating content on complex topics, these models may produce text that seems plausible but is ultimately misleading or biased. This highlights a significant limitation: the models’ inability to discern truth from falsehood or to apply ethical considerations in their reasoning.
Furthermore, the impressive performance of LLMs in specific tasks often leads to the assumption that they possess a general reasoning capability. However, their success is largely confined to well-defined problems with clear patterns. When faced with tasks that require abstract thinking, common sense, or an understanding of nuanced human experiences, these models frequently fall short. This is because they lack the experiential knowledge and emotional intelligence that inform human reasoning. As a result, their outputs can be superficial, lacking the depth and insight that characterize human thought.
In addition to these limitations, the interpretability of LLMs poses a challenge. The complexity of these models makes it difficult to understand how they arrive at specific outputs, leading to a “black box” problem. This lack of transparency can be problematic, especially in applications where accountability and trust are paramount. Without a clear understanding of the decision-making process, it becomes challenging to assess the reliability and validity of the models’ reasoning.
In conclusion, while large language models represent a significant advancement in artificial intelligence, it is crucial to temper expectations regarding their reasoning abilities. They excel at generating text that mimics human language but fall short of true understanding and reasoning. As we continue to integrate these models into various aspects of society, it is imperative to remain aware of their limitations and to approach their outputs with a critical eye. By doing so, we can harness their potential while mitigating the risks associated with overestimating their capabilities.
Cognitive Illusions: Why Large Language Models Aren’t Truly Intelligent
The rapid advancement of artificial intelligence, particularly in the realm of large language models (LLMs), has sparked widespread fascination and debate. These models, capable of generating human-like text, have been heralded as groundbreaking tools with the potential to revolutionize industries ranging from customer service to creative writing. However, amidst the excitement, there is a growing concern that the reasoning abilities of these models are being overestimated. This overestimation stems from a cognitive illusion, where the sophistication of language output is mistaken for genuine understanding and intelligence.
To begin with, it is essential to understand the fundamental workings of large language models. These models, such as OpenAI’s GPT series, are trained on vast datasets comprising diverse text from the internet. Through this training, they learn to predict the next word in a sentence, thereby generating coherent and contextually relevant text. While this process allows them to mimic human-like conversation, it does not equate to true comprehension or reasoning. The models operate based on patterns and probabilities rather than an understanding of the content they produce.
Moreover, the illusion of intelligence is further compounded by the models’ ability to perform tasks that appear to require reasoning. For instance, LLMs can solve mathematical problems, answer trivia questions, and even engage in philosophical discussions. However, these capabilities are not indicative of genuine cognitive processes. Instead, they are the result of statistical correlations learned during training. The models do not possess an internal representation of concepts or the ability to engage in abstract thinking. They merely generate responses that are statistically likely to be correct based on their training data.
In addition, the limitations of large language models become evident when they encounter tasks that require common sense reasoning or real-world knowledge. Despite their impressive language generation capabilities, these models often produce responses that are nonsensical or factually incorrect. This is because they lack an understanding of the world and cannot apply context beyond the text they have been trained on. Consequently, their outputs can be misleading, especially when they are presented with ambiguous or complex queries.
Furthermore, the overestimation of LLMs’ reasoning abilities can have significant implications. In fields such as healthcare, legal services, and education, reliance on these models without recognizing their limitations could lead to erroneous decisions and outcomes. It is crucial for users and developers to maintain a critical perspective, acknowledging that while LLMs are powerful tools, they are not infallible or capable of independent thought.
In conclusion, the cognitive illusion surrounding large language models’ reasoning abilities highlights the need for a nuanced understanding of their capabilities. While these models have made remarkable strides in natural language processing, they remain fundamentally limited by their lack of true comprehension and reasoning. As society continues to integrate AI into various aspects of life, it is imperative to approach these technologies with both optimism and caution. By recognizing the distinction between language generation and genuine intelligence, we can better harness the potential of LLMs while mitigating the risks associated with their overestimation.
The Role of Human Oversight in AI Decision-Making
The rapid advancement of artificial intelligence, particularly large language models (LLMs), has sparked significant interest and debate regarding their capabilities and limitations. These models, trained on vast datasets, have demonstrated remarkable proficiency in generating human-like text, translating languages, and even composing poetry. However, there is a growing concern that their reasoning abilities are often overestimated. This overestimation can lead to misplaced trust in AI systems, which underscores the critical importance of human oversight in AI decision-making processes.
To begin with, it is essential to understand the nature of large language models. These models operate primarily on pattern recognition and statistical correlations rather than genuine comprehension or reasoning. They excel at predicting the next word in a sentence based on the context provided by preceding words. While this allows them to produce coherent and contextually relevant text, it does not equate to true understanding or the ability to reason through complex problems. Consequently, when tasked with decisions that require nuanced judgment or ethical considerations, LLMs may fall short, as they lack the intrinsic human qualities of empathy, moral reasoning, and common sense.
Moreover, the overestimation of LLMs’ reasoning abilities can lead to significant risks, particularly when these models are deployed in high-stakes environments such as healthcare, legal systems, or autonomous vehicles. In these contexts, the consequences of erroneous decisions can be severe, affecting human lives and societal structures. Therefore, it is imperative to maintain a robust framework of human oversight to ensure that AI systems are used responsibly and ethically. Human oversight acts as a safeguard, providing the necessary checks and balances to mitigate potential errors and biases inherent in AI models.
Furthermore, the role of human oversight extends beyond merely monitoring AI outputs. It involves a collaborative approach where human expertise complements the computational power of AI. By integrating human judgment with AI capabilities, organizations can harness the strengths of both entities to achieve more accurate and reliable outcomes. For instance, in medical diagnostics, AI can assist in analyzing vast amounts of data to identify patterns, while human doctors provide the critical interpretation and contextual understanding needed for accurate diagnosis and treatment planning.
In addition, fostering transparency and accountability in AI decision-making processes is crucial. This involves ensuring that AI systems are designed with explainability in mind, allowing humans to understand the rationale behind AI-generated decisions. By doing so, stakeholders can make informed judgments about the reliability and appropriateness of AI recommendations. This transparency also facilitates trust between AI systems and their human users, which is essential for the successful integration of AI into various sectors.
In conclusion, while large language models represent a significant technological advancement, it is vital to recognize their limitations in reasoning and decision-making. The overestimation of their capabilities can lead to unintended consequences, emphasizing the need for human oversight. By maintaining a balanced approach that leverages the strengths of both AI and human intelligence, society can ensure that AI systems are used ethically and effectively. As we continue to explore the potential of AI, it is crucial to prioritize human oversight to safeguard against the risks associated with over-reliance on these powerful yet imperfect tools.
The Dangers of Overestimating AI Capabilities in Critical Applications
The rapid advancement of artificial intelligence, particularly in the realm of large language models (LLMs), has sparked both excitement and concern across various sectors. These models, which are designed to understand and generate human-like text, have demonstrated remarkable capabilities in tasks such as language translation, content creation, and even coding. However, the enthusiasm surrounding their potential often leads to an overestimation of their reasoning abilities, which can pose significant risks when applied to critical applications. As we delve into the intricacies of this issue, it is essential to understand the limitations inherent in these models and the potential consequences of misjudging their capabilities.
To begin with, large language models are fundamentally statistical tools that predict the likelihood of a sequence of words based on vast amounts of data. While they can mimic human-like responses and exhibit a semblance of understanding, they lack true comprehension and reasoning. Their outputs are generated based on patterns learned from the data they were trained on, rather than any genuine understanding of the world. Consequently, when these models are deployed in critical applications such as healthcare, legal decision-making, or autonomous systems, the risk of error due to their lack of reasoning becomes a pressing concern.
Moreover, the overestimation of LLMs’ reasoning abilities can lead to a false sense of security among users and developers. This misplaced confidence may result in the deployment of AI systems without adequate oversight or human intervention, potentially leading to catastrophic outcomes. For instance, in the medical field, relying on AI for diagnostic purposes without human verification could result in misdiagnoses, adversely affecting patient outcomes. Similarly, in the legal domain, the use of AI to assess legal documents or predict case outcomes without human oversight could lead to unjust decisions, undermining the integrity of the legal system.
In addition to these risks, the overreliance on LLMs can stifle critical thinking and innovation. When organizations place undue trust in AI systems, there is a tendency to overlook the importance of human expertise and judgment. This can lead to a reduction in the development of human skills and a diminished capacity for problem-solving, as individuals become increasingly dependent on AI-generated solutions. Furthermore, the assumption that AI can replace human reasoning may discourage investment in research and development aimed at enhancing human-AI collaboration, which is crucial for leveraging the strengths of both entities.
To mitigate these dangers, it is imperative to adopt a balanced approach that recognizes the capabilities and limitations of large language models. This involves implementing robust validation and verification processes to ensure that AI systems are reliable and accurate. Additionally, fostering a culture of transparency and accountability in AI development can help build trust and ensure that these technologies are used responsibly. Encouraging interdisciplinary collaboration between AI researchers, domain experts, and ethicists can also contribute to the development of AI systems that are both effective and ethical.
In conclusion, while large language models have the potential to revolutionize various industries, it is crucial to remain cognizant of their limitations. Overestimating their reasoning abilities can lead to significant risks, particularly in critical applications where accuracy and reliability are paramount. By adopting a cautious and informed approach, we can harness the benefits of AI while minimizing the potential for harm, ultimately ensuring that these technologies serve as valuable tools rather than sources of unintended consequences.
Bridging the Gap: Enhancing AI with Human-Like Reasoning Skills
The rapid advancement of artificial intelligence, particularly in the realm of large language models (LLMs), has sparked widespread fascination and optimism about their potential applications. These models, powered by sophisticated algorithms and vast datasets, have demonstrated remarkable capabilities in generating human-like text, translating languages, and even composing poetry. However, amidst the excitement, there is a growing concern that the reasoning abilities of these models are often overestimated. This overestimation stems from a misunderstanding of the fundamental nature of LLMs and their limitations in replicating human-like reasoning.
To begin with, it is essential to understand that large language models are fundamentally statistical tools. They operate by identifying patterns in the data they have been trained on, rather than possessing an inherent understanding of the content. This pattern recognition allows them to predict the next word in a sentence or generate coherent text based on a given prompt. While this can create the illusion of reasoning, it is crucial to recognize that these models do not possess true cognitive abilities. They lack the capacity for abstract thought, critical analysis, and the nuanced understanding that characterizes human reasoning.
Moreover, the training process of LLMs is heavily reliant on the quality and diversity of the data they are exposed to. Consequently, their outputs are limited by the biases and gaps present in the training datasets. This limitation can lead to erroneous conclusions or inappropriate responses, particularly in complex scenarios that require a deep understanding of context and subtleties. For instance, when faced with ethical dilemmas or ambiguous situations, LLMs may struggle to provide satisfactory answers, as they do not possess the moral reasoning or empathy that humans apply in such cases.
Furthermore, the overestimation of LLMs’ reasoning abilities can have significant implications for their deployment in real-world applications. In fields such as healthcare, law, and education, where critical decision-making is paramount, relying solely on AI-generated insights without human oversight can lead to detrimental outcomes. It is imperative to recognize that while LLMs can assist in processing large volumes of information and identifying patterns, they should not be viewed as replacements for human expertise and judgment.
To bridge the gap between current AI capabilities and human-like reasoning, researchers are exploring various approaches. One promising avenue is the integration of symbolic reasoning with neural networks, which aims to combine the strengths of both paradigms. Symbolic reasoning, which involves the manipulation of symbols and rules, can provide a framework for more structured and logical decision-making. By incorporating this approach, AI systems could potentially enhance their reasoning abilities and offer more reliable and context-aware solutions.
In addition, fostering collaboration between AI and human experts is crucial for maximizing the benefits of these technologies. By leveraging the strengths of both parties, it is possible to create hybrid systems that capitalize on the efficiency of AI while retaining the depth of human insight. This collaborative approach can ensure that AI systems are used as tools to augment human capabilities, rather than as standalone entities.
In conclusion, while large language models have made significant strides in natural language processing, it is important to temper expectations regarding their reasoning abilities. Recognizing their limitations and potential biases is essential for their responsible deployment. By pursuing innovative research avenues and fostering collaboration between AI and humans, we can work towards enhancing AI systems with more human-like reasoning skills, ultimately leading to more effective and ethical applications.
Q&A
1. **Question:** What is a common misconception about large language models (LLMs)?
**Answer:** A common misconception is that LLMs possess human-like reasoning abilities, when in fact they primarily rely on pattern recognition and statistical correlations.
2. **Question:** Why might people overestimate the reasoning capabilities of LLMs?
**Answer:** People might overestimate these capabilities due to the models’ ability to generate coherent and contextually relevant text, which can give the illusion of understanding and reasoning.
3. **Question:** How do LLMs generate responses?
**Answer:** LLMs generate responses by predicting the next word in a sequence based on the patterns and data they have been trained on, rather than through logical reasoning or comprehension.
4. **Question:** What is a limitation of LLMs in terms of reasoning?
**Answer:** A limitation is that LLMs lack true understanding and cannot engage in abstract reasoning or comprehend the underlying meaning of complex concepts.
5. **Question:** How can the overestimation of LLMs’ abilities impact their use?
**Answer:** Overestimating their abilities can lead to inappropriate reliance on LLMs for tasks requiring critical thinking, decision-making, or nuanced understanding, potentially resulting in errors or misinformation.
6. **Question:** What should be considered when evaluating the capabilities of LLMs?
**Answer:** It is important to consider that LLMs are tools designed for specific tasks and should be used with an understanding of their limitations, particularly in reasoning and comprehension.The overestimation of large language models’ reasoning abilities stems from their impressive performance in generating human-like text, which can create an illusion of understanding and cognitive processing. However, these models primarily rely on pattern recognition and statistical correlations within the data they have been trained on, rather than genuine comprehension or logical reasoning. While they can mimic reasoning by producing plausible responses, they lack true understanding, intentionality, and the ability to engage in abstract thought. This discrepancy highlights the need for caution in attributing human-like reasoning capabilities to these models and underscores the importance of continued research to enhance their interpretative and reasoning skills.
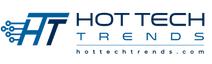