In recent advancements within the field of materials science, artificial intelligence (AI) has emerged as a transformative tool, particularly in the study and analysis of crystalline materials. AI models, leveraging sophisticated algorithms and vast datasets, have demonstrated remarkable capabilities in unveiling the intricate structures of these materials. By automating the complex process of structural determination, AI not only accelerates the pace of discovery but also enhances the accuracy of identifying atomic arrangements within crystals. This breakthrough holds significant implications for various industries, including electronics, pharmaceuticals, and renewable energy, where understanding the precise structure of materials is crucial for innovation and development. As AI continues to evolve, its integration into materials science promises to unlock new potentials and drive forward the next generation of technological advancements.
Advancements In AI-Driven Crystallography
In recent years, the field of crystallography has witnessed a transformative shift, largely driven by advancements in artificial intelligence (AI). The unveiling of crystalline structures, once a labor-intensive and time-consuming process, is now being revolutionized by AI models that promise to enhance both the speed and accuracy of structural determination. This development is particularly significant as it opens new avenues for research and application in various scientific domains, including materials science, chemistry, and pharmacology.
Traditionally, the determination of crystalline structures relied heavily on techniques such as X-ray diffraction, which, while effective, required significant manual intervention and expertise. Researchers would painstakingly analyze diffraction patterns to infer the atomic arrangement within a crystal. However, the advent of AI has introduced a paradigm shift. By leveraging machine learning algorithms, AI models can now process vast amounts of diffraction data with unprecedented efficiency. These models are trained on extensive datasets, enabling them to recognize complex patterns and predict the arrangement of atoms within a crystal lattice with remarkable precision.
One of the most compelling advantages of AI-driven crystallography is its ability to handle complex and disordered systems that have traditionally posed challenges to conventional methods. AI models excel in identifying subtle patterns within noisy data, allowing researchers to explore materials that were previously deemed too difficult to analyze. This capability is particularly beneficial in the study of amorphous materials and complex alloys, where traditional methods often fall short. Consequently, AI is not only accelerating the pace of discovery but also expanding the scope of materials that can be studied.
Moreover, the integration of AI into crystallography is fostering interdisciplinary collaboration. By bridging the gap between computer science and materials science, researchers are developing more sophisticated models that can simulate and predict the properties of new materials. This synergy is paving the way for the design of novel materials with tailored properties, which could have far-reaching implications for industries ranging from electronics to pharmaceuticals. For instance, the ability to predict the crystalline structure of a drug compound can significantly expedite the drug development process, potentially leading to more effective and targeted therapies.
Despite these promising advancements, the integration of AI into crystallography is not without its challenges. The accuracy of AI models is heavily dependent on the quality and diversity of the training data. Ensuring that these models are trained on comprehensive datasets that encompass a wide range of materials is crucial to their success. Additionally, there is a need for continued collaboration between domain experts and AI specialists to refine these models and address any limitations.
In conclusion, the application of AI in crystallography represents a significant leap forward in the study of crystalline materials. By enhancing the speed and accuracy of structural determination, AI is not only transforming the field but also unlocking new possibilities for scientific discovery and innovation. As researchers continue to refine these models and expand their capabilities, the potential for AI-driven crystallography to impact various scientific and industrial domains is immense. The future of crystallography, augmented by AI, promises to be one of accelerated discovery and unprecedented insight into the fundamental building blocks of matter.
Revolutionizing Material Science With AI Models
The advent of artificial intelligence (AI) has ushered in a new era of innovation across various scientific disciplines, and material science is no exception. Recently, a groundbreaking AI model has been developed to unveil the intricate structures of crystalline materials, marking a significant leap forward in the field. This development holds the potential to revolutionize how scientists understand and manipulate materials at the atomic level, thereby opening new avenues for technological advancements.
Crystalline materials, characterized by their highly ordered atomic structures, are fundamental to numerous applications, ranging from electronics to pharmaceuticals. Traditionally, determining the structure of these materials has been a labor-intensive process, often requiring sophisticated techniques such as X-ray crystallography or electron microscopy. These methods, while effective, are time-consuming and demand a high level of expertise. However, the introduction of AI into this domain promises to streamline the process significantly.
The AI model in question employs advanced machine learning algorithms to predict the atomic arrangements within crystalline materials. By analyzing vast datasets of known crystal structures, the model learns to identify patterns and correlations that are not immediately apparent to human researchers. This capability allows the AI to predict the structure of new materials with remarkable accuracy, even when only limited data is available. Consequently, this reduces the reliance on traditional experimental methods, thereby accelerating the pace of discovery and innovation.
Moreover, the AI model’s ability to predict crystalline structures has profound implications for material design. By understanding the atomic arrangement, scientists can tailor materials to exhibit specific properties, such as enhanced strength, conductivity, or thermal resistance. This level of precision in material design is crucial for developing next-generation technologies, including more efficient batteries, advanced semiconductors, and novel pharmaceuticals. As a result, industries that rely heavily on material science stand to benefit immensely from these advancements.
In addition to its practical applications, the AI model also contributes to the theoretical understanding of crystalline materials. By uncovering previously unknown structures, the model provides insights into the fundamental principles governing material formation and stability. This knowledge not only enriches the scientific community’s understanding but also guides future research endeavors, fostering a more comprehensive exploration of the material world.
Furthermore, the integration of AI into material science exemplifies the broader trend of interdisciplinary collaboration in modern research. By combining expertise from computer science, physics, and chemistry, researchers can tackle complex problems more effectively than ever before. This collaborative approach not only enhances the capabilities of AI models but also ensures that their development is informed by a deep understanding of the scientific challenges at hand.
As we look to the future, the potential of AI in material science appears boundless. Continued advancements in machine learning algorithms and computational power will likely lead to even more sophisticated models, capable of tackling increasingly complex materials. Moreover, as AI becomes more integrated into research workflows, it will undoubtedly inspire new methodologies and paradigms, further transforming the landscape of material science.
In conclusion, the unveiling of crystalline structures by AI models represents a pivotal moment in material science. By streamlining the discovery process and enabling precise material design, AI is poised to drive significant technological advancements across various industries. As researchers continue to harness the power of AI, the possibilities for innovation and discovery in material science are truly limitless.
AI’s Role In Discovering New Crystalline Structures
The advent of artificial intelligence (AI) has revolutionized numerous fields, and materials science is no exception. Recently, AI models have been instrumental in unveiling the structures of crystalline materials, a breakthrough that holds significant promise for various industries. Crystalline materials, characterized by their highly ordered atomic structures, are fundamental to numerous applications, ranging from electronics to pharmaceuticals. Understanding their structures is crucial for developing new materials with enhanced properties. However, traditional methods of determining these structures, such as X-ray crystallography, are often time-consuming and require significant expertise. This is where AI comes into play, offering a more efficient and accurate alternative.
AI models, particularly those based on machine learning algorithms, have demonstrated remarkable capabilities in predicting the structures of crystalline materials. By training on vast datasets of known crystal structures, these models can learn the underlying patterns and relationships between atomic arrangements and their properties. Consequently, they can predict the structures of new materials with impressive accuracy. This ability not only accelerates the discovery process but also opens up possibilities for exploring materials that were previously difficult to analyze using conventional methods.
Moreover, the integration of AI in this domain is not limited to structure prediction. AI can also assist in optimizing the synthesis of crystalline materials. By analyzing data from previous experiments, AI models can identify optimal conditions for synthesizing materials with desired properties. This capability is particularly valuable in the development of materials for specific applications, such as semiconductors or catalysts, where precise control over the material’s structure is essential.
In addition to enhancing efficiency, AI-driven approaches contribute to a deeper understanding of the fundamental principles governing crystalline structures. By analyzing large datasets, AI can uncover hidden patterns and correlations that may not be apparent through traditional analysis. This insight can lead to the development of new theoretical models and frameworks, further advancing the field of materials science.
Furthermore, the use of AI in discovering new crystalline structures aligns with the broader trend of digital transformation in scientific research. As computational power continues to grow, the ability to process and analyze large datasets becomes increasingly feasible. AI models, with their capacity to handle complex data, are well-suited to take advantage of this trend, offering a powerful tool for researchers in their quest to discover new materials.
However, it is important to acknowledge the challenges associated with the integration of AI in this field. One significant concern is the quality and diversity of the data used to train AI models. Ensuring that these datasets are comprehensive and representative is crucial for the accuracy and reliability of the predictions. Additionally, the interpretability of AI models remains a topic of ongoing research. While these models can provide accurate predictions, understanding the rationale behind these predictions is essential for gaining trust and acceptance within the scientific community.
In conclusion, AI models are playing an increasingly vital role in discovering new crystalline structures, offering a more efficient and insightful approach compared to traditional methods. By leveraging the power of machine learning, researchers can accelerate the discovery process, optimize material synthesis, and gain a deeper understanding of the principles governing crystalline materials. As the field continues to evolve, addressing challenges related to data quality and model interpretability will be crucial for realizing the full potential of AI in materials science. Ultimately, the integration of AI in this domain promises to drive innovation and unlock new possibilities for a wide range of applications.
The Impact Of AI On Crystalline Material Research
The advent of artificial intelligence (AI) has revolutionized numerous fields, and its impact on crystalline material research is no exception. As researchers strive to understand the intricate structures of crystalline materials, AI models have emerged as powerful tools that offer unprecedented insights. These models are capable of analyzing vast datasets with remarkable speed and accuracy, thereby accelerating the discovery and development of new materials. The integration of AI into this domain not only enhances our understanding of existing materials but also paves the way for the creation of novel compounds with tailored properties.
Traditionally, the study of crystalline materials has relied heavily on experimental techniques such as X-ray diffraction and electron microscopy. While these methods have been instrumental in elucidating the atomic arrangements within crystals, they are often time-consuming and require significant expertise. In contrast, AI models can process and interpret complex data much more efficiently. By leveraging machine learning algorithms, these models can predict the structures of crystalline materials based on their chemical compositions, thus reducing the need for exhaustive experimental procedures.
Moreover, AI models excel in identifying patterns and correlations that may not be immediately apparent to human researchers. For instance, they can analyze the relationships between a material’s structure and its properties, such as conductivity or strength. This capability is particularly valuable in the field of materials science, where understanding these relationships is crucial for designing materials with specific functionalities. By uncovering these hidden connections, AI models enable researchers to make informed decisions about which materials to synthesize and test, thereby streamlining the research process.
In addition to enhancing our understanding of crystalline materials, AI models also facilitate the discovery of new materials. Through techniques such as generative design, AI can propose novel structures that have not yet been synthesized. These proposed structures can then be evaluated for their potential applications, such as in electronics, energy storage, or catalysis. This approach not only expands the repertoire of available materials but also accelerates the development of technologies that rely on advanced materials.
Furthermore, the use of AI in crystalline material research is not limited to theoretical predictions. AI models can also assist in the interpretation of experimental data, providing insights that might otherwise be overlooked. For example, they can help identify subtle features in diffraction patterns or suggest possible structural models that fit the observed data. This synergy between AI and experimental techniques enhances the reliability and accuracy of research findings, ultimately leading to a deeper understanding of material behavior.
Despite the significant advantages offered by AI, it is important to acknowledge the challenges associated with its implementation. The development of accurate AI models requires large datasets, which may not always be available for certain materials. Additionally, the interpretability of AI predictions remains a concern, as these models often operate as “black boxes” that do not readily reveal the reasoning behind their conclusions. Nevertheless, ongoing advancements in AI technology and the increasing availability of data are expected to address these challenges, further solidifying the role of AI in crystalline material research.
In conclusion, the integration of AI into the study of crystalline materials represents a transformative shift in the field. By offering rapid and accurate predictions, uncovering hidden patterns, and facilitating the discovery of new materials, AI models are reshaping the landscape of materials science. As researchers continue to harness the power of AI, the potential for groundbreaking discoveries in crystalline material research is boundless, promising a future where materials are designed with unprecedented precision and efficiency.
How AI Models Are Transforming Crystallography
The field of crystallography, which involves the study of crystal structures and their properties, has long been a cornerstone of materials science, chemistry, and physics. Traditionally, the determination of crystalline structures has relied on techniques such as X-ray diffraction, neutron diffraction, and electron microscopy. These methods, while effective, are often time-consuming and require significant expertise to interpret the data accurately. However, recent advancements in artificial intelligence (AI) are poised to revolutionize this domain by offering new ways to analyze and predict the structures of crystalline materials with unprecedented speed and accuracy.
AI models, particularly those based on machine learning algorithms, have demonstrated remarkable capabilities in processing vast amounts of data and identifying patterns that may not be immediately apparent to human researchers. In the context of crystallography, AI can be trained on extensive datasets of known crystal structures, learning the intricate relationships between atomic arrangements and their resulting properties. This training enables AI to predict the structures of new, uncharacterized materials, thereby accelerating the discovery process.
One of the most significant advantages of using AI in crystallography is its ability to handle complex, multi-dimensional data. Crystalline materials often exhibit intricate patterns that can be challenging to decipher using traditional methods. AI models, however, can analyze these patterns with high precision, identifying subtle features that might otherwise be overlooked. This capability not only enhances the accuracy of structural predictions but also provides deeper insights into the fundamental principles governing crystal formation.
Moreover, AI-driven approaches can significantly reduce the time required to determine crystal structures. Traditional methods often involve iterative processes of hypothesis testing and refinement, which can be labor-intensive and time-consuming. In contrast, AI models can rapidly generate predictions, allowing researchers to focus their efforts on experimental validation and further exploration. This efficiency is particularly valuable in fields such as pharmaceuticals and materials engineering, where the timely discovery of new materials can have substantial economic and societal impacts.
In addition to predicting crystal structures, AI models are also being used to optimize the synthesis of crystalline materials. By analyzing data from previous experiments, AI can suggest optimal conditions for crystal growth, such as temperature, pressure, and chemical composition. This capability not only improves the yield and quality of the resulting crystals but also reduces the trial-and-error approach traditionally associated with material synthesis.
Despite these promising developments, the integration of AI into crystallography is not without challenges. One of the primary concerns is the quality and diversity of the data used to train AI models. Incomplete or biased datasets can lead to inaccurate predictions, underscoring the importance of curating comprehensive and representative data. Additionally, the interpretability of AI models remains a critical issue. While AI can provide accurate predictions, understanding the underlying rationale for these predictions is essential for gaining scientific insights and ensuring the reliability of the results.
In conclusion, AI models are transforming the field of crystallography by offering new tools for analyzing and predicting the structures of crystalline materials. Through their ability to process complex data, reduce analysis time, and optimize material synthesis, AI-driven approaches hold the potential to accelerate discoveries and enhance our understanding of crystal structures. As researchers continue to refine these models and address existing challenges, the integration of AI into crystallography promises to unlock new frontiers in materials science and beyond.
Future Prospects Of AI In Crystalline Material Analysis
The advent of artificial intelligence (AI) in the realm of material science has ushered in a new era of discovery and innovation, particularly in the analysis of crystalline materials. These materials, characterized by their highly ordered atomic structures, are pivotal in a myriad of applications ranging from electronics to pharmaceuticals. The ability to accurately determine their structures is crucial for advancing technology and developing new materials with tailored properties. Traditionally, the analysis of crystalline structures has relied heavily on techniques such as X-ray diffraction and electron microscopy. While these methods are highly effective, they are often time-consuming and require significant expertise to interpret complex data. This is where AI models have begun to demonstrate their transformative potential.
AI models, particularly those based on machine learning algorithms, have shown remarkable proficiency in analyzing and predicting the structures of crystalline materials. By training on vast datasets of known crystal structures, these models can learn to recognize patterns and make predictions about unknown structures with impressive accuracy. This capability not only accelerates the process of material analysis but also opens up new possibilities for discovering materials that were previously difficult to analyze using conventional methods. Moreover, AI models can handle the vast amounts of data generated by modern experimental techniques, providing insights that might be overlooked by human analysts.
One of the most promising aspects of AI in crystalline material analysis is its potential to facilitate the discovery of new materials. By predicting the properties of hypothetical materials, AI can guide experimental efforts towards the most promising candidates, thereby reducing the time and resources required for material development. This predictive capability is particularly valuable in the search for materials with specific properties, such as superconductors or catalysts, where the parameter space is vast and complex. Furthermore, AI models can be used to simulate the behavior of materials under different conditions, providing valuable information about their stability and performance in real-world applications.
In addition to accelerating the discovery of new materials, AI also holds promise for enhancing our understanding of existing materials. By analyzing the vast amounts of data generated by experiments, AI can uncover subtle patterns and correlations that might be missed by traditional analysis methods. This deeper understanding can lead to the development of more efficient and sustainable materials, as well as the optimization of existing materials for specific applications. Moreover, AI can assist in the design of experiments, suggesting optimal conditions and parameters to achieve desired outcomes, thereby streamlining the research process.
Despite these promising prospects, the integration of AI into crystalline material analysis is not without challenges. One of the primary concerns is the quality and diversity of the data used to train AI models. Ensuring that models are trained on comprehensive and representative datasets is crucial for their accuracy and reliability. Additionally, the interpretability of AI models remains a significant challenge, as the decision-making processes of these models are often opaque. Addressing these challenges will require collaboration between AI researchers and material scientists, as well as the development of new methodologies and tools.
In conclusion, the future prospects of AI in crystalline material analysis are both exciting and promising. By enhancing our ability to analyze and predict the structures and properties of materials, AI has the potential to revolutionize the field of material science. As researchers continue to refine AI models and address existing challenges, the integration of AI into material analysis is likely to yield significant advancements in technology and innovation, ultimately contributing to a more sustainable and technologically advanced future.
Q&A
1. **What is the AI model’s primary function in the study of crystalline materials?**
The AI model’s primary function is to predict and unveil the atomic structures of crystalline materials, which can accelerate the discovery and design of new materials.
2. **How does the AI model improve the study of crystalline materials?**
The AI model improves the study by providing rapid and accurate predictions of crystal structures, reducing the need for time-consuming and expensive experimental methods.
3. **What techniques does the AI model use to predict crystalline structures?**
The AI model typically uses machine learning techniques, such as neural networks or deep learning algorithms, trained on large datasets of known crystal structures.
4. **What are the potential applications of this AI model in industry?**
Potential applications include the development of new materials for electronics, pharmaceuticals, energy storage, and catalysis, among others.
5. **What challenges does the AI model face in predicting crystalline structures?**
Challenges include handling complex and diverse crystal systems, ensuring the accuracy of predictions, and integrating with existing experimental methods for validation.
6. **How does the AI model impact the speed of materials discovery?**
The AI model significantly accelerates materials discovery by quickly generating hypotheses about possible structures, allowing researchers to focus on the most promising candidates for experimental validation.The unveiling of crystalline material structures by AI models marks a significant advancement in materials science, offering unprecedented insights into the atomic arrangements and properties of these materials. By leveraging machine learning algorithms and vast datasets, AI can predict and model complex crystalline structures with high accuracy and efficiency, surpassing traditional methods in speed and scope. This capability accelerates the discovery and design of new materials with tailored properties for various applications, from electronics to pharmaceuticals. The integration of AI in this field not only enhances our understanding of material science but also paves the way for innovative solutions to technological challenges, driving progress in multiple industries.
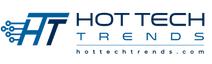