The Breakthrough Robot Syllabus represents a significant advancement in the field of robotics, enabling autonomous skill transfer among machines. This innovative framework allows robots to learn and share skills with one another, effectively creating a collaborative network of machines capable of adapting to new tasks and environments with minimal human intervention. By leveraging cutting-edge technologies in machine learning and artificial intelligence, the syllabus facilitates the seamless exchange of knowledge and capabilities, thereby enhancing the efficiency and versatility of robotic systems across various industries. This development not only accelerates the deployment of robots in complex scenarios but also paves the way for more intelligent and autonomous robotic ecosystems.
Understanding the Breakthrough Robot Syllabus: A New Era in Autonomous Skill Transfer
The advent of the Breakthrough Robot Syllabus marks a significant milestone in the field of robotics, heralding a new era in autonomous skill transfer among machines. This innovative framework is designed to enable robots to learn and share skills with one another, thereby enhancing their ability to perform complex tasks without human intervention. At its core, the Breakthrough Robot Syllabus leverages advanced machine learning algorithms and artificial intelligence to facilitate the seamless transfer of knowledge between robots, allowing them to adapt to new environments and challenges with unprecedented efficiency.
To understand the significance of this development, it is essential to consider the traditional limitations faced by robots in learning and executing tasks. Historically, robots have been programmed to perform specific functions, with little capacity for adaptation or learning beyond their initial programming. This rigidity has often necessitated extensive human involvement in reprogramming and retraining robots for new tasks, thereby limiting their utility in dynamic and unpredictable environments. However, the Breakthrough Robot Syllabus addresses these limitations by introducing a standardized framework through which robots can autonomously acquire and refine skills.
The implementation of this syllabus involves a multi-faceted approach that combines elements of deep learning, neural networks, and cloud-based data sharing. By utilizing deep learning techniques, robots can analyze vast amounts of data to identify patterns and develop strategies for task execution. Neural networks, on the other hand, enable robots to simulate human-like learning processes, allowing them to improve their performance over time through trial and error. Furthermore, the integration of cloud-based data sharing ensures that once a robot masters a particular skill, it can upload this knowledge to a centralized database, making it accessible to other robots within the network.
One of the most compelling aspects of the Breakthrough Robot Syllabus is its potential to revolutionize industries that rely heavily on automation. For instance, in manufacturing, robots equipped with this syllabus can quickly adapt to changes in production lines, reducing downtime and increasing efficiency. Similarly, in healthcare, robots can learn from each other to perform intricate surgical procedures with greater precision, thereby improving patient outcomes. Moreover, the ability to transfer skills autonomously can significantly enhance the capabilities of robots in disaster response scenarios, where adaptability and quick learning are crucial.
Despite its promising potential, the widespread adoption of the Breakthrough Robot Syllabus is not without challenges. Concerns regarding data security and privacy are paramount, as the sharing of information between robots necessitates robust safeguards to prevent unauthorized access and misuse. Additionally, the ethical implications of autonomous skill transfer must be carefully considered, particularly in contexts where human jobs may be displaced by increasingly capable machines. Nevertheless, ongoing research and development efforts are focused on addressing these issues, ensuring that the benefits of this technology can be realized while minimizing potential risks.
In conclusion, the Breakthrough Robot Syllabus represents a transformative advancement in the field of robotics, offering a framework for autonomous skill transfer that promises to enhance the versatility and efficiency of machines across various sectors. By enabling robots to learn from each other and adapt to new challenges, this innovative approach has the potential to redefine the role of automation in our society. As researchers and industry leaders continue to explore the possibilities of this technology, it is clear that the Breakthrough Robot Syllabus is poised to play a pivotal role in shaping the future of robotics.
How Autonomous Skill Transfer is Revolutionizing Machine Learning
The advent of autonomous skill transfer among machines marks a significant milestone in the field of machine learning, promising to revolutionize how robots learn and adapt to new tasks. This breakthrough is largely attributed to the development of a novel robot syllabus, which enables machines to share and acquire skills from one another with unprecedented efficiency. As the demand for intelligent automation continues to grow across various industries, the ability for machines to autonomously transfer skills is poised to enhance productivity, reduce costs, and accelerate innovation.
At the core of this innovation is the concept of a robot syllabus, a structured framework that allows machines to communicate and exchange knowledge seamlessly. Traditionally, robots have been programmed to perform specific tasks, requiring extensive human intervention to reprogram them for new activities. However, with the introduction of this syllabus, robots can now autonomously learn from each other, bypassing the need for manual reprogramming. This is achieved through advanced algorithms that facilitate the transfer of skills, enabling machines to understand and replicate tasks performed by their peers.
The implications of autonomous skill transfer are profound, particularly in manufacturing and logistics, where robots are increasingly deployed to perform complex operations. For instance, in a manufacturing plant, a robot equipped with welding skills can now teach another robot how to perform the same task, thereby streamlining the production process. This not only reduces the time and resources required for training but also ensures a consistent quality of work across the board. Moreover, as robots continue to learn from each other, they can adapt to changes in the production line, enhancing their flexibility and responsiveness to dynamic environments.
Furthermore, the ability for machines to autonomously transfer skills extends beyond industrial applications. In healthcare, for example, surgical robots can share techniques and procedures, improving the precision and effectiveness of medical interventions. Similarly, in the realm of autonomous vehicles, cars can exchange information about driving conditions and routes, enhancing safety and efficiency on the roads. This cross-domain applicability underscores the transformative potential of autonomous skill transfer, as it enables machines to operate with a level of intelligence and adaptability previously thought unattainable.
Despite these promising developments, the implementation of autonomous skill transfer is not without challenges. Ensuring the security and integrity of the knowledge being transferred is paramount, as any compromise could lead to significant operational disruptions. Additionally, ethical considerations must be addressed, particularly concerning the potential displacement of human workers as machines become more capable of performing complex tasks. Nevertheless, with ongoing research and development, these challenges are being actively addressed, paving the way for a future where machines and humans can coexist and collaborate harmoniously.
In conclusion, the breakthrough in autonomous skill transfer among machines represents a paradigm shift in machine learning, offering a glimpse into a future where robots can learn and adapt with minimal human intervention. By leveraging a structured robot syllabus, machines can now share and acquire skills with remarkable efficiency, unlocking new possibilities across various sectors. As this technology continues to evolve, it holds the promise of not only enhancing productivity and innovation but also redefining the relationship between humans and machines in the modern world.
The Impact of Robot Syllabus on Future Robotics and Automation
The advent of the breakthrough robot syllabus marks a significant milestone in the field of robotics and automation, promising to revolutionize how machines learn and interact with their environment. This innovative framework enables autonomous skill transfer among machines, a development that could redefine the capabilities of robots across various industries. As we delve into the implications of this technology, it is essential to understand how it functions and the potential it holds for the future.
At its core, the robot syllabus is a structured set of guidelines and protocols that allow robots to share and acquire skills from one another without human intervention. This system is akin to a curriculum for machines, where each robot can contribute its learned experiences to a collective knowledge base. Consequently, when a new robot is introduced into the system, it can access this repository of skills and knowledge, thereby accelerating its learning process. This capability is particularly beneficial in dynamic environments where adaptability and quick learning are crucial.
The impact of this technology on future robotics is profound. Traditionally, programming robots to perform specific tasks has been a time-consuming and labor-intensive process. Each machine had to be individually programmed and trained, often requiring significant human oversight. However, with the robot syllabus, this paradigm shifts dramatically. Robots can now learn from each other, reducing the need for extensive programming and allowing for more efficient deployment in various settings. This not only saves time and resources but also enhances the scalability of robotic solutions.
Moreover, the autonomous skill transfer facilitated by the robot syllabus fosters a new level of collaboration among machines. In manufacturing, for instance, robots equipped with this technology can seamlessly integrate into production lines, learning from their counterparts and optimizing their performance in real-time. This leads to increased productivity and reduced downtime, as machines can quickly adapt to changes in the production process. Similarly, in sectors such as healthcare, robots can share knowledge about patient care techniques, improving the quality and consistency of service delivery.
Furthermore, the implications of the robot syllabus extend beyond individual industries. As robots become more adept at learning and sharing skills, they can tackle more complex tasks that were previously deemed too challenging. This opens up new possibilities for automation in areas such as disaster response, where robots can be deployed to hazardous environments, learning from each other to navigate and perform rescue operations effectively. The potential for innovation is vast, as machines continue to evolve and expand their capabilities through shared learning.
However, the widespread adoption of the robot syllabus also raises important considerations. Ensuring the security and integrity of the shared knowledge base is paramount, as any compromise could have far-reaching consequences. Additionally, ethical considerations surrounding the autonomy of machines and their decision-making processes must be addressed to prevent unintended outcomes. As the technology progresses, it will be crucial for stakeholders to collaborate in establishing guidelines and standards that govern the use of autonomous skill transfer among robots.
In conclusion, the breakthrough robot syllabus represents a transformative advancement in robotics and automation. By enabling autonomous skill transfer among machines, it paves the way for more efficient, adaptable, and collaborative robotic systems. As industries continue to explore the potential of this technology, it is clear that the future of robotics will be shaped by the ability of machines to learn from one another, driving innovation and progress across diverse fields.
Key Benefits of Implementing Autonomous Skill Transfer in Robotics
The advent of a breakthrough robot syllabus that enables autonomous skill transfer among machines marks a significant milestone in the field of robotics. This innovative development offers numerous key benefits, fundamentally transforming how robots learn and interact with their environments. By facilitating the seamless transfer of skills from one machine to another, this technology not only enhances efficiency but also broadens the scope of robotic applications across various industries.
One of the primary benefits of implementing autonomous skill transfer in robotics is the substantial reduction in training time. Traditionally, programming robots to perform specific tasks has been a time-consuming and labor-intensive process, often requiring extensive human intervention. However, with the ability to autonomously transfer skills, robots can quickly acquire new capabilities by learning from their peers. This process significantly accelerates the deployment of robotic systems, allowing industries to adapt swiftly to changing demands and operational requirements.
Moreover, autonomous skill transfer enhances the adaptability and versatility of robotic systems. In dynamic environments where tasks and conditions frequently change, the ability of robots to learn from each other ensures that they can adjust to new challenges without the need for constant reprogramming. This adaptability is particularly beneficial in sectors such as manufacturing, logistics, and healthcare, where the ability to respond to unforeseen circumstances is crucial for maintaining operational continuity and efficiency.
In addition to improving adaptability, autonomous skill transfer also promotes innovation by enabling the sharing of specialized knowledge across different robotic platforms. For instance, a robot designed for precision assembly in the automotive industry can transfer its expertise to a robot operating in the electronics sector, thereby expanding the range of tasks that each machine can perform. This cross-domain skill transfer not only enhances the capabilities of individual robots but also fosters collaboration and innovation across industries, driving technological advancement and economic growth.
Furthermore, the implementation of autonomous skill transfer contributes to cost savings by reducing the need for extensive human oversight and intervention. As robots become more self-sufficient in acquiring and refining skills, organizations can allocate resources more efficiently, focusing on strategic initiatives rather than routine programming and maintenance tasks. This shift not only lowers operational costs but also allows human workers to engage in more complex and value-added activities, ultimately enhancing productivity and job satisfaction.
Another significant advantage of autonomous skill transfer is its potential to improve safety in environments where human-robot interaction is prevalent. By enabling robots to learn from each other, this technology minimizes the risk of errors and accidents, as machines can quickly adapt to best practices and safety protocols established by their peers. This capability is particularly important in sectors such as construction and healthcare, where the safety of both human workers and end-users is paramount.
In conclusion, the breakthrough robot syllabus that facilitates autonomous skill transfer among machines offers a multitude of benefits that are poised to revolutionize the robotics industry. By reducing training time, enhancing adaptability, promoting innovation, and contributing to cost savings and safety improvements, this technology represents a significant leap forward in the development of intelligent and autonomous robotic systems. As industries continue to embrace this transformative capability, the potential for increased efficiency, productivity, and innovation becomes increasingly apparent, paving the way for a future where robots play an even more integral role in our daily lives and work environments.
Challenges and Solutions in Developing a Robot Syllabus for Skill Transfer
The development of a robot syllabus for skill transfer among machines represents a significant milestone in the field of robotics and artificial intelligence. However, this breakthrough is not without its challenges. One of the primary obstacles in creating such a syllabus is the inherent complexity of encoding human-like learning processes into machines. Unlike humans, who can intuitively grasp and adapt to new skills through observation and practice, robots require meticulously programmed instructions to perform even the simplest tasks. This necessitates a comprehensive understanding of both the task at hand and the underlying principles that govern it, which can be a daunting endeavor.
Moreover, the diversity of tasks that robots are expected to perform adds another layer of complexity. From industrial applications to healthcare and domestic assistance, each domain presents unique challenges that must be addressed within the syllabus. For instance, a robot designed for manufacturing might need to learn precision and efficiency, while one intended for caregiving must prioritize empathy and adaptability. Consequently, the syllabus must be versatile enough to accommodate a wide range of skills while ensuring that the learning process remains efficient and effective.
To address these challenges, researchers have turned to advanced machine learning techniques, such as reinforcement learning and neural networks, which allow robots to learn from experience and improve over time. By simulating various scenarios and providing feedback, these techniques enable robots to refine their skills autonomously. However, the implementation of such methods is not without its hurdles. The computational power required to process vast amounts of data and the time needed for training can be prohibitive, necessitating the development of more efficient algorithms and hardware.
In addition to technical challenges, there are also ethical considerations to be taken into account. As robots become more autonomous, questions arise regarding accountability and decision-making. Ensuring that robots adhere to ethical guidelines and make decisions that align with human values is crucial. This requires the integration of ethical frameworks into the robot syllabus, which can be a complex task given the subjective nature of ethics.
Despite these challenges, there are promising solutions on the horizon. Collaborative efforts between academia, industry, and policymakers are paving the way for the development of standardized protocols and guidelines for robot skill transfer. By fostering an environment of open collaboration and knowledge sharing, these initiatives aim to accelerate the development of a robust robot syllabus that can be widely adopted.
Furthermore, the advent of cloud-based robotics offers a potential solution to the computational challenges associated with skill transfer. By leveraging cloud computing, robots can access vast resources and share knowledge in real-time, significantly reducing the time and cost associated with training. This not only enhances the efficiency of the learning process but also facilitates the rapid dissemination of new skills across different machines.
In conclusion, while the development of a robot syllabus for skill transfer presents numerous challenges, the potential benefits are immense. By enabling machines to learn and adapt autonomously, we can unlock new possibilities in automation and artificial intelligence, ultimately leading to more efficient and versatile robotic systems. Through continued research and collaboration, the vision of a standardized robot syllabus is becoming increasingly attainable, promising a future where machines can seamlessly acquire and transfer skills, much like humans do.
Case Studies: Successful Implementations of Autonomous Skill Transfer in Robotics
In recent years, the field of robotics has witnessed remarkable advancements, particularly in the realm of autonomous skill transfer among machines. This innovative concept, which allows robots to learn and adapt skills from one another without human intervention, has been successfully implemented in various case studies, showcasing its potential to revolutionize industries. One such breakthrough is the development of a comprehensive robot syllabus, designed to facilitate seamless skill transfer across different robotic platforms. This syllabus serves as a standardized framework, enabling machines to communicate and share knowledge efficiently.
The implementation of this robot syllabus has been particularly successful in manufacturing environments, where robots are required to perform complex tasks with precision and consistency. For instance, in an automotive assembly line, robots equipped with the syllabus can quickly learn new assembly techniques from their counterparts, significantly reducing downtime and increasing productivity. This capability is especially beneficial in scenarios where production lines need to be reconfigured to accommodate new models or design changes. By leveraging the autonomous skill transfer, manufacturers can ensure that their robotic workforce remains agile and responsive to evolving demands.
Moreover, the healthcare sector has also benefited from the adoption of autonomous skill transfer among medical robots. In surgical settings, robots can now acquire new procedural skills from other machines, enhancing their ability to assist surgeons in performing intricate operations. This not only improves the accuracy and efficiency of surgical procedures but also reduces the learning curve for new robotic systems introduced into the operating room. Consequently, hospitals can provide better patient care while optimizing their investment in robotic technology.
Transitioning to the logistics industry, the implementation of the robot syllabus has enabled warehouse robots to adapt to various tasks, such as sorting, packing, and transporting goods. By sharing skills autonomously, these robots can quickly adjust to changes in inventory or order fulfillment processes, ensuring that operations run smoothly even during peak demand periods. This adaptability is crucial for e-commerce companies that rely on efficient logistics to meet customer expectations and maintain a competitive edge.
Furthermore, the agricultural sector has seen promising results from the integration of autonomous skill transfer in robotic systems. Agricultural robots, tasked with planting, harvesting, and monitoring crops, can now learn from each other to optimize their performance. For example, a robot that has mastered a particular harvesting technique can share this knowledge with others, leading to improved yield and reduced waste. This collaborative approach not only enhances productivity but also supports sustainable farming practices by minimizing resource consumption.
In addition to these industry-specific applications, the robot syllabus has broader implications for the future of robotics. By enabling machines to learn from one another, researchers can accelerate the development of more sophisticated and versatile robotic systems. This, in turn, paves the way for new applications in fields such as disaster response, where robots can be deployed to assess and mitigate hazards in real-time.
In conclusion, the successful implementation of autonomous skill transfer among machines, facilitated by a standardized robot syllabus, represents a significant milestone in the evolution of robotics. As industries continue to embrace this technology, the potential for increased efficiency, adaptability, and innovation becomes increasingly apparent. By fostering collaboration among machines, we are not only enhancing their capabilities but also unlocking new possibilities for the future of automation.
Q&A
1. **What is the Breakthrough Robot Syllabus?**
The Breakthrough Robot Syllabus is a structured framework designed to enable robots to autonomously learn and transfer skills among different machines, enhancing their adaptability and efficiency in various tasks.
2. **How does the syllabus enable skill transfer?**
The syllabus utilizes advanced algorithms and machine learning techniques to allow robots to share learned skills and knowledge, facilitating seamless skill transfer and reducing the need for individual programming.
3. **What are the key components of the syllabus?**
Key components include a standardized set of protocols for communication, a library of transferable skills, and a learning module that allows robots to adapt skills to new environments or tasks.
4. **What industries could benefit from this technology?**
Industries such as manufacturing, logistics, healthcare, and service sectors could benefit significantly by improving automation, reducing costs, and increasing productivity through autonomous skill transfer.
5. **What challenges does the syllabus address?**
The syllabus addresses challenges such as the high cost and time associated with programming individual robots, the need for flexibility in dynamic environments, and the integration of heterogeneous robotic systems.
6. **What is the future potential of this technology?**
The future potential includes creating more intelligent and versatile robotic systems capable of collaborating with humans, adapting to new tasks with minimal intervention, and accelerating the development of fully autonomous robotic networks.The breakthrough in robot syllabus enabling autonomous skill transfer among machines represents a significant advancement in robotics and artificial intelligence. By allowing robots to share and transfer skills autonomously, this development enhances the efficiency and adaptability of robotic systems across various industries. It reduces the need for extensive programming and manual intervention, facilitating quicker deployment and integration of robots into new tasks and environments. This innovation not only accelerates the learning curve for machines but also paves the way for more collaborative and intelligent robotic ecosystems, ultimately driving progress in automation and machine learning.
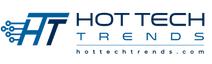