Are you ready to unlock the full potential of your agricultural operations and maximize crop yield? Harnessing the power of AI and machine learning can revolutionize the way you approach farming, optimizing productivity, conserving resources, and increasing profitability.
Imagine being able to predict crop diseases, monitor soil conditions, and implement proactive measures to prevent crop loss. With machine learning algorithms and computer vision technology, this is now possible. By analyzing satellite imagery, real-time data, and environmental factors, AI tools can provide valuable insights into crop health, pest infestation, and weather patterns.
In this blog, we will dive deep into the world of machine learning in agriculture, exploring its various applications and real-life examples. From smallholder farmers in rural areas to large agricultural enterprises, we will explore how AI-powered solutions are transforming the landscape of Indian agriculture, ensuring food security and sustainable practices.
Join us as we unveil the innovative ways AI tools, predictive analytics, and computer vision technology are revolutionizing crop yield optimization, resource management, and farm profitability analysis. Get ready to take your farming practices to new heights with the power of AI and machine learning.
Introduction to Machine Learning in Agriculture
Machine learning, a subset of artificial intelligence (AI), is revolutionizing the agriculture industry by optimizing crop yields and resource usage. By leveraging large amounts of data and advanced algorithms, machine learning enables farmers to make data-driven decisions and improve productivity.
One of the key applications of machine learning in agriculture is crop yield prediction. By analyzing factors such as satellite imagery, soil moisture, and historical data, machine learning algorithms can accurately predict crop yields. This allows farmers to optimize their planting strategies, allocate resources efficiently, and ensure maximum harvests.
Machine learning also plays a vital role in pest and disease detection. AI algorithms can analyze images of crops to identify signs of pest infestations or disease outbreaks. Early detection enables farmers to take preventive measures, saving crops from potential damage and reducing the need for excessive pesticide use.
Another area where machine learning excels is soil and water management. Real-time data and sensors provide insights into soil health, moisture levels, and nutrient content. Machine learning algorithms analyze this data to optimize irrigation schedules and fertilizer application, promoting sustainable farming practices and reducing water waste.
In addition to enhancing crop production, machine learning improves food quality assessment. By analyzing nutritional content, pesticide residues, and contaminants, AI algorithms help ensure food safety and enable farmers to meet quality standards. This benefits both consumers and farmers by increasing transparency and boosting confidence in the produce.
Moreover, machine learning can contribute to farm profitability analysis. By analyzing various factors such as crop prices, market trends, and input costs, machine learning algorithms provide insights for better decision-making. Farm management tools driven by AI help farmers make informed choices, optimize resource allocation, and enhance profitability.
Machine learning is transforming the agriculture industry, empowering farmers with innovative tools and techniques. From optimizing crop yields to improving resource management, the potential of machine learning in agriculture is immense. In the following sections, we will explore specific use cases, examples, and technologies that showcase the power of AI in revolutionizing farming practices.
Crop Yield Prediction
In the realm of agriculture, accurate crop yield prediction plays a crucial role in optimizing resource allocation, decision-making, and overall farm management. Machine learning algorithms, combined with satellite imagery, have emerged as a powerful tool for accurately predicting crop yields. By analyzing vast amounts of data, these algorithms can make accurate predictions based on various environmental factors and historical data.
Utilizing Satellite Imagery
Satellite imagery allows for a comprehensive view of crop fields, capturing detailed information about crop health, soil conditions, and environmental factors. Machine learning models can be trained to process and analyze these images, extracting valuable insights that contribute to precise yield predictions. By integrating satellite data with other relevant inputs such as weather data and soil moisture levels, these models can generate highly accurate crop yield predictions.
Benefits of Machine Learning in Crop Yield Prediction
The implementation of machine learning algorithms for crop yield prediction offers several benefits for farmers and the agriculture industry as a whole.
– Improved Resource Allocation: Accurate crop yield predictions enable farmers to optimize the allocation of resources such as water, fertilizers, and pesticides. By understanding the potential yield of different areas within their fields, farmers can make data-driven decisions regarding resource use, resulting in improved efficiency and reduced waste.
– Enhanced Decision-Making: Machine learning models provide farmers with valuable insights to support decision-making. By having access to accurate crop yield predictions, farmers can make informed choices about planting schedules, crop selection, and even market demand.
– Risk Mitigation: Crop yield prediction helps farmers mitigate risks associated with unpredictable weather patterns, pests, and diseases. By anticipating potential crop losses, farmers can take preventive measures in a timely manner, reducing crop damage and increasing overall productivity.
Challenges in Implementing Crop Yield Prediction
While machine learning holds great promise for accurate crop yield prediction, there are challenges that need to be overcome to ensure its successful implementation.
– Quality and Availability of Data: Accurate crop yield prediction requires the availability of high-quality, reliable data. Obtaining historical crop yield data, soil condition data, and other relevant information can be challenging, especially for smallholder farmers or those with limited resources.
– Complexity of Algorithms: Machine learning algorithms can be complex and require significant computational power for training and prediction. This can pose a challenge for farmers who may not have access to advanced technology or the necessary expertise to implement and interpret these algorithms effectively.
– Localized Variability: Variability in crop growth and yield can be highly localized, even within a small area. Machine learning models need to account for such variability to provide accurate predictions, which can be challenging due to the diverse range of factors influencing crop growth.
In conclusion, machine learning algorithms, combined with satellite imagery, offer a powerful and efficient approach to crop yield prediction in agriculture. With accurate predictions, farmers can optimize resource allocation, make informed decisions, and mitigate risks, ultimately leading to improved productivity and profitability. However, addressing challenges related to data availability, algorithm complexity, and localized variability is crucial for successful implementation. By harnessing the potential of machine learning, farmers can optimize crop production and contribute to a more sustainable and reliable food supply.
Pest and Disease Detection
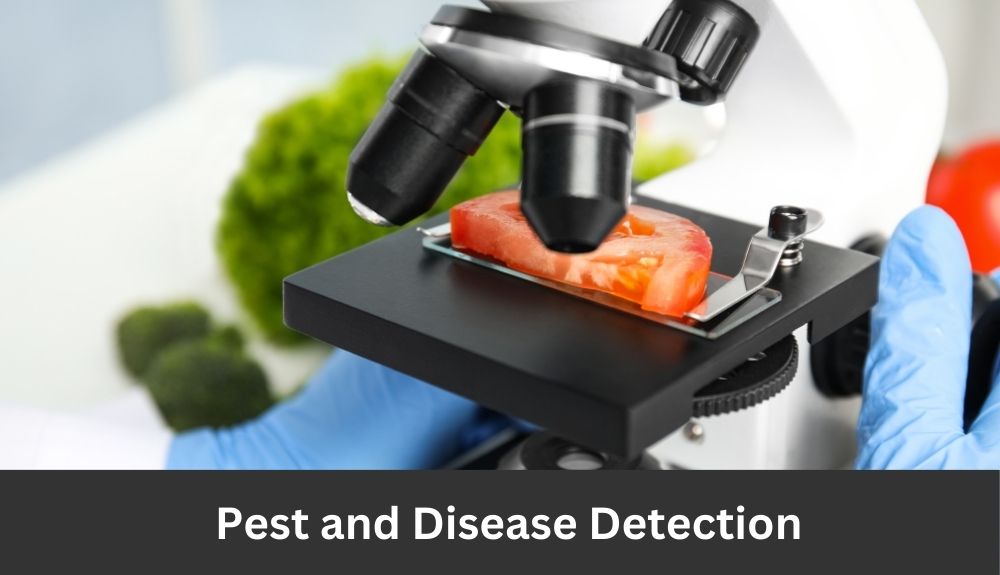
Pests and diseases pose significant threats to crop health and productivity, leading to substantial yield losses for farmers. However, with the advent of machine learning, detecting and preventing pest infestations and crop diseases has become more efficient and effective. By leveraging advanced algorithms and AI-powered solutions, farmers can now identify potential issues early on and implement proactive measures to protect their crops. Here, we will explore the role of machine learning in pest and disease detection, showcasing real-life examples of AI-powered solutions and the impact they have on crop health and productivity.
Early Detection and Diagnosis
Machine learning algorithms have the ability to analyze vast amounts of data, including images of crops, environmental factors, and historical data, to identify patterns and indicators of pest infestation or disease outbreak. Using computer vision technology, these algorithms can recognize visual cues of crop distress, such as discoloration, lesions, or unusual growth patterns, allowing farmers to take timely action.
AI-Powered Pest Management Systems
AI-driven pest management systems are revolutionizing the way farmers tackle pest infestations. These systems utilize data from various sources, including weather patterns, pest life cycles, and field conditions, to create predictive models that help farmers understand when and where pests are likely to strike. By being able to anticipate the threat, farmers can take preventive measures, such as applying targeted pesticides or deploying pheromone traps at the right time and place, minimizing the use of harmful chemicals and maximizing the effectiveness of pest control.
Disease Recognition and Diagnosis
Machine learning models are also being trained to recognize the symptoms of various crop diseases. By analyzing factors such as leaf discoloration, wilting, or the presence of specific pathogens, these models can provide accurate and rapid disease diagnosis. This enables farmers to quickly identify the disease affecting their crops and take appropriate action, such as implementing disease-resistant varieties, adjusting irrigation and nutrient management, or applying the necessary treatments.
AI-Powered Crop Health Monitoring
AI-powered sensors and drones equipped with multispectral cameras can capture high-resolution images of crops, allowing for real-time monitoring of plant health. These images are analyzed by machine learning algorithms, which can detect subtle changes in crop conditions, even before visible symptoms appear. By continuously monitoring crop health, farmers can identify potential issues early on, enabling them to intervene promptly and prevent further damage.
Impact on Crop Productivity and Sustainability
The integration of machine learning in pest and disease detection has a profound impact on crop productivity and sustainability. By enabling early detection and accurate diagnosis, farmers can minimize crop losses and optimize their resources by implementing targeted interventions. This leads to reduced pesticide use, less environmental impact, and improved farm profitability. Moreover, the use of AI-powered solutions in pest and disease management contributes to overall food security, as it helps ensure a steady supply of high-quality crops.
In conclusion, machine learning plays a crucial role in detecting and preventing pests and diseases in crops. With the ability to analyze large volumes of data, AI-powered solutions provide farmers with early warning signs and accurate diagnostics, allowing for timely interventions and proactive measures. By harnessing the power of machine learning, farmers can protect their crops, maximize yields, and contribute to a more sustainable and secure food system.
Soil and Water Management
Machine learning techniques are revolutionizing soil and water management practices in agriculture, enabling farmers to optimize resource usage and make data-driven decisions for improved crop yields. By leveraging real-time data and advanced sensors, machine learning algorithms can provide valuable insights into soil conditions, moisture levels, and water availability, leading to more efficient and sustainable farming practices.
Real-Time Data for Resource Allocation
Machine learning algorithms can process large amounts of real-time data collected from sensors placed in fields to monitor soil moisture, temperature, and other environmental factors. This data provides farmers with accurate and up-to-date information about the condition of their soil, enabling them to make informed decisions regarding irrigation schedules and water usage.
Precision Irrigation
One of the main applications of machine learning in soil and water management is precision irrigation. By analyzing data from sensors and historical weather patterns, machine learning models can predict crop water requirements and adjust irrigation schedules accordingly. This allows farmers to provide their crops with the optimal amount of water, reducing waste and improving water-use efficiency.
Preventive Measures Against Water Stress
Machine learning algorithms can also help farmers identify early signs of water stress in their crops by analyzing various factors such as soil moisture, leaf temperature, and crop growth patterns. By detecting these indicators, farmers can take proactive measures to mitigate water stress and minimize crop losses.
Predictive Modeling for Soil Fertility
Machine learning techniques can analyze soil data to create predictive models that determine soil fertility and nutrient levels. By understanding the nutrient composition and overall health of the soil, farmers can optimize fertilizer application, ensuring that crops receive the necessary nutrients for optimal growth while minimizing the use of excessive fertilizers that can harm the environment.
Enhanced Water Resource Management
In addition to optimizing irrigation practices, machine learning can assist in water resource management. By analyzing historical data on water availability, usage patterns, and weather conditions, machine learning algorithms can help farmers make informed decisions about water allocation and conservation strategies. This ensures the sustainable use of water resources and helps mitigate the impact of unpredictable weather patterns on crop production.
Lists:
Benefits of Machine Learning in Soil and Water Management:
– Optimized irrigation schedules
– Reduced water waste
– Improved water-use efficiency
– Early detection of water stress in crops
– Predictive modeling for soil fertility
– Enhanced water resource management
Examples of Machine Learning Applications:
– Predictive irrigation models based on real-time data
– Soil nutrient prediction and fertilizer optimization
– Crop water stress detection algorithms
– Water resource allocation models based on historical data
By leveraging machine learning techniques, farmers can significantly improve soil and water management practices, leading to higher crop yields, reduced resource wastage, and more sustainable agricultural systems. With the power of real-time data and advanced analytics, agriculture is moving towards a more efficient and environmentally conscious future.
Food Quality Assessment
In today’s rapidly evolving agricultural landscape, the use of machine learning in food quality assessment has proven to be a game-changer. By leveraging advanced algorithms and data analysis techniques, machine learning offers new possibilities for evaluating and ensuring the safety and nutritional value of our food. From analyzing nutritional content to detecting pesticide residues and contaminants, machine learning is revolutionizing food quality assessment.
Analyzing Nutritional Content
Machine learning algorithms can process vast amounts of data to accurately analyze the nutritional content of various food items. By training models on extensive datasets, scientists and researchers can develop algorithms capable of determining the precise nutritional composition of a given food sample. This information is crucial for consumers monitoring their diets and for farmers seeking to optimize their crop production to cater to specific nutritional demands.
Detecting Pesticide Residues and Contaminants
One of the significant challenges in food production is the presence of pesticide residues and contaminants, which can have adverse effects on both human health and the environment. Machine learning models can be trained to identify and quantify these substances, helping farmers take proactive measures to ensure the safety of their crops, minimize the use of pesticides, and adhere to food safety regulations. By detecting and addressing potential issues at an early stage, machine learning empowers farmers to maintain food quality standards and provide safer products to consumers.
Ensuring Food Safety and Compliance
Machine learning also plays a crucial role in ensuring food safety and compliance with regulatory standards. By analyzing data from multiple sources, including remote sensing and real-time monitoring systems, machine learning algorithms can track environmental factors that may affect food quality, such as weather patterns and soil conditions. This proactive approach allows farmers to implement preventive measures and mitigate the risks of crop diseases, pest infestations, and other factors that could compromise food safety.
Benefits for Consumers and Farmers
The integration of machine learning in food quality assessment offers significant benefits for both consumers and farmers. For consumers, it provides assurance that the food they consume is safe, nutritious, and meets their dietary needs. By leveraging machine learning, farmers can optimize their farming practices, reduce crop loss, minimize the use of pesticides, and adopt more sustainable and environmentally friendly approaches. Ultimately, machine learning enhances the overall quality of the food supply chain, promoting food security and ensuring a healthier and more sustainable future.
In conclusion, machine learning is transforming food quality assessment by enabling accurate analysis of nutritional content, detection of pesticide residues and contaminants, and ensuring food safety and compliance. By harnessing the power of machine learning, we can enhance the quality, safety, and sustainability of our food supply, benefiting both consumers and farmers alike.
Farm Profitability Analysis
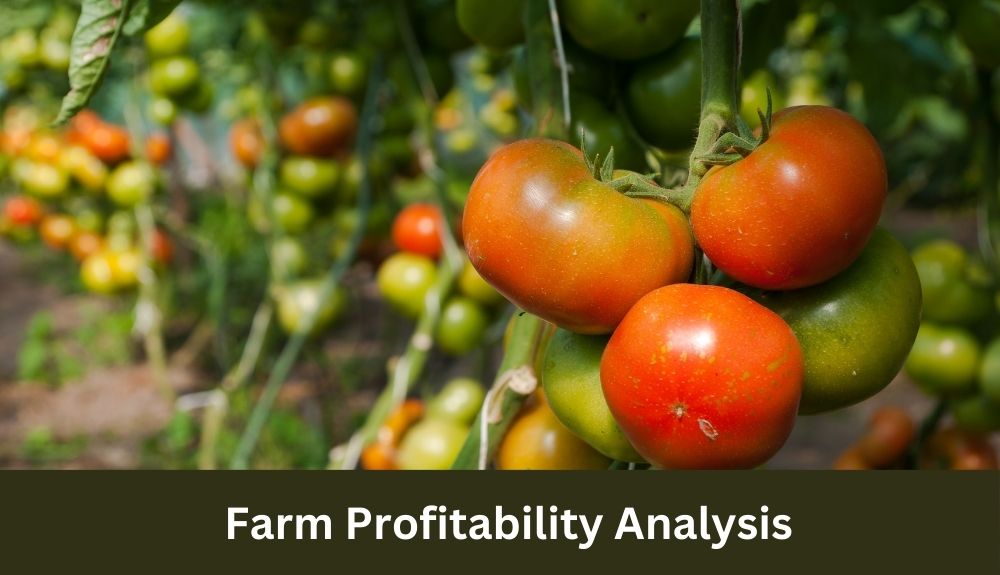
In today’s rapidly evolving agricultural landscape, farmers are constantly seeking ways to improve their profitability and make informed decisions. Machine learning algorithms offer a powerful tool to analyze various factors and drive farm profitability. By leveraging crop prices, market trends, and input costs, farmers can gain insights that enable them to optimize their operations and make data-driven decisions.
Analyzing Crop Prices and Market Trends
One key aspect of farm profitability analysis involves studying crop prices and market trends. Machine learning algorithms can process vast amounts of historical and real-time data to identify patterns and predict future price fluctuations. This information empowers farmers to make strategic decisions regarding crop selection, timing of sales, and market entry. For example, predictive models can help farmers identify the optimal time to sell their crops for maximum profit or determine which crops to cultivate based on market demand.
Optimizing Input Costs
Managing input costs is crucial for improving farm profitability. Machine learning algorithms can analyze data on fertilizer use, pest control methods, and irrigation practices to identify areas where costs can be minimized without compromising crop quality and yield. By optimizing resource allocation, farmers can reduce waste, improve efficiency, and ultimately increase their profit margins. AI-powered systems can provide recommendations on the most cost-effective and sustainable practices, helping farmers make informed decisions regarding inputs.
AI-Driven Farm Management Tools
The advent of AI-driven farm management tools has revolutionized the way farmers analyze profitability. These tools combine data analytics, machine learning, and advanced algorithms to provide comprehensive insights into various aspects of farm operations. For instance, AI-powered systems can assess the impact of weather patterns, climate change, and environmental factors on crop yield and profitability. By integrating data from sensors, remote sensing technologies, and satellite imagery, these tools offer farmers a holistic view of their operations and enable them to take proactive steps to mitigate risks and optimize outcomes.
Real-life examples of AI-driven farm management tools include AI-based credit scoring systems that help farmers access loans and financial services, AI algorithms that optimize water resource management by analyzing soil moisture levels and weather data, and AI-powered crop disease detection systems that enable early intervention and minimize crop losses. These tools empower farmers with actionable insights, enabling them to make informed decisions and enhance their profitability.
In conclusion, machine learning algorithms play a pivotal role in farm profitability analysis by analyzing crop prices, market trends, and input costs. By leveraging the power of AI-driven farm management tools, farmers can optimize their operations, minimize risks, and make data-driven decisions that ultimately enhance profitability. Embracing these technologies empowers farmers to thrive in a rapidly changing agricultural landscape and secure a sustainable future.
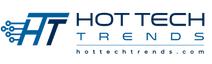