Data enrichment is a critical process in the realm of data management and analytics, aimed at enhancing the quality and value of raw data by supplementing it with additional information. Effective strategies for data enrichment are essential for organizations seeking to leverage their data assets for improved decision-making, customer insights, and operational efficiency. These strategies involve a combination of techniques and tools designed to refine, augment, and contextualize data, transforming it into a more comprehensive and actionable resource. By integrating external data sources, employing advanced analytics, and utilizing machine learning algorithms, businesses can achieve a more nuanced understanding of their data landscape. This enriched data not only supports more accurate predictions and personalized customer experiences but also drives innovation and competitive advantage in an increasingly data-driven world.
Understanding Data Enrichment: Key Concepts and Benefits
Data enrichment is a critical process in the realm of data management, aimed at enhancing the quality and utility of raw data by supplementing it with additional information. This process transforms basic data into a more valuable asset, enabling organizations to gain deeper insights and make more informed decisions. Understanding the key concepts and benefits of data enrichment is essential for businesses seeking to leverage their data effectively.
At its core, data enrichment involves integrating external data sources with existing internal data to create a more comprehensive dataset. This can include appending demographic information, geographic data, or behavioral insights to customer records. By doing so, organizations can develop a more nuanced understanding of their customers, leading to improved targeting and personalization in marketing efforts. For instance, a retail company might enrich its customer database with demographic data to tailor its marketing campaigns more precisely, thereby increasing engagement and conversion rates.
Moreover, data enrichment is not limited to customer data. It can also be applied to product information, financial data, and operational metrics, among others. By enriching product data with attributes such as specifications, reviews, and competitive pricing, businesses can enhance their product catalogs, making them more appealing to potential buyers. Similarly, enriching financial data with market trends and economic indicators can provide a more robust foundation for strategic planning and risk management.
One of the primary benefits of data enrichment is the improvement in data accuracy and completeness. Raw data often contains gaps or inaccuracies that can lead to flawed analyses and misguided decisions. Enrichment processes help fill these gaps by cross-referencing multiple data sources, thereby increasing the reliability of the data. This, in turn, enhances the quality of insights derived from data analytics, leading to more effective decision-making.
Furthermore, data enrichment facilitates better customer segmentation and profiling. By incorporating additional data points, businesses can segment their customer base more precisely, identifying distinct groups with specific needs and preferences. This enables more targeted marketing strategies and personalized customer experiences, which are crucial for building customer loyalty and driving sales growth.
In addition to these benefits, data enrichment also supports enhanced predictive analytics. By enriching datasets with historical and real-time data, organizations can develop more accurate predictive models. These models can forecast customer behavior, market trends, and operational outcomes with greater precision, allowing businesses to anticipate changes and adapt proactively.
However, it is important to recognize that data enrichment is not without its challenges. Ensuring data privacy and compliance with regulations such as GDPR is paramount when handling enriched data. Organizations must implement robust data governance frameworks to protect sensitive information and maintain customer trust. Additionally, the integration of disparate data sources can be complex, requiring sophisticated tools and expertise to ensure seamless data merging and consistency.
In conclusion, data enrichment is a powerful strategy for enhancing the value of data within an organization. By understanding its key concepts and benefits, businesses can unlock deeper insights, improve decision-making, and gain a competitive edge in the market. As data continues to grow in volume and complexity, the importance of effective data enrichment strategies will only increase, making it a vital component of modern data management practices.
Leveraging Third-Party Data Sources for Enhanced Insights
In the contemporary landscape of data-driven decision-making, organizations are increasingly recognizing the value of data enrichment as a means to gain deeper insights and enhance their strategic initiatives. Data enrichment involves augmenting existing datasets with additional information from external sources, thereby providing a more comprehensive view of the subject matter. One of the most effective strategies for data enrichment is leveraging third-party data sources, which can significantly enhance the quality and depth of insights derived from data analysis.
To begin with, third-party data sources offer a wealth of information that can complement and enhance internal datasets. These sources can include demographic data, social media activity, purchasing behavior, and more. By integrating such diverse data points, organizations can develop a more nuanced understanding of their customers, markets, and operational environments. For instance, a retail company might enrich its customer database with demographic information from a third-party provider, enabling it to tailor marketing campaigns more precisely to different segments of its audience.
Moreover, the use of third-party data sources can help organizations overcome the limitations of their internal data. Often, internal data may be incomplete or lack the granularity needed for detailed analysis. By incorporating external data, organizations can fill these gaps and achieve a more holistic view. This is particularly beneficial in industries where customer behavior is influenced by a wide array of external factors. For example, in the financial sector, integrating economic indicators and market trends from third-party sources can provide a more comprehensive risk assessment model.
In addition to enhancing data quality, third-party data sources can also facilitate predictive analytics. By enriching datasets with historical and real-time data from external sources, organizations can improve the accuracy of their predictive models. This is crucial for making informed decisions in areas such as inventory management, demand forecasting, and customer retention strategies. For instance, a logistics company might use weather data from a third-party provider to predict potential disruptions in supply chain operations and adjust its logistics plans accordingly.
However, while the benefits of leveraging third-party data sources are substantial, organizations must also navigate certain challenges. Data privacy and compliance are paramount concerns, as the integration of external data must adhere to relevant regulations such as GDPR or CCPA. Organizations must ensure that third-party data providers comply with these standards and that data is handled securely throughout the enrichment process. Additionally, the quality and reliability of third-party data must be rigorously assessed to avoid introducing inaccuracies into the enriched dataset.
Furthermore, integrating third-party data requires robust data management and integration capabilities. Organizations must invest in the necessary infrastructure and tools to seamlessly merge external data with their internal systems. This includes data cleansing, transformation, and validation processes to ensure that the enriched data is accurate and actionable. Advanced data integration platforms and APIs can facilitate this process, enabling organizations to efficiently manage and utilize enriched datasets.
In conclusion, leveraging third-party data sources for data enrichment presents a powerful opportunity for organizations to enhance their insights and drive strategic decision-making. By augmenting internal datasets with external information, organizations can achieve a more comprehensive understanding of their environment, improve predictive analytics, and ultimately gain a competitive edge. However, to fully realize these benefits, organizations must address challenges related to data privacy, quality, and integration, ensuring that their data enrichment efforts are both effective and compliant.
Best Practices for Data Cleansing and Standardization
Data enrichment is a critical process in the realm of data management, serving as a cornerstone for organizations aiming to enhance the quality and utility of their data assets. As businesses increasingly rely on data-driven decision-making, the importance of data cleansing and standardization cannot be overstated. These practices ensure that data is accurate, consistent, and ready for enrichment, ultimately leading to more informed business strategies and improved operational efficiency.
To begin with, data cleansing involves identifying and rectifying errors and inconsistencies within a dataset. This process is essential for eliminating inaccuracies that could skew analysis and lead to misguided conclusions. Common issues addressed during data cleansing include duplicate records, incomplete fields, and incorrect data entries. By employing automated tools and algorithms, organizations can efficiently detect and correct these anomalies, thereby enhancing the reliability of their data.
Following data cleansing, standardization plays a pivotal role in ensuring that data is uniform and comparable across different sources. This involves converting data into a consistent format, which is particularly important when integrating data from multiple systems. For instance, standardizing date formats, measurement units, and categorical values can significantly reduce confusion and errors during data analysis. Moreover, standardization facilitates seamless data integration, enabling organizations to create a comprehensive view of their operations and customer interactions.
Once data has been cleansed and standardized, it is primed for enrichment. Data enrichment involves augmenting existing data with additional information from external sources, thereby providing a more complete and nuanced understanding of the dataset. This process can include appending demographic information, geographic data, or behavioral insights, which can enhance the depth and breadth of analysis. For example, enriching customer data with social media activity or purchasing behavior can offer valuable insights into consumer preferences and trends.
To effectively implement data enrichment, organizations should adopt a strategic approach that aligns with their specific business objectives. This begins with clearly defining the goals of the enrichment process, such as improving customer segmentation, enhancing predictive analytics, or optimizing marketing campaigns. By establishing clear objectives, organizations can focus their efforts on acquiring the most relevant and impactful data.
Furthermore, selecting the right data sources is crucial for successful enrichment. Organizations should evaluate potential data providers based on the quality, accuracy, and relevance of their data offerings. Partnering with reputable data vendors can ensure that the enriched data is reliable and adds genuine value to the organization’s existing datasets.
In addition to selecting appropriate data sources, organizations must also consider the ethical implications of data enrichment. This includes ensuring compliance with data privacy regulations and obtaining necessary consent from individuals whose data is being used. By prioritizing ethical data practices, organizations can build trust with their customers and stakeholders, thereby fostering a positive reputation and mitigating potential legal risks.
In conclusion, effective data enrichment hinges on robust data cleansing and standardization practices. By ensuring that data is accurate, consistent, and ethically sourced, organizations can unlock the full potential of their data assets. This not only enhances decision-making capabilities but also drives innovation and competitive advantage in an increasingly data-driven world. As such, investing in comprehensive data management strategies is essential for organizations seeking to thrive in today’s dynamic business environment.
Integrating Machine Learning for Advanced Data Enrichment
In the rapidly evolving landscape of data management, the integration of machine learning for advanced data enrichment has emerged as a pivotal strategy for organizations seeking to enhance the value of their data assets. As businesses increasingly rely on data-driven insights to inform decision-making, the need for enriched data that provides deeper, more actionable insights has become paramount. Machine learning, with its ability to analyze vast amounts of data and identify patterns, offers a powerful tool for achieving this enrichment.
To begin with, machine learning algorithms can be employed to automate the process of data enrichment, thereby reducing the time and resources traditionally required for manual data processing. By leveraging supervised learning techniques, organizations can train models on labeled datasets to predict and fill in missing information, thus enhancing the completeness and accuracy of their data. This approach not only streamlines data management processes but also ensures that the enriched data is consistent and reliable.
Moreover, unsupervised learning methods, such as clustering and association rule learning, can be utilized to uncover hidden relationships within datasets. These techniques enable organizations to identify patterns and correlations that may not be immediately apparent, providing a more nuanced understanding of the data. For instance, clustering algorithms can group similar data points together, revealing insights into customer segmentation or product categorization that can inform targeted marketing strategies.
In addition to these methods, natural language processing (NLP) plays a crucial role in enriching unstructured data, such as text from social media, customer reviews, or support tickets. By applying NLP techniques, organizations can extract meaningful information from text data, such as sentiment analysis or topic modeling, which can then be integrated with structured data to provide a more comprehensive view of customer opinions and behaviors. This enriched data can be instrumental in shaping customer experience strategies and improving service delivery.
Furthermore, the integration of machine learning for data enrichment is not without its challenges. One significant concern is the quality of the input data, as machine learning models are only as good as the data they are trained on. Therefore, it is essential to ensure that the data used for training is clean, accurate, and representative of the real-world scenarios the model will encounter. Additionally, organizations must be mindful of potential biases in the data, which can lead to skewed results and perpetuate existing inequalities.
To address these challenges, organizations should implement robust data governance frameworks that include regular audits and validation checks to maintain data quality. Moreover, incorporating explainable AI techniques can help demystify the decision-making processes of machine learning models, allowing stakeholders to understand and trust the enriched data outputs.
In conclusion, integrating machine learning for advanced data enrichment offers a transformative approach to unlocking the full potential of organizational data. By automating enrichment processes, uncovering hidden patterns, and extracting insights from unstructured data, machine learning enables businesses to derive more value from their data assets. However, to fully realize these benefits, organizations must prioritize data quality and transparency, ensuring that their machine learning initiatives are both effective and ethical. As the field of data science continues to advance, the strategic integration of machine learning for data enrichment will undoubtedly remain a cornerstone of successful data management practices.
Ensuring Data Privacy and Compliance in Enrichment Processes
In the realm of data enrichment, ensuring data privacy and compliance is paramount. As organizations increasingly rely on enriched data to drive decision-making and enhance customer experiences, they must navigate a complex landscape of privacy regulations and ethical considerations. The process of data enrichment involves augmenting existing data with additional information from external sources, thereby increasing its value and utility. However, this process also introduces potential risks related to data privacy and compliance, necessitating the implementation of effective strategies to mitigate these concerns.
To begin with, understanding the legal framework governing data privacy is crucial. Regulations such as the General Data Protection Regulation (GDPR) in Europe and the California Consumer Privacy Act (CCPA) in the United States set stringent requirements for data handling and protection. Organizations must ensure that their data enrichment processes comply with these regulations to avoid hefty fines and reputational damage. This involves obtaining explicit consent from individuals before using their data for enrichment purposes and providing them with clear information about how their data will be used.
Moreover, data minimization is a key principle that organizations should adhere to during the enrichment process. This principle dictates that only the data necessary for a specific purpose should be collected and processed. By limiting the amount of data used in enrichment, organizations can reduce the risk of privacy breaches and ensure compliance with data protection regulations. Additionally, implementing robust data anonymization techniques can further protect individual privacy. Anonymization involves removing or altering personally identifiable information (PII) so that individuals cannot be readily identified, thus safeguarding their privacy while still allowing for valuable insights to be gleaned from the data.
Furthermore, organizations should establish comprehensive data governance frameworks to oversee their data enrichment activities. These frameworks should include policies and procedures for data access, usage, and sharing, ensuring that all data handling practices align with legal and ethical standards. Regular audits and assessments of data enrichment processes can help identify potential compliance gaps and areas for improvement. By fostering a culture of accountability and transparency, organizations can build trust with stakeholders and demonstrate their commitment to data privacy.
In addition to internal measures, collaborating with reputable third-party data providers is essential for maintaining data privacy and compliance. Organizations should conduct thorough due diligence when selecting data partners, ensuring that they adhere to high standards of data protection and ethical practices. Establishing clear contractual agreements that outline data usage, security measures, and compliance obligations can further safeguard against potential privacy breaches.
Finally, investing in employee training and awareness programs is vital for ensuring that all staff members understand the importance of data privacy and compliance in the enrichment process. By equipping employees with the knowledge and skills needed to handle data responsibly, organizations can minimize the risk of human error and enhance their overall data protection efforts.
In conclusion, while data enrichment offers significant benefits, it also presents challenges related to data privacy and compliance. By understanding the legal landscape, adhering to data minimization principles, establishing robust governance frameworks, collaborating with trustworthy partners, and investing in employee training, organizations can effectively navigate these challenges. Ultimately, these strategies not only protect individual privacy but also enhance the integrity and value of enriched data, enabling organizations to leverage it responsibly and ethically.
Measuring the Impact of Data Enrichment on Business Outcomes
Data enrichment has emerged as a pivotal strategy for businesses aiming to enhance their decision-making processes and improve overall outcomes. By augmenting existing data with additional information, organizations can gain deeper insights into customer behavior, market trends, and operational efficiencies. However, to truly harness the power of data enrichment, it is essential to measure its impact on business outcomes effectively. This measurement not only validates the investment in data enrichment but also guides future strategies for data utilization.
To begin with, one of the primary methods to measure the impact of data enrichment is through key performance indicators (KPIs). By establishing clear KPIs before implementing data enrichment strategies, businesses can track changes and improvements over time. For instance, if a company enriches its customer data to include demographic information, it can monitor changes in customer engagement metrics, such as click-through rates or conversion rates. These metrics provide tangible evidence of how enriched data can lead to more targeted marketing efforts and, consequently, better business outcomes.
Moreover, another effective strategy is conducting A/B testing. By comparing outcomes from enriched data sets against those from non-enriched data sets, businesses can directly observe the benefits of data enrichment. This method allows for a controlled environment where variables are minimized, ensuring that any observed differences in outcomes can be attributed to the data enrichment process. For example, a retail company might use enriched data to personalize email marketing campaigns for one group of customers while sending generic emails to another. By analyzing the results, the company can determine the effectiveness of data enrichment in driving sales and customer engagement.
In addition to these methods, businesses should also consider the qualitative impact of data enrichment. While quantitative metrics are crucial, qualitative insights can provide a more nuanced understanding of how data enrichment influences business outcomes. Customer feedback, employee testimonials, and case studies can offer valuable perspectives on the effectiveness of data enrichment strategies. For instance, sales teams might report that enriched data has improved their ability to identify and prioritize high-value leads, leading to more efficient sales processes and better customer relationships.
Furthermore, it is important to recognize that the impact of data enrichment may vary across different departments and functions within an organization. Therefore, a comprehensive approach to measurement should involve collaboration across various teams. By engaging stakeholders from marketing, sales, operations, and IT, businesses can ensure that the benefits of data enrichment are realized across the entire organization. This collaborative approach not only facilitates a more holistic assessment of data enrichment’s impact but also fosters a culture of data-driven decision-making.
Finally, as businesses continue to evolve in an increasingly data-driven world, it is crucial to remain adaptable and open to new methods of measuring the impact of data enrichment. Emerging technologies, such as artificial intelligence and machine learning, offer new opportunities for analyzing enriched data and deriving insights. By staying abreast of these advancements, businesses can refine their measurement strategies and continue to optimize their data enrichment efforts.
In conclusion, measuring the impact of data enrichment on business outcomes is a multifaceted process that requires a combination of quantitative and qualitative approaches. By leveraging KPIs, A/B testing, and cross-departmental collaboration, businesses can effectively assess the value of their data enrichment strategies. As technology continues to advance, organizations must remain agile and innovative in their measurement techniques to fully realize the potential of enriched data in driving business success.
Q&A
1. **What is data enrichment?**
Data enrichment is the process of enhancing existing data by adding additional information from external sources, improving its quality, accuracy, and value.
2. **Why is data enrichment important?**
Data enrichment is crucial because it provides a more comprehensive view of customers, improves decision-making, enhances personalization, and increases the effectiveness of marketing and sales strategies.
3. **What are some common data enrichment techniques?**
Common techniques include demographic enrichment, firmographic enrichment, behavioral data integration, and geographic data enhancement.
4. **How can machine learning be used in data enrichment?**
Machine learning can automate the process of identifying patterns and correlations in data, predict missing values, and classify data into meaningful categories, thus enhancing the enrichment process.
5. **What are the challenges of data enrichment?**
Challenges include data privacy concerns, ensuring data accuracy and consistency, integrating data from multiple sources, and managing the cost and complexity of enrichment processes.
6. **What tools are commonly used for data enrichment?**
Tools like Clearbit, ZoomInfo, InsideView, and Salesforce Data.com are commonly used for data enrichment, providing access to vast databases and integration capabilities.Effective data enrichment strategies are crucial for enhancing the value and utility of data within an organization. These strategies involve augmenting existing data with additional information to provide deeper insights, improve decision-making, and enhance customer experiences. Key strategies include leveraging third-party data sources to fill gaps and provide context, using machine learning algorithms to identify patterns and predict trends, and employing data integration techniques to ensure seamless data flow across systems. Additionally, maintaining data quality through regular cleansing and validation processes is essential to ensure accuracy and reliability. By implementing these strategies, organizations can transform raw data into a powerful asset that drives innovation and competitive advantage.
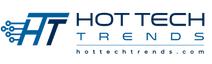