Innovative AI training methods are at the forefront of addressing contemporary challenges, revolutionizing the way artificial intelligence systems learn and adapt. As the demand for more sophisticated and efficient AI solutions grows, researchers and developers are exploring novel approaches to enhance the training process. These methods include leveraging advanced machine learning algorithms, incorporating large-scale data sets, and utilizing cutting-edge technologies such as reinforcement learning and transfer learning. By focusing on improving the accuracy, efficiency, and adaptability of AI models, these innovative training techniques are poised to tackle pressing issues across various sectors, from healthcare and finance to autonomous systems and natural language processing. As a result, they hold the potential to significantly impact the development of AI, ensuring it meets the complex demands of the modern world.
Personalized Learning Pathways In AI Training
In recent years, the field of artificial intelligence (AI) has witnessed remarkable advancements, driven by the need to address complex challenges across various sectors. One of the most significant developments in this domain is the emergence of personalized learning pathways in AI training. These innovative methods are designed to cater to the unique needs and learning paces of individual learners, thereby enhancing the overall effectiveness of AI education. As the demand for skilled AI professionals continues to grow, personalized learning pathways offer a promising solution to bridge the gap between traditional education models and the dynamic requirements of the AI industry.
To begin with, personalized learning pathways leverage data-driven insights to tailor educational content and experiences to the specific needs of each learner. This approach contrasts sharply with conventional one-size-fits-all models, which often fail to accommodate the diverse backgrounds and learning styles of students. By utilizing advanced algorithms and machine learning techniques, personalized learning systems can analyze a learner’s progress, strengths, and weaknesses in real-time. Consequently, these systems can adapt the curriculum to focus on areas that require improvement while reinforcing concepts that have already been mastered.
Moreover, personalized learning pathways in AI training are not limited to content customization. They also incorporate adaptive assessment methods that provide immediate feedback to learners. This continuous feedback loop enables students to identify their mistakes and misconceptions promptly, fostering a deeper understanding of complex AI concepts. Furthermore, adaptive assessments can adjust the difficulty level of questions based on the learner’s performance, ensuring that they are neither overwhelmed nor under-challenged. This dynamic approach to assessment not only enhances learning outcomes but also boosts learner engagement and motivation.
In addition to content and assessment customization, personalized learning pathways often include mentorship and peer collaboration components. These elements are crucial in creating a supportive learning environment where students can exchange ideas, seek guidance, and gain diverse perspectives. Mentorship programs connect learners with experienced AI professionals who can provide valuable insights and career advice. Meanwhile, collaborative projects encourage teamwork and problem-solving skills, which are essential in real-world AI applications. By fostering a sense of community and collaboration, personalized learning pathways help learners develop both technical and interpersonal skills.
Furthermore, the integration of personalized learning pathways in AI training is facilitated by the proliferation of online learning platforms and digital resources. These platforms offer a wide range of interactive tools and multimedia content that cater to different learning preferences. For instance, learners can access video lectures, interactive simulations, and coding exercises that provide hands-on experience with AI technologies. The flexibility of online learning also allows students to progress at their own pace, accommodating their individual schedules and commitments.
As we look to the future, the potential of personalized learning pathways in AI training is immense. By harnessing the power of AI itself, these methods can continuously evolve and improve, offering increasingly sophisticated and effective learning experiences. As a result, they hold the promise of producing a new generation of AI professionals who are not only technically proficient but also adaptable and innovative thinkers. In conclusion, personalized learning pathways represent a significant step forward in addressing the present-day challenges of AI education, ensuring that learners are well-equipped to meet the demands of an ever-changing technological landscape.
Integrating Real-World Scenarios In AI Curriculum
In recent years, the field of artificial intelligence (AI) has witnessed remarkable advancements, driven by the need to address complex real-world challenges. As AI systems become increasingly integrated into various sectors, the demand for innovative training methods that incorporate real-world scenarios has grown significantly. This approach not only enhances the practical applicability of AI models but also ensures that they are equipped to handle the intricacies of dynamic environments. Consequently, integrating real-world scenarios into AI curricula has emerged as a pivotal strategy in preparing AI systems to meet present-day challenges effectively.
To begin with, the integration of real-world scenarios into AI training involves the use of diverse datasets that reflect the complexities and nuances of actual environments. Traditional AI training often relies on static datasets that may not capture the variability and unpredictability of real-world situations. By contrast, incorporating dynamic datasets that simulate real-life conditions allows AI models to learn from a broader spectrum of experiences. This approach enhances the models’ ability to generalize and adapt to new situations, thereby improving their robustness and reliability in practical applications.
Moreover, the inclusion of real-world scenarios in AI curricula fosters a deeper understanding of context, which is crucial for effective decision-making. AI systems trained in controlled environments may struggle to interpret contextual cues when deployed in the real world. By exposing these systems to scenarios that mimic real-life conditions, they can develop a more nuanced understanding of context, enabling them to make more informed and accurate decisions. This is particularly important in fields such as healthcare, autonomous driving, and finance, where contextual awareness is essential for ensuring safety and efficacy.
In addition to enhancing contextual understanding, integrating real-world scenarios into AI training also promotes ethical and responsible AI development. Real-world environments often present ethical dilemmas and challenges that require careful consideration. By incorporating these scenarios into AI curricula, developers can instill a sense of ethical responsibility in AI systems, ensuring that they adhere to societal norms and values. This approach not only mitigates the risk of unintended consequences but also fosters public trust in AI technologies.
Furthermore, the use of real-world scenarios in AI training encourages interdisciplinary collaboration, which is vital for addressing complex challenges. Real-world problems often span multiple domains, requiring expertise from various fields to develop comprehensive solutions. By integrating scenarios that reflect this complexity, AI curricula can facilitate collaboration between computer scientists, domain experts, and other stakeholders. This collaborative approach not only enriches the training process but also leads to the development of more holistic and effective AI solutions.
Finally, the integration of real-world scenarios into AI training is instrumental in bridging the gap between research and application. While theoretical advancements in AI are crucial, their practical implementation often presents unique challenges. By incorporating real-world scenarios into the training process, researchers can identify and address potential obstacles early on, ensuring that AI models are not only theoretically sound but also practically viable. This alignment between research and application accelerates the deployment of AI technologies, enabling them to address pressing societal challenges more effectively.
In conclusion, the integration of real-world scenarios into AI curricula represents a significant step forward in the development of robust, context-aware, and ethically responsible AI systems. By enhancing the practical applicability of AI models, fostering interdisciplinary collaboration, and bridging the gap between research and application, this approach equips AI systems to tackle present-day challenges with greater efficacy. As the field of AI continues to evolve, the incorporation of real-world scenarios will undoubtedly play a crucial role in shaping the future of AI development and deployment.
Collaborative AI Training Through Peer Learning
In the rapidly evolving landscape of artificial intelligence, innovative training methods are essential to address the complex challenges of the present day. One such method gaining traction is collaborative AI training through peer learning. This approach leverages the collective intelligence of multiple AI systems, enabling them to learn from each other in a manner akin to human peer learning. By fostering an environment where AI models can exchange knowledge and insights, this method not only enhances the learning process but also accelerates the development of more robust and versatile AI systems.
At the core of collaborative AI training is the concept of mutual learning, where AI models are designed to interact and share information. This interaction is facilitated through a structured framework that allows models to communicate their findings, errors, and strategies. As a result, each model benefits from the experiences and perspectives of its peers, leading to a more comprehensive understanding of the tasks at hand. This is particularly beneficial in scenarios where data is scarce or where the complexity of the problem requires diverse approaches.
Moreover, peer learning in AI training addresses the issue of bias, a significant challenge in the field. By exposing AI models to a variety of perspectives and data interpretations, collaborative training helps mitigate the risk of developing biased algorithms. This is achieved by ensuring that no single model’s perspective dominates the learning process, thereby promoting a more balanced and equitable outcome. Consequently, AI systems trained through peer learning are better equipped to handle real-world applications where fairness and inclusivity are paramount.
In addition to reducing bias, collaborative AI training enhances the adaptability of AI systems. In traditional training methods, models are often limited by the scope of their initial programming and data exposure. However, through peer learning, AI models can continuously update and refine their knowledge base by assimilating new information from their peers. This dynamic learning process enables AI systems to adapt to changing environments and emerging challenges more effectively than those trained in isolation.
Furthermore, the collaborative approach fosters innovation by encouraging AI models to explore unconventional solutions. When AI systems are exposed to diverse problem-solving strategies from their peers, they are more likely to experiment with novel approaches. This not only leads to the discovery of more efficient algorithms but also drives the advancement of AI technology as a whole. By promoting a culture of experimentation and innovation, peer learning contributes to the development of AI systems that are not only more capable but also more creative.
Despite its numerous advantages, implementing collaborative AI training presents certain challenges. Ensuring effective communication between AI models requires sophisticated algorithms and protocols that can handle the complexity of information exchange. Additionally, maintaining a balance between collaboration and competition among AI models is crucial to prevent any single model from dominating the learning process. Addressing these challenges necessitates ongoing research and development to refine the methodologies and technologies underpinning peer learning.
In conclusion, collaborative AI training through peer learning represents a promising avenue for tackling present-day challenges in artificial intelligence. By harnessing the power of collective intelligence, this approach not only enhances the learning capabilities of AI systems but also promotes fairness, adaptability, and innovation. As the field of AI continues to evolve, embracing such innovative training methods will be essential to unlocking the full potential of artificial intelligence in addressing the complex issues of our time.
Gamification Techniques To Enhance AI Skill Acquisition
In recent years, the field of artificial intelligence has witnessed remarkable advancements, driven by the need to address complex challenges across various sectors. As AI systems become increasingly sophisticated, the demand for skilled professionals capable of developing and managing these technologies has surged. To meet this demand, innovative training methods have emerged, with gamification techniques playing a pivotal role in enhancing AI skill acquisition. By integrating game-like elements into educational frameworks, these techniques offer a dynamic and engaging approach to learning, thereby transforming the traditional paradigms of AI education.
Gamification, the application of game-design elements in non-game contexts, has proven to be an effective strategy in motivating learners and improving their engagement. In the context of AI training, gamification leverages elements such as points, badges, leaderboards, and challenges to create an interactive learning environment. This approach not only makes the learning process more enjoyable but also fosters a competitive spirit among participants, encouraging them to strive for excellence. As a result, learners are more likely to retain information and develop a deeper understanding of complex AI concepts.
Moreover, gamification techniques facilitate experiential learning, which is crucial for mastering AI skills. By simulating real-world scenarios, these techniques allow learners to apply theoretical knowledge in practical settings. For instance, AI training programs often incorporate simulations that mimic real-life challenges, enabling participants to experiment with different algorithms and strategies. This hands-on experience is invaluable, as it helps learners develop problem-solving skills and adapt to the rapidly evolving AI landscape. Furthermore, the immediate feedback provided in gamified environments allows learners to identify areas for improvement and refine their skills accordingly.
In addition to enhancing engagement and experiential learning, gamification techniques also promote collaboration and teamwork. Many AI training programs incorporate multiplayer elements, where participants work together to solve complex problems. This collaborative approach mirrors the real-world dynamics of AI development, where interdisciplinary teams must collaborate to achieve common goals. By fostering a sense of community and shared purpose, gamification encourages learners to exchange ideas, share knowledge, and learn from one another. This collaborative learning environment not only enhances individual skill acquisition but also prepares participants for the collaborative nature of AI work in professional settings.
Furthermore, gamification techniques can be tailored to accommodate diverse learning styles and preferences. By offering a variety of challenges and activities, these techniques cater to different levels of expertise and areas of interest. For instance, some learners may prefer coding challenges that test their programming skills, while others may be more inclined towards strategic games that require critical thinking and decision-making. This flexibility ensures that all participants can engage with the material in a way that resonates with them, thereby maximizing the effectiveness of the training program.
In conclusion, gamification techniques represent a promising approach to enhancing AI skill acquisition in the face of present-day challenges. By creating an engaging, experiential, and collaborative learning environment, these techniques address the limitations of traditional educational methods and prepare learners for the complexities of the AI field. As the demand for AI expertise continues to grow, the integration of gamification into training programs will likely play an increasingly important role in shaping the next generation of AI professionals. Through innovative approaches such as these, the potential of AI can be harnessed to address the pressing challenges of our time.
Adaptive AI Training Platforms For Dynamic Learning
In the rapidly evolving landscape of artificial intelligence, the need for adaptive AI training platforms has become increasingly apparent. As AI systems are integrated into various sectors, from healthcare to finance, the demand for more sophisticated and responsive training methods has surged. These innovative platforms are designed to address present-day challenges by offering dynamic learning environments that can adjust to the ever-changing requirements of AI applications.
One of the primary challenges in AI training is the sheer volume and complexity of data that must be processed. Traditional training methods often struggle to keep pace with the influx of new information, leading to outdated models that fail to perform optimally. Adaptive AI training platforms, however, are equipped with the capability to continuously learn and evolve. By leveraging machine learning algorithms that can self-adjust based on new data inputs, these platforms ensure that AI systems remain relevant and effective.
Moreover, the integration of real-time feedback mechanisms into these platforms allows for immediate adjustments in training protocols. This is particularly beneficial in environments where rapid decision-making is crucial, such as in autonomous vehicles or real-time financial trading systems. By incorporating feedback loops, adaptive platforms can fine-tune AI models on-the-fly, enhancing their accuracy and reliability.
Another significant advantage of adaptive AI training platforms is their ability to personalize learning experiences. Just as personalized education has transformed human learning, these platforms tailor training processes to the specific needs of each AI model. This customization is achieved through the use of advanced analytics and data-driven insights, which identify the unique strengths and weaknesses of a model. Consequently, training can be directed towards areas that require improvement, optimizing the overall performance of the AI system.
Furthermore, the scalability of adaptive AI training platforms is a critical factor in their effectiveness. As organizations grow and their data needs expand, these platforms can seamlessly scale to accommodate increased demands. This scalability is facilitated by cloud-based infrastructures, which provide the necessary computational power and storage capacity. As a result, businesses can deploy AI solutions without the constraints of limited resources, ensuring that their systems can handle larger datasets and more complex tasks.
In addition to scalability, the collaborative nature of adaptive AI training platforms fosters innovation and knowledge sharing. By enabling multiple stakeholders to contribute to the training process, these platforms create a collaborative ecosystem where ideas and solutions can be exchanged freely. This collaborative approach not only accelerates the development of AI models but also enhances their robustness by incorporating diverse perspectives and expertise.
Despite the numerous benefits, the implementation of adaptive AI training platforms is not without its challenges. Ensuring data privacy and security remains a top priority, as these platforms often handle sensitive information. To address this, robust encryption methods and strict access controls are essential components of any adaptive training system. Additionally, the ethical implications of AI training must be carefully considered, particularly in terms of bias and fairness. By incorporating ethical guidelines into the training process, developers can mitigate potential risks and ensure that AI systems operate in a just and equitable manner.
In conclusion, adaptive AI training platforms represent a significant advancement in the field of artificial intelligence. By offering dynamic, personalized, and scalable learning environments, these platforms effectively tackle present-day challenges and pave the way for more intelligent and responsive AI systems. As technology continues to advance, the role of adaptive training methods will undoubtedly become even more critical in shaping the future of AI.
Leveraging Virtual Reality For Immersive AI Education
In recent years, the integration of virtual reality (VR) into educational frameworks has revolutionized the way artificial intelligence (AI) is taught and understood. As AI continues to permeate various sectors, the demand for innovative training methods that can effectively convey complex concepts has grown exponentially. Virtual reality, with its immersive capabilities, offers a unique solution to this challenge by providing an interactive and engaging learning environment that traditional methods often lack.
To begin with, virtual reality facilitates a hands-on approach to AI education, allowing learners to engage with AI models and algorithms in a simulated environment. This experiential learning method enables students to visualize abstract concepts, such as neural networks and machine learning processes, in a tangible manner. By interacting with these models in a three-dimensional space, learners can gain a deeper understanding of how AI systems function and how they can be applied to solve real-world problems. Consequently, this immersive experience not only enhances comprehension but also fosters creativity and innovation among students.
Moreover, virtual reality offers the advantage of personalized learning experiences, which are crucial in addressing the diverse needs of learners. Through adaptive VR platforms, educators can tailor the content and pace of instruction to suit individual learning styles and proficiencies. This customization ensures that each student can progress at their own pace, thereby maximizing their potential and minimizing the risk of falling behind. Additionally, VR environments can simulate a wide range of scenarios and datasets, providing learners with the opportunity to experiment with AI applications in various contexts. This versatility is particularly beneficial in preparing students for the dynamic and ever-evolving nature of the AI industry.
Furthermore, the collaborative nature of virtual reality enhances the learning experience by promoting teamwork and communication skills. In a VR setting, students can work together on projects, share insights, and solve problems collectively, regardless of their physical location. This aspect of VR education is especially pertinent in today’s globalized world, where cross-cultural collaboration is often essential. By fostering a sense of community and shared purpose, virtual reality not only enriches the educational experience but also prepares students for the collaborative demands of the modern workforce.
In addition to these educational benefits, virtual reality also addresses some of the logistical challenges associated with traditional AI training methods. For instance, VR can significantly reduce the need for physical resources and infrastructure, such as computer labs and specialized equipment. This reduction in resource dependency makes AI education more accessible and cost-effective, particularly for institutions with limited budgets. Moreover, the scalability of VR platforms allows for the seamless integration of new content and updates, ensuring that learners have access to the latest advancements in AI technology.
In conclusion, the incorporation of virtual reality into AI education represents a significant advancement in addressing the challenges of teaching complex and rapidly evolving subjects. By providing an immersive, personalized, and collaborative learning environment, VR not only enhances comprehension and engagement but also prepares students for the demands of the AI industry. As technology continues to advance, it is imperative that educational institutions embrace these innovative methods to equip learners with the skills and knowledge necessary to thrive in an increasingly AI-driven world. Through the strategic use of virtual reality, educators can unlock new possibilities in AI training, ultimately contributing to the development of a more informed and capable workforce.
Q&A
1. **Question:** What is transfer learning, and how does it address current AI training challenges?
**Answer:** Transfer learning involves using a pre-trained model on a new, related task, reducing the need for large datasets and computational resources. It addresses challenges by improving efficiency and performance in AI training, especially when data is scarce.
2. **Question:** How does reinforcement learning contribute to innovative AI training methods?
**Answer:** Reinforcement learning trains AI models through trial and error, using feedback from their actions to learn optimal behaviors. It is particularly useful for dynamic environments and complex decision-making tasks, addressing challenges in adaptability and real-time learning.
3. **Question:** What role does federated learning play in modern AI training?
**Answer:** Federated learning allows AI models to be trained across multiple decentralized devices or servers while keeping data localized. This method enhances privacy and security, addressing challenges related to data sharing and compliance with regulations.
4. **Question:** How does unsupervised learning tackle the challenge of labeled data scarcity?
**Answer:** Unsupervised learning enables AI models to identify patterns and structures in unlabeled data, reducing the dependency on labeled datasets. This approach is crucial for tasks like clustering and anomaly detection, where labeled data is limited or unavailable.
5. **Question:** What is the significance of few-shot learning in AI training?
**Answer:** Few-shot learning allows models to learn from a very small number of examples, mimicking human-like learning capabilities. It addresses the challenge of training AI with limited data, making it valuable for applications where data collection is difficult or expensive.
6. **Question:** How do generative adversarial networks (GANs) innovate AI training methods?
**Answer:** GANs consist of two neural networks, a generator and a discriminator, that train together to produce realistic data samples. They tackle challenges in data augmentation and synthetic data generation, enhancing model robustness and diversity in training datasets.Innovative AI training methods are increasingly addressing present-day challenges by enhancing the adaptability, efficiency, and ethical considerations of AI systems. Techniques such as transfer learning, reinforcement learning, and federated learning are enabling AI models to learn from diverse datasets, improve decision-making processes, and maintain data privacy. Additionally, the integration of explainable AI and bias mitigation strategies ensures that AI systems are transparent and fair, fostering trust and accountability. These advancements are crucial in meeting the demands of rapidly evolving industries and societal needs, ultimately driving the responsible and effective deployment of AI technologies.
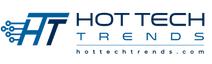