Creating trustworthy AI solutions for healthcare is a critical endeavor that combines cutting-edge technology with ethical responsibility to enhance patient care and improve health outcomes. As artificial intelligence continues to revolutionize the healthcare industry, it is imperative to ensure that these innovations are reliable, transparent, and aligned with the highest standards of patient safety and privacy. Trustworthy AI in healthcare involves rigorous validation of algorithms, adherence to regulatory standards, and the incorporation of ethical considerations to prevent biases and ensure equitable access to care. By fostering collaboration among technologists, healthcare professionals, and policymakers, we can develop AI solutions that not only advance medical research and diagnostics but also earn the trust of patients and practitioners alike, ultimately leading to a more effective and compassionate healthcare system.
Ensuring Data Privacy And Security In AI Healthcare Solutions
In the rapidly evolving landscape of healthcare, artificial intelligence (AI) has emerged as a transformative force, offering unprecedented opportunities to enhance patient care, streamline operations, and improve diagnostic accuracy. However, as AI solutions become increasingly integrated into healthcare systems, ensuring data privacy and security has become a paramount concern. The sensitive nature of healthcare data, coupled with the potential risks associated with data breaches, necessitates robust measures to protect patient information while leveraging AI’s capabilities.
To begin with, the foundation of trustworthy AI solutions in healthcare lies in the implementation of stringent data privacy protocols. Healthcare organizations must adhere to established regulations such as the Health Insurance Portability and Accountability Act (HIPAA) in the United States or the General Data Protection Regulation (GDPR) in Europe. These regulations provide a framework for safeguarding patient data, ensuring that AI systems are designed with privacy by default. By embedding privacy considerations into the development process, organizations can mitigate risks and build trust with patients and stakeholders.
Moreover, the adoption of advanced encryption techniques is crucial in protecting healthcare data from unauthorized access. Encryption ensures that data is transformed into a secure format that can only be accessed by authorized individuals. This is particularly important when data is transmitted between different systems or stored in cloud environments. By employing end-to-end encryption, healthcare providers can ensure that patient information remains confidential and secure throughout its lifecycle.
In addition to encryption, implementing robust access controls is essential for maintaining data security. Access controls determine who can view or modify data within an AI system, thereby preventing unauthorized access. Role-based access control (RBAC) is a widely used approach that assigns permissions based on an individual’s role within an organization. This ensures that only those with a legitimate need to access specific data can do so, reducing the risk of data breaches and unauthorized disclosures.
Furthermore, the use of anonymization and de-identification techniques can enhance data privacy in AI healthcare solutions. By removing or obfuscating personally identifiable information (PII), these techniques allow for the use of healthcare data in AI models without compromising patient privacy. This is particularly valuable in research and development, where large datasets are required to train AI algorithms. Anonymized data can be shared and analyzed without exposing sensitive patient information, thus facilitating innovation while maintaining privacy.
Another critical aspect of ensuring data privacy and security in AI healthcare solutions is continuous monitoring and auditing. Regular audits of AI systems can identify potential vulnerabilities and ensure compliance with privacy regulations. By proactively monitoring data access and usage, healthcare organizations can detect and respond to security incidents in a timely manner, minimizing potential damage.
Finally, fostering a culture of privacy and security awareness within healthcare organizations is essential. Training staff on data protection best practices and the importance of maintaining patient confidentiality can significantly reduce the risk of human error, which is often a leading cause of data breaches. By promoting a culture of vigilance and accountability, healthcare providers can ensure that all employees understand their role in safeguarding patient data.
In conclusion, as AI continues to revolutionize healthcare, ensuring data privacy and security remains a critical challenge. By implementing robust privacy protocols, employing advanced encryption and access controls, utilizing anonymization techniques, conducting regular audits, and fostering a culture of awareness, healthcare organizations can create trustworthy AI solutions that protect patient information while harnessing the transformative potential of AI.
Implementing Ethical Guidelines For AI In Healthcare
Implementing ethical guidelines for AI in healthcare is a critical endeavor that requires a multifaceted approach, ensuring that these advanced technologies are both effective and trustworthy. As artificial intelligence continues to revolutionize the healthcare industry, it is imperative to address the ethical considerations that accompany its integration. The potential of AI to enhance diagnostic accuracy, streamline administrative processes, and personalize patient care is immense. However, without a robust ethical framework, these benefits may be overshadowed by concerns related to privacy, bias, and accountability.
To begin with, the protection of patient privacy is paramount. AI systems in healthcare often rely on vast amounts of personal data to function effectively. Therefore, it is essential to implement stringent data protection measures. This includes ensuring compliance with existing regulations such as the Health Insurance Portability and Accountability Act (HIPAA) in the United States and the General Data Protection Regulation (GDPR) in Europe. Moreover, healthcare organizations must adopt advanced encryption techniques and anonymization processes to safeguard sensitive information. By prioritizing data privacy, healthcare providers can foster trust among patients, who may otherwise be hesitant to share their personal information.
In addition to privacy concerns, addressing bias in AI algorithms is crucial. AI systems are only as good as the data they are trained on, and if this data is biased, the outcomes can be skewed. This is particularly concerning in healthcare, where biased algorithms could lead to disparities in treatment and care. To mitigate this risk, it is essential to use diverse and representative datasets during the development of AI models. Furthermore, continuous monitoring and evaluation of AI systems are necessary to identify and rectify any biases that may emerge over time. By doing so, healthcare providers can ensure that AI solutions are equitable and do not inadvertently perpetuate existing inequalities.
Accountability is another key aspect of implementing ethical guidelines for AI in healthcare. As AI systems become more autonomous, determining responsibility for their actions becomes increasingly complex. It is vital to establish clear lines of accountability, ensuring that healthcare providers, AI developers, and other stakeholders understand their roles and responsibilities. This can be achieved through comprehensive documentation and transparent communication regarding the decision-making processes of AI systems. Additionally, involving interdisciplinary teams in the development and deployment of AI solutions can provide diverse perspectives and enhance accountability.
Furthermore, fostering collaboration between stakeholders is essential for the successful implementation of ethical guidelines. This includes engaging with policymakers, healthcare professionals, AI developers, and patients to create a shared understanding of the ethical implications of AI in healthcare. By facilitating open dialogue and collaboration, stakeholders can work together to develop guidelines that are both practical and effective. This collaborative approach can also help to build public trust in AI technologies, as stakeholders demonstrate their commitment to ethical practices.
In conclusion, implementing ethical guidelines for AI in healthcare is a complex but necessary task. By prioritizing patient privacy, addressing bias, ensuring accountability, and fostering collaboration, healthcare providers can create trustworthy AI solutions that enhance patient care while upholding ethical standards. As AI continues to evolve, ongoing efforts to refine and adapt these guidelines will be crucial in maintaining the delicate balance between innovation and ethics in the healthcare industry.
Building Transparent AI Models For Medical Applications
In the rapidly evolving landscape of healthcare, the integration of artificial intelligence (AI) presents both unprecedented opportunities and significant challenges. As AI technologies become increasingly embedded in medical applications, the need for transparency in these systems has never been more critical. Building transparent AI models is essential to ensure trustworthiness, reliability, and ethical compliance in healthcare solutions. This transparency is not merely a technical requirement but a fundamental aspect that influences the acceptance and effectiveness of AI in medical settings.
To begin with, transparency in AI models refers to the ability to understand and interpret how these systems make decisions. In healthcare, where decisions can have profound implications on patient outcomes, it is crucial that AI models operate in a manner that is both explainable and interpretable. This means that healthcare professionals should be able to comprehend the rationale behind AI-driven recommendations or diagnoses. By fostering an environment where AI decisions are transparent, healthcare providers can better trust and rely on these technologies, ultimately leading to improved patient care.
Moreover, transparency in AI models is closely linked to the concept of accountability. When AI systems are transparent, it becomes easier to identify and address errors or biases that may arise. This is particularly important in healthcare, where biases in AI models can lead to disparities in treatment and outcomes. By ensuring that AI models are transparent, developers can more effectively audit and refine these systems, thereby minimizing the risk of biased or erroneous outputs. This process not only enhances the reliability of AI solutions but also aligns with ethical standards that prioritize patient safety and equity.
In addition to accountability, transparency also facilitates collaboration between AI developers and healthcare professionals. When AI models are designed with transparency in mind, they become more accessible to clinicians who may not have a technical background. This accessibility allows for a more collaborative approach to AI implementation, where healthcare professionals can provide valuable insights and feedback to refine and optimize AI systems. Such collaboration is vital for creating AI solutions that are not only technically sound but also practically applicable in real-world medical scenarios.
Furthermore, regulatory compliance is another area where transparency plays a pivotal role. As regulatory bodies increasingly scrutinize AI applications in healthcare, transparent models are more likely to meet compliance standards. Transparent AI systems can provide clear documentation and evidence of their decision-making processes, which is essential for regulatory approval. This compliance is crucial for the widespread adoption of AI in healthcare, as it assures stakeholders that these technologies adhere to established guidelines and standards.
In conclusion, building transparent AI models for medical applications is a multifaceted endeavor that requires careful consideration of various factors. By prioritizing transparency, developers can create AI solutions that are trustworthy, accountable, and compliant with regulatory standards. This transparency not only enhances the reliability and effectiveness of AI in healthcare but also fosters a collaborative environment where healthcare professionals and AI developers can work together to improve patient outcomes. As the healthcare industry continues to embrace AI, the emphasis on transparency will be a guiding principle that ensures these technologies are used ethically and effectively, ultimately benefiting patients and healthcare providers alike.
Enhancing Patient-Provider Trust Through AI Technologies
In the rapidly evolving landscape of healthcare, the integration of artificial intelligence (AI) technologies has emerged as a transformative force, promising to enhance patient-provider trust significantly. As healthcare systems worldwide grapple with increasing demands and complexities, AI offers innovative solutions that can streamline processes, improve diagnostic accuracy, and personalize patient care. However, the successful implementation of AI in healthcare hinges on the establishment of trust between patients and providers, a critical component that ensures the ethical and effective use of these technologies.
To begin with, AI technologies have the potential to revolutionize patient-provider interactions by providing more accurate and timely information. For instance, AI algorithms can analyze vast amounts of medical data to identify patterns and predict outcomes with a level of precision that surpasses human capabilities. This can lead to earlier diagnoses and more effective treatment plans, thereby fostering trust as patients experience improved health outcomes. Moreover, AI can assist healthcare providers in making informed decisions by offering evidence-based recommendations, which can enhance the quality of care and reinforce the trust patients place in their providers.
Furthermore, AI can play a pivotal role in personalizing patient care, a factor that significantly contributes to building trust. By leveraging machine learning algorithms, AI systems can tailor treatment plans to individual patient needs, taking into account their unique medical histories, genetic profiles, and lifestyle factors. This personalized approach not only improves the efficacy of treatments but also demonstrates a commitment to patient-centered care, thereby strengthening the trust relationship. Patients are more likely to trust providers who understand their specific needs and offer customized solutions rather than a one-size-fits-all approach.
In addition to improving diagnostic and treatment processes, AI technologies can enhance transparency and communication, which are essential elements of trust. AI-driven platforms can facilitate better communication between patients and providers by providing clear and accessible information about medical conditions, treatment options, and potential outcomes. This transparency empowers patients to make informed decisions about their healthcare, fostering a sense of agency and trust. Moreover, AI can streamline administrative processes, reducing wait times and improving the overall patient experience, which further contributes to building trust.
However, the integration of AI in healthcare is not without challenges. Concerns about data privacy and security are paramount, as AI systems often require access to sensitive patient information. To address these concerns, it is crucial to implement robust data protection measures and ensure compliance with relevant regulations. By prioritizing data security, healthcare providers can alleviate patient fears and build trust in AI technologies. Additionally, ethical considerations must be at the forefront of AI development and deployment. Ensuring that AI systems are free from biases and operate transparently is essential to maintaining trust. This requires ongoing monitoring and evaluation of AI algorithms to ensure they deliver fair and unbiased outcomes.
In conclusion, the potential of AI technologies to enhance patient-provider trust in healthcare is immense. By improving diagnostic accuracy, personalizing care, and enhancing transparency, AI can significantly strengthen the trust relationship. However, realizing this potential requires a concerted effort to address data privacy concerns and ethical considerations. As healthcare systems continue to embrace AI, fostering trust will be paramount to ensuring that these technologies are used effectively and ethically, ultimately leading to better health outcomes for patients.
Validating AI Algorithms For Accurate Healthcare Outcomes
In the rapidly evolving landscape of healthcare, the integration of artificial intelligence (AI) presents both unprecedented opportunities and significant challenges. As AI technologies become increasingly sophisticated, their potential to revolutionize healthcare delivery is immense. However, ensuring that these AI solutions are trustworthy and reliable is paramount, particularly when it comes to validating AI algorithms for accurate healthcare outcomes. The process of validation is crucial, as it determines the efficacy and safety of AI applications in clinical settings.
To begin with, the validation of AI algorithms in healthcare involves rigorous testing and evaluation to ensure that they perform as intended. This process is essential to establish the credibility of AI systems, as it provides evidence that these technologies can deliver accurate and reliable results. Validation typically involves a series of steps, including data collection, algorithm training, and performance assessment. Each of these steps must be meticulously executed to ensure that the AI system is robust and capable of handling the complexities of real-world healthcare scenarios.
One of the primary challenges in validating AI algorithms is the quality and diversity of the data used for training. High-quality data is the foundation of any successful AI system, as it directly influences the algorithm’s ability to learn and make accurate predictions. In healthcare, data must be comprehensive and representative of diverse patient populations to avoid biases that could lead to erroneous outcomes. Therefore, it is imperative to use datasets that encompass a wide range of demographic and clinical variables. This diversity ensures that the AI system can generalize its findings across different patient groups, thereby enhancing its reliability and trustworthiness.
Moreover, transparency in the development and validation process is critical to building trust in AI solutions. Stakeholders, including healthcare providers, patients, and regulatory bodies, must have confidence in the AI system’s decision-making processes. This requires clear documentation of the algorithm’s design, the data used, and the validation methods employed. By providing transparency, developers can facilitate a better understanding of how AI systems operate, which in turn fosters trust and acceptance among users.
In addition to transparency, collaboration between AI developers and healthcare professionals is essential for successful validation. Healthcare experts bring invaluable insights into clinical workflows and patient care, which can inform the design and implementation of AI systems. By working together, developers and clinicians can ensure that AI solutions are not only technically sound but also aligned with the practical needs of healthcare settings. This collaborative approach helps to identify potential pitfalls early in the development process, thereby reducing the risk of errors and improving the overall quality of the AI system.
Furthermore, continuous monitoring and evaluation of AI systems post-deployment are vital to maintaining their accuracy and reliability. The healthcare environment is dynamic, with new medical knowledge and practices emerging regularly. AI systems must be adaptable to these changes to remain effective. Ongoing validation efforts, including real-world testing and feedback loops, enable developers to refine algorithms and address any issues that may arise over time. This iterative process ensures that AI solutions remain relevant and continue to deliver accurate healthcare outcomes.
In conclusion, the validation of AI algorithms is a critical component in creating trustworthy AI solutions for healthcare. By focusing on data quality, transparency, collaboration, and continuous evaluation, developers can build AI systems that are not only accurate but also reliable and accepted by the healthcare community. As AI continues to transform healthcare, these validation practices will play a pivotal role in ensuring that AI technologies contribute positively to patient care and outcomes.
Addressing Bias And Fairness In AI-Driven Healthcare Systems
In the rapidly evolving landscape of healthcare, artificial intelligence (AI) has emerged as a transformative force, offering unprecedented opportunities to enhance patient care, streamline operations, and improve diagnostic accuracy. However, as AI-driven healthcare systems become more prevalent, addressing issues of bias and fairness has become a critical concern. Ensuring that these systems are trustworthy requires a concerted effort to identify, understand, and mitigate biases that may inadvertently arise during the development and deployment of AI technologies.
Bias in AI can manifest in various forms, often stemming from the data used to train these systems. Historical data, which serves as the foundation for AI algorithms, may reflect existing societal biases, leading to skewed outcomes that disproportionately affect certain groups. For instance, if a dataset predominantly represents a specific demographic, the AI system may perform well for that group but poorly for others, resulting in unequal healthcare delivery. Consequently, it is imperative to ensure that training data is diverse and representative of the entire population to minimize the risk of bias.
Moreover, the complexity of AI algorithms can obscure the decision-making process, making it challenging to identify and rectify biases. This opacity, often referred to as the “black box” problem, necessitates the development of explainable AI models that provide transparency into how decisions are made. By enhancing the interpretability of AI systems, healthcare providers can better understand the factors influencing outcomes and take corrective actions when biases are detected. This transparency not only fosters trust among healthcare professionals but also empowers patients to make informed decisions about their care.
In addition to data diversity and algorithmic transparency, the involvement of multidisciplinary teams is crucial in addressing bias and fairness in AI-driven healthcare systems. Collaboration among data scientists, healthcare professionals, ethicists, and patient advocates can provide a holistic perspective on potential biases and their implications. By incorporating diverse viewpoints, these teams can identify biases that may not be immediately apparent and develop strategies to mitigate them effectively. Furthermore, engaging with patients and communities can offer valuable insights into their unique needs and concerns, ensuring that AI solutions are equitable and inclusive.
Regulatory frameworks also play a vital role in promoting fairness in AI-driven healthcare systems. Policymakers and regulatory bodies must establish guidelines that mandate rigorous testing and validation of AI technologies to ensure they meet ethical and fairness standards. These regulations should emphasize accountability, requiring developers to demonstrate that their systems have been thoroughly evaluated for bias and that mechanisms are in place to address any disparities that arise. By holding developers accountable, regulatory frameworks can incentivize the creation of AI solutions that prioritize fairness and equity.
Finally, continuous monitoring and evaluation are essential to maintaining fairness in AI-driven healthcare systems. As these technologies are deployed in real-world settings, ongoing assessment is necessary to identify new biases that may emerge over time. Implementing feedback loops that allow for the regular review and refinement of AI models can help ensure that they remain fair and effective. By fostering a culture of continuous improvement, healthcare organizations can adapt to changing circumstances and uphold the principles of fairness and equity in their AI-driven initiatives.
In conclusion, creating trustworthy AI solutions for healthcare requires a multifaceted approach to addressing bias and fairness. By prioritizing data diversity, algorithmic transparency, multidisciplinary collaboration, regulatory oversight, and continuous evaluation, stakeholders can develop AI-driven healthcare systems that are equitable, inclusive, and capable of delivering high-quality care to all patients. As the healthcare industry continues to embrace AI, these efforts will be instrumental in building trust and ensuring that technological advancements benefit everyone, regardless of their background or circumstances.
Q&A
1. **What is a key consideration for creating trustworthy AI in healthcare?**
Ensuring data privacy and security is a key consideration, as healthcare data is highly sensitive and must be protected to maintain patient trust.
2. **How can bias be minimized in AI healthcare solutions?**
Bias can be minimized by using diverse and representative datasets during the training phase and continuously monitoring AI outputs for any signs of bias.
3. **Why is transparency important in AI healthcare solutions?**
Transparency is crucial because it allows healthcare professionals to understand how AI systems make decisions, which is essential for trust and accountability.
4. **What role does regulatory compliance play in trustworthy AI for healthcare?**
Adhering to regulatory standards, such as HIPAA in the U.S., ensures that AI solutions meet legal requirements for patient safety and data protection.
5. **How can AI solutions be validated in the healthcare context?**
AI solutions can be validated through rigorous clinical trials and peer-reviewed studies to ensure their efficacy and safety in real-world healthcare settings.
6. **What is the importance of involving healthcare professionals in AI development?**
Involving healthcare professionals ensures that AI solutions are practical, relevant, and aligned with clinical needs, which enhances their trustworthiness and adoption.Creating trustworthy AI solutions for healthcare is essential to ensure patient safety, enhance clinical outcomes, and foster public confidence in AI technologies. Trustworthy AI in healthcare must prioritize ethical considerations, including patient privacy, data security, and transparency in AI decision-making processes. It requires rigorous validation and testing to ensure accuracy and reliability, as well as compliance with regulatory standards. Collaboration between technologists, healthcare professionals, and policymakers is crucial to address potential biases and ensure equitable access to AI benefits. By focusing on these principles, the healthcare industry can harness the potential of AI to improve patient care while maintaining trust and integrity.
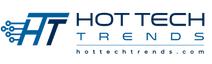