In recent years, the field of materials science has witnessed a transformative leap forward with the advent of a groundbreaking AI technique designed to accelerate the prediction of thermal properties in materials. This innovative approach leverages advanced machine learning algorithms to analyze and predict how materials conduct and store heat, a critical factor in a wide array of applications ranging from electronics to energy systems. By significantly reducing the time and computational resources traditionally required for such predictions, this AI-driven method not only enhances the efficiency of materials research but also opens new avenues for the development of advanced materials with tailored thermal properties. This breakthrough holds the potential to revolutionize industries by enabling faster innovation cycles and more sustainable material solutions.
Revolutionizing Material Science: How AI is Transforming Thermal Property Predictions
In recent years, the field of material science has witnessed a transformative shift, largely driven by the integration of artificial intelligence (AI) into research methodologies. One of the most significant advancements in this domain is the development of a breakthrough AI technique that accelerates the prediction of thermal properties in materials. This innovation not only enhances the efficiency of material design but also opens new avenues for the development of advanced materials with tailored thermal characteristics.
Traditionally, predicting the thermal properties of materials, such as thermal conductivity and specific heat capacity, has been a labor-intensive and time-consuming process. It often involves complex simulations and extensive experimental testing, which can delay the development of new materials. However, the advent of AI has introduced a paradigm shift, enabling researchers to bypass some of these cumbersome steps. By leveraging machine learning algorithms, scientists can now predict thermal properties with remarkable speed and accuracy, thus expediting the material discovery process.
The core of this breakthrough AI technique lies in its ability to learn from vast datasets of material properties and their corresponding thermal characteristics. By training on these datasets, the AI model can identify patterns and correlations that are not immediately apparent through traditional methods. This capability allows the model to make accurate predictions about the thermal properties of new or untested materials, significantly reducing the need for exhaustive experimental validation. Consequently, researchers can focus their efforts on the most promising material candidates, thereby streamlining the development pipeline.
Moreover, this AI-driven approach is not limited to predicting thermal properties alone. It also facilitates the exploration of novel materials with unique thermal behaviors that were previously difficult to identify. For instance, materials with high thermal conductivity are crucial for applications in electronics and energy systems, where efficient heat dissipation is essential. Conversely, materials with low thermal conductivity are valuable for thermal insulation purposes. The ability to rapidly predict and optimize these properties can lead to significant advancements in various industries, from consumer electronics to aerospace engineering.
In addition to accelerating the prediction process, AI techniques also enhance the accuracy of thermal property estimations. By continuously learning from new data and refining its algorithms, the AI model can improve its predictive capabilities over time. This iterative learning process ensures that the predictions remain reliable and relevant, even as new materials and technologies emerge. Furthermore, the integration of AI into material science fosters a more collaborative research environment, where interdisciplinary teams can leverage AI tools to address complex challenges and drive innovation.
As the field of material science continues to evolve, the role of AI in predicting thermal properties is expected to grow even more prominent. Researchers are already exploring the potential of combining AI with other advanced technologies, such as quantum computing and high-throughput experimentation, to further enhance the accuracy and efficiency of material predictions. This convergence of technologies promises to unlock new possibilities in material design and application, ultimately leading to the development of smarter, more sustainable materials.
In conclusion, the breakthrough AI technique for predicting thermal properties represents a significant leap forward in material science. By accelerating the prediction process and improving accuracy, AI is transforming the way researchers approach material design and discovery. As this technology continues to advance, it holds the potential to revolutionize industries reliant on materials with specific thermal characteristics, paving the way for innovative solutions to some of the world’s most pressing challenges.
The Role of Breakthrough AI Techniques in Advancing Material Thermal Analysis
In recent years, the field of material science has witnessed significant advancements, particularly in the analysis of thermal properties, which are crucial for a wide range of applications, from electronics to aerospace engineering. A breakthrough in artificial intelligence (AI) techniques has emerged as a pivotal tool in accelerating the prediction of these thermal properties, offering unprecedented speed and accuracy. This development is reshaping the landscape of material thermal analysis, providing researchers and engineers with powerful new capabilities.
Traditionally, predicting the thermal properties of materials has been a complex and time-consuming process. It often involves intricate calculations and extensive experimental testing, which can be both costly and labor-intensive. However, the advent of AI has introduced a paradigm shift in this domain. By leveraging machine learning algorithms, researchers can now analyze vast datasets and identify patterns that were previously undetectable. This capability not only enhances the accuracy of predictions but also significantly reduces the time required to obtain results.
One of the most promising AI techniques in this context is the use of neural networks, which are designed to mimic the human brain’s ability to recognize patterns and make decisions. These networks can be trained on existing data to predict the thermal properties of new materials with remarkable precision. As a result, researchers can explore a broader range of materials and configurations, accelerating the discovery of novel materials with desirable thermal characteristics.
Moreover, the integration of AI into material thermal analysis is not limited to prediction alone. It also facilitates the optimization of materials for specific applications. For instance, by using AI-driven simulations, engineers can identify the optimal composition and structure of a material to achieve desired thermal properties, such as high thermal conductivity or low thermal expansion. This capability is particularly valuable in industries where thermal management is critical, such as in the design of electronic devices that require efficient heat dissipation.
In addition to enhancing prediction and optimization, AI techniques also contribute to a deeper understanding of the fundamental mechanisms governing thermal properties. By analyzing large datasets, AI can uncover hidden correlations and insights that may not be apparent through traditional methods. This deeper understanding can lead to the development of new theories and models, further advancing the field of material science.
Despite these promising developments, it is important to acknowledge the challenges associated with the implementation of AI in material thermal analysis. One of the primary concerns is the quality and availability of data. AI models require large amounts of high-quality data to be effective, and obtaining such data can be a significant hurdle. Additionally, there is a need for interdisciplinary collaboration between material scientists and AI experts to ensure that the models are both accurate and applicable to real-world scenarios.
Nevertheless, the potential benefits of AI in this field are immense. As AI techniques continue to evolve and improve, they are likely to play an increasingly central role in material thermal analysis. This will not only accelerate the pace of innovation but also open up new possibilities for the development of advanced materials with tailored thermal properties. In conclusion, the integration of breakthrough AI techniques into material thermal analysis represents a significant advancement, offering a powerful tool for researchers and engineers to explore and optimize the thermal properties of materials with unprecedented efficiency and insight.
Accelerating Innovation: AI’s Impact on Predicting Thermal Properties of New Materials
In recent years, the field of materials science has witnessed a transformative shift, largely driven by the integration of artificial intelligence (AI) into research methodologies. One of the most significant advancements in this domain is the development of a breakthrough AI technique that accelerates the prediction of thermal properties for new materials. This innovation holds the potential to revolutionize the way scientists and engineers approach the design and application of materials across various industries.
Traditionally, predicting the thermal properties of materials has been a time-consuming and resource-intensive process. It often involves complex calculations and extensive experimental testing to determine how a material will conduct heat, respond to temperature changes, or withstand thermal stress. These properties are crucial for applications ranging from electronics and aerospace to energy storage and construction. However, the conventional methods have been limited by their reliance on empirical data and the need for precise control over experimental conditions.
The advent of AI has introduced a paradigm shift in this area by offering a more efficient and accurate approach to predicting thermal properties. By leveraging machine learning algorithms, researchers can now analyze vast datasets of material properties and identify patterns that were previously undetectable. This capability allows for the rapid screening of potential materials, significantly reducing the time required to identify candidates with desirable thermal characteristics.
One of the key advantages of using AI in this context is its ability to handle the complexity and variability inherent in material properties. Machine learning models can be trained on diverse datasets, encompassing a wide range of materials and conditions, enabling them to make predictions with a high degree of accuracy. Furthermore, these models can continuously improve as more data becomes available, enhancing their predictive power over time.
Moreover, the integration of AI into thermal property prediction is not limited to improving accuracy and speed. It also facilitates the discovery of novel materials with unique thermal properties that may have been overlooked using traditional methods. By exploring a broader design space, AI can identify unconventional material combinations and structures that exhibit superior performance, opening new avenues for innovation.
The impact of this breakthrough AI technique extends beyond the realm of materials science. Industries that rely heavily on materials with specific thermal properties stand to benefit significantly from these advancements. For instance, in the electronics industry, where managing heat dissipation is critical for device performance and longevity, the ability to quickly identify materials with optimal thermal conductivity can lead to more efficient and reliable products. Similarly, in the field of renewable energy, materials with enhanced thermal properties can improve the efficiency of solar panels and energy storage systems, contributing to more sustainable energy solutions.
In conclusion, the integration of AI into the prediction of thermal properties for new materials represents a significant leap forward in materials science. By accelerating the discovery and optimization of materials with tailored thermal characteristics, this breakthrough technique not only enhances the efficiency of research and development processes but also paves the way for innovative applications across various industries. As AI continues to evolve and its capabilities expand, its role in shaping the future of materials science and engineering is poised to become even more profound, driving progress and innovation at an unprecedented pace.
From Lab to AI: How Machine Learning is Enhancing Thermal Property Predictions
In recent years, the field of materials science has witnessed a transformative shift with the integration of artificial intelligence, particularly machine learning, into its research methodologies. This evolution is especially evident in the prediction of thermal properties of materials, a critical aspect that influences the design and application of materials across various industries. Traditionally, determining the thermal properties of materials, such as thermal conductivity and specific heat capacity, has been a labor-intensive process, often requiring extensive experimental work and complex simulations. However, the advent of a breakthrough AI technique is now accelerating these predictions, offering a promising alternative to conventional methods.
Machine learning, a subset of artificial intelligence, has emerged as a powerful tool in this domain due to its ability to analyze vast datasets and identify patterns that may not be immediately apparent to human researchers. By training algorithms on existing data, machine learning models can predict the thermal properties of new materials with remarkable accuracy. This capability not only reduces the time and resources required for experimental validation but also opens up new avenues for the discovery and development of novel materials with tailored thermal characteristics.
One of the key advantages of using machine learning in thermal property predictions is its ability to handle the complexity and non-linearity inherent in material behaviors. Traditional computational methods often struggle with these challenges, as they require simplifications and assumptions that can compromise accuracy. In contrast, machine learning models can learn directly from data, capturing intricate relationships between material composition, structure, and thermal properties. This data-driven approach allows for more precise predictions, which are crucial for applications ranging from electronics cooling to thermal barrier coatings in aerospace.
Moreover, the integration of machine learning into materials science is fostering a more collaborative and interdisciplinary research environment. By bridging the gap between experimentalists and computational scientists, machine learning facilitates a more holistic understanding of material properties. Researchers can now leverage AI to rapidly screen potential materials, narrowing down candidates for experimental testing. This synergy not only accelerates the research process but also enhances the overall efficiency of material development pipelines.
Despite these advancements, challenges remain in the widespread adoption of machine learning for thermal property predictions. One significant hurdle is the availability and quality of data. Machine learning models require large, high-quality datasets to achieve reliable predictions. However, such datasets are often scarce, particularly for novel materials. To address this issue, researchers are increasingly turning to data augmentation techniques and collaborative data-sharing platforms, which can help build more comprehensive datasets.
Furthermore, the interpretability of machine learning models is another area of concern. While these models can provide accurate predictions, understanding the underlying mechanisms driving these predictions is crucial for gaining scientific insights and ensuring trust in AI-driven methodologies. Efforts are underway to develop more interpretable models and visualization tools that can elucidate the decision-making processes of machine learning algorithms.
In conclusion, the integration of machine learning into the prediction of thermal properties represents a significant leap forward in materials science. By offering a faster, more accurate, and cost-effective alternative to traditional methods, this breakthrough AI technique is poised to revolutionize the way researchers approach material design and development. As the field continues to evolve, ongoing collaboration and innovation will be essential to overcoming existing challenges and unlocking the full potential of AI in materials science.
The Future of Material Design: AI-Driven Thermal Property Prediction Techniques
In recent years, the field of material science has witnessed a transformative shift with the integration of artificial intelligence (AI) techniques, particularly in predicting thermal properties of materials. This advancement is pivotal as it addresses the growing demand for materials with specific thermal characteristics, essential for applications ranging from electronics to aerospace. Traditionally, predicting thermal properties such as thermal conductivity and specific heat capacity has been a labor-intensive process, often requiring extensive experimental work and complex simulations. However, the advent of AI-driven techniques has introduced a paradigm shift, offering a more efficient and accurate approach to material design.
One of the most significant breakthroughs in this domain is the development of machine learning models that can predict thermal properties with remarkable precision. These models are trained on vast datasets comprising experimental results and theoretical calculations, enabling them to identify patterns and correlations that might be overlooked by conventional methods. By leveraging these patterns, AI models can predict the thermal behavior of new materials, thereby accelerating the material discovery process. This capability is particularly beneficial in the early stages of material design, where rapid screening of potential candidates is crucial.
Moreover, the integration of AI in thermal property prediction is not limited to enhancing accuracy and speed. It also facilitates the exploration of novel materials that were previously deemed too complex to analyze. For instance, AI algorithms can handle high-dimensional data and complex interactions within materials, providing insights into the underlying mechanisms that govern thermal properties. This ability to delve deeper into the material’s structure-function relationship opens up new avenues for innovation, allowing researchers to tailor materials with unprecedented precision.
In addition to these advancements, AI-driven techniques offer a sustainable approach to material design. By reducing the reliance on extensive experimental trials, these methods minimize resource consumption and environmental impact. This aligns with the broader goal of sustainable development, where efficient use of resources is paramount. Furthermore, the predictive power of AI can guide experimental efforts, ensuring that only the most promising materials are subjected to further testing, thereby optimizing the use of time and resources.
Despite these promising developments, the integration of AI in thermal property prediction is not without challenges. One of the primary concerns is the quality and diversity of the data used to train AI models. Ensuring that datasets are comprehensive and representative of real-world conditions is crucial for the reliability of predictions. Additionally, there is a need for interdisciplinary collaboration between material scientists and AI experts to refine these models and address any limitations.
Looking ahead, the future of material design is poised to be increasingly reliant on AI-driven techniques. As these methods continue to evolve, they hold the potential to revolutionize the way materials are designed and utilized across various industries. The ability to predict thermal properties accurately and efficiently will not only enhance the performance of existing materials but also pave the way for the development of next-generation materials with tailored properties. In conclusion, the integration of AI in thermal property prediction represents a significant leap forward in material science, promising a future where innovation is driven by data and computational power.
Unveiling the Potential: AI’s Role in Rapid Thermal Property Assessment for Materials
In recent years, the field of materials science has witnessed a transformative shift, largely driven by the integration of artificial intelligence (AI) into research methodologies. One of the most promising advancements in this domain is the development of a breakthrough AI technique that significantly accelerates the prediction of thermal properties in materials. This innovation not only enhances the efficiency of material design but also opens new avenues for applications across various industries, from electronics to aerospace.
Traditionally, the assessment of thermal properties, such as thermal conductivity and specific heat capacity, has relied on time-consuming and resource-intensive experimental procedures. These methods, while accurate, often require extensive laboratory work and sophisticated equipment, which can be a bottleneck in the rapid development of new materials. However, the advent of AI-driven techniques has begun to alleviate these challenges by offering a faster and more cost-effective alternative.
The core of this breakthrough lies in the ability of AI algorithms to learn from vast datasets of material properties and their corresponding thermal characteristics. By employing machine learning models, researchers can now predict the thermal behavior of new materials with remarkable accuracy. This process involves training the AI on existing data, allowing it to identify patterns and correlations that might be imperceptible to human researchers. Consequently, once trained, these models can quickly evaluate the thermal properties of novel materials, thus expediting the research and development cycle.
Moreover, the integration of AI in thermal property prediction is not limited to mere acceleration. It also enhances the precision of predictions by continuously refining its models as more data becomes available. This iterative learning process ensures that the AI remains up-to-date with the latest material discoveries and innovations, thereby maintaining its relevance and accuracy over time. Furthermore, the use of AI reduces the likelihood of human error, which can often skew experimental results and lead to costly setbacks in material development.
In addition to improving efficiency and accuracy, AI-driven thermal property prediction also facilitates the exploration of new material compositions that were previously deemed too complex or resource-intensive to investigate. By simulating various scenarios and compositions, AI can identify promising candidates for further study, thereby broadening the scope of material research. This capability is particularly valuable in the quest for sustainable materials, where the need for environmentally friendly and efficient solutions is paramount.
The implications of this breakthrough extend beyond the laboratory, impacting industries that rely heavily on material performance. For instance, in the electronics industry, where thermal management is crucial for device reliability and performance, the ability to rapidly assess thermal properties can lead to the development of more efficient cooling solutions. Similarly, in aerospace, where materials are subjected to extreme thermal conditions, AI-driven predictions can aid in the design of components that withstand high temperatures while maintaining structural integrity.
In conclusion, the integration of AI into the prediction of thermal properties represents a significant leap forward in materials science. By accelerating the assessment process, enhancing accuracy, and enabling the exploration of new material possibilities, this breakthrough technique holds the potential to revolutionize the way materials are developed and utilized across various sectors. As AI continues to evolve and improve, its role in materials science is likely to expand, paving the way for even more innovative and efficient solutions in the future.
Q&A
1. **What is the breakthrough AI technique?**
The breakthrough AI technique involves using advanced machine learning models to predict the thermal properties of materials more efficiently and accurately than traditional methods.
2. **How does this AI technique work?**
The technique leverages large datasets and sophisticated algorithms to identify patterns and relationships in material properties, enabling rapid predictions of thermal behavior without the need for extensive physical testing.
3. **What are the benefits of this AI technique?**
The benefits include significantly reduced time and cost for predicting thermal properties, increased accuracy in predictions, and the ability to explore a wider range of materials for potential applications.
4. **What industries could be impacted by this AI technique?**
Industries such as electronics, aerospace, automotive, and materials science could be significantly impacted, as they often require precise thermal management solutions.
5. **What challenges does this AI technique address?**
It addresses challenges related to the time-consuming and resource-intensive nature of traditional thermal property testing, as well as the limitations in exploring new materials due to these constraints.
6. **What is the potential future impact of this AI technique?**
The potential future impact includes accelerating the development of new materials with tailored thermal properties, enhancing energy efficiency, and fostering innovation in various high-tech industries.The breakthrough AI technique for accelerating thermal property predictions in materials represents a significant advancement in materials science and engineering. By leveraging machine learning algorithms and vast datasets, this approach enhances the speed and accuracy of predicting thermal properties, which are crucial for the development of new materials and technologies. This innovation not only reduces the time and cost associated with traditional experimental methods but also opens up new possibilities for designing materials with tailored thermal characteristics. As a result, industries such as electronics, aerospace, and energy can benefit from more efficient thermal management solutions, leading to improved performance and sustainability. Overall, this AI-driven method marks a pivotal step forward in the integration of artificial intelligence with materials research, promising to accelerate innovation and application in various technological fields.
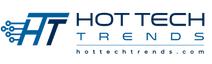