The rapid proliferation of artificial intelligence (AI) startups in recent years has sparked both excitement and skepticism within the tech industry. While the potential for groundbreaking innovations and transformative solutions is immense, the harsh reality is that most AI startups are destined to fail. This high failure rate can be attributed to several critical factors, including the intense competition in the AI landscape, the substantial financial and technical resources required to develop and scale AI technologies, and the challenges of navigating complex regulatory environments. Additionally, many AI startups struggle with finding a viable business model, differentiating their offerings in a crowded market, and addressing ethical concerns related to AI deployment. As a result, despite the initial promise and enthusiasm, only a small fraction of AI startups manage to achieve long-term success and sustainability.
Lack Of Clear Market Need
In the rapidly evolving landscape of artificial intelligence, startups are emerging at an unprecedented rate, each promising to revolutionize industries and transform the way we live and work. However, despite the enthusiasm and innovation driving these ventures, a significant number of AI startups are destined to fail. One of the primary reasons for this high failure rate is the lack of a clear market need for their products or services. Understanding this challenge is crucial for entrepreneurs and investors alike as they navigate the complex world of AI innovation.
To begin with, the allure of AI technology often leads startups to focus more on the capabilities of their solutions rather than the actual problems they aim to solve. This technology-first approach can result in products that, while technically impressive, do not address any specific pain points faced by potential customers. Consequently, these startups struggle to find a market fit, as their offerings do not resonate with the needs or priorities of their target audience. Without a clear understanding of the market demand, even the most advanced AI solutions can become irrelevant.
Moreover, the AI industry is characterized by rapid technological advancements and intense competition. Startups that fail to identify a distinct market need may find themselves overshadowed by competitors who have a clearer vision of their value proposition. In such a competitive environment, it is not enough to simply develop a product that leverages AI; startups must also demonstrate how their solution provides tangible benefits to users. This requires a deep understanding of the industry landscape and the ability to anticipate and respond to evolving customer needs.
Furthermore, the lack of a clear market need can lead to difficulties in securing funding and resources. Investors are increasingly cautious about backing AI startups that do not have a well-defined market strategy. They seek assurance that the startup has a viable path to profitability, which is often contingent on addressing a genuine market demand. Without this assurance, startups may struggle to attract the necessary capital to sustain their operations and scale their business.
In addition to these challenges, AI startups must also contend with the complexities of integrating their solutions into existing systems and workflows. Even if a startup identifies a potential market need, the practicalities of implementation can pose significant hurdles. Organizations may be hesitant to adopt new AI technologies due to concerns about compatibility, cost, and the potential disruption to their operations. Startups that do not adequately address these concerns may find it difficult to gain traction in the market.
To mitigate the risk of failure, AI startups must prioritize understanding and validating the market need for their solutions. This involves engaging with potential customers early in the development process to gather feedback and insights. By doing so, startups can refine their offerings to better align with customer expectations and demonstrate a clear value proposition. Additionally, maintaining flexibility and adaptability is crucial, as market needs can shift rapidly in response to technological advancements and changing industry dynamics.
In conclusion, while the potential of AI technology is vast, the success of AI startups hinges on their ability to identify and address a clear market need. By focusing on solving real-world problems and demonstrating tangible value to customers, startups can increase their chances of success in this competitive and rapidly evolving field. As the AI landscape continues to mature, those ventures that prioritize market alignment will be better positioned to thrive and make a lasting impact.
Insufficient Funding And Resources
In the rapidly evolving landscape of artificial intelligence, startups are emerging at an unprecedented rate, each promising to revolutionize industries and redefine the future. However, despite the initial enthusiasm and groundbreaking ideas, a significant number of these AI startups are destined to fail. One of the primary reasons for this high failure rate is insufficient funding and resources, which are crucial for sustaining innovation and growth in this highly competitive field.
To begin with, the development of AI technologies requires substantial financial investment. From hiring skilled data scientists and engineers to acquiring the necessary computational infrastructure, the costs can be prohibitive. Many startups, driven by visionary founders, often underestimate these financial demands. They may secure initial seed funding, which provides a temporary boost, but as the complexity of their projects grows, so does the need for additional capital. Without adequate funding, these startups struggle to maintain momentum, leading to stalled projects and, ultimately, failure.
Moreover, the competition for funding in the AI sector is fierce. Venture capitalists and investors are inundated with proposals, making it challenging for startups to stand out. Those that do manage to secure funding often find it insufficient to cover the long-term development and scaling of their technologies. This is particularly true for startups that focus on niche markets or highly specialized applications, where the potential for immediate returns may not be as apparent to investors. Consequently, these startups face a precarious financial situation, unable to fully realize their innovative potential.
In addition to financial constraints, AI startups frequently encounter a shortage of resources, particularly in terms of talent and expertise. The demand for skilled professionals in AI far exceeds the supply, creating a competitive job market where startups must vie with established tech giants for top talent. This talent gap can severely hinder a startup’s ability to innovate and execute its vision effectively. Without access to experienced data scientists, machine learning engineers, and domain experts, startups may struggle to develop robust AI models and solutions, further diminishing their chances of success.
Furthermore, the lack of resources extends beyond human capital to include data, which is the lifeblood of AI development. Startups often face challenges in acquiring high-quality, diverse datasets necessary for training and refining their AI models. Unlike established companies that have access to vast amounts of proprietary data, startups must navigate legal, ethical, and logistical hurdles to obtain the data they need. This data scarcity can lead to suboptimal model performance and limit the startup’s ability to deliver on its promises.
In light of these challenges, it becomes evident that insufficient funding and resources are significant barriers to the success of AI startups. While innovative ideas and cutting-edge technologies are essential, they are not enough to guarantee success in the absence of adequate financial backing and access to critical resources. To mitigate these risks, startups must adopt strategic approaches to funding, such as building strong investor relationships and exploring alternative financing options. Additionally, fostering partnerships with academic institutions, industry leaders, and other startups can provide access to the talent and data necessary for growth.
In conclusion, while the allure of AI innovation continues to attract entrepreneurs and investors alike, the harsh reality is that most AI startups are destined to fail due to insufficient funding and resources. Addressing these challenges requires a multifaceted approach that combines financial acumen, strategic partnerships, and a keen understanding of the competitive landscape. Only then can AI startups hope to overcome the odds and achieve lasting success in this dynamic and demanding field.
Overreliance On Hype And Buzzwords
In the rapidly evolving landscape of technology, artificial intelligence (AI) has emerged as a beacon of innovation and potential. However, despite the promise it holds, a significant number of AI startups are finding themselves on a precarious path to failure. One of the primary reasons for this unfortunate trend is the overreliance on hype and buzzwords, which often overshadows the substantive development and application of AI technologies.
To begin with, the allure of AI is undeniable. It captivates investors, entrepreneurs, and the public alike with visions of transformative capabilities and unprecedented efficiencies. This allure, however, can lead to an environment where the excitement surrounding AI becomes more important than the actual technology itself. Startups, eager to capitalize on this enthusiasm, often resort to using buzzwords such as “machine learning,” “deep learning,” and “neural networks” without a clear understanding or plan for their practical implementation. Consequently, these terms become more of a marketing tool than a reflection of the startup’s core competencies or technological advancements.
Moreover, the pressure to secure funding and gain a competitive edge in the crowded AI market can drive startups to exaggerate their capabilities. In their pitches to investors, many startups emphasize their use of cutting-edge AI technologies, even when their actual products or services are only tangentially related to AI. This misrepresentation not only misleads investors but also sets unrealistic expectations for the startup’s performance and growth. As a result, when the time comes to deliver on these promises, many startups find themselves unable to meet the high expectations they have set, leading to disappointment and, ultimately, failure.
In addition to misleading investors, the overreliance on hype can also have detrimental effects on the internal dynamics of a startup. When a company’s focus is primarily on maintaining its image as an AI innovator, it may neglect the necessary investment in research and development. This can lead to a lack of genuine innovation and a failure to create products that truly leverage AI’s potential. Furthermore, the emphasis on buzzwords can create a disconnect between the technical team and the business side of the startup, as engineers and developers may feel pressured to conform to marketing narratives rather than pursue meaningful technological advancements.
Furthermore, the saturation of AI-related buzzwords in the market can lead to a dilution of their meaning and impact. As more startups adopt these terms without substantiating their claims, the credibility of AI as a transformative technology is undermined. This can result in skepticism from both investors and consumers, making it increasingly difficult for genuinely innovative AI startups to distinguish themselves and gain the trust they need to succeed.
In conclusion, while the potential of AI is vast and its applications are numerous, the overreliance on hype and buzzwords poses a significant threat to the success of AI startups. To avoid the pitfalls associated with this trend, startups must prioritize substance over style, focusing on developing robust technologies and delivering tangible results. By doing so, they can build a foundation of trust and credibility that will not only attract investment but also ensure long-term sustainability and success in the competitive AI landscape.
Inadequate Talent And Expertise
In the rapidly evolving landscape of artificial intelligence, startups are emerging at an unprecedented rate, each vying to carve out a niche in this transformative field. However, despite the enthusiasm and innovation driving these ventures, a significant number of AI startups are destined to fail. One of the primary reasons for this high failure rate is the inadequate talent and expertise that plagues many of these fledgling companies. Understanding the complexities of AI requires a deep well of knowledge and experience, which is often lacking in new startups.
To begin with, the field of artificial intelligence is inherently complex and multifaceted, demanding a high level of technical expertise. AI encompasses a wide range of subfields, including machine learning, natural language processing, computer vision, and robotics, each requiring specialized knowledge. Startups often struggle to find individuals who possess the necessary skills across these diverse areas. The shortage of qualified professionals is exacerbated by the fact that larger, more established tech companies frequently attract top talent with lucrative offers and the promise of working on cutting-edge projects. Consequently, startups are left competing for a limited pool of experts, which can hinder their ability to innovate and develop robust AI solutions.
Moreover, the rapid pace of advancements in AI technology means that staying current is a constant challenge. Startups must not only recruit skilled individuals but also ensure that their teams are continuously learning and adapting to new developments. This requires a commitment to ongoing education and training, which can be resource-intensive for startups operating on tight budgets. Without the ability to keep pace with technological advancements, startups risk falling behind their competitors, ultimately leading to their downfall.
In addition to technical expertise, successful AI startups require a deep understanding of the industries they aim to disrupt. Many startups fail because they lack domain-specific knowledge, which is crucial for developing AI solutions that address real-world problems effectively. For instance, an AI startup focused on healthcare must have a team that understands the intricacies of medical data, regulatory requirements, and patient care. Without this expertise, the startup may develop solutions that are technically sound but fail to meet the practical needs of the industry.
Furthermore, the interdisciplinary nature of AI necessitates collaboration between experts from various fields, including data science, software engineering, and domain-specific areas. Startups often struggle to foster such collaboration due to a lack of diverse talent and expertise within their teams. This can lead to siloed thinking and a failure to integrate different perspectives, ultimately resulting in suboptimal AI solutions.
In light of these challenges, it is evident that inadequate talent and expertise are significant barriers to the success of AI startups. To overcome these obstacles, startups must prioritize building teams with a diverse range of skills and experiences. This may involve seeking partnerships with academic institutions, investing in employee training programs, or leveraging remote work to access a global talent pool. By addressing the talent gap, AI startups can enhance their ability to innovate and develop solutions that have a meaningful impact.
In conclusion, while the allure of AI continues to attract entrepreneurs and investors alike, the path to success is fraught with challenges. Inadequate talent and expertise remain critical factors contributing to the high failure rate of AI startups. By recognizing and addressing these issues, startups can improve their chances of success and contribute to the ongoing evolution of artificial intelligence.
Poor Data Quality And Management
In the rapidly evolving landscape of artificial intelligence, startups are emerging at an unprecedented rate, each promising to revolutionize industries with innovative solutions. However, despite the initial enthusiasm and potential, a significant number of these AI startups are destined to fail. One of the primary reasons for this high failure rate is poor data quality and management, which are critical components in the development and deployment of AI technologies.
To begin with, data is the lifeblood of AI systems. These systems rely heavily on large volumes of high-quality data to learn, adapt, and make accurate predictions. Unfortunately, many startups underestimate the complexity and importance of data management. They often assume that acquiring data is a straightforward task, overlooking the challenges associated with data collection, cleaning, and integration. Consequently, they end up with datasets that are incomplete, inconsistent, or biased, which can severely undermine the performance of their AI models.
Moreover, the issue of data quality is compounded by the fact that many startups lack the necessary expertise in data management. While they may have talented AI researchers and developers, they often do not have professionals who specialize in data governance and quality assurance. This gap in expertise can lead to poor data handling practices, such as inadequate data validation and insufficient data preprocessing. As a result, the AI models developed by these startups may produce unreliable or inaccurate results, ultimately eroding customer trust and confidence.
In addition to the challenges of data quality, startups also face significant hurdles in data management. Effective data management involves not only storing and organizing data but also ensuring its accessibility and security. Many startups, in their rush to develop AI solutions, neglect to implement robust data management frameworks. This oversight can lead to data silos, where information is isolated and difficult to access, hindering collaboration and innovation. Furthermore, inadequate data security measures can expose startups to data breaches and compliance issues, which can be detrimental to their reputation and financial stability.
Transitioning from data management to the broader implications, it is important to recognize that poor data quality and management can have far-reaching consequences for AI startups. For instance, without reliable data, startups may struggle to demonstrate the efficacy of their AI solutions to potential investors and clients. This lack of demonstrable success can impede their ability to secure funding and establish a foothold in the market. Additionally, startups that fail to address data quality issues may find it challenging to scale their operations, as their AI models may not perform consistently across different contexts and applications.
Furthermore, the competitive nature of the AI industry means that startups cannot afford to overlook the importance of data quality and management. Established companies with more resources and experience are likely to have more sophisticated data strategies, giving them a significant advantage. Startups that do not prioritize data quality and management risk falling behind, as they may be unable to compete with the superior performance and reliability of their competitors’ AI solutions.
In conclusion, while AI startups hold great promise for innovation and disruption, their success is heavily contingent on their ability to manage and utilize data effectively. Poor data quality and management are critical factors that contribute to the high failure rate of these startups. By recognizing and addressing these challenges, AI startups can improve their chances of success and make meaningful contributions to the advancement of artificial intelligence.
Intense Competition And Market Saturation
In the rapidly evolving landscape of artificial intelligence, the allure of launching a startup in this domain is undeniable. Entrepreneurs are drawn to the potential of AI to revolutionize industries, solve complex problems, and generate substantial financial returns. However, despite the promising opportunities, most AI startups are destined to fail due to intense competition and market saturation. Understanding the dynamics of this competitive environment is crucial for aspiring entrepreneurs and investors alike.
To begin with, the AI sector is characterized by a high barrier to entry, primarily due to the significant resources required for research and development. Established tech giants such as Google, Amazon, and Microsoft have already invested heavily in AI, creating sophisticated algorithms and amassing vast datasets. These companies possess the financial muscle and technical expertise to maintain a competitive edge, making it challenging for new entrants to differentiate themselves. Consequently, startups often struggle to compete with these industry behemoths, which can offer superior products and services at a lower cost.
Moreover, the rapid pace of technological advancement in AI means that startups must continuously innovate to stay relevant. This constant need for innovation places immense pressure on startups, which often operate with limited resources and smaller teams. While innovation is the lifeblood of any tech startup, the relentless demand for cutting-edge solutions can lead to burnout and resource depletion. As a result, many startups find themselves unable to sustain the momentum required to keep up with the competition.
In addition to the challenges posed by established players, the AI market is becoming increasingly saturated. The proliferation of AI startups has led to a crowded marketplace where differentiation is difficult to achieve. With numerous companies offering similar solutions, customers have a wide array of choices, making it challenging for any single startup to capture significant market share. This saturation not only intensifies competition but also drives down prices, further squeezing the margins of startups that are already operating on tight budgets.
Furthermore, the complexity of AI technology itself presents a formidable challenge. Developing AI solutions requires a deep understanding of machine learning algorithms, data science, and domain-specific knowledge. Startups must navigate these complexities while also addressing ethical considerations and regulatory requirements, which are becoming more stringent as AI technologies become more pervasive. The need to balance technical innovation with ethical responsibility adds another layer of difficulty for startups striving to establish themselves in the market.
Despite these challenges, some AI startups do manage to succeed, often by identifying niche markets or developing unique solutions that address specific customer needs. However, even these successful ventures face the risk of being acquired by larger companies, which can stifle their growth and innovation potential. While acquisition can provide a lucrative exit strategy for founders, it also underscores the difficulty of achieving long-term independence and success in the AI sector.
In conclusion, the intense competition and market saturation in the AI industry create a challenging environment for startups. The dominance of established tech giants, the relentless demand for innovation, and the complexities of AI technology all contribute to the high failure rate of new ventures. For those considering entering this space, a clear understanding of these challenges and a strategic approach to differentiation and innovation are essential. Only by navigating these hurdles can AI startups hope to carve out a sustainable niche in this competitive landscape.
Q&A
1. **Question:** What is a common reason for the failure of AI startups related to data?
**Answer:** Many AI startups fail due to inadequate access to high-quality, diverse, and large datasets necessary for training effective AI models.
2. **Question:** How does the talent pool affect the success of AI startups?
**Answer:** AI startups often struggle to attract and retain skilled talent, as there is a high demand for experienced AI professionals, leading to a competitive and costly hiring environment.
3. **Question:** Why is scalability a challenge for AI startups?
**Answer:** AI startups frequently face difficulties in scaling their solutions from prototypes to production-ready systems, often due to technical complexities and infrastructure limitations.
4. **Question:** What role does market fit play in the failure of AI startups?
**Answer:** Many AI startups fail because they develop technology without a clear understanding of market needs, resulting in products that do not solve real-world problems or lack a viable customer base.
5. **Question:** How does competition impact the survival of AI startups?
**Answer:** The AI industry is highly competitive, with numerous startups and established tech giants vying for market share, making it challenging for new entrants to differentiate themselves and gain traction.
6. **Question:** In what way does regulatory compliance pose a risk to AI startups?
**Answer:** Navigating complex and evolving regulatory landscapes can be daunting for AI startups, leading to potential legal challenges and increased operational costs if compliance is not adequately addressed.Most AI startups are destined to fail due to several critical factors. Firstly, the AI industry is highly competitive, with large tech companies dominating the market, making it difficult for startups to gain a foothold. Secondly, AI development requires significant resources, including access to vast amounts of data and computational power, which many startups lack. Thirdly, there is often a gap between AI research and practical, scalable applications, leading to challenges in product-market fit. Additionally, regulatory and ethical concerns can pose significant hurdles, as startups must navigate complex legal landscapes and address public concerns about privacy and bias. Finally, the rapid pace of technological advancement means that startups must continuously innovate to stay relevant, which can be unsustainable. These challenges collectively contribute to the high failure rate of AI startups.
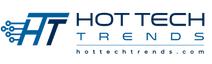