RT-2, or Robotics Transformer 2, is an advanced AI model designed to bridge the gap between visual and linguistic inputs and actionable outputs. Building upon its predecessor, RT-2 leverages cutting-edge transformer architecture to process and integrate diverse data types, enabling it to understand and execute complex tasks in real-world environments. By converting visual cues and language instructions into precise actions, RT-2 represents a significant leap forward in robotics and AI, enhancing the ability of machines to interact seamlessly with their surroundings and perform tasks with greater autonomy and accuracy.
Understanding RT-2: Bridging Visual and Linguistic Inputs for Actionable Insights
RT-2 represents a significant advancement in the field of artificial intelligence, particularly in its ability to seamlessly integrate visual and linguistic inputs to generate actionable insights. This model, developed through extensive research and innovation, is designed to process and interpret complex data from both visual and textual sources, thereby enabling it to perform tasks that require a nuanced understanding of the world. The development of RT-2 is rooted in the need for AI systems that can operate in environments where information is not solely text-based or image-based but a combination of both. This dual-input capability allows RT-2 to function more effectively in real-world scenarios, where the ability to interpret and act upon diverse data types is crucial.
At the core of RT-2’s functionality is its sophisticated architecture, which integrates advanced machine learning techniques to process and synthesize information. By leveraging deep learning algorithms, RT-2 can analyze visual data, such as images or videos, and extract meaningful features that are relevant to the task at hand. Simultaneously, it processes linguistic inputs, understanding context, semantics, and the nuances of human language. This dual processing capability is what sets RT-2 apart from traditional models that are limited to either visual or linguistic data. The model’s ability to bridge these two domains is achieved through a series of interconnected neural networks that work in tandem to ensure that the information from both inputs is harmonized and utilized effectively.
Moreover, RT-2’s design incorporates a feedback mechanism that allows it to learn from its actions and improve over time. This iterative learning process is essential for refining the model’s performance and ensuring that it can adapt to new and unforeseen situations. As RT-2 encounters various scenarios, it continuously updates its knowledge base, enhancing its ability to make informed decisions. This adaptability is particularly important in dynamic environments where conditions can change rapidly, requiring the model to respond with agility and precision.
In addition to its technical capabilities, RT-2 is also designed with ethical considerations in mind. The development team has prioritized transparency and accountability, ensuring that the model’s decision-making processes are understandable and traceable. This focus on ethical AI is crucial in building trust with users and stakeholders, as it addresses concerns about the potential misuse or unintended consequences of advanced AI systems. By incorporating ethical guidelines into its design, RT-2 aims to set a standard for responsible AI development.
Furthermore, the potential applications of RT-2 are vast and varied, spanning industries such as healthcare, autonomous vehicles, and robotics. In healthcare, for instance, RT-2 could assist in diagnosing medical conditions by analyzing visual data from medical imaging alongside patient records. In the realm of autonomous vehicles, the model could enhance navigation systems by interpreting road signs and spoken instructions simultaneously. Similarly, in robotics, RT-2 could enable machines to perform complex tasks by understanding both visual cues and verbal commands.
In conclusion, RT-2 represents a groundbreaking step forward in the integration of visual and linguistic inputs for actionable insights. Its advanced architecture, coupled with its ability to learn and adapt, positions it as a powerful tool in the evolving landscape of artificial intelligence. As RT-2 continues to develop and refine its capabilities, it holds the promise of transforming how AI systems interact with the world, offering new possibilities for innovation and problem-solving across various sectors.
The Evolution of AI Models: How RT-2 Enhances Interaction with Multimodal Data
The evolution of artificial intelligence models has been marked by significant advancements in their ability to process and interpret complex data. One of the most recent breakthroughs in this field is the development of RT-2, an advanced model that seamlessly converts visual and linguistic inputs into actionable outputs. This innovation represents a significant leap forward in enhancing interaction with multimodal data, a capability that is increasingly crucial in today’s data-driven world.
To understand the significance of RT-2, it is essential to consider the context of its development. Traditional AI models have typically been designed to handle either visual or linguistic data, but not both simultaneously. This limitation has often resulted in fragmented processing capabilities, where models excel in one domain but falter in another. However, the demand for more integrated solutions has driven researchers to explore models that can effectively process and interpret multiple types of data inputs. RT-2 emerges as a response to this demand, offering a sophisticated approach to multimodal data processing.
RT-2’s ability to convert visual and linguistic inputs into actions is rooted in its advanced architecture, which integrates state-of-the-art techniques from both computer vision and natural language processing. By leveraging these techniques, RT-2 can analyze images and text concurrently, extracting meaningful insights from each and synthesizing them into coherent actions. This capability is particularly valuable in applications where understanding context from both visual and textual information is crucial, such as in autonomous systems, human-computer interaction, and assistive technologies.
Moreover, the model’s design incorporates a robust learning framework that enables it to adapt to diverse data inputs and scenarios. This adaptability is achieved through a combination of supervised and unsupervised learning methods, allowing RT-2 to refine its understanding of complex data patterns over time. As a result, the model can improve its performance with continued exposure to new data, making it a dynamic tool for real-world applications.
In addition to its technical prowess, RT-2 also addresses some of the ethical and practical challenges associated with AI models. By providing a more holistic understanding of multimodal data, RT-2 can contribute to more transparent and explainable AI systems. This transparency is crucial for building trust with users and ensuring that AI-driven decisions are fair and accountable. Furthermore, the model’s ability to process diverse data types can help mitigate biases that may arise from relying solely on one form of data, thereby promoting more equitable outcomes.
As we look to the future, the implications of RT-2’s capabilities are vast. Its potential applications span a wide range of industries, from healthcare and education to entertainment and beyond. For instance, in healthcare, RT-2 could enhance diagnostic tools by integrating patient records with medical imaging, leading to more accurate and timely diagnoses. In education, the model could facilitate personalized learning experiences by analyzing students’ interactions with both visual and textual content.
In conclusion, RT-2 represents a significant advancement in the evolution of AI models, offering a powerful solution for interacting with multimodal data. Its ability to convert visual and linguistic inputs into actions not only enhances the functionality of AI systems but also addresses critical challenges related to transparency and bias. As researchers continue to refine and expand upon this technology, RT-2 is poised to play a pivotal role in shaping the future of artificial intelligence, driving innovation across various sectors and improving the way we interact with complex data.
Practical Applications of RT-2 in Real-World Scenarios
The development of RT-2, an advanced model capable of converting visual and linguistic inputs into actions, marks a significant milestone in the field of artificial intelligence. This innovative model has the potential to revolutionize various industries by enhancing the way machines interact with their environment. As we explore the practical applications of RT-2 in real-world scenarios, it becomes evident that its capabilities extend far beyond theoretical constructs, offering tangible benefits across multiple domains.
One of the most promising applications of RT-2 is in the realm of autonomous vehicles. By integrating visual and linguistic inputs, RT-2 can enable vehicles to better understand and respond to complex driving environments. For instance, the model can process visual data from cameras and sensors to identify road signs, pedestrians, and other vehicles, while simultaneously interpreting verbal instructions or traffic updates. This dual-input processing allows for more accurate decision-making, ultimately enhancing the safety and efficiency of autonomous driving systems. Moreover, RT-2’s ability to learn from diverse data sources means that it can adapt to different driving conditions and regulations across various regions, making it a versatile tool for global deployment.
In addition to transportation, RT-2 holds significant potential in the healthcare sector. Medical professionals can leverage this model to improve diagnostic processes and patient care. For example, RT-2 can analyze medical images, such as X-rays or MRIs, alongside patient records and clinical notes to provide comprehensive insights into a patient’s condition. This integration of visual and linguistic data can lead to more accurate diagnoses and personalized treatment plans. Furthermore, RT-2 can assist in surgical procedures by interpreting visual feeds from cameras and responding to verbal commands from surgeons, thereby enhancing precision and reducing the likelihood of human error.
The retail industry also stands to benefit from the implementation of RT-2. Retailers can utilize this model to enhance customer experiences through more intuitive and responsive service systems. For instance, RT-2 can process visual data from in-store cameras to monitor customer behavior and preferences, while simultaneously interpreting verbal feedback or inquiries. This capability allows retailers to tailor their offerings and improve customer satisfaction. Additionally, RT-2 can be employed in inventory management, where it can analyze visual inputs from warehouse cameras and linguistic data from inventory databases to optimize stock levels and streamline supply chain operations.
Moreover, RT-2’s applications extend to the field of robotics, where it can significantly enhance the functionality and adaptability of robotic systems. By processing visual and linguistic inputs, robots equipped with RT-2 can perform complex tasks in dynamic environments. For example, in manufacturing settings, robots can interpret visual cues from assembly lines and respond to verbal instructions from human operators, leading to more efficient and flexible production processes. This capability is particularly valuable in industries that require high levels of customization and rapid adaptation to changing demands.
In conclusion, the practical applications of RT-2 in real-world scenarios are vast and varied, offering transformative potential across multiple sectors. By seamlessly integrating visual and linguistic inputs, this advanced model enables machines to interact with their environment in more sophisticated and meaningful ways. As industries continue to explore and implement RT-2, the benefits of this technology will become increasingly apparent, paving the way for a future where artificial intelligence plays an integral role in enhancing human capabilities and improving quality of life.
RT-2’s Impact on Robotics: From Perception to Action
The development of RT-2, an advanced model capable of converting visual and linguistic inputs into actions, marks a significant milestone in the field of robotics. This innovative model represents a leap forward in the integration of perception and action, a crucial aspect of robotic functionality. By seamlessly bridging the gap between understanding and execution, RT-2 enhances the ability of robots to interact with their environment in a more intuitive and efficient manner.
At the core of RT-2’s functionality is its sophisticated ability to process and interpret complex data inputs. Unlike traditional models that often require separate systems for visual and linguistic processing, RT-2 integrates these capabilities into a unified framework. This integration allows the model to analyze visual cues and linguistic commands simultaneously, thereby enabling a more cohesive understanding of tasks. For instance, when a robot equipped with RT-2 is given a command to “pick up the red ball,” it can visually identify the object and execute the action with remarkable precision. This seamless transition from perception to action is a testament to the model’s advanced design.
Moreover, RT-2’s impact extends beyond mere task execution. The model’s ability to learn from diverse data inputs allows it to adapt to new environments and tasks with minimal reprogramming. This adaptability is particularly valuable in dynamic settings where robots must respond to changing conditions. By leveraging machine learning techniques, RT-2 can refine its understanding of tasks over time, improving its performance and efficiency. This continuous learning process not only enhances the robot’s capabilities but also reduces the need for constant human intervention, thereby increasing operational autonomy.
In addition to its technical prowess, RT-2 also addresses some of the longstanding challenges in robotics, such as the need for context-aware decision-making. Traditional robotic systems often struggle with tasks that require an understanding of context or nuanced instructions. However, RT-2’s integrated approach allows it to consider contextual information when interpreting commands, leading to more accurate and contextually appropriate actions. This capability is particularly beneficial in complex environments where robots must navigate intricate scenarios, such as healthcare settings or disaster response operations.
Furthermore, the implications of RT-2’s advancements are far-reaching, with potential applications across various industries. In manufacturing, for example, robots equipped with RT-2 can streamline production processes by efficiently handling tasks that require both precision and adaptability. In the service sector, these robots can enhance customer interactions by understanding and responding to verbal requests with greater accuracy. Additionally, in fields such as agriculture and logistics, RT-2 can optimize operations by enabling robots to perform tasks that require a high degree of situational awareness and adaptability.
In conclusion, the introduction of RT-2 represents a transformative step in the evolution of robotics. By effectively merging visual and linguistic inputs into actionable outputs, this advanced model enhances the capability of robots to perform complex tasks with greater autonomy and precision. As RT-2 continues to evolve and improve, it holds the promise of revolutionizing the way robots interact with their environment, ultimately paving the way for more intelligent and versatile robotic systems. The potential applications of RT-2 are vast, and its impact on the robotics industry is poised to be profound, ushering in a new era of innovation and efficiency.
Challenges and Opportunities in Developing RT-2 for Multimodal Integration
The development of RT-2, an advanced model designed to convert visual and linguistic inputs into actionable outputs, presents both significant challenges and promising opportunities in the realm of multimodal integration. As technology continues to evolve, the demand for systems capable of processing and integrating diverse types of data has grown exponentially. RT-2 stands at the forefront of this evolution, aiming to bridge the gap between visual perception and linguistic comprehension to facilitate more intuitive human-computer interactions.
One of the primary challenges in developing RT-2 lies in the complexity of integrating visual and linguistic data streams. Visual data, characterized by its rich and often ambiguous nature, requires sophisticated processing to extract meaningful information. Simultaneously, linguistic data demands a nuanced understanding of context, semantics, and syntax. Merging these two distinct modalities into a cohesive framework necessitates advanced algorithms capable of handling the intricacies of each data type while ensuring seamless interaction between them. This integration is further complicated by the need for real-time processing, which requires the model to operate efficiently without compromising accuracy.
Moreover, the variability inherent in both visual and linguistic inputs poses another significant hurdle. Visual inputs can vary widely in terms of lighting, perspective, and quality, while linguistic inputs can differ in dialect, tone, and complexity. Developing a model that can robustly handle such variability is crucial for ensuring the reliability and versatility of RT-2. This challenge is compounded by the need for the model to generalize across different contexts and applications, from autonomous vehicles to assistive technologies, each with its unique set of requirements and constraints.
Despite these challenges, the development of RT-2 offers numerous opportunities for innovation and advancement. By successfully integrating visual and linguistic inputs, RT-2 has the potential to revolutionize how machines interpret and respond to the world around them. This capability could lead to significant improvements in fields such as robotics, where the ability to understand and act upon complex, multimodal information is essential for performing tasks in dynamic environments. Additionally, RT-2 could enhance human-computer interaction by enabling more natural and intuitive communication, thereby reducing the cognitive load on users and making technology more accessible to a broader audience.
Furthermore, the insights gained from developing RT-2 could inform future research in artificial intelligence and machine learning. The techniques and methodologies employed in creating this model could be applied to other areas of study, fostering cross-disciplinary collaboration and innovation. As researchers continue to explore the potential of multimodal integration, the lessons learned from RT-2 could pave the way for new approaches to data processing and interpretation, ultimately contributing to the advancement of intelligent systems.
In conclusion, while the development of RT-2 presents a range of challenges, particularly in terms of integrating and processing diverse data types, it also offers exciting opportunities for progress in multimodal integration. By addressing these challenges and leveraging the potential of RT-2, researchers and developers can unlock new possibilities for technology that is more responsive, intuitive, and capable of understanding the complexities of the world. As we continue to push the boundaries of what is possible, RT-2 stands as a testament to the power of innovation and the promise of a future where machines and humans can interact more seamlessly than ever before.
Future Prospects: How RT-2 Could Transform Human-Computer Interaction
The development of RT-2, an advanced model capable of converting visual and linguistic inputs into actions, marks a significant milestone in the evolution of human-computer interaction. This innovative technology has the potential to revolutionize the way humans interact with machines, offering a more intuitive and seamless experience. As we explore the future prospects of RT-2, it is essential to consider how this model could transform various aspects of our daily lives and industries.
To begin with, RT-2’s ability to process and integrate both visual and linguistic information allows for a more natural interaction between humans and computers. Unlike traditional models that rely on predefined commands or limited input types, RT-2 can understand and respond to complex instructions that combine visual cues with verbal commands. This capability opens up new possibilities for creating more responsive and adaptive systems that can better understand and anticipate user needs. For instance, in the realm of personal assistants, RT-2 could enable devices to perform tasks based on a combination of spoken instructions and visual context, such as identifying objects in a room and executing related actions.
Moreover, the implications of RT-2 extend beyond personal use, offering transformative potential in various industries. In healthcare, for example, this model could enhance diagnostic tools by allowing medical professionals to interact with systems that can interpret both visual data, such as medical images, and linguistic inputs, like patient descriptions. This integration could lead to more accurate diagnoses and personalized treatment plans. Similarly, in the field of education, RT-2 could facilitate more interactive and engaging learning experiences. By understanding both visual content and verbal explanations, educational software could provide tailored feedback and support to students, adapting to their individual learning styles and needs.
Furthermore, the integration of RT-2 into robotics could significantly advance automation and efficiency in manufacturing and logistics. Robots equipped with this model could interpret complex instructions that involve both visual and verbal elements, enabling them to perform tasks with greater precision and adaptability. This capability could lead to more flexible production lines and improved quality control processes, ultimately enhancing productivity and reducing operational costs.
In addition to these industry-specific applications, RT-2 holds promise for improving accessibility and inclusivity in technology. By enabling more intuitive interactions, this model could make digital interfaces more accessible to individuals with disabilities, such as those with visual or hearing impairments. For example, RT-2 could facilitate the development of assistive technologies that translate visual information into verbal descriptions or vice versa, empowering users to engage with digital content more effectively.
As we look to the future, it is clear that the potential of RT-2 to transform human-computer interaction is vast. However, it is also important to consider the ethical implications and challenges associated with its deployment. Ensuring data privacy, addressing biases in model training, and maintaining transparency in decision-making processes are critical considerations that must be addressed to harness the full potential of this technology responsibly.
In conclusion, RT-2 represents a significant advancement in the field of human-computer interaction, offering the potential to create more intuitive, responsive, and inclusive systems. As this technology continues to evolve, it is poised to transform various industries and improve the way we interact with machines, ultimately enhancing our daily lives and expanding the possibilities of what technology can achieve.
Q&A
1. **What is RT-2?**
RT-2 (Robotic Transformer 2) is an advanced AI model developed by Google DeepMind that integrates vision and language understanding to enable robots to perform complex tasks by converting visual and linguistic inputs into actions.
2. **How does RT-2 work?**
RT-2 uses a transformer-based architecture to process both visual and textual data, allowing it to understand and interpret complex instructions and scenarios, and then translate this understanding into actionable steps for robotic systems.
3. **What are the key features of RT-2?**
Key features include its ability to generalize from limited data, integrate multimodal inputs, and perform zero-shot learning, which allows it to execute tasks it hasn’t been explicitly trained on by leveraging its understanding of language and vision.
4. **What are the applications of RT-2?**
RT-2 can be applied in various fields such as autonomous robotics, industrial automation, and assistive technologies, where robots need to understand and act upon complex instructions in dynamic environments.
5. **What advancements does RT-2 offer over previous models?**
RT-2 offers improved generalization capabilities, better integration of multimodal data, and enhanced performance in executing complex tasks without extensive retraining, making it more versatile and efficient than its predecessors.
6. **What challenges does RT-2 address?**
RT-2 addresses challenges related to the integration of visual and linguistic data, enabling robots to perform tasks with higher accuracy and adaptability, and reducing the need for extensive task-specific training data.RT-2, or Robotics Transformer 2, represents a significant advancement in the field of robotics by integrating visual and linguistic inputs to generate actionable outputs. This model leverages the capabilities of large-scale transformer architectures to process and understand complex multimodal data, enabling robots to perform tasks with greater autonomy and adaptability. By converting visual cues and language instructions into precise actions, RT-2 enhances the robot’s ability to interact with and respond to its environment in a more human-like manner. This development not only improves the efficiency and versatility of robotic systems but also paves the way for more intuitive human-robot interactions, potentially transforming various industries by automating complex tasks that require a nuanced understanding of both visual and linguistic information.
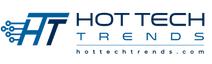